Uncovering the Depths of Data Science: A Comprehensive Guide
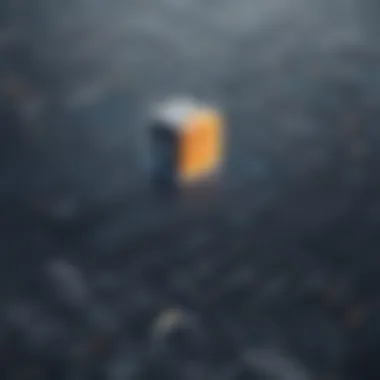
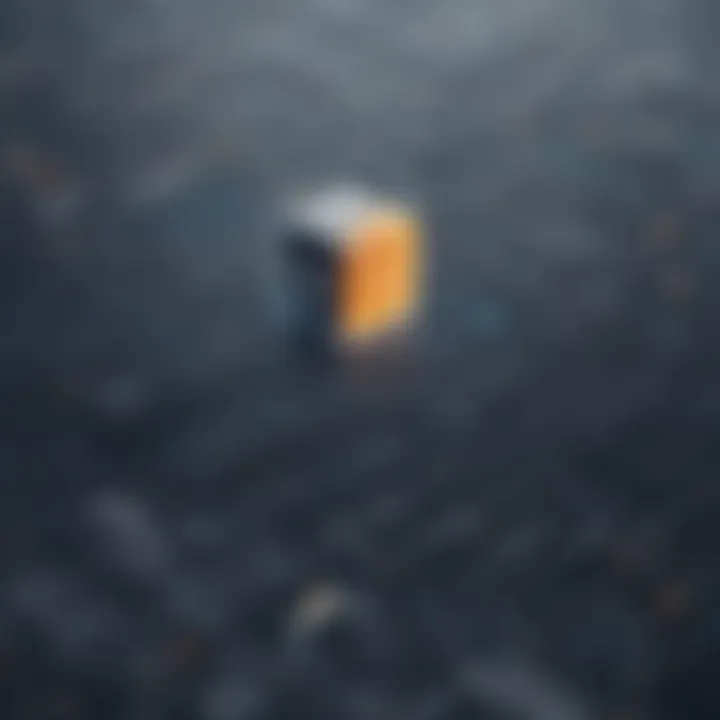
Tech Trend Analysis
In this section, we will delve into the current trend of data science, offering an insightful overview of its evolution in the modern tech landscape. Data science has emerged as a pivotal aspect of technology, revolutionizing how businesses operate and make decisions. The implications for consumers are significant, with personalized recommendations, predictive analytics, and targeted advertisements becoming more prevalent. Looking into the future, data science is poised to further enhance user experiences, refine business strategies, and drive innovation across industries.
Product Reviews
When reviewing data science as a product of technological advancement, we must consider its features and specifications. Data science tools enable organizations to extract valuable insights from vast data sets, enhancing decision-making processes and forecasting trends. Through performance analysis, it is evident that data science can optimize operations, boost efficiency, and uncover hidden patterns. However, like any product, there are pros and cons to using data science, such as its high initial implementation costs versus the long-term benefits it provides. Overall, a recommendation for businesses is to invest in data science solutions tailored to their specific needs and objectives.
How-To Guides
To provide a comprehensive understanding of data science, this section will offer a step-by-step guide on its principles and applications. Starting with an introduction to the topic, readers will learn about the core concepts of data science, including data collection, analysis, and interpretation. The step-by-step instructions will cover methodologies such as machine learning, data visualization, and statistical modeling, essential for leveraging data science effectively. Additionally, tips and tricks for optimizing data science processes and troubleshooting common issues will be highlighted, empowering readers with practical knowledge and skills in the field.
Industry Updates
Data science continues to shape the tech industry, with recent developments driving innovation and transformation. Analyzing market trends reveals the growing demand for data scientists, data analysts, and AI specialists across various sectors. These shifts have a significant impact on businesses, as those embracing data science gain a competitive edge through enhanced decision-making and targeted marketing strategies. For consumers, industry updates translate into improved services, individualized products, and a more personalized online experience, reflecting the pervasive influence of data science in today's digital economy.
Introduction to Data Science
Data science, a pivotal field in today's tech landscape, serves as the bedrock for data-driven decision-making and technological advancements. In this enlightening guide, we delve into the core principles and methodologies that define data science's essence. By unraveling the dynamic role of data scientists and the intricacies of big data analytics, we aim to provide a holistic understanding of this evolving domain.
Defining Data Science
The Evolution of Data Science
The evolution of data science traces back to its inception, highlighting the progression from traditional methods to sophisticated data analysis techniques. This evolution signifies a paradigm shift in how we interpret and utilize data, paving the way for innovative solutions and insights. The adaptability and scalability of modern data science approaches make it a vital component of today's tech discourse, driving efficiency and intelligence in decision-making processes.
The Interdisciplinary Nature of Data Science
The interdisciplinary nature of data science emphasizes its convergence of various domains such as statistics, computer science, and domain expertise. This intermingling of disciplines enriches data science by fostering diverse perspectives and approaches to problem-solving. As a result, data scientists can leverage a broad spectrum of knowledge to tackle complex real-world challenges and extract meaningful insights from data repositories.
The Core Components of Data Science
At the heart of data science lie its core components, including data collection, data processing, data analysis, and interpretation. These fundamental pillars form the backbone of every data science endeavor, guiding professionals in harnessing the power of data for strategic decision-making. By understanding and honing these components, data scientists can extract valuable knowledge from raw data, enabling them to drive innovation and progress in their respective fields.
Importance of Data Science
Driving Data-Driven Decision-Making
Data science plays a pivotal role in steering organizations towards data-driven decision-making, underpinned by insights extracted from complex datasets. By utilizing advanced analytical tools and predictive algorithms, data scientists empower businesses to make informed choices based on empirical evidence rather than intuition. This shift towards data-backed decision-making enhances operational efficiency and competitiveness, setting organizations on a trajectory towards sustained success.
Transforming Businesses Through Insights
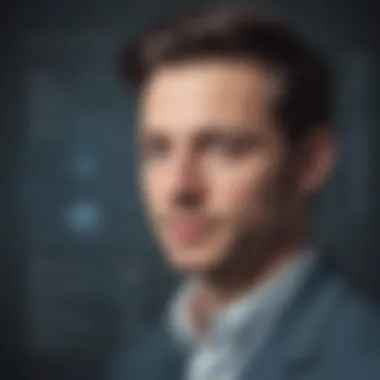
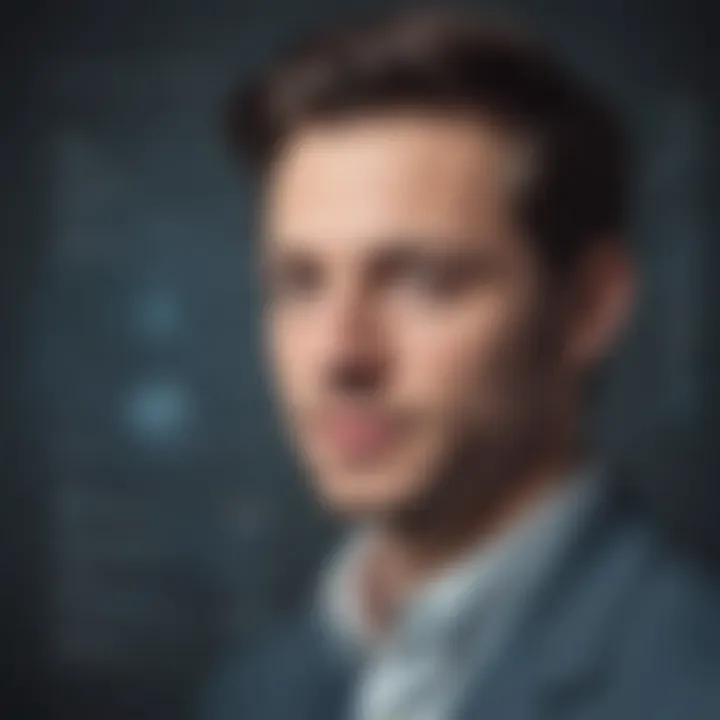
The transformative power of data science lies in its ability to unearth actionable insights that drive business growth and development. Through advanced analytics and trend forecasting, companies can optimize their operations, streamline processes, and identify new opportunities for expansion. The strategic integration of data science frameworks empowers businesses to capitalize on market trends and consumer preferences, ultimately fostering innovation and adaptability.
Impact on Technological Advancements
Data science serves as a catalyst for technological advancements, fueling innovation across various industries and sectors. By harnessing the power of machine learning algorithms and predictive models, organizations can revolutionize product development, enhance user experiences, and optimize resource allocation. The marriage of data science and technology ushers in a new era of discovery and efficiency, shaping the future of digital transformation and technological progress.
Key Concepts in Data Science
Data science plays a pivotal role in today's technological landscape. Analyzing and interpreting vast amounts of data have become essential for businesses to make informed decisions. In this article, we delve deep into the key concepts of data science, shedding light on its fundamental principles, methodologies, and applications. Understanding these concepts is crucial for driving innovation, fostering growth, and gaining a competitive edge in the modern data-driven world.
Big Data Analytics
Understanding big data
The concept of understanding big data lies at the core of data science. It involves processing and making sense of massive volumes of data to extract valuable insights. The key characteristic of understanding big data is its scalability, allowing organizations to analyze diverse data types efficiently. This aspect is fundamental for this article as it underscores the importance of effectively managing and leveraging substantial datasets. However, one challenge of understanding big data is ensuring data accuracy and avoiding bias in analysis.
Analyzing large-scale data sets
Analyzing large-scale data sets is a critical aspect of big data analytics. It enables businesses to identify trends, patterns, and correlations within extensive datasets. The key characteristic of this process is its ability to uncover hidden insights that can drive strategic decision-making. In the context of this article, analyzing large-scale data sets is vital for showcasing the breadth and depth of data science applications. Despite its advantages in revealing valuable information, analyzing large-scale data sets may pose challenges in terms of data processing speed and resource utilization.
Tools and techniques in big data analytics
Tools and techniques in big data analytics are indispensable for streamlining data processing and analysis tasks. From data visualization platforms to machine learning algorithms, these tools enhance the efficiency and accuracy of data-driven processes. The key characteristic of these tools is their ability to handle diverse data formats and perform complex computations seamlessly. In this article, highlighting various tools and techniques in big data analytics is essential for illustrating the practical applications of data science. Nonetheless, the variety of tools available may present a learning curve for users and require continuous updates to stay relevant in a rapidly evolving field.
Machine Learning
Fundamentals of machine learning
The fundamentals of machine learning form the backbone of data science algorithms. They encompass the principles and techniques used to train algorithms to recognize patterns and make predictions based on data. The key characteristic of these fundamentals is their adaptability to varying datasets and problem domains. Understanding the fundamentals of machine learning is crucial for emphasizing the role of predictive analytics and decision-making in data science. However, implementing machine learning models effectively may require substantial computational resources and domain expertise.
Supervised and unsupervised learning
Supervised and unsupervised learning are two primary paradigms in machine learning. Supervised learning involves training algorithms on labeled data to predict outcomes, while unsupervised learning focuses on finding patterns and structures in unlabeled data. The key characteristic of these approaches is their distinct methodologies for extracting meaningful information from datasets. Discussing supervised and unsupervised learning in this article is paramount for showcasing the diversity of machine learning applications. Nonetheless, selecting the most suitable learning approach for a specific task can be challenging due to the need for high-quality labeled data and selecting appropriate algorithms.
Applications of machine learning in data science
The applications of machine learning in data science span a wide range of industries and use cases. From recommendation systems to predictive modeling, machine learning algorithms drive innovation and efficiency in various domains. The key characteristic of these applications is their ability to automate decision-making processes and adapt to changing environments. Exploring the practical applications of machine learning in this article is essential for highlighting its transformative impact on businesses and society. Nevertheless, deploying machine learning models requires rigorous testing and validation to ensure accurate results and avoid biased outcomes.
Roles in Data Science
Exploring the critical aspect of Roles in Data Science within the comprehensive guide of Unveiling Data Science, delves into the fundamental pillars that support the data ecosystem. By spotlighting the distinct functions and responsibilities in this realm, readers gain a nuanced understanding of the significance of roles within the data science domain. Emphasizing the specific elements, benefits, and considerations surrounding Roles in Data Science provides a holistic viewpoint on the essential role played by individuals in this field.
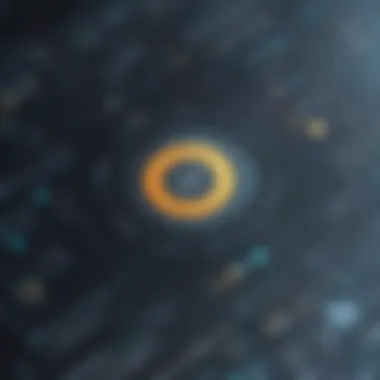
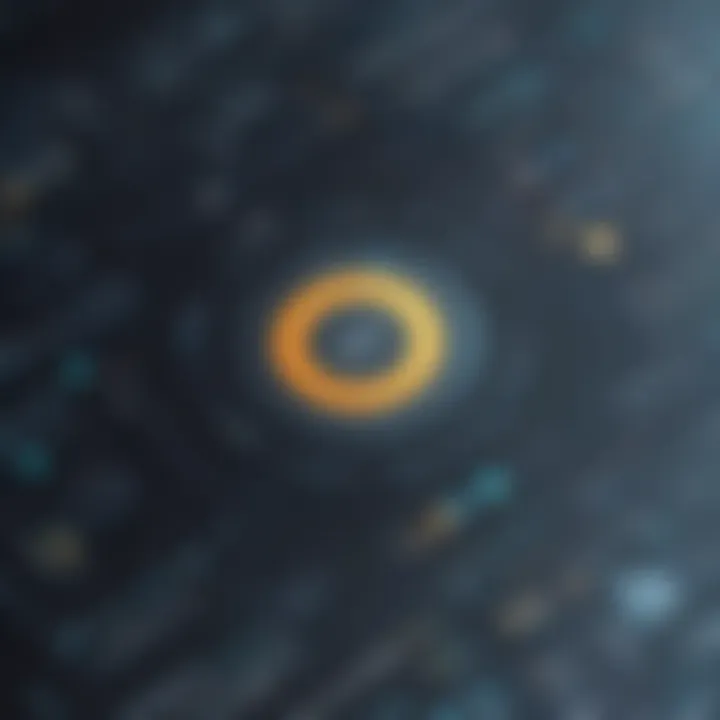
Data Scientist
Skills required for data scientists
The expertise demanded of data scientists plays a pivotal role in shaping the outcomes of data-driven projects. Proficiency in statistical analysis, programming languages like Python and R, and data visualization tools are quintessential for navigating the complexities of data. These skills are essential for interpreting data patterns, developing predictive models, and extracting actionable insights to drive informed decision-making processes within organizations. Acquiring these skills not only amplifies the data scientist's analytical capabilities but also enhances their problem-solving acumen, making them indispensable assets in today's data-centric world.
Responsibilities and duties
The responsibilities shoulder by data scientists extend beyond mere data analysis; they encompass the entire lifecycle of a data project. From understanding business objectives to collecting, cleaning, and processing data, data scientists are entrusted with ensuring data quality and reliability. Additionally, they are responsible for developing machine learning models, conducting in-depth analysis, and presenting findings to non-technical stakeholders. This multifaceted role necessitates strong communication skills, strategic thinking, and a deep understanding of business processes to drive data initiatives successfully.
Career prospects in data science
The field of data science offers a plethora of opportunities for career growth and advancement. With the increasing demand for data professionals across industries, data science roles continue to be highly sought after. By acquiring a diverse skill set that combines technical expertise with business acumen, data scientists can explore various career pathways in fields such as healthcare, finance, e-commerce, and more. The versatility of data science careers enables professionals to transition into specialized roles like data architect, data engineer, or machine learning engineer, ensuring continuous learning and professional development.
Data Engineer
Role in data infrastructure
Data engineers play a critical role in building and maintaining a robust data infrastructure that supports data-intensive applications. By designing and implementing data pipelines, data engineers facilitate the flow of data from source to destination, ensuring data accessibility and reliability. Their expertise in database management systems, ETL processes, and cloud technologies enables organizations to streamline data operations efficiently.
Data processing and management
The core responsibility of data engineers lies in processing and managing large volumes of data effectively. By implementing data processing frameworks like Apache Hadoop or Spark, data engineers can ingest, transform, and store data efficiently for analysis. This meticulous process ensures data quality, consistency, and performance, essential for deriving actionable insights and facilitating data-driven decision-making within organizations.
Collaboration with data scientists
Collaboration between data engineers and data scientists forms the bedrock of successful data projects. By fostering strong communication channels, data engineers enable seamless collaboration with data scientists to understand project requirements, iterate on data models, and deploy solutions effectively. This synergy between technical expertise and domain knowledge cultivates a dynamic environment where data projects thrive, driving innovation and business growth.
Applications of Data Science
In this ultimate guide on data science, an extensive exploration of the crucial topic of Applications of Data Science is undertaken. This segment delves into the pivotal role of data science in modern technology, emphasizing its practical applications and enduring significance in the rapidly evolving digital landscape. By shedding light on various elements such as Predictive Analytics, Data Visualization, and others, this guide aims to provide tech enthusiasts and industry professionals with an in-depth understanding of how data science drives innovation and informs strategic decision-making processes.
Predictive Analytics
Forecasting trends and patterns
Forecasting trends and patterns plays a paramount role within the domain of data science by enabling organizations to anticipate future developments and proactively adjust their strategies. The uniqueness of this aspect lies in its ability to analyze historical data trends and extrapolate insights to forecast potential outcomes accurately. By leveraging statistical algorithms and machine learning techniques, forecasting trends and patterns empowers businesses to make informed decisions, minimize risks, and capitalize on emerging opportunities. Despite its benefits, challenges such as data accuracy and model complexity exist, which require continuous refinement and validation to enhance the reliability of predictive analytics.
Risk assessment and fraud detection
Risk assessment and fraud detection represent critical components of data science, safeguarding organizations against potential threats and financial losses. The essence of this aspect lies in its capability to identify anomalies, detect fraudulent activities, and mitigate risks in real-time. By employing advanced algorithms and anomaly detection mechanisms, risk assessment and fraud detection algorithms enhance security measures and preserve the integrity of data assets. However, the evolving nature of fraudulent tactics necessitates ongoing enhancements and adaptive strategies to counter new challenges and ensure robust security protocols.

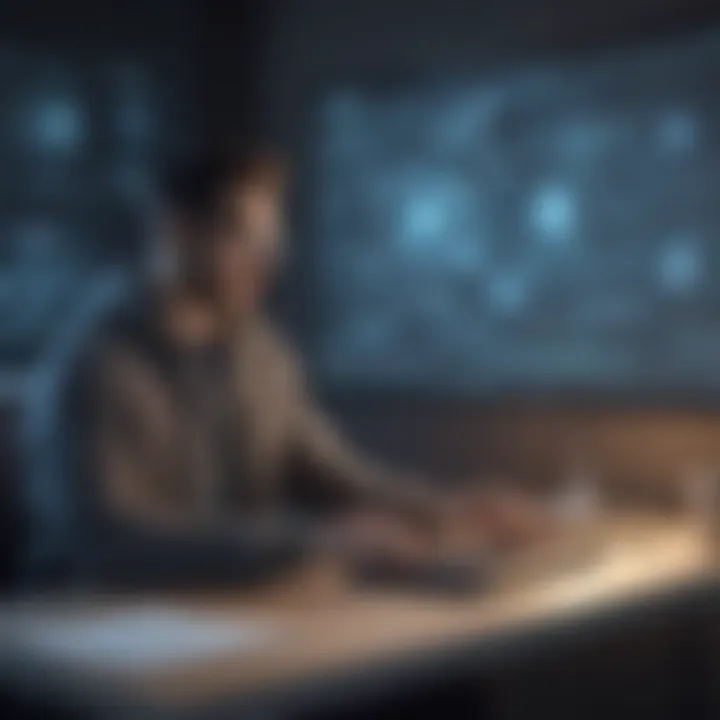
Personalization in marketing and services
Personalization in marketing and services revolutionizes customer engagement strategies by tailoring products and services to individual preferences and trends. This aspect excels in offering personalized recommendations, targeted advertisements, and customized experiences that enhance customer satisfaction and loyalty. The key feature of personalization lies in its ability to leverage customer data to create unique user profiles and deliver hyper-personalized offerings. While personalization drives customer retention and increases conversion rates, privacy concerns and data protection regulations underscore the importance of ethical data usage and consent management in marketing practices.
Data Visualization
Visual representation of data
Data visualization plays a pivotal role in data science by translating complex datasets into visually compelling graphics and charts that facilitate data interpretation and decision-making. The significance of this aspect lies in its capacity to present information in a clear, concise manner, enabling stakeholders to gain actionable insights and identify trends swiftly. The unique feature of visual representation of data is its ability to transform raw data into intuitive visual narratives that enhance comprehension and analytical capabilities. Although data visualization enhances data-driven storytelling and facilitates communication, design choices and visual clarity influence the effectiveness of conveying insights to diverse audiences.
Interactive dashboards and tools
Interactive dashboards and tools empower users with dynamic interfaces and real-time data interactions, fostering proactive decision-making and performance monitoring. This feature excels in providing customizable visualization options, interactive elements, and drill-down capabilities that enhance user engagement and analytical flexibility. The key characteristic of interactive dashboards lies in their capacity to offer customizable views, filters, and interactivity that enable users to explore data trends and patterns effortlessly. While interactive dashboards enhance data accessibility and user experience, designing intuitive interfaces and ensuring data accuracy are crucial for maintaining usability and data integrity.
Enhancing decision-making processes
Enhancing decision-making processes through data visualization tools promotes informed and data-driven strategies by presenting insights in a visually appealing and informative format. This aspect contributes to improved decision quality, increased operational efficiency, and strategic alignment across diverse business functions. The unique feature of enhancing decision-making processes is its ability to synthesize complex information into actionable intelligence, enabling stakeholders to make timely decisions based on data-driven evidence. Despite its advantages in streamlining decision-making workflows and fostering collaboration, user training and data governance frameworks are essential for maximizing the value of data visualization tools and optimizing decision outcomes.
Challenges and Future Trends
Data science is a field constantly evolving, presenting new challenges and exploring future trends that shape various industries today. In this section, we delve into the pivotal aspects driving innovation and transformation in the realm of data science.
Ethical Considerations in Data Science
Privacy and Data Security
Privacy and data security play a crucial role in the ethical considerations of data science. Safeguarding sensitive information and ensuring data protection are paramount in the age of digitalization. Data privacy regulations such as GDPR emphasize the importance of ethical data handling practices. While privacy enhances user trust and maintains confidentiality, data security shields against cyber threats, ensuring the integrity of information.
Algorithmic Bias and Fairness
Algorithmic bias and fairness are significant considerations in data science ethics. Addressing bias in data models is essential to prevent discriminatory outcomes. Striving for fairness in algorithm design enhances inclusivity and equity in decision-making processes. Despite challenges, mitigating bias leads to more transparent and accurate results, aligning with ethical standards and promoting diversity.
Regulatory Compliance
Regulatory compliance is a critical aspect of operating in the data science landscape. Adhering to laws and standards ensures ethical data practices and accountability. Compliance frameworks like HIPAA and PCI DSS establish guidelines for secure data handling. While compliance may pose challenges, it fosters trust among stakeholders and upholds legal responsibilities, ultimately enhancing data integrity and organizational reputation.
Emerging Technologies
IoT and Data Integration
The integration of IoT devices with data systems revolutionizes data collection and analysis. IoT enhances real-time data tracking and aids in decision-making processes through interconnected devices. Leveraging IoT technology enables enhanced operational efficiency and predictive analytics. Despite concerns about data security and scalability, IoT integration offers valuable insights and empowers data-driven strategies.
Blockchain in Data Verification
Blockchain technology enhances data verification and immutability in data science applications. Utilizing decentralized ledgers ensures transparent and secure data transactions. The distributed nature of blockchain mitigates risks of data tampering and unauthorized access. By incorporating blockchain in data validation, organizations secure data integrity and establish trust in data exchanges, reinforcing the credibility of information.
AI Advancements in Data Processing
AI advancements drive efficient data processing and intricate pattern recognition capabilities. Machine learning algorithms optimize data analysis and automate tasks, enhancing data processing speeds and accuracy. AI-powered tools like natural language processing revolutionize data interpretation and decision support systems. While challenges in AI ethics and interpretability persist, embracing AI advancements empowers organizations to leverage data effectively and gain competitive advantages in the dynamic tech landscape.