Unraveling the Complexities of a Random Dataset: A Deep Dive into Insights
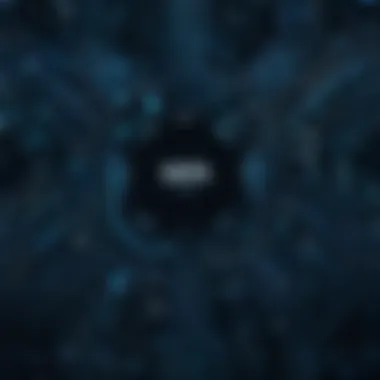
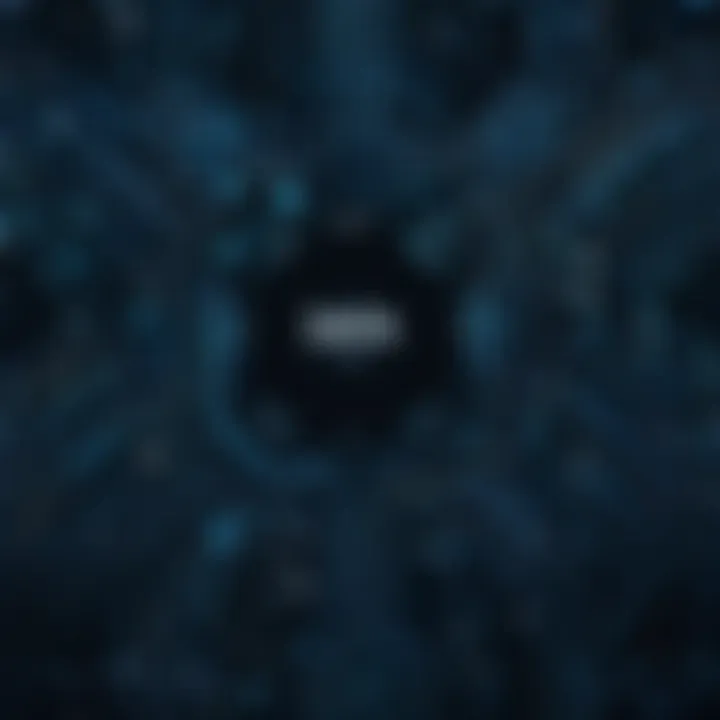
Tech Trend Analysis
In the realm of data analysis, trends play a vital role in shaping methodologies and insights. Understanding the current trend in data exploration is crucial for tech enthusiasts and industry professionals seeking innovative solutions. By examining the prevalent patterns in data utilization and analysis techniques, one can gain a competitive edge in harnessing the potential of random datasets to uncover hidden gems that offer transformative value.
Product Reviews
When venturing into the maze of a random dataset, it's akin to exploring a new product in the tech market. Just like a diligent consumer researching a product, delving into a dataset requires a thorough examination of its features and specifications. Analyzing performance metrics becomes the compass guiding your journey through the dataset. By weighing the pros and cons of each analytical approach, one can determine the most efficient and effective method to glean valuable insights. Ultimately, a well-rounded recommendation based on comprehensive review and analysis is essential for maximizing the dataset's potential.
How-To Guides
Embarking on the exploration of a random dataset necessitates a structured approach resembling a detailed how-to guide. With a clear introduction to the dataset's scope and objectives, setting the foundation for data analysis becomes paramount. Step-by-step instructions on data collection, preprocessing, analysis, and visualization serve as building blocks for an in-depth exploration. Offering tips and tricks along the way enhances the efficiency and accuracy of the analysis, while troubleshooting common challenges ensures a smooth and successful data exploration process.
Industry Updates
Keeping abreast of the latest technological advancements and market trends is essential for industry professionals navigating the ever-evolving tech landscape. Recent developments in data analysis tools and methodologies hold significant implications for businesses and consumers alike. Through meticulous analysis of market trends, one can anticipate potential impacts on industry practices and consumer behaviors. By synthesizing these insights with the exploration of random datasets, one can gain a holistic understanding of how industry updates shape the landscape of data analysis and interpretation.
Introduction
In the realm of data exploration and analysis, the significance of an adept introduction cannot be overstated. It serves as the gateway to unveiling the hidden treasures of a random dataset, setting the stage for the intricate journey that lies ahead. An effective introduction not only piques the curiosity of the audience but also provides a roadmap for the exploration that follows. Within the context of this article, delving into the depths of a random dataset demands a well-crafted introduction that shines a light on the complexities and insights waiting to be unearthed. This section sets the tone for what lies ahead, capturing the essence of data exploration and analysis.
Understanding the Significance of Data
Data as the Foundation of Insights
Data as the foundation of insights plays a pivotal role in driving meaningful outcomes from any dataset under scrutiny. It forms the bedrock upon which conclusions are drawn and decisions are made, acting as a fundamental building block in the realm of data analysis. The unique characteristic of data as the foundation of insights lies in its ability to transform raw information into actionable knowledge, making it an indispensable asset in the process of unraveling the intricacies of a random dataset. While its advantages are abundant, one must also carefully navigate potential shortcomings such as data bias or inaccuracies that could skew the analysis results. Therefore, understanding and leveraging the power of data as the foundation of insights is essential for extracting valuable conclusions from any dataset.
The Role of Data in Decision Making
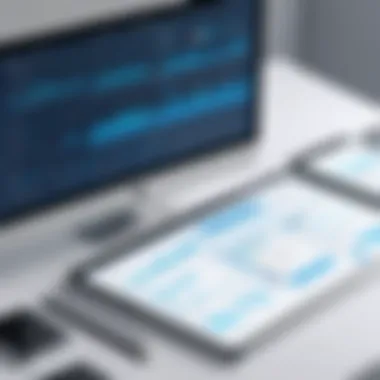
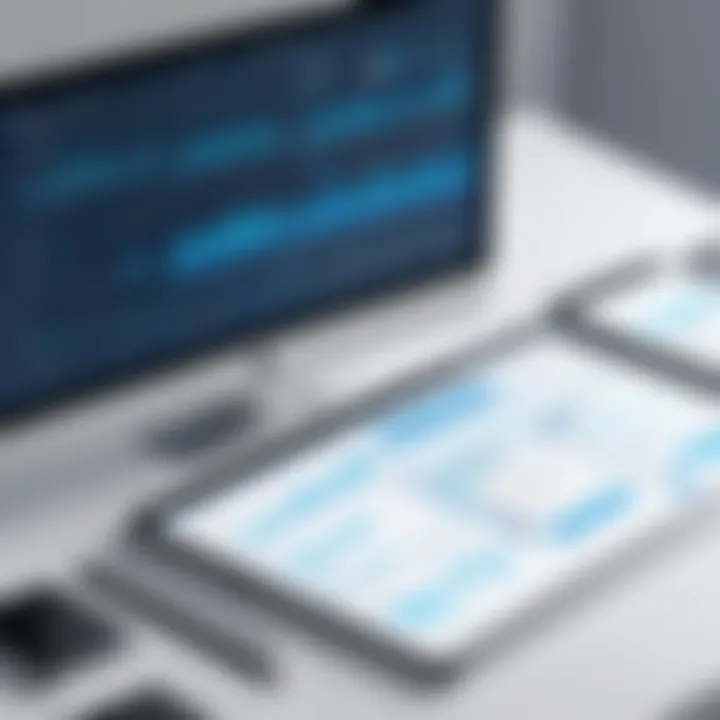
The role of data in decision making is paramount, particularly when traversing through a random dataset teeming with information waiting to be deciphered. Data plays a crucial function in providing the necessary insights and evidence required to make informed decisions across various industries and domains. Its key characteristic lies in its ability to offer a factual basis for decision-making processes, reducing uncertainties and enhancing precision in choice selection. Embracing the role of data in decision making within the context of exploring a random dataset opens doors to opportunities for discovery and innovation. However, it is imperative to acknowledge that while data illuminates the path forward, it also poses challenges such as information overload or misinterpretation, which must be managed effectively to derive accurate conclusions. Consequently, acknowledging and embracing the role of data in decision making is essential for navigating the complexities of a random dataset with precision and clarity.
Data Collection
Methods and Techniques
Traditional Approaches
Traditional approaches in data collection are time-tested methods that have been utilized for generations to gather information. Their contribution to the overall goal of understanding a random dataset is substantial due to their reliability and simplicity. The key characteristic of traditional approaches lies in their ability to collect data through manual means, such as surveys, interviews, or observational studies. This hands-on approach ensures direct interaction with the subjects, leading to rich and contextual data. One of the advantages of traditional approaches is their high level of control over the data collection process, allowing for targeted responses and in-depth insights. However, a notable disadvantage is the potential for bias or errors introduced during manual data collection, which must be carefully managed to ensure the reliability of the dataset.
Modern Data Collection Tools
In contrast, modern data collection tools leverage technology to streamline and enhance the data collection process for exploring random datasets. Their contribution to the overarching goal of data analysis is marked by efficiency and scalability. The key characteristic of modern data collection tools is their automation and digitization of data gathering, using tools such as online surveys, sensors, or web scraping. This automation accelerates the data collection process, enabling the handling of large volumes of data with ease. A significant advantage of modern tools is their ability to capture real-time data and responses, providing instant insights for analysis. However, a potential disadvantage lies in the reliance on technology, which may introduce complexities or technical challenges that require expertise to address effectively.
Data Cleaning
In the realm of data exploration, the significance of data cleaning cannot be overstated. Data cleaning, also known as data cleansing or data scrubbing, is a crucial step in the data analysis process that involves detecting and correcting errors, inconsistencies, and anomalies in the dataset. It is like preparing a canvas before a painter begins to create their masterpiece - essential groundwork that sets the stage for accurate and reliable analysis.
Data cleaning plays a pivotal role in ensuring the quality and integrity of the dataset, as it directly impacts the accuracy of any insights or models derived from the data. By eliminating or rectifying errors such as missing values and outliers, data cleaning enhances the robustness of the analysis, enabling researchers to draw meaningful conclusions and make informed decisions based on reliable data. Without proper data cleaning, the integrity of analytical results can be compromised, leading to flawed insights and inaccurate interpretations.
One of the key elements of data cleaning is the identification and handling of missing values. Missing values, denoted as Na N (Not a Number) or NULL, can significantly impact the analysis by skewing statistical measures and distorting patterns within the dataset. Effective handling of missing values involves strategies such as imputation (replacing missing values with estimated or calculated values), deletion (removing records or variables with missing values), or categorization (treating missing values as a separate category). Each approach has its own implications and considerations, depending on the nature of the data and the research objectives.
Another critical aspect of data cleaning is the implementation of outlier detection techniques. Outliers are data points that deviate significantly from the rest of the dataset, potentially indicating errors, anomalies, or rare events. Detecting and dealing with outliers is essential for maintaining the integrity and reliability of the analysis, as these anomalous data points can skew results and mislead interpretations. Outlier detection techniques range from simple methods like z-score analysis and box plots to advanced algorithms such as Isolation Forest and Local Outlier Factor, each offering unique advantages and limitations in identifying and handling outliers within the dataset.
Exploratory Data Analysis (EDA)
Exploratory Data Analysis (EDA) plays a crucial role in shaping the narrative of this article, focusing on uncovering hidden patterns, trends, and relationships within the dataset. By delving deep into the dataset through EDA, we can extract valuable insights that form the foundation for further analysis and decision-making processes. EDA involves a systematic approach to examining the dataset, identifying outliers, understanding data distributions, and exploring correlations between variables, paving the way for more advanced modeling techniques.
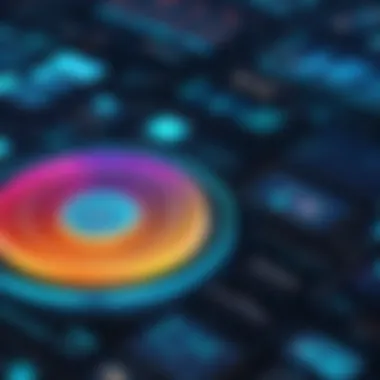
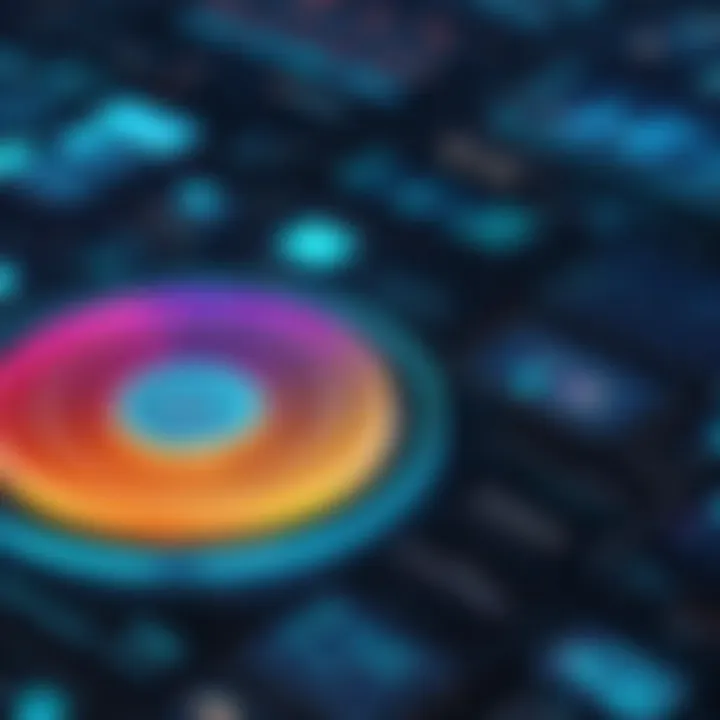
Visualizing the Dataset
Distribution Plots
Distribution Plots offer a visual representation of the distribution of data points within a dataset, enabling us to grasp the central tendency, spread, and skewness of variables. The key characteristic of Distribution Plots lies in their ability to showcase the frequency of different values, shedding light on the data's distribution pattern. In this article, Distribution Plots serve as a fundamental tool for understanding the data distribution, outlier detection, and identifying potential data transformations. Despite their simplicity, Distribution Plots are highly beneficial for gaining initial insights into the data's shape and structure, setting the stage for more in-depth analysis.
Correlation Heatmaps
Correlation Heatmaps provide a clear visualization of the relationships between variables by displaying correlation coefficients in a color-coded matrix format. The essential feature of Correlation Heatmaps is their capacity to highlight strong positive or negative correlations, offering insights into how variables interact with each other. In the context of this article, Correlation Heatmaps serve as a powerful tool for feature selection, identifying multicollinearity, and understanding the dependencies between variables. While Correlation Heatmaps are valuable for detecting patterns and relationships, they may oversimplify complex interactions and mask nonlinear dependencies, necessitating a nuanced interpretation in the analysis process.
Feature Engineering
Transforming Raw Data
Creating New Features
Creating new features involves deriving valuable insights from existing data elements. In the realm of feature engineering, creating new features can unlock hidden patterns or correlations within the dataset. This process adds depth and complexity to the analysis, enabling the extraction of more meaningful information. The key characteristic of creating new features lies in its ability to provide a new perspective on the data, facilitating the discovery of novel relationships. In this article, creating new features is emphasized as a strategic approach to improving the quality and relevance of the dataset for subsequent analytical tasks.
Encoding Categorical Variables
Encoding categorical variables is another essential aspect of feature engineering. In feature engineering, encoding categorical variables involves converting qualitative data into a numerical format that can be easily processed by machine learning algorithms. The key advantage of encoding categorical variables is its ability to incorporate categorical data into the analysis effectively. By transforming qualitative variables into a quantitative form, this process enables the algorithm to interpret and utilize the information more efficiently. However, one must be cautious of potential drawbacks such as the introduction of bias or loss of information during the encoding process. In this article, encoding categorical variables is highlighted as a critical step in preparing the dataset for robust analysis.
Model Building
In the realm of data analysis, perhaps one of the most critical stages is Model Building. This phase involves the selection, training, and evaluation of machine learning models on the dataset at hand. The importance of Model Building cannot be overstated in this article about exploring a random dataset. It serves as the crux where raw data transforms into actionable insights. By delving into Model Building, we navigate through the intricacies of algorithms, fine-tuning parameters, and iteratively enhancing predictive capabilities. Through this meticulous process, data scientists can unearth patterns, trends, and relationships that drive informed decision-making.
Selecting the Right Algorithm

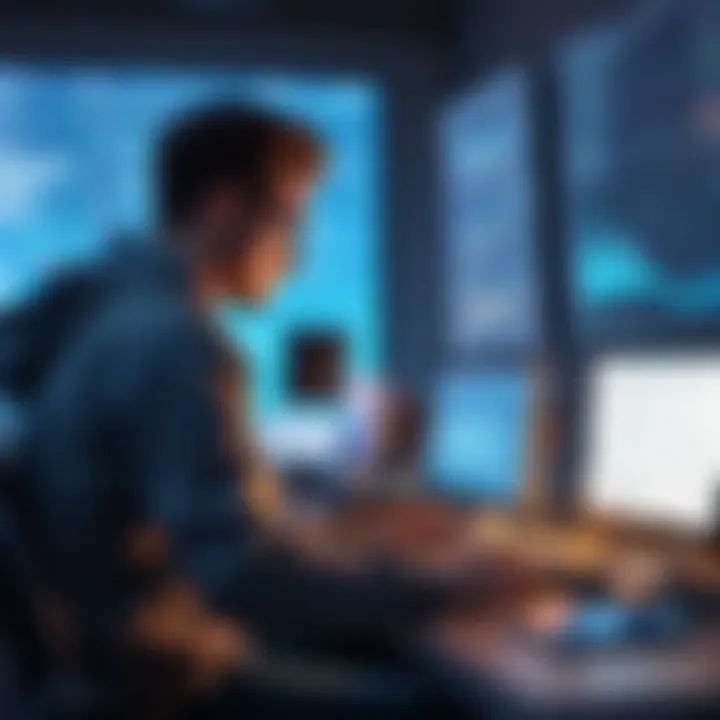
Supervised Learning Models
When we zoom in on Supervised Learning Models within the context of this article, we uncover a fundamental aspect of machine learning. Supervised learning involves training models on labeled data with defined input-output pairs. The key characteristic of Supervised Learning Models lies in their ability to learn from historical data to make predictions or classifications on new, unseen data. This characteristic makes Supervised Learning Models a popular choice in this article as it empowers stakeholders to forecast outcomes based on past observations. Despite their efficacy, one must acknowledge the limitation of Supervised Learning Models - they heavily rely on the quality and representativeness of training data, which can introduce bias or inaccuracies.
Unsupervised Learning Approaches
Switching gears to Unsupervised Learning Approaches, we shift towards a different paradigm of model development. Unsupervised learning tasks involve exploring data for hidden patterns or structures without explicit guidance from labeled examples. The primary feature of Unsupervised Learning Approaches that shines in this article is their capacity to discover intrinsic relationships within the dataset, leading to insights that may elude human perception. By embracing Unsupervised Learning Approaches, analysts can uncover clusters, anomalies, or associations that lay dormant within the data, offering a fresh perspective and potential avenues for further investigation. However, it is vital to note that the interpretability of results and the need for human validation are notable challenges when deploying Unsupervised Learning Approaches in real-world scenarios.
Model Evaluation
Model evaluation holds a pivotal role in the realm of data analysis and machine learning, as it determines the effectiveness and performance of the model in handling the dataset under scrutiny. In the context of this article, model evaluation serves as the critical phase where the success of the entire analytical process is gauged. Through various metrics and techniques, analysts can ascertain the accuracy and reliability of their models in making predictions and uncovering patterns within the dataset. By delving deep into model evaluation, this section aims to provide insights into how different algorithms behave and perform under varying circumstances, shedding light on their strengths and limitations.
Assessing Model Performance
Accuracy Metrics
Accuracy metrics stand as one of the foundational pillars of assessing a model's performance, offering a quantifiable measure of how well the model predicts outcomes compared to the actual data. In the landscape of this article, accuracy metrics play a crucial role in quantifying the predictive accuracy of the models developed through the data analysis process. The key characteristic of accuracy metrics lies in their ability to provide a clear and straightforward measurement of the model's correctness, making them a popular choice for evaluating model performance in this context. However, it is essential to note that accuracy metrics may exhibit certain limitations, especially when dealing with imbalanced datasets or situations where false positivesnegatives carry significant consequences. Despite this, accuracy metrics remain a valuable tool for assessing model performance and guiding decision-making processes within the data analysis journey.
Cross-Validation Techniques
Cross-validation techniques offer a robust method for validating the performance and generalizability of machine learning models. In the scope of this article, cross-validation techniques play a vital role in refining and optimizing the models constructed during the data analysis phase. The key characteristic of cross-validation lies in its ability to assess how well a model will generalize to an independent dataset, ensuring that the predictive power observed during model training holds true in real-world scenarios. By leveraging cross-validation techniques, analysts can mitigate the risk of overfitting and assess the model's performance across different subsets of the dataset, enhancing its reliability and applicability. However, it is essential to acknowledge that cross-validation techniques may introduce computational overhead, especially with large datasets, requiring careful consideration and resource management to ensure effective model evaluation and performance assessment.
Conclusion
In this article, the Conclusion section serves as the culmination of the intricate journey through a random dataset. Understanding the significance of wrapping up the analysis process is essential for tech enthusiasts and industry professionals alike. Summarizing key findings and future implications is crucial to distill the complex information uncovered during exploration. By presenting a clear and concise wrap-up, readers can grasp the overarching insights obtained from the dataset.
Unveiling Insights
Key Findings
Delving into the Key Findings subtopic sheds light on specific discoveries made during the analysis of the random dataset. These findings play a pivotal role in shaping the overall narrative of the exploration, offering crucial insights into the dataset's characteristics and patterns. Understanding the essence of these key findings is paramount, as they provide valuable information that contributes to the broader understanding of the dataset. One key characteristic of these findings is their ability to unveil hidden trends and relationships within the data, offering a deeper understanding of its underlying structure. The unique feature of key findings lies in their capacity to guide decision-making processes and strategic outcomes based on data-driven insights.
Future Implications
Exploring the Future Implications section dives into the long-term consequences and applications of the insights garnered from the random dataset analysis. This aspect adds a forward-thinking dimension to the exploration, highlighting potential opportunities and challenges that may arise based on the current findings. The key characteristic of future implications is their ability to forecast trends and inform future decision-making processes in a proactive manner. By contemplating the future implications of the dataset analysis, stakeholders can anticipate changes, strategize effectively, and stay ahead of the curve. One notable feature of future implications is their role in guiding innovation and progress, leveraging data insights to drive meaningful transformation within the industry.