Unraveling the Depths of AI, Machine Learning, and Deep Learning
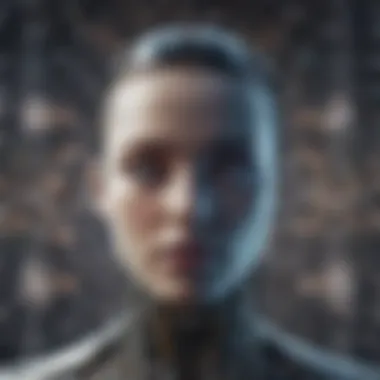
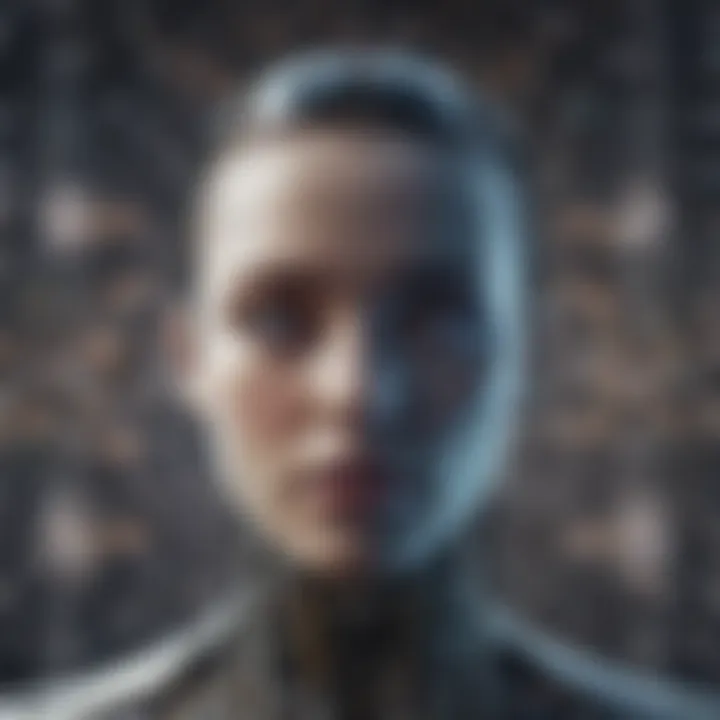
Tech Trend Analysis
In contemporary times, the technological landscape is witnessing a significant shift towards the integration of artificial intelligence (AI), machine learning, and deep learning. The current trend reflects a heightened focus on leveraging these advanced technologies to enhance efficiency and drive innovation across various sectors. For consumers, this paradigm shift translates into personalized experiences, intelligent automation, and the democratization of complex processes. Looking ahead, the future holds promising possibilities, such as seamless human-machine collaboration, enhanced predictive analytics, and deeper insights into consumer behavior and preferences.
Product Reviews
Moving onwards to product reviews, it is crucial to understand the nuances of AI, machine learning, and deep learning applications within different systems. These technologies encompass a wide array of features and specifications that cater to diverse industry needs. From the analysis of performance metrics to evaluating the pros and cons of implementation, each aspect contributes to the overall recommendation for integrating these solutions. By diving deep into product functionalities, users can glean insights into maximizing operational efficiency, optimizing decision-making processes, and unlocking transformative capabilities for their businesses.
How-To Guides
When delving into the realm of AI, machine learning, and deep learning, a comprehensive how-to guide is indispensable for enthusiasts and professionals alike. This introductory section lays the groundwork for understanding the basics of these technologies, elucidating complex concepts in a simplified manner. Through step-by-step instructions, users can navigate the intricacies of implementing AI algorithms, deploying machine learning models, and harnessing the power of deep neural networks effectively. Additionally, tips and tricks offer valuable insights for optimizing performance, refining methodologies, and troubleshooting common challenges that may arise during implementation.
Industry Updates
Amidst the rapidly evolving tech landscape, staying abreast of industry updates is imperative for informed decision-making and strategic planning. Recent developments in AI, machine learning, and deep learning epitomize the ongoing revolution in digital transformation and data-driven decision-making. By analyzing market trends, businesses can gain a competitive edge, adapt to changing consumer preferences, and tailor their offerings to meet evolving demands. The impact of these technological advancements reverberates across industries, influencing business operations, consumer behavior, and the overall technological ecosystem.
Introduction
In this pivotal section, we lay the groundwork for exploring the intricate world of artificial intelligence, machine learning, and deep learning. With technology advancing at an unprecedented pace, understanding these concepts is crucial for individuals and industries looking to stay at the forefront of innovation. By delving into the definitions, differences, and applications of AI, ML, and DL, we set the stage for a comprehensive deep dive. This section serves as a gateway to the fascinating realm where human intelligence converges with computational power, shaping the future of technology-driven possibilities.
Defining Artificial Intelligence
AI Overview
Embarking on the AI Overview journey, we uncover the essence of artificial intelligence - the simulated intelligence displayed by machines. This segment outlines how AI mimics human cognitive functions like learning and problem-solving to drive automation and efficiency in various domains. The allure of AI Overview lies in its ability to enhance decision-making processes, optimize tasks, and revolutionize industries through data-driven insights. Despite concerns about AI potentially replacing human roles, its capacity to augment human capabilities remains a predominant theme.
AI History
Traversing through the corridors of AI History unveils the evolution and milestones that have shaped the contemporary AI landscape. From its conceptualization to practical applications, AI History encapsulates the growth trajectory of artificial intelligence, underlining its transformative impact on society. By examining past challenges and breakthroughs, we gain a profound understanding of the intricate nuances that have propelled AI to its current zenith.
AI Capabilities
Unveiling the multifaceted AI Capabilities illuminates the diverse facets of artificial intelligence that empower varied industries. From data analytics to predictive modeling, AI Capabilities showcase the breadth of functionalities that redefine conventional processes and open avenues for innovation. Highlighting the adaptability and scalability of AI applications, this segment underscores the boundless potential for integration across sectors, heralding a new era of intelligent technological advancements.
Evolution of Machine Learning
Machine Learning Basics
Embarking on the Machine Learning Basics expedition unravels the fundamental principles that underpin this transformative subset of AI. Grasping the essence of algorithms and statistical models, Machine Learning Basics equips individuals with the tools to extract meaningful insights from datasets. As a cornerstone of contemporary artificial intelligence, the significance of Machine Learning Basics permeates diverse sectors, fueling advancements in predictive analytics and optimization strategies.
Supervised Learning
Navigating the realm of Supervised Learning delves into the realm of labeled data and pattern recognition to drive predictive modeling and decision-making processes. Through training algorithms on historical input-output pairs, Supervised Learning enables machines to extrapolate trends and correlations, facilitating informed predictions. The efficacy and interpretability of Supervised Learning methodologies position it as a quintessential tool in data-driven enterprises, offering a reliable framework for deriving actionable insights.
Unsupervised Learning
Venturing into Unsupervised Learning territory unveils the realm of unlabeled data exploration and pattern discovery without predefined outcomes. By uncovering hidden structures and relationships within datasets, Unsupervised Learning empowers enterprises to unveil latent insights and streamline processes through clustering and dimensionality reduction techniques. The autonomy and versatility of Unsupervised Learning methodologies accentuate its significance in anomaly detection, segmentation, and optimization tasks.
Reinforcement Learning
Embarking on the Reinforcement Learning odyssey introduces a dynamic paradigm where machines learn through rewards and reinforcements, mimicking human decision-making processes. By navigating complex environments and optimizing actions based on outcomes, Reinforcement Learning paves the way for autonomous systems and adaptive agents. The resilience and adaptability of Reinforcement Learning models make them instrumental in optimizing strategies, real-time decision-making, and autonomous operations in evolving ecosystems.
Deep Dive into Deep Learning
Neural Networks
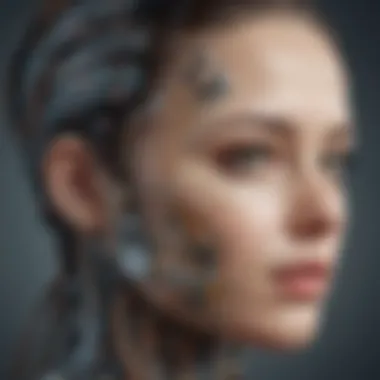
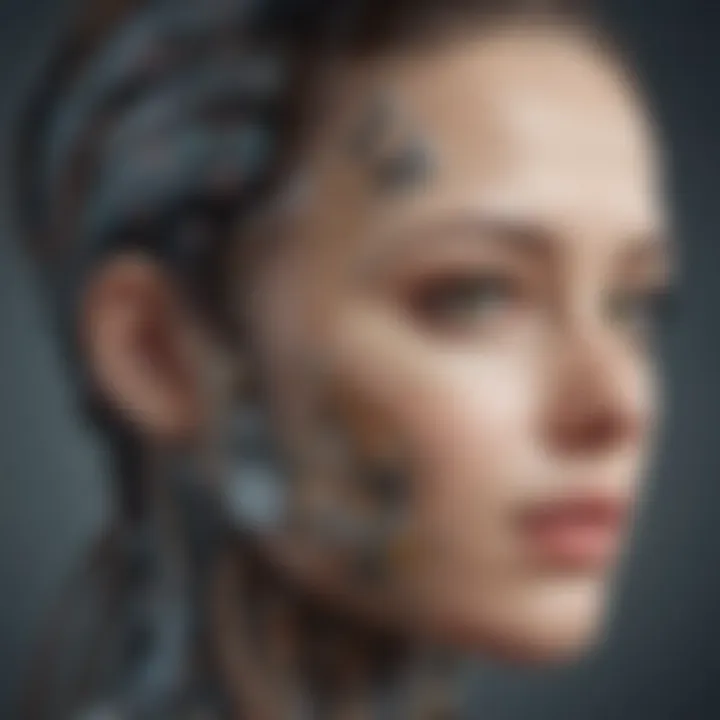
Exploring the intricate realm of Neural Networks unravels a network of interconnected nodes that emulate the human brain's neural architecture. From Convolutional Neural Networks (CNNs) to Recurrent Neural Networks (RNNs), the diverse array of neural network architectures showcases the versatility and capabilities of deep learning in image recognition, language processing, and predictive modeling. The prowess of Neural Networks lies in their ability to comprehend complex patterns, adapt to varying inputs, and drive innovation across industries.
Deep Learning Applications
Delving into Deep Learning Applications sheds light on the transformative impact of deep learning algorithms in revolutionizing industries. From image recognition and natural language processing to predictive analytics, Deep Learning Applications exemplify the versatility and scalability of deep learning in addressing diverse challenges. By harnessing the power of artificial neural networks, deep learning applications redefine conventional processes, optimize outcomes, and unlock new possibilities in the realms of healthcare, finance, and beyond.
Deep Learning Algorithms
Navigating through Deep Learning Algorithms reveals a spectrum of sophisticated mathematical models and optimization techniques that underpin deep learning methodologies. From backpropagation to stochastic gradient descent, Deep Learning Algorithms showcase the robust frameworks that drive neural network training and refinement. The precision and efficiency of deep learning algorithms position them as cornerstones of modern AI research, facilitating breakthroughs in pattern recognition, anomaly detection, and decision support systems.
AI vs. Machine Learning
In exploring the intricate world of artificial intelligence (AI) and machine learning (ML), it is imperative to distinguish between the two to grasp their distinct functionalities. AI represents the broader domain mimicking human intelligence, while ML is a subset focusing on self-learning algorithms. The crux lies in AI's ability to imitate human cognition, reasoning, and decision-making. ML, conversely, delves into pattern recognition and iterative learning from data without explicit programming instructions. Understanding this dissimilarity is crucial to harnessing their potential effectively.
Distinguishing AI and
Core Differences
Core differences transcend the surface disparities between AI and ML, delineating their fundamental essence. While AI embodies the overarching concept of intelligence simulation, ML emphasizes the optimization of algorithm performance through experience. The core distinction lies in AI's all-encompassing nature, aiming to replicate human intellectual capabilities, whereas ML's prime focus is on enhancing decision-making based on data patterns. This divergence forms the bedrock of technological advancements across industries.
Common Misconceptions
The labyrinth of common misconceptions surrounding AI and ML often clouds the understanding of these technologies. One prevalent fallacy is viewing AI and ML as interchangeable terms, overlooking the nuanced disparity in their functionalities. Dispelling these myths is pivotal to navigating the realm of AI and ML, unraveling their true potential in revolutionizing varied sectors.
Real-world Examples
Real-world applications elucidate the practical relevance of AI and ML, fortifying their significance in modern contexts. From self-driving cars leveraging AI for autonomous navigation to personalized recommendation systems powered by ML algorithms, real-world instances underscore the transformative impact of these technologies. Unveiling these examples not only bolsters understanding but also inspires innovative implementations in diverse fields.
Applications of AI and
Business Intelligence
Business intelligence harnesses the capabilities of AI and ML to extract insights from complex datasets, enabling data-driven decision-making. AI's cognitive computing aids in predictive analytics, while ML's predictive modeling optimizes operational efficiencies. The fusion of AI and ML in business intelligence empowers organizations to propel strategic growth and gain a competitive edge in dynamic markets.
Healthcare
The healthcare sector stands to benefit immensely from AI and ML integration, fostering advancements in diagnostics, personalized treatment plans, and drug discovery. AI's diagnostic accuracy and ML's predictive analytics enhance patient care outcomes, revolutionizing healthcare delivery. Embracing these technologies heralds a new era of precision medicine and improved operational efficiencies in healthcare systems.
Autonomous Vehicles
Autonomous vehicles epitomize the epitome of AI and ML applications, revolutionizing the transportation landscape. AI algorithms empower vehicles to perceive their environment and make real-time decisions, ensuring safer and more efficient travel experiences. ML-based predictive modeling enhances route optimization, traffic prediction, and driver assistance systems, paving the way for a paradigm shift in urban mobility.
Future Trends
AI Ethics
As AI technologies proliferate, ethical considerations gain prominence in ensuring responsible AI development and deployment. Ethical frameworks guide the ethical implications of AI algorithms, addressing concerns such as bias mitigation and data privacy. Upholding AI ethics is paramount to fostering trust in AI systems and safeguarding against potential societal repercussions, underscoring the imperative of ethical AI governance.
Advancements
Advancements in ML algorithms continuously reshape the technological landscape, imbuing machine learning with enhanced predictive capabilities and efficiency. From neural networks to deep learning architectures, ML advancements push the boundaries of data analytics and pattern recognition, unlocking new possibilities for predictive modeling and decision support systems. Keeping abreast of ML advancements is essential to harnessing the full potential of data-driven insights and innovation.
Industry Impacts
AI and ML's impact on diverse industries transcends incremental changes, catalyzing paradigm shifts in operations and customer experiences. Industries such as finance, healthcare, and manufacturing witness substantial transformations through AI-driven automation, data analytics, and personalized services. Understanding these industry impacts elucidates the strategic imperatives for organizations to adapt to evolving market dynamics and technological disruptions, shaping the future of work and consumer experiences.
Deep Learning Demystified
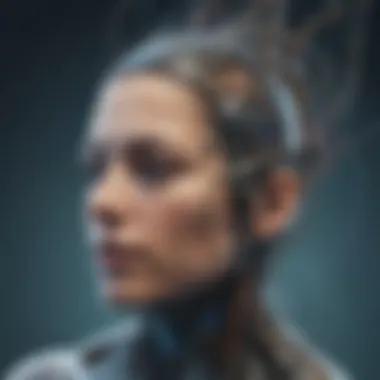
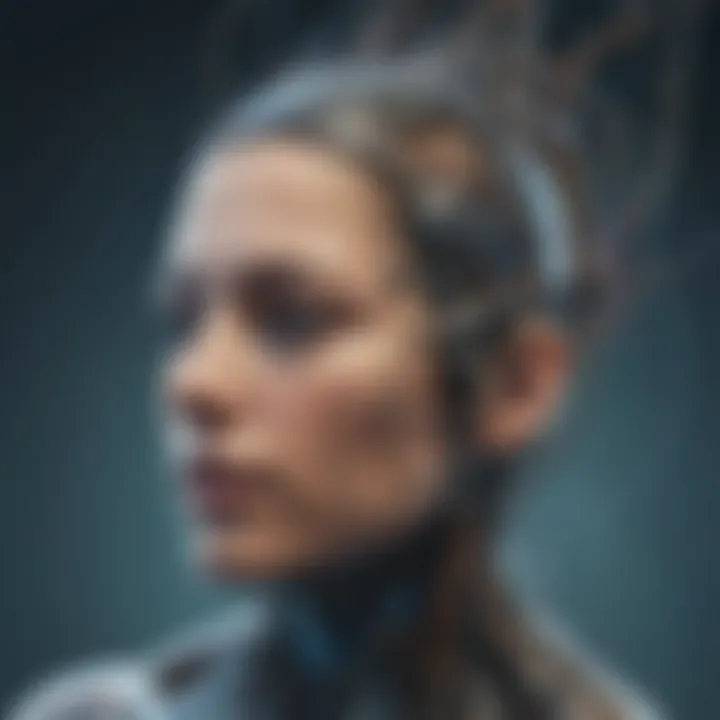
In this section, we will delve into the complexities of Deep Learning to demystify its inner workings and significance. Deep Learning holds a pivotal role in this article as it uncovers the intricate layers of neural networks, leading to a profound understanding of AI applications. By dissecting the core concepts of Deep Learning, we aim to provide readers with a comprehensive overview of this advanced technology. Exploring the specifics, benefits, and considerations of Deep Learning Demystified elucidates its critical role in revolutionizing industries and shaping the future landscape of artificial intelligence.
Understanding Neural Networks
CNNs
Convolutional Neural Networks (CNNs) are a fundamental element of Deep Learning architecture that plays a crucial role in image recognition and spatial data analysis. Their ability to detect patterns and extract features from vast amounts of visual data makes them indispensable in various AI applications. CNNs' key characteristic lies in their hierarchical structure, enabling them to learn complex representations hierarchically, contributing to enhanced feature extraction. Moreover, the unique feature of shared weights in CNNs optimizes model parameters, significantly reducing computational expenses while maintaining high performance. Despite their advantages in image-related tasks, CNNs may face limitations in handling sequential data efficiently.
RNNs
Recurrent Neural Networks (RNNs) are designed to process sequential data by retaining memory of previous inputs, making them proficient in tasks requiring contextual understanding such as natural language processing and speech recognition. The key characteristic of RNNs lies in their recurrent connections, which allow information to persist throughout the network, fostering temporal dependencies in data analysis. One of the distinguishing features of RNNs is their flexibility in handling input of varying lengths, a critical advantage in scenarios with dynamic data sequences. However, RNNs may encounter challenges in capturing long-term dependencies due to vanishing or exploding gradient issues, impacting their performance in tasks requiring extended memory.
Autoencoders
Autoencoders serve as unsupervised learning tools that aim to reconstruct the input data, focusing on capturing essential features and reducing dimensionality through an encoder-decoder architecture. The key characteristic of Autoencoders lies in their ability to learn compact representations of data with minimal loss, making them efficient in anomaly detection and data denoising tasks. The unique feature of Autoencoders lies in their capacity to learn latent representations, enabling them to uncover underlying patterns in complex data sets. While Autoencoders excel in feature learning and generation tasks, they may struggle with capturing intricate features in high-dimensional data effectively.
Applications of Deep Learning
Image Recognition
Image Recognition stands at the forefront of Deep Learning applications, revolutionizing fields such as autonomous driving, medical imaging, and security systems. Its key characteristic lies in its ability to process visual data, enabling machines to identify objects, scenes, or patterns with remarkable accuracy. The unique feature of transfer learning in Image Recognition allows models to leverage pre-trained knowledge for new tasks, enhancing efficiency and scalability. However, deploying Image Recognition systems may pose challenges in data diversity and model adaptability across varying environments.
NLP
Natural Language Processing (NLP) constitutes a vital domain of Deep Learning applications, empowering machines to understand, interpret, and generate human language. The key characteristic of NLP resides in its capacity to process textual data, facilitating tasks such as sentiment analysis, machine translation, and content generation. The unique feature of attention mechanisms in NLP enhances model performance by focusing on relevant parts of input texts, improving contextual understanding. Nevertheless, NLP applications may confront complexities in semantic ambiguity and linguistic variations, impacting the accuracy and robustness of language models.
Predictive Analytics
Predictive Analytics harnesses the power of Deep Learning to forecast future trends, behaviors, and outcomes based on historical data patterns. Its key characteristic lies in predictive modeling techniques, enabling organizations to make data-driven decisions and optimize resource allocation. The unique feature of ensemble learning in Predictive Analytics combines multiple models to enhance predictive accuracy and mitigate overfitting issues. However, challenges may arise in data quality, model interpretability, and the need for continuous model validation to ensure forecasting precision.
Challenges and Future Prospects
Data Privacy
Data Privacy emerges as a critical concern in Deep Learning applications, emphasizing the ethical and regulatory aspects of handling sensitive information. Its key characteristic lies in ensuring secure data processing, storage, and transmission to safeguard user privacy and prevent unauthorized access. The unique feature of differential privacy in Data Privacy mechanisms enables organizations to analyze data while preserving individuals' confidentiality through noise addition techniques. Despite its advantages in data protection, Data Privacy may face challenges in balancing privacy preservation with data utility and model accuracy.
Scalability Issues
Scalability Issues pose significant hurdles in the deployment of Deep Learning models at scale, impacting efficiency and performance across diverse computing environments. The key characteristic of scalability challenges lies in optimizing model training and inference processes to handle increasing data volumes and computational resources. The unique feature of distributed computing solutions in addressing Scalability Issues enhances model parallelism and resource utilization, accelerating model training and deployment. Nevertheless, managing scalability complexities demands robust infrastructure, efficient data pipelines, and comprehensive monitoring systems to ensure seamless and reliable Deep Learning operations.
Advancements in
Advancements in Deep Learning drive continuous innovation and technological growth, offering new possibilities and capabilities in AI research and applications. The key characteristic of DL advancements focuses on enhancing model architectures, optimizing algorithms, and improving model interpretability to address emerging challenges and industry demands. The unique feature of self-supervised learning techniques in DL advancements facilitates autonomous model training without extensive labeled data, promoting efficient learning and adaptation to diverse tasks. Despite their benefits, DL advancements may raise concerns regarding model robustness, interpretability, and ethical implications, necessitating ongoing research and collaboration to navigate future developments effectively.
Innovations and Impact
Artificial intelligence has sparked a wave of innovations with profound impacts across various sectors, redefining traditional paradigms and shaping future trajectories. In this piece, we unravel the significance of innovations and their far-reaching impact on our rapidly evolving landscape. By dissecting recent developments in AI, machine learning, and deep learning, we illuminate the pivotal role these technologies play in revolutionizing industries and enhancing operational efficiencies.
Recent Developments
AI in Robotics
The integration of artificial intelligence in robotics is a groundbreaking advancement that has revolutionized automation processes and enriched industrial operations. Characterized by its adaptability and precision, AI-enabled robots are adept at performing intricate tasks with unparalleled accuracy. This fusion of AI and robotics not only streamlines production processes but also paves the way for enhanced efficiency and productivity. Despite its undeniable advantages, challenges such as the complexity of human-robot collaboration and ethical considerations regarding autonomous decision-making loom large in the realm of AI-powered robotics.
in Finance
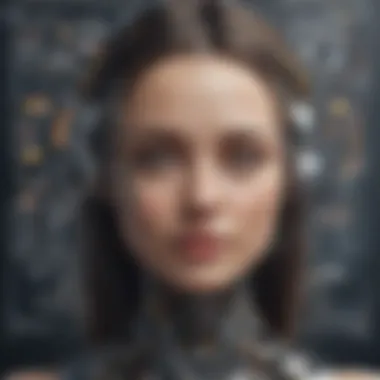
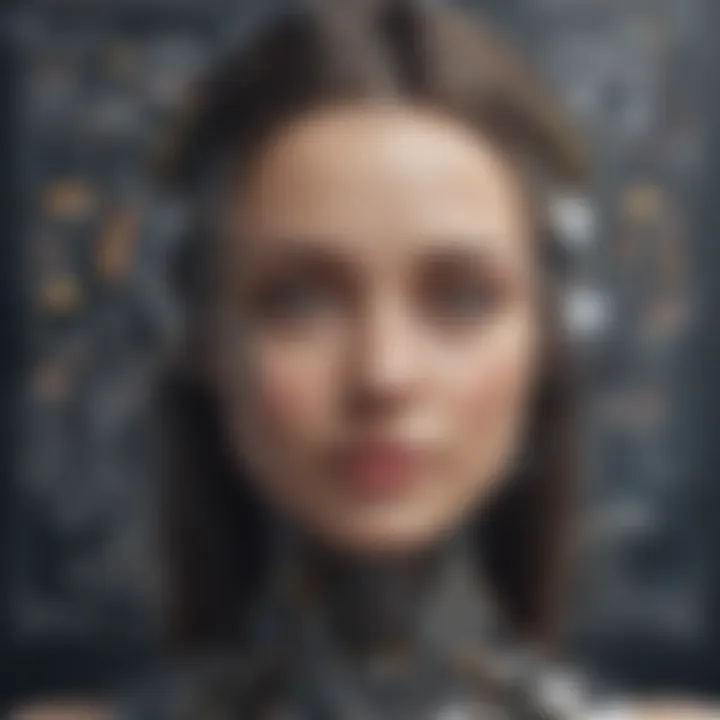
Machine learning applications in the realm of finance have redefined how organizations analyze data, manage risks, and make strategic decisions. With its ability to detect patterns, trends, and anomalies in financial datasets, ML drives accurate predictions and informed financial strategies. The key characteristic of machine learning in finance lies in its predictive analytics capabilities, empowering institutions to optimize operations and enhance investment outcomes. However, concerns around data privacy, algorithm bias, and regulatory compliance pose significant challenges to the widespread adoption of machine learning in the financial sector.
in Healthcare
Deep learning applications in healthcare have redefined medical diagnostics, treatment strategies, and patient care, ushering in a new era of precision medicine. Leveraging neural networks and convolutional architectures, deep learning algorithms analyze complex medical imaging data with unprecedented accuracy, facilitating early disease detection and personalized treatment plans. The distinctive feature of deep learning in healthcare lies in its ability to extract insightful patterns from voluminous medical data, enabling healthcare providers to deliver targeted interventions and improve patient outcomes. Yet, concerns over data security, algorithm interpretability, and ethical use of patient information remain critical considerations in deploying deep learning solutions within healthcare ecosystems.
Societal Implications
Job Displacement
The automation and digital transformation fueled by AI and machine learning technologies have raised concerns regarding job displacement and the future of work. While technological advancements enhance operational efficiencies and drive economic growth, the displacement of traditional roles and the emergence of new skill demands pose challenges to workforce adaptation and socioeconomic sustainability. Job displacement represents a fundamental shift in labor dynamics, necessitating comprehensive reskilling initiatives and robust workforce policies to mitigate the impact of automation on employment opportunities.
Ethical Concerns
The ethical considerations surrounding AI, machine learning, and deep learning technologies underscore the need for responsible development and deployment practices. Ethical concerns encompass a wide spectrum of issues, including algorithmic bias, data privacy infringements, autonomous decision-making dilemmas, and societal repercussions of AI-driven systems. Addressing these ethical dilemmas requires multidisciplinary collaboration, regulatory frameworks, and ethical guidelines to ensure that technological advancements align with ethical standards and societal values.
Policy Recommendations
In response to the ethical and societal challenges posed by AI innovations, policymakers and industry stakeholders are advocating for robust policy recommendations to govern the ethical use of AI technologies. Policy recommendations encompass a spectrum of initiatives, including data protection regulations, algorithm transparency requirements, bias mitigation strategies, and accountability frameworks for AI-driven systems. The pivotal role of policy recommendations lies in fostering a regulatory environment that balances innovation with ethical considerations, safeguarding societal well-being and promoting responsible AI development.
The Path Ahead
Technological Advancements
The trajectory of AI, machine learning, and deep learning hinges on continuous technological advancements that push the boundaries of innovation and exploration. Technological advancements encompass a spectrum of developments, from algorithmic enhancements and hardware optimizations to the integration of AI with emerging technologies such as quantum computing and edge computing. The key characteristic of technological advancements lies in their ability to unlock new possibilities, catalyze breakthrough innovations, and reshape the technological landscape. However, navigating the ethical, legal, and societal implications of rapid technological advancements remains a critical endeavor for industry leaders, policymakers, and researchers.
Regulatory Frameworks
The establishment of robust regulatory frameworks is integral to guiding the responsible development and deployment of AI technologies within diverse sectors. Regulatory frameworks set standards for data privacy, algorithm transparency, cybersecurity protocols, and ethical use of AI applications to safeguard user rights and public interests. The importance of regulatory frameworks lies in their capacity to instill trust in AI systems, ensure compliance with legal standards, and uphold ethical principles in technological innovation. Despite the complexities inherent in crafting effective regulatory frameworks, collaboration between policymakers, industry experts, and ethicists is essential to navigate the evolving AI regulatory landscape.
AI Governance
AI governance frameworks are critical mechanisms for overseeing the ethical, legal, and operational dimensions of AI technologies within organizational contexts. The key characteristic of AI governance lies in its capacity to define accountability structures, risk management protocols, and ethical guidelines for AI strategy implementation. By establishing clear governance frameworks, organizations can effectively manage the risks associated with AI technologies, uphold ethical standards, and align AI initiatives with corporate values and societal expectations. The complexity of AI governance warrants proactive measures to promote transparency, accountability, and responsible AI practices across diverse industries and organizational settings.
Conclusion
Key Takeaways
AI Empowering Innovations
AI Empowering Innovations play a pivotal role in driving advancements across industries. The unique characteristic of AI lies in its ability to enhance efficiency and productivity through automation and smart decision-making processes. This innovation is a popular choice in industries seeking to streamline operations and optimize workflows. However, challenges such as data security and ethical concerns may arise with the adoption of AI Empowering Innovations.
Driving Efficiency
ML Driving Efficiency focuses on leveraging data-driven insights to improve processes and decision-making. The key characteristic of machine learning lies in its adaptive nature, allowing systems to learn from data and optimize performance autonomously. This aspect makes ML an invaluable tool for optimizing various functions within businesses. Yet, ensuring data quality and addressing biases are essential considerations in implementing ML for driving efficiency.
Shaping the Future
DL Shaping the Future encompasses the potential of deep learning algorithms to revolutionize how we interact with technology. The key characteristic of deep learning is its ability to process vast amounts of complex data to extract meaningful patterns and insights. This makes DL instrumental in shaping innovations across sectors such as healthcare, finance, and more. Despite its transformative potential, scalability challenges and data privacy issues need to be addressed to fully harness the benefits of DL.
Final Thoughts
Embracing Technological Evolution
Embracing Technological Evolution is imperative in staying competitive and relevant in today's fast-paced digital landscape. The key characteristic of technological evolution is its dynamic nature, constantly pushing boundaries and driving progress. This proactive approach to embracing technological advancements is beneficial for organizations looking to future-proof their operations. However, ensuring seamless transitions and upskilling workforce are critical aspects to consider.
Continuous Learning Imperative
Continuous Learning Imperative underscores the necessity of ongoing education and skill development in the face of technological disruptions. The key characteristic of continuous learning is its role in fostering adaptability and innovation. By prioritizing continuous learning, individuals and businesses can stay agile and responsive to evolving industry trends. While the benefits of continuous learning are immense, balancing work commitments with ongoing education can pose challenges.
Impacts on Society
Impacts on Society reflect the wide-ranging consequences of technological advancements on individuals and communities. The key characteristic of societal impacts is the ripple effect of technology on daily lives, economies, and social structures. Understanding and mitigating these impacts are crucial for creating sustainable solutions and inclusive progress. However, navigating ethical dilemmas and ensuring equitable access to technology remain critical considerations in managing society's technological evolution.