Security Challenges in Big Data: An In-Depth Analysis

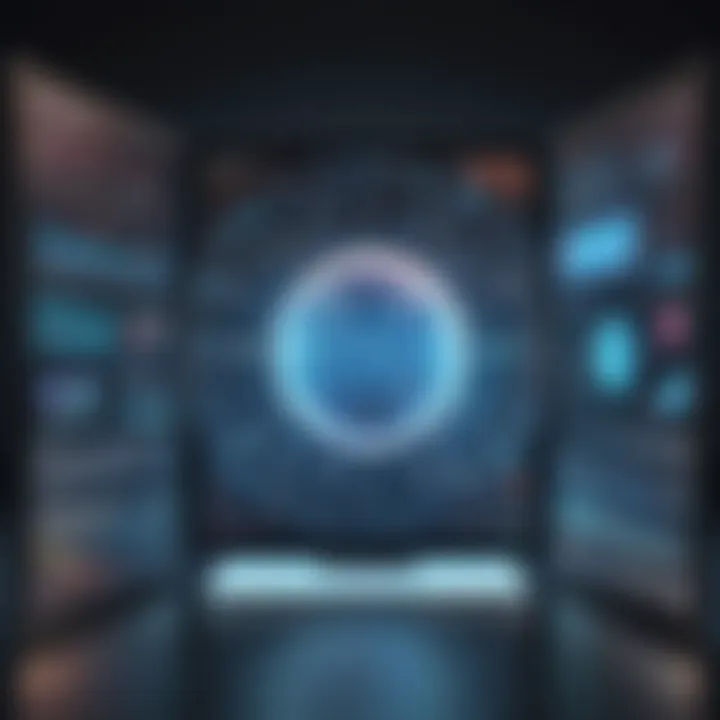
Intro
As organizations increasingly rely on big data to steer their strategic decisions, the security landscape around this data becomes crucial. Security challenges emerge from vast datasets that contain sensitive information, necessitating a closer examination of vulnerabilities that can compromise data integrity, confidentiality, and availability. This exploration serves to emphasize the importance of addressing potential risks that come with employing big data technologies.
This article will dissect the key threats to security in big data environments, while also presenting proactive measures and technologies that can help mitigate these risks. By navigating this complex landscape, readers can better safeguard their enterprises' information and maintain compliance with relevant regulations.
Prelude to Big Data Security Issues
In the current digital landscape, big data serves as a cornerstone for driving innovations across industries. However, these advancements come with notable security challenges. Understanding these concerns is vital for organizations that rely on extensive datasets. This section outlines the nuances of big data security issues, presenting a foundation upon which later discussions regarding threats and protective measures will build.
Defining Big Data
Big data refers to the massive volumes of structured and unstructured data that inundate a business daily. These datasets present both opportunities and challenges. They can originate from diverse sources such as social media interactions, transaction records, IoT devices, and more. The characteristics of big data encompass volume, velocity, and variety. Volume signifies the sheer amount of data, velocity pertains to the speed at which data is collected, and variety refers to the different types of data formats.
Organizations often leverage big data to uncover insights, improve decision-making, and enhance customer experiences. However, the store of information introduces complexities, particularly around security. These complexities range from data storage methods to the handling of real-time analytics increases. As the quantity of data grows, so do the orchestrated threats aiming to compromise it.
Importance of Data Security
The significance of data security in the realm of big data cannot be overstated. Protecting information remains imperative for several reasons:
- Confidentiality: Organizations must ensure that sensitive data remains accessible only to authorized users. Breaches can lead to unauthorized access, resulting in data leakage or manipulation.
- Integrity: Data must remain accurate and trustworthy. If tampered with, the consequences can be dire, leading to faulty decisions based on flawed information.
- Availability: Ensuring the data is available when needed is crucial for operational efficiency. Denial of Service attacks, for instance, can prevent access to essential information.
Additionally, the regulatory landscape surrounding data protection has become more stringent. Compliance with regulations, such as GDPR and CCPA, is not just a legal requirement but also a matter of maintaining trust with customers and stakeholders. As organizations navigate these complexities, the repercussions of neglecting data security can extend beyond financial losses to long-term reputational damage.
"In a world where data drives decisions, compromising that data can jeopardize the entire organizational framework."
Understanding the importance of data security leads to a more informed approach to managing big data. Organizations must invest in robust security measures tailored to their specific environments. Through this understanding, they can strive not only for compliance but also for a competitive edge in their respective fields.
The Landscape of Big Data Security
Big data security is becoming critical. Organizations utilize massive datasets to gain insights and drive decisions. However, this dependence on expansive data brings significant risks that need addressing. Understanding the landscape of big data security helps identify vulnerabilities and tailor solutions accordingly. Several factors make this area particularly complicated, including the variety of big data environments and the security challenges they face.
Types of Big Data Environments
Big data operates in various environments that include cloud platforms, on-premises data centers, and hybrid systems. Each environment has unique characteristics and security requirements.
- Cloud Platforms: Services like Amazon Web Services (AWS), Google Cloud Platform, and Microsoft Azure allow companies to store vast amounts of data off-site. While these platforms offer flexibility and scalability, they also expose datasets to potential breaches.
- On-Premises Data Centers: Here, organizations maintain their data within their physical space. This control can be advantageous for security, but it also requires significant investment in infrastructure and skilled personnel to manage and protect sensitive information.
- Hybrid Systems: Many organizations use a combination of both cloud and on-premises solutions for better functionality and performance. However, this combination can complicate security measures, creating more points of vulnerability.
Each of these environments requires different strategies and technologies to safeguard data. Therefore, it is essential to identify the structure of one's big data landscape to implement effective security measures.
Common Security Challenges
Organizations face several security challenges within big data contexts. Addressing these involves understanding the specific threats that can compromise data integrity, confidentiality, and availability.
- Data Breaches: Unauthorized access to sensitive information remains a primary threat. Whether through insider threats or external attacks, the risk of data exposure is alarming.
- Inadequate Security Protocols: Many organizations struggle to implement comprehensive security policies that keep pace with evolving technology. Poorly enforced protocols can lead to vulnerabilities.
- Data Privacy Issues: With increasing regulations, ensuring compliance with data privacy laws, such as GDPR or CCPA, becomes another challenge. Non-compliance can lead to significant fines and damage to reputation.
- Insufficient Monitoring and Response: Many systems lack the necessary monitoring tools to detect potential threats adequately. Without real-time analytics and an incident response plan, organizations can respond too slowly to mitigate damage.
These challenges highlight the necessity for an organized and systematic approach to managing security in any big data environment. Addressing them proactively not only secures data but also builds trust with clients and stakeholders.
"As organizations increasingly rely on large datasets, the potential vulnerabilities due to data breaches and inadequate security protocols are magnified."
Understanding the broader landscape of big data security allows organizations to recognize their unique vulnerabilities and prioritize effective security measures.
Data Breaches and Their Impact
Data breaches represent a critical concern when examining security issues within the realm of big data. They reveal the vulnerabilities that can compromise the integrity, confidentiality, and availability of sensitive information. Understanding the nuances of data breaches is essential for organizations that rely on vast datasets for decision-making. The rise in the volumes and varieties of data, coupled with sophisticated cyber threats, enhances the importance of addressing this topic. A breach is not just a loss of data; it significantly impacts organizations and their stakeholders in profound ways.
Prevalence of Data Breaches
The frequency of data breaches is alarming. Recent statistics show that millions of records are exposed every day across various sectors. In 2022 alone, there were reports of over 4,000 data breaches in different industries. This trend indicates that breaches happen almost every minute. The prevalent types of data involved include personally identifiable information (PII), payment details, and corporate secrets. Organizations that experience these crises can face exposure to immense financial costs, legal ramifications, and damage to their reputation.
"Over 4,000 data breaches reported in 2022 highlight the urgency of addressing security in big data environments."
Factors contributing to this rise include increased hacking attempts, insider threats, and implementation of inadequate security measures. With the expansion of the Internet of Things (IoT) and cloud computing, the attack surface continues to widen, facilitating greater vulnerabilities. It is imperative for companies to continuously monitor and improve their security practices.
Consequences of Data Breaches
The aftermath of a data breach extends far beyond immediate financial losses. The consequences can be categorized as follows:
- Financial Costs: The cost of dealing with a data breach can be monumental. Organizations often face expenses related to remediation, investigation, and potential compensation.
- Reputation Damage: Trust is vital in the business world. A breach can damage an organization’s reputation and customer loyalty, leading to declining sales.
- Legal Repercussions: Many organizations face lawsuits and regulatory fines when they fail to protect data adequately. Compliance violations can lead to significant penalties under regulations such as the General Data Protection Regulation (GDPR).
In addition, the impact can be felt across a broader range of stakeholders. Employees may experience job insecurity, while customers could find their data misused or exposed to fraud. The overall effect of a breach diminishes the perceived value of data, which is crucial in a data-driven economy.
To navigate this landscape, organizations should consider the implementation of comprehensive data security programs, regular audits, and employee training on security awareness.
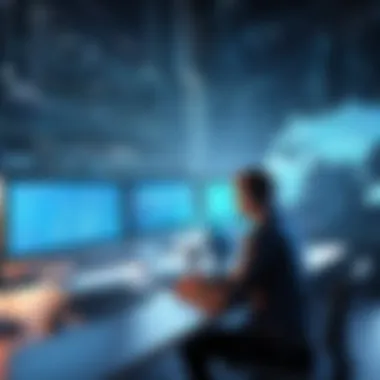
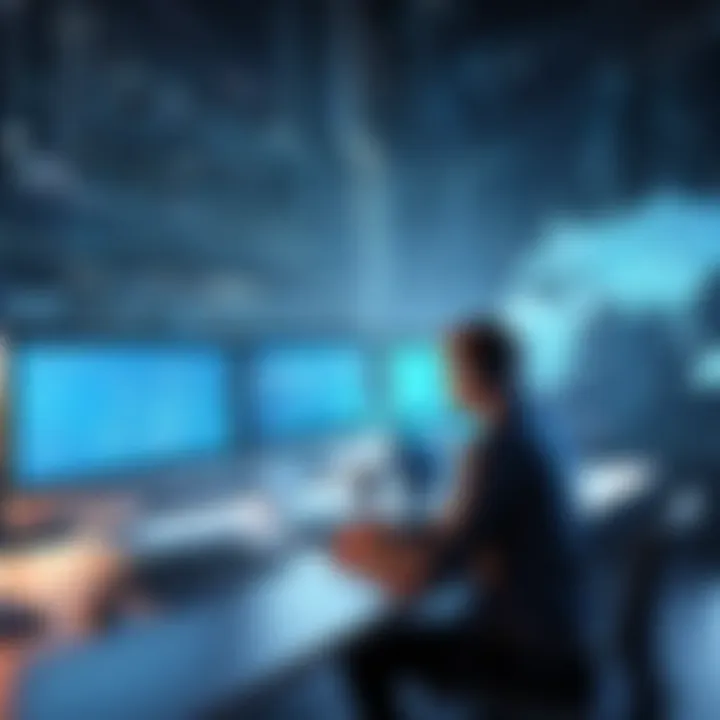
Threat Vectors in Big Data Security
In the realm of big data security, understanding threat vectors is essential. These vectors are the possible points through which an attack can be initiated. Identifying these vectors helps organizations create robust security measures.
Recognizing the importance of threat vectors is critical in preventing unauthorized access and data breaches. The big data environment is diverse and constantly evolving, giving rise to complex security landscapes. By thoroughly understanding these vectors, organizations can better prepare to combat potential attacks, thus safeguarding sensitive information and maintaining trust.
Insider Threats
Insider threats represent a significant risk to data security. These threats can arise from current or former employees, contractors, or business partners who have inside information. Insider threats can be categorized into two types: malicious insiders and negligent insiders. Malicious insiders may exploit their access for personal gain, while negligent insiders might inadvertently expose data due to carelessness.
Effective management of insider threats revolves around a few key strategies:
- Access Control: Limiting data access based on roles helps minimize exposure.
- Monitoring and Auditing: Regular oversight of user activities can identify malicious actions early.
- Cultural Awareness: Fostering a company culture focused on security can deter potential insider threats.
Understanding the behavior and motivations of employees is crucial for minimizing insider threats. Organizations can benefit from strong security policies and training programs that emphasize the importance of data protection.
"Protecting data from insider threats requires vigilance and a proactive approach."
External Attacks
External attacks pose another serious threat to big data security. These attacks come from outside the organization, typically through the Internet, utilizing various techniques such as phishing, malware, and Distributed Denial of Service (DDoS) attacks. The landscape of external threats is ever-changing, requiring organizations to stay informed and adaptable.
Some common types of external attacks include:
- Phishing: Deceptive emails designed to trick employees into revealing sensitive information.
- Ransomware: Malicious software that encrypts data and demands payment for access.
- DDoS: Overloading a system to make it unavailable, affecting operations.
To mitigate external threats, organizations should consider implementing the following practices:
- Firewalls and Intrusion Detection Systems: These technologies help block unauthorized access and detect suspicious activities.
- Regular Software Updates: Keeping systems up-to-date can address vulnerabilities before they are exploited.
- Employee Training: Continuous education on security awareness can empower employees to recognize and respond to potential threats.
In summary, both insider threats and external attacks create complex challenges for securing big data. A comprehensive approach, considering these threat vectors, is essential for maintaining data integrity and confidentiality.
Regulatory Compliance Challenges
In the realm of big data security, regulatory compliance represents a critical pillar. Organizations must navigate a complex landscape of laws and regulations that govern how data is collected, stored, and processed. Non-compliance not only leads to heavy financial penalties but can also tarnish a company’s reputation, making it imperative for businesses to stay abreast of changing legal frameworks.
Different regions present differing regulations, each with unique requirements that an organization must consider before handling data. Failure to comply can result in significant liabilities, as regulatory bodies are increasing their scrutiny over data practices. Therefore, understanding these requirements is crucial for any organization engaged in big data operations.
The benefits of robust compliance strategies are significant. They promote trust between organizations and consumers, enhance data security practices, and ultimately result in a more secure environment for all stakeholders involved. Moreover, well-defined regulatory frameworks can streamline operations and facilitate smoother data management processes, allowing organizations to focus on their core competencies instead of constantly revisiting compliance issues.
"The cost of compliance is minimal compared to the consequences of non-compliance."
Global Privacy Regulations
Global privacy regulations are diverse and often contradictory. The General Data Protection Regulation (GDPR) in the European Union sets a high standard for data protection and privacy. It emphasizes the need for user consent when collecting data and grants individuals rights over their personal information. Similarly, the California Consumer Privacy Act (CCPA) introduces stringent requirements that organizations must follow when dealing with consumer data in California.
Understanding these laws is essential, especially for companies operating in multiple jurisdictions. Failure to comply can result in massive fines and sanctions. Each regulation carries its own set of obligations which organizations must adhere to, such as:
- Implementing data protection by design and by default.
- Facilitating consumer rights to access, correction, and deletion of their data.
- Appointing data protection officers where required.
Organizations must conduct thorough audits of their data practices to ensure adherence to these global regulations, integrating compliance as a core component of their business strategy.
Sector-Specific Requirements
Certain sectors impose additional compliance requirements due to the sensitive nature of the data they handle. For example:
- Healthcare: The Health Insurance Portability and Accountability Act (HIPAA) mandates strict confidentiality and security measures for patient information.
- Finance: The Sarbanes-Oxley Act (SOX) emphasizes the importance of accurate financial reporting and requires organizations to maintain detailed audit trails.
Sector-specific requirements can significantly influence how organizations manage and protect their data. These regulations often carry heavy penalties for non-compliance and can also impact an organization’s operational capabilities.
In these sectors, organizations must ensure:
- Compliance training is provided to all employees.
- Security practices align with specific regulatory obligations.
- Regular accountability audits are conducted to prove compliance.
Staying compliant in the face of these requirements not only helps organizations avoid legal repercussions but also fosters industry trust and enhances operational integrity.
Technological Solutions for Big Data Security
In an era where data breaches are rising, it is crucial to adopt robust security measures. Technological solutions for big data security serve as a frontline defense against threats to data integrity, confidentiality, and availability. These solutions not only mitigate risk but also enhance trust among stakeholders. Organizations depend on big data analytics for decision-making, making it vital to secure this data. The implementation of effective security technologies ensures smoother operations and compliance with regulations.
Data Encryption Techniques
Data encryption is a cornerstone of any security strategy in the realm of big data. It involves encoding information to prevent unauthorized access. There are two primary types of encryption: symmetric encryption and asymmetric encryption. Symmetric encryption uses the same key for both encryption and decryption, making it faster but less secure. Asymmetric encryption involves a pair of keys, enhancing security but at the cost of speed.
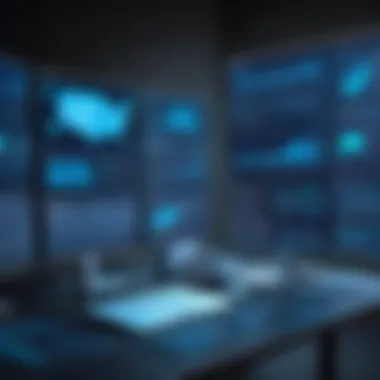
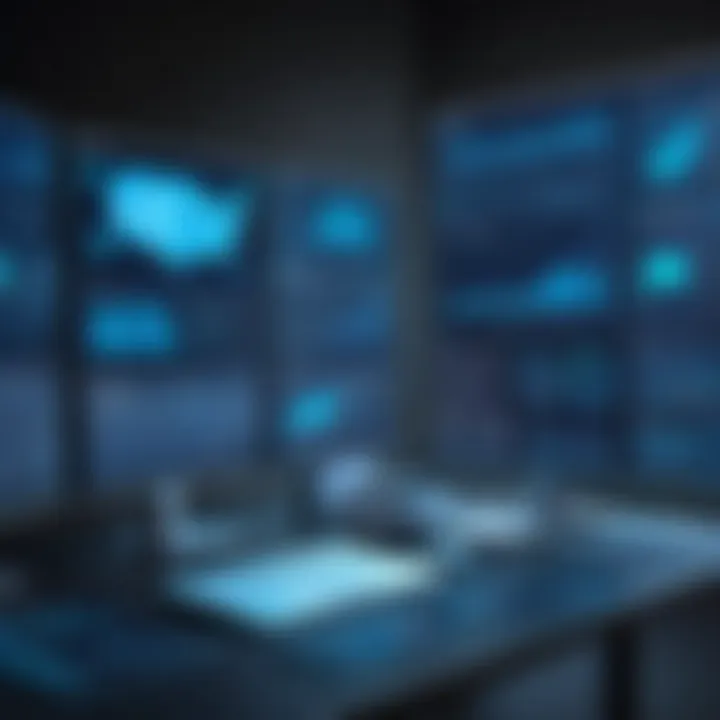
When applying encryption to big data, organizations must consider:
- Performance Impact: Encryption can slow down data processing.
- Key Management: Proper handling of encryption keys is critical for maintaining security.
- Compliance: Adhering to regulations such as GDPR, which mandate data protection through encryption.
Implementing strong encryption techniques will assure that sensitive data remains secure even if it is accessed without authorization.
Access Control Mechanisms
Securing big data goes beyond encryption; it also includes implementing effective access control mechanisms. Access control ensures that only authorized users can access certain data sets. There are various access control models:
- Role-based Access Control (RBAC): Limits access based on user roles.
- Attribute-based Access Control (ABAC): Grants access based on user attributes and environmental factors.
Utilizing these models can help organizations tailor their access policies. It's important to regularly review and update access permissions, adapting to changes within the organization. Using strong authentication methods such as multi-factor authentication reduces the risk of unauthorized access.
Anomaly Detection Systems
Anomaly detection systems play a crucial role in identifying unusual patterns that may indicate a breach. These systems use algorithms to analyze data traffic and flag any activities deviating from the norm. Early detection can significantly decrease the potential impact of a security incident. Organizations can deploy several methods for anomaly detection:
- Statistical Techniques: Identify outliers by establishing a baseline for normal behavior.
- Machine Learning Algorithms: These can learn over time, improving detection capability.
"Anomaly detection is not just about identifying breaches; it's about anticipating threats before they escalate."
Integrating anomaly detection with existing security measures can create layered defenses. This approach fosters a proactive security culture while enhancing the organization’s ability to respond to threats quickly.
Best Practices for Securing Big Data
Securing big data is a multifaceted challenge that requires strategic approaches tailored to the unique characteristics of large datasets. As organizations harness big data for insights and innovation, they must prioritize security measures to protect sensitive information from unauthorized access and breaches. Implementing best practices not only mitigates risks but also fosters trust among stakeholders. This section examines essential best practices that every organization should adopt to strengthen their data security posture.
Implementing Security Policies
Establishing clear security policies is vital in the realm of big data security. A well-defined policy acts as a foundational guideline for all data handling and usage processes within an organization. It sets the tone regarding data access, incident response, and compliance requirements. Effective security policies should encompass:
- Data Classification and Handling: Specify how various types of data should be categorized and managed based on sensitivity.
- Access Controls: Define who has access to data, under what conditions, and the protocols for revoking access when necessary.
- Incident Response Plans: Ensure there are steps ready for addressing data breaches, including communication protocols and mitigation strategies.
"A strong security policy not only protects the data but also enhances organizational resilience against threats."
Regular Security Audits
Conducting regular security audits is essential for identifying potential vulnerabilities and ensuring compliance with internal policies and external regulations. Audits provide an opportunity to review existing security measures and assess their effectiveness. Key aspects of security audits include:
- Risk Assessment: Identify potential security risks associated with big data environments, including infrastructure and application vulnerabilities.
- Policy Compliance: Review adherence to established security policies and identify gaps that need addressing.
- System Monitoring: Evaluate systems and processes for detecting anomalies or unusual activity that could indicate a breach.
Frequent audits allow for proactive identification of weaknesses before they can be exploited. This practice not only improves security but also instills confidence in stakeholders.
Employee Training and Awareness
Human error remains one of the most significant risks to data security. Consequently, training employees on security best practices is crucial. Organizations should foster a culture of security awareness that encourages vigilance and responsibility regarding data protection. Essential elements include:
- Regular Training Sessions: Hold training on data security policies, phishing attacks, and safe data handling practices.
- Simulations and Drills: Conduct simulations to test employee responses to security incidents. This preparedness can greatly reduce reaction times in actual scenarios.
- Updates on Emerging Threats: Share information on new types of threats and how employees can identify and respond to them effectively.
By emphasizing the importance of security among employees, organizations can minimize the risk of data breaches caused by human negligence.
Case Studies of Big Data Security Failures
Understanding case studies of big data security failures provides important insights into the vulnerabilities present in these systems. By examining real-world breaches, we can identify patterns of failure, assess the impact of data breaches, and discern lessons essential for improving security protocols. Notably, these cases act as cautionary tales that highlight the necessity for robust data protection measures.
High-Profile Data Breaches
High-profile data breaches serve as critical examples of what can go wrong in the domain of big data management. Several incidents have left lasting marks on companies and consumers alike, demonstrating weaknesses in security strategies and the dire repercussions of such failures.
For instance, the Equifax breach in 2017 is a prime example where the sensitive information of approximately 147 million individuals was compromised. This breach occurred primarily due to an unpatched vulnerability in the Apache Struts framework that the company had failed to address. The aftermath included significant financial penalties, litigation expenses, and a substantial loss of consumer trust. Such an incident underscores the importance of timely updates and diligent monitoring of security systems.
Another notable case is the 2014 breach at Yahoo, which was discovered years after it occurred. Initially reported as affecting 1 billion accounts, further investigations revealed that it actually impacted all 3 billion user accounts. The attackers exploited weak security protocols, which resulted in personal information being exposed directly to threats. This case highlights the necessity for comprehensive security assessments and the need for organizations to adopt continuous threat detection strategies.
Lessons Learned
The exploration of these high-profile incidents reveals critical lessons for organizations handling big data. Firms can benefit by adopting several key takeaways:
- Proactive Vulnerability Management: Organizations must prioritize regular security updates and vulnerability assessments. Implementing a thorough patch management strategy is crucial to mitigate potential threats before they can be exploited.
- Employee Training: Staff awareness is a vital component of data security. Many breaches are a result of human error. Training employees to recognize potential phishing attempts and other social engineering tactics can decrease the likelihood of breaches.
- Robust Incident Response Plans: Having a well-defined incident response plan can make a significant difference when breaches occur. Organizations should be prepared to address security incidents quickly to minimize damage and protect sensitive data.
- Transparency with Customers: After a data breach, organizations should communicate openly with affected parties. Transparency can help rebuild trust and demonstrate responsibility in handling personal information.
Understanding past failures in big data security enables organizations to bolster their defenses and reduce future risks.
In summary, case studies of big data security failures reveal the shortcomings of existing security practices. They underscore the significance of vigilance, proactive defense mechanisms, and a culture of security awareness within organizations. This knowledge serves as a valuable asset in shaping secure and resilient big data environments.
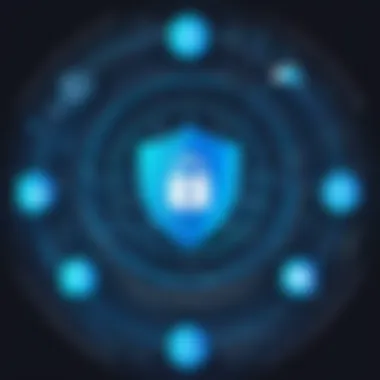
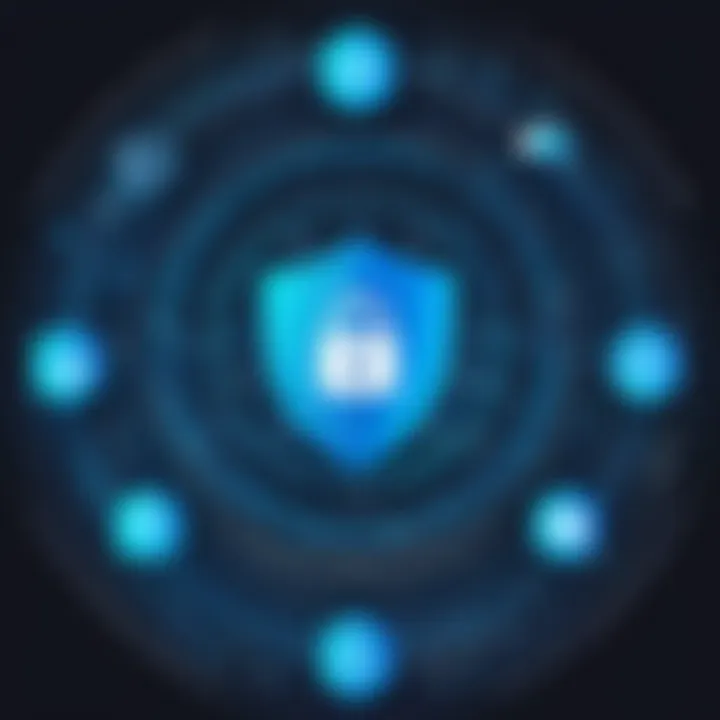
Emerging Trends in Big Data Security
The rise of big data has reshaped how organizations process and manage information. With this transformation come emerging trends that aim to overcome the security challenges inherent to vast datasets. It is essential for professionals in the tech industry to stay informed about these trends. This knowledge not only informs their strategies but also enhances their ability to protect sensitive information.
The following sections will discuss two key trends: Artificial Intelligence in Security and Blockchain Technology. Each of these innovations contributes uniquely to the evolving landscape of big data security, offering various tools and methodologies for organizations to consider.
Artificial Intelligence in Security
Artificial Intelligence (AI) is revolutionizing security measures within big data environments. AI algorithms can analyze massive datasets in real-time, identifying patterns that indicate potential threats. This capability enhances threat detection beyond the reach of traditional security measures.
Some benefits of using AI in data security include:
- Automated Threat Detection: AI systems can continuously monitor network behavior, spotting anomalies that suggest security breaches faster than human analysts could.
- Predictive Analytics: Machine learning models can forecast potential threats by analyzing historical attack data, helping organizations proactively implement security measures.
- Enhanced Response Times: AI can provide immediate reactions to detected threats, retrieving and processing data in milliseconds, which is crucial for minimizing damage.
By integrating AI technologies, organizations not only improve incident response but also strengthen their overall security posture against evolving cyber threats.
"AI's ability to learn from each interaction makes it a powerful ally in the fight against data breaches."
Blockchain Technology
Blockchain technology brings a new perspective to data security, particularly in terms of data integrity and transparency. As a decentralized system, blockchain ensures that data cannot be easily altered or tampered with, providing an additional layer of security critical for big data applications.
Some notable characteristics of blockchain technology relevant to data security are:
- Immutability: Once data is recorded in a blockchain, it cannot be changed. This feature ensures data integrity, making it difficult for malicious actors to manipulate information.
- Decentralization: By distributing data across multiple nodes in a network, blockchain mitigates the risk of a single point of failure. This reduces vulnerabilities commonly associated with centralized systems.
- Transparency: Every transaction on the blockchain is publicly visible, fostering accountability and trust among users. This transparency can help organizations identify discrepancies or unusual activity quickly.
Future Directions in Big Data Security
The rapidly evolving landscape of big data poses distinct challenges for security. As organizations integrate advanced analytics into their operations, securing data becomes paramount. Future directions in big data security delve into innovative solutions and evolving practices that can mitigate risks associated with data breaches and compliance failures. This section explores these dimensions, emphasizing the distinct elements and considerations organizations must address.
Predictive Analytics for Threat Detection
Predictive analytics is emerging as a cornerstone in big data security. This technique uses statistical algorithms and machine learning to identify potential threats before they materialize. By analyzing historical data patterns, organizations can detect anomalies or unusual behaviors that might indicate a security breach.
Key aspects of predictive analytics in security include:
- Data Mining: Mining vast amounts of data to identify patterns associated with threats.
- Real-Time Monitoring: Constant examination of network traffic and user behavior to flag suspicious activity.
- Incident Response: Facilitating swift responses through alerts generated by predictive models.
Utilizing predictive analytics not only enhances threat detection capabilities but also optimizes resource allocation. Organizations can prioritize their security efforts on areas exhibiting higher risk, allocating time and personnel efficiently. Thus, this approach not only protects data integrity but also supports a proactive security posture.
Evolving Compliance Landscape
The compliance landscape is continuously shifting, influenced by emerging technologies and global regulatory changes. Organizations must navigate through a complex array of laws pertaining to data protection, privacy, and cybersecurity. As big data technologies develop, so too do the expectations of regulators and stakeholders.
Factors influencing the evolving compliance landscape include:
- Global Regulations: Compliance with regulations such as the General Data Protection Regulation (GDPR) and California Consumer Privacy Act (CCPA).
- Industry Standards: Adhering to specific industry frameworks such as the Health Insurance Portability and Accountability Act (HIPAA) in healthcare.
- Adaptability: The need for organizations to adapt their data security strategies as regulations change.
Moving forward, organizations must embed compliance into their data governance frameworks. This means establishing clear policies, conducting regular audits, and leveraging technology to ensure adherence. An agile compliance strategy not only protects against legal ramifications but enhances organizational credibility in the marketplace.
"In the realm of big data, security is not just an option; it is a requirement that supports the continuity and trust in business operations."
Addressing these future directions in big data security ultimately defines how effectively organizations can harness the power of big data while safeguarding against vulnerabilities.
Culmination
The conclusion of this article serves a crucial role in summarizing the explorations into security issues in big data. It reflects on the multiplicity of challenges faced by organizations in an age where vast amounts of data are processed and stored. The necessity to address these security risks cannot be overstated. Organizations must prioritize data integrity, confidentiality, and availability in their operational frameworks.
One central element emphasized here is the interconnectedness of data breaches, compliance issues, and technological solutions. A robust security framework is not merely beneficial; it is essential. Potential consequences of neglecting security can range from financial losses to reputational damage.
Benefits of implementing strong security measures are significant. Enhancing security protocols facilitates trust among stakeholders, reduces the likelihood of data breaches, and ensures compliance with regulations. Therefore, organizations must consider their security strategies carefully, ensuring they are dynamic and responsive to new threats as they arise.
"In a landscape where big data vulnerabilities are increasing, adopting a proactive security approach is no longer optional; it is a prerequisite for survival."
As organizations venture deeper into the realm of big data, understanding the associated security risks will empower them to safeguard their information assets effectively. This conclusion encapsulates the pressing need to evolve security practices to stay ahead of potential threats.
Summary of Key Points
In summary, the major points outlined throughout this article include:
- Defining big data and the inherent security challenges that come with it.
- An overview of threat vectors including insider threats and external attacks.
- Understanding the implications of data breaches and their impact on organizations.
- The importance of complying with regulatory frameworks to safeguard data integrity.
- Technological advancements such as encryption and anomaly detection that provide solutions to mitigate security risks.
- Best practices for organizations to adopt to enhance their data security posture.
Call to Action for Organizations
Organizations are urged to take immediate action towards securing their big data frameworks. The following recommendations can serve as a guide:
- Conduct comprehensive security audits to identify potential vulnerabilities within existing data processes.
- Adopt new technologies like artificial intelligence and machine learning for enhanced threat detection and response.
- Train employees on data security awareness, as human error remains a significant factor in data breaches.
- Implement strict access controls and regular review measures to ensure that only authorized personnel access sensitive data.
- Stay informed about emerging regulatory requirements and ensure compliance to mitigate legal risks.
By acting on these recommendations, organizations can bolster their defense mechanisms in an increasingly data-driven world.