Navigating Product Management in Machine Learning
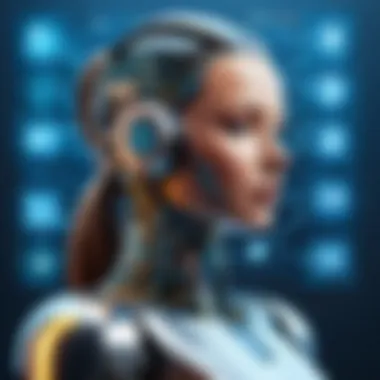
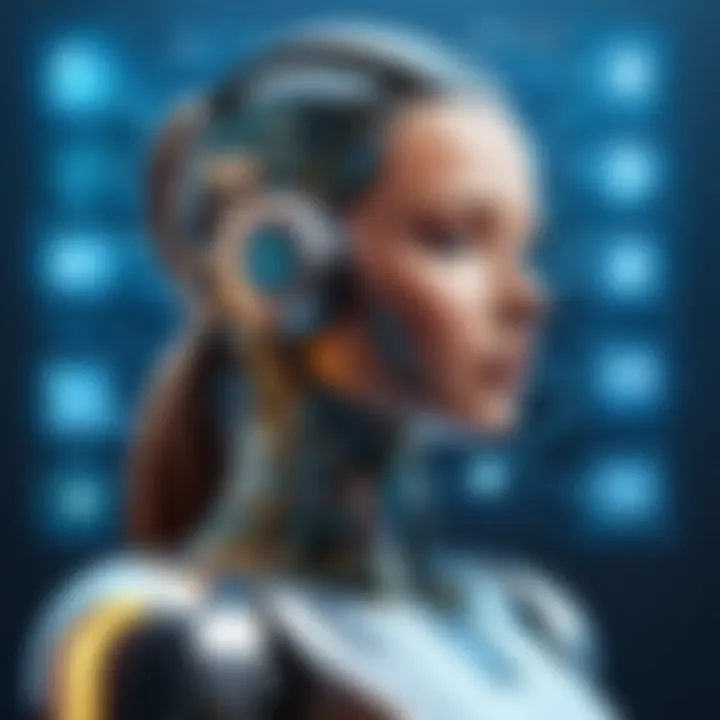
Intro
Understanding how to develop a machine learning product requires a nuanced approach. It's about aligning the technical prowess of the data science team with the business goals established by stakeholders. Some might say it’s akin to a dance; each step, each decision, influences the overall performance. Fostering effective collaborations across diverse disciplines is crucial for achieving success in this intricate environment.
In this article, we aim to unravel the key components that underpin effective product management within the sphere of machine learning. From the importance of a solid data strategy to the critical evaluation of user experience, every aspect plays a role in shaping the final product. By examining best practices and methodologies, we provide a comprehensive lens through which professionals can view their responsibilities and navigate the challenges that lie ahead.
Tech Trend Analysis
Overview of the current trend
The current wave of excitement around artificial intelligence and machine learning has transformed various industries. Organizations are increasingly embracing machine learning as a core element of their product strategies. This trend not only facilitates automation but also unlocks innovative possibilities that were previously unattainable. Companies like Google, Amazon, and Facebook deploy advanced machine learning techniques to enhance their product offerings, tailor user experiences, and drive efficiencies.
Implications for consumers
Consumers are beginning to reap the advantages of machine learning technology. From personalized recommendations to smarter customer service, it enriches the user experience like never before. With companies gathering and analyzing vast amounts of data, they can understand consumer behavior better than ever. However, this shift also raises questions about privacy and data security, as consumers become more aware of how their information is utilized.
Future predictions and possibilities
In the whirlwind world of technology, product management for machine learning hits differently than more traditional avenues. There's an air of novelty – a unique blend of data science and user-focused design that demands managers to wear many hats. Essentially, product management is the art of tailoring a product to meet market needs while ensuring it aligns with the overarching business goals. In the context of machine learning, this takes on an even deeper significance. Businesses are increasingly relying on data-driven decision-making, making it crucial to weave machine learning models seamlessly into the fabric of their product offerings.
One major consideration when diving into product management within this field is understanding the life cycle of machine learning products. Unique to this realm is the iterative nature of model development, where a single product can constantly evolve based on fresh data and user feedback. This places product managers in a pivotal position, acting as a bridge between technical teams and stakeholders in order to harmonize the often divergent perspectives on product success.
The relevance of honing in on product management strategies for machine learning cannot be overstated. With strong expertise in this area, one isn’t just creating products but rather crafting solutions that can drive real impact. Let's break down what product management entails:
Defining Product Management
At its core, product management in machine learning revolves around guiding the entire process of product development. It includes:
- Understanding user needs: Grounding product strategy in real user input.
- Prioritizing features and improvements: Distinguishing what adds genuine value versus what’s merely nice to have.
- Aligning with business objectives: Keeping the broader goals in sight, whether it’s market share, customer satisfaction, or revenue targets.
In machine learning, a product manager must also understand the technical nuances, such as model performance and data considerations. While they don’t need to be a data scientist, a basic grasp of how algorithms operate and how data informs decision-making is essential. This involves working hands-on with various stakeholders to clarify objectives and drive the product vision.
Unique Challenges of Machine Learning Product Management
Diving into machine learning means dealing with complexities not found in standard product management. Here are some of the distinctive hurdles:
- Data Dependency: The success of any machine learning project hangs on quality data. Often, managers must strategize around sourcing, cleaning, and maintaining the data pipeline. The process here can be convoluted, especially if data exists in silos.
- Model Interpretation: Machine learning models can sometimes be black boxes; deciphering what influenced a particular output may be challenging. This adds a layer of complexity in setting user expectations and explaining outcomes.
- Rapid Evolution of Technology: Keeping pace with advancements in machine learning techniques isn’t just beneficial; it’s essential. Solutions that seem cutting-edge today may become outdated in the blink of an eye, placing pressure on managers to pivot efficiently.
- Regulatory Concern: With growing data privacy laws, and ethical considerations, product managers must navigate strict guidelines that govern how data is used.
"In the relentless march of technology, those who adapt quickly and strategically will prevail."
The Product Lifecycle for Machine Learning
The Product Lifecycle for Machine Learning encompasses a series of stages crucial for the success and longevity of any product developed in this arena. Recognizing each phase helps product managers navigate the intricacies of machine learning, from the initial concept to the continuous improvement of deployed models. Acknowledging the unique characteristics of machine learning products is essential, as they differ significantly from traditional software products due to their reliance on data and algorithms.
One of the primary benefits of understanding this lifecycle is the ability to align technical and business goals throughout product development. This alignment not only streamlines processes but also fosters communication within teams, ensuring all members work towards a common objective. In a rapidly evolving landscape like machine learning, it’s imperative for product managers to stay ahead of the curve, adapting strategies as necessary based on emerging technologies and shifting user expectations.
Conceptualization and Feasibility Analysis
In the initial stage, conceptualization and feasibility analysis serve as the bedrock for any successful machine learning product. This phase begins with identifying a genuine problem that needs addressing—typically an issue where machine learning can provide measurable improvements over existing solutions. For instance, consider a retail company aiming to enhance customer personalization. By pinpointing specific user behaviors and preferences, product managers can identify how machine learning can bridge the gap.
Feasibility analysis involves assessing the data availability and quality, as these contribute directly to the potential success of machine learning models. At this stage, employing metrics such as data volume, diversity, and accuracy is key. It’s helpful to create a framework that outlines possible data sources, either internal or external, and to evaluate whether these sources can yield reliable results.
Development Phases
The development phases are where ideas start to materialize into tangible products. Unlike traditional software development, machine learning's iterative nature requires continuous cycles of training and testing. A typical approach involves using agile methodologies, allowing teams to adapt quickly to changes or challenges.
Collaboration between product managers, data scientists, and engineers is paramount here. Data scientists build and tune models while engineers focus on integrating these models into user-friendly applications. During this phase, regular meetings and check-ins help ensure that everyone remains aligned on the product vision while addressing any blockers promptly.
Launch and Market Penetration Strategies
Once development reaches completion, launching the product becomes the next focal point. A well-thought-out launch strategy is indispensable for maximizing market impact. Product managers must consider various marketing tactics, such as targeted advertising or partnering with influential tech blogs, to attract users eager for innovation. A key strategy often employed is leveraging beta testers to gather real user feedback before the final rollout.
Identifying a unique value proposition is fundamental. Users must clearly understand how the new machine learning product offers advantages over existing solutions. To this end, creating a comprehensive user guide or onboarding experience can also enhance initial interactions, setting the stage for long-term engagement.
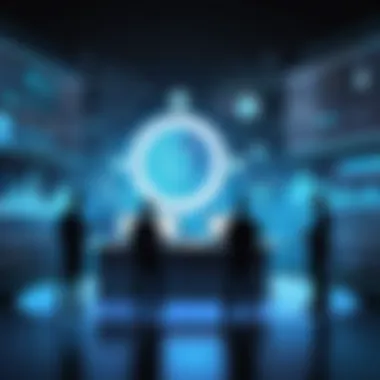
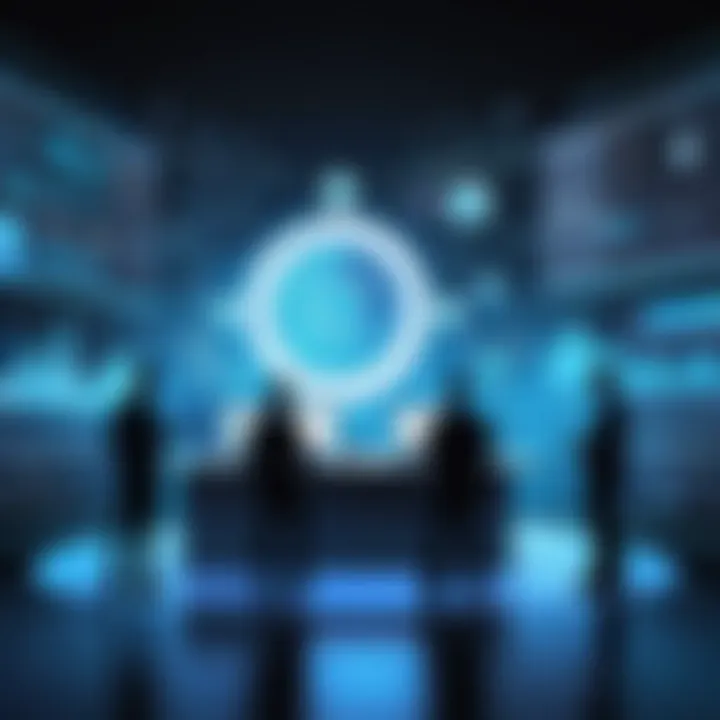
Ongoing Evaluation and Iteration
The product lifecycle does not conclude with the launch; rather, it transitions into ongoing evaluation and iteration. Continuous monitoring of user interactions, performance metrics, and satisfaction rates is critical. Utilizing tools for real-time analytics allows product managers to gather valuable insights into how well the product meets user needs.
Regular updates should be planned based on user feedback and emerging trends in machine learning. This could mean refining algorithms, enhancing user interfaces, or even pivoting the product’s focus based on market demand.
"Innovation is not the product of logical thought, although the final result is tied to logical structure."
Revisiting the data strategy frequently ensures that the model remains robust and relevant, as data drifts can lead to decreased performance over time. Effective iteration not only keeps products aligned with user expectations but also fosters a culture of adaptability within the team, paving the way for future successes.
Data Management and Strategy
Data management in the realm of machine learning is not just a supportive function; it serves as the linchpin in product management. To put it simply, effective data management can make or break a machine learning product. As the insatiable appetite for data grows, the techniques and strategies we formulate to harness that data are crucial. This section delves into the unique components of data management, underscoring its notable significance for both product managers and technical teams alike.
Understanding Data Sources
Data sources are the bedrock upon which machine learning models are built. Without a robust understanding of where data originates, a project can tilt on its axis. Data can come from various streams like public datasets, proprietary company databases, APIs, and even user-generated content. For instance, consider a product that analyzes social media sentiments. It might rely on real-time data from platforms like Twitter or Facebook, needing to navigate APIs that provide this data.
Recognizing the nuances of each data source is essential. Some sources might be rich and vibrant, while others could be sparse or laden with noise. In this dynamic field, it’s vital for product managers to cultivate a keen awareness of these data sources to guide teams effectively. Neglecting this step can result in flawed assumptions, leading to a less effective product.
Types of Data Sources
- Structured Data: Found in databases, easily searchable and quantifiable, such as sales figures.
- Unstructured Data: Non-traditional formats like emails or social posts that need more effort to analyze.
- Semi-Structured Data: A blend of both, such as JSON files, which can contain organized data but lack strict schema.
Data Quality Assessment Techniques
The adage "garbage in, garbage out" rings particularly true in the machine learning context. Ensuring the quality of data is not a mere checkbox task; it is an art form that requires careful orchestration. Product managers should prioritize robust data quality assessment techniques in their strategies to guarantee that models are trained on reliable data.
To evaluate data quality effectively, several techniques can be employed:
- Data Profiling: This technique allows managers to gain insights into data by examining its content, structure, and format. It’s akin to a health check-up; you need to know what you're dealing with before making changes.
- Statistical Analysis: Leveraging statistical methods to understand data distribution helps identify anomalies and outliers that could skew results.
- Data Validation Rules: Establishing rules and thresholds can help catch errors at the entry point, ensuring quality right from the start.
Implementing these techniques creates a safety net that ultimately enhances model performance and ensures user satisfaction.
Data Governance and Ethical Considerations
In today's environment, data is akin to currency; it holds immense value, but with power comes responsibility. Thus, establishing data governance practices is non-negotiable. This aspect of data management involves creating frameworks that dictate how data can be accessed, shared, and utilized, making transparent accountability a cornerstone of the process.
Furthermore, ethical considerations must be woven into the data governance fabric. Questions of consent, privacy, and bias need to stand at the forefront of decision-making. Unless these ethical aspects are diligently addressed, a product risks losing user trust, which can have catastrophic implications.
"Data ethics is not just adding another layer; it must cascade through every decision point."
Some important points regarding ethical data management include:
- Prioritizing user consent by being transparent about data usage.
- Regularly auditing algorithms for biases that could emerge from skewed data sets.
- Ensuring compliance with regulations such as GDPR or CCPA, avoiding the pitfalls of legal repercussions.
Taken together, these facets of data management shape the underlying architecture of machine learning products. Proper understanding and navigation of data sources, rigorous quality assessment, and steadfast governance practices all contribute to a coherent and reliable product that users can trust.
Collaboration with Technical Teams
In the realm of machine learning, the synergy between product management and technical teams plays a pivotal role. Collaboration in this context doesn't just improve processes; it can significantly impact the end product's success. Understanding how to navigate this collaboration is essential. Effective communication can bridge the gap between the often abstract world of algorithms and the more practical concerns of users and stakeholders.
Interfacing with Data Scientists and Engineers
Interfacing with data scientists and engineers can sometimes feel like trying to herd cats. The terms and methodologies can seem foreign, yet they are the backbone of machine learning products. A product manager's familiarity with core concepts like predictive modeling, feature selection, and data preprocessing is crucial. It’s not only about knowing the tech lingo; it’s about fostering an environment where data scientists and engineers feel comfortable sharing their insights and hurdles.
Effective meetings should encourage open dialogue, where questions can be raised and ideas can flow freely. For instance, using collaborative tools like JIRA or Trello keeps everyone on the same page regarding project timelines and responsibilities. These tools help demystify project progress and help address any blockers that may arise during development.
Additionally, agile methodologies can enhance these interactions. Weekly sprints allow quick feedback and ensure that all team members, including technical personnel, are aligned towards a common goal. This not only speeds up development but also strengthens team bonds.
Indeed, providing a clear roadmap and establishing frequent checkpoints can help maintain momentum. Here are a few tips for better interfacing:
- Encourage regular check-ins: Schedule consistent meetings to provide updates and discuss roadblocks.
- Foster an inclusive environment: Create a safe space where engineers and data scientists can voice their concerns without apprehension.
- Use visual aids: Diagrams or flowcharts can clarify complex concepts, ensuring everyone understands the technical aspects.
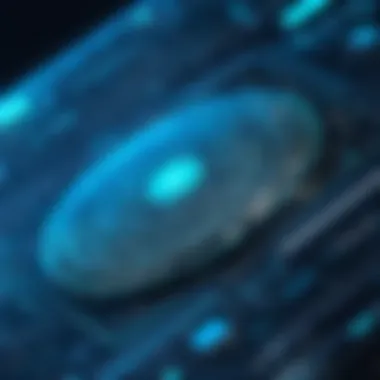
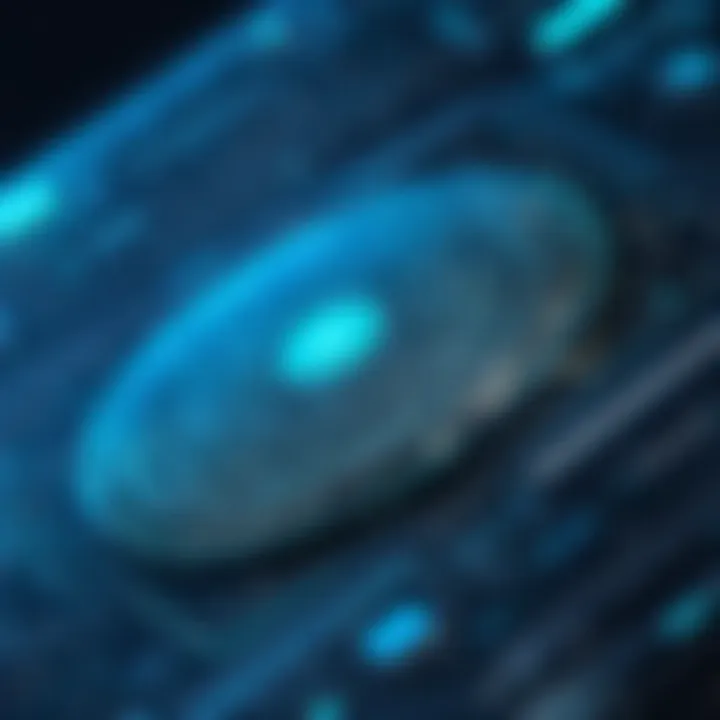
Ensuring Alignment on Goals and Metrics
Alignment on goals and metrics within product teams is essential, particularly in machine learning projects characterized by rapid iterations and evolving requirements. Without a shared understanding, there's a risk of drifting off course, leading to wasted resources and missed deadlines.
Establishing clear, measurable goals from the start provides both focus and direction. The best approach is to engage both technical teams and business units in the goal-setting process. For example, integrating business objectives with technical feasibility can result in more realistic timelines and deliverables. One effective way to ensure this alignment is by using SMART criteria—specific, measurable, achievable, relevant, and time-bound indicators.
When it comes to metrics, it can be tricky. The technical teams might look at precision and recall, while product managers often focus on user satisfaction and market impact. Bridging these perspectives is vital for mutual understanding. A possible solution is to develop a shared framework. For instance:
- Define primary KPIs: Choose key performance indicators that apply to both technical execution and user experience.
- Regularly review metrics: Hold retrospectives to ensure that everyone understands the current performance against these benchmarks.
- Celebrate shared successes: Acknowledging achievements fosters a collaborative culture and motivates all parties involved.
"A successful product is not created in isolation. The best teams unite diverse skills and perspectives to innovate and succeed."
By putting a premium on effective collaboration and alignment, product managers can leverage the full potential of machine learning technologies. This united front can drive towards not only functional products but also ones that resonate deeply with users. As the landscape of machine learning continues to evolve, the power of cohesive teamwork cannot be overemphasized.
User-Centric Design in Machine Learning Products
User-centric design stands as a fundamental element in the landscape of machine learning products. This approach emphasizes creating products that prioritize user needs, ensuring that functionality aligns seamlessly with user expectations. In the realm of machine learning, where the technology can frequently feel abstract or overly complex, leveraging user-centric design can mean the difference between a product that thrives and one that falls flat.
The importance of user-centric design is manifold. Firstly, it creates a more intuitive experience for users. If a product speaks directly to its audience, avoiding unnecessary jargon or complexity, it stands a far better chance of being embraced by its user base. Secondly, user-centric design encourages ongoing feedback, integrating user insights into the product lifecycle. This isn't just a one-off effort; it’s a continuous loop where products evolve based on real-world usage and user sentiment.
To provide clarity on why this matters in machine learning,
- Relevance: Products that align with user needs have a higher adoption rate.
- Engagement: When users feel understood, they're more likely to engage consistently with the product.
- Satisfaction: Tailoring features to resolve actual user pain points enhances overall satisfaction.
In a world rife with competition, taking the time and effort to place users at the center amplifies the relevance and effectiveness of machine learning solutions.
Understanding User Needs and Pain Points
In order to create machine learning products that resonate, product managers must develop an intricate understanding of their users. This involves digging deep to uncover the specific challenges users face within their workflows. Identifying pain points often requires combining qualitative research with quantitative data to triangulate the most pressing concerns.
To do this effectively, consider employing methods such as:
- Interviews: Engaging directly with users can yield rich insights into their experiences and challenges.
- Surveys: These tools can capture broader trends across a wider user base.
- Usability testing: Observing users interact with the product highlights areas of confusion or frustration.
By synthesizing the data gathered from these approaches, product managers can clearly delineate user profiles and behaviors, which will inform every subsequent design choice. This user-centered perspective ensures that the solutions crafted are not just technologically sound, but also directly correlate with user needs.
Designing Effective User Interfaces
The interface is the face of any product, particularly for those rooted in machine learning. A thoughtfully designed UI plays a critical role in how users interact with machine learning tools. First impressions often matter, and a clean, well-organized interface can enhance usability dramatically. It conveys professionalism and ease, which can instill confidence in users as they navigate complex tools.
When designing interfaces for machine learning products, several principles should be adhered to:
- Simplicity: Clarity of design minimizes cognitive load, allowing users to focus on tasks rather than navigation.
- Consistency: Maintaining uniformity in design elements fosters familiarity, reducing the learning curve for users.
- Feedback: Users should receive clear and immediate responses to their actions. This vital interaction reinforces their engagement and understanding of the product.
Ultimately, an effective user interface is about balance. It must be sophisticated enough to capture the capabilities of machine learning while remaining accessible to the average user. A product may boast advanced algorithms, but without an intuitive UI, even the best technology can miss its mark.
"In product management, focusing on user needs is like putting a compass in your pocket—it guides every decision you make."
Performance Metrics and Product Success
In the realm of product management, particularly within machine learning, the notion of performance metrics is not just a component; it is the backbone that shapes decision-making processes and strategic directions. Without clearly defined metrics, it becomes incredibly difficult to measure success, optimize processes, or understand user behavior. Product managers rely heavily on empirical data gathered from various performance indicators. These give insight into what’s working and what needs adjusting.
Performance metrics allow teams to align their efforts with business objectives, ensuring that every step taken leads towards achieving overarching goals. In the fast-paced environment of machine learning, where technology is continually evolving, it becomes vital to utilize metrics that accurately reflect user engagement, satisfaction, and product effectiveness.
"Performance metrics serve as the lighthouse guiding the ship of product development amidst the fog of uncertainty."
Defining Key Performance Indicators (KPIs)
Key Performance Indicators (KPIs) are the specific metrics that help gauge the progress and performance of a machine learning product. They inform product managers not only about how effective a product is but also about where improvements should be made. Defining these indicators requires careful consideration of several factors:
- Objective Alignment: KPIs must directly relate to the strategic objectives of the organization. For instance, if the goal is to improve user retention, then measuring daily active users might be a key indicator.
- Measurable Outcomes: The metrics selected should yield quantifiable data. This could include things like user engagement rates or error rates in predictions made by machine learning models.
- Relevance to Users: Understanding the end-user experience is essential. Metrics that measure customer satisfaction, like Net Promoter Score (NPS), provide qualitative insights that numbers alone cannot.
- Adaptability: As market needs and technologies evolve, KPIs should also be flexible. Regular reviews help in adjusting them, ensuring they stay relevant over time.
Establishing these KPIs effectively lays the groundwork for informed improvements and strategic pivots later in the product lifecycle.
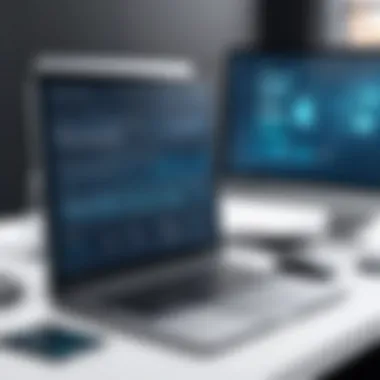
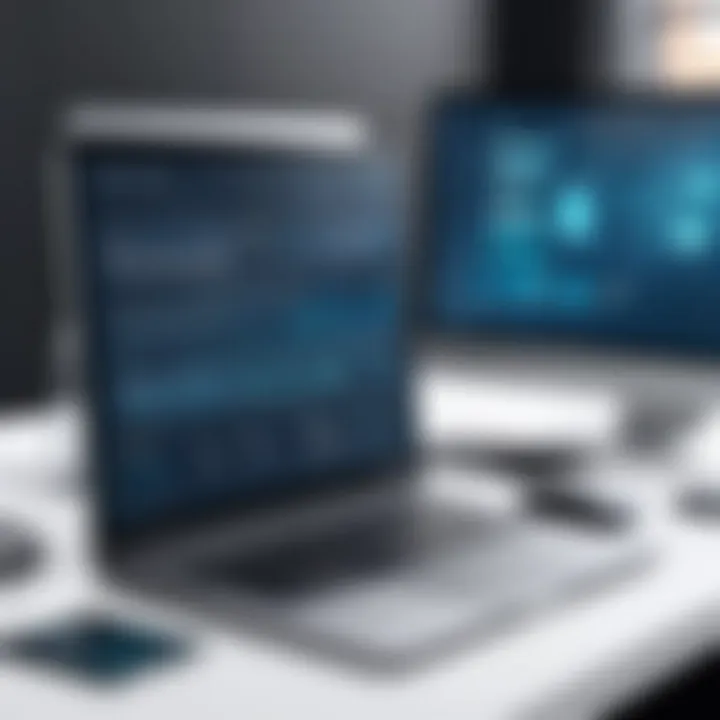
User Feedback and Iterative Improvements
User feedback is an invaluable asset in the product management toolkit, especially for machine learning products where the landscape can shift rapidly based on evolving technologies and user expectations. Gathering user feedback involves a mix of qualitative and quantitative approaches. Surveys, usability testing, and direct interviews can uncover insights that numbers alone might mask.
- Fine-Tuning Product Features: By analyzing user feedback, product managers can identify and prioritize features that customers find helpful versus those that create obstacles in their processes.
- Recognizing Patterns: Understanding patterns in user feedback can highlight systemic issues or areas of success in the product. If many users express frustration over a specific feature, it should be addressed promptly.
- Creating a Continuous Loop: This continual process of collecting feedback, making necessary improvements, followed by more itemized feedback fosters a culture of adaptability. It can lead to what is often referred to as iterative improvement, ensuring the product evolves alongside user demands.
Navigating Ethical and Regulatory Challenges
Navigating the landscape of ethical and regulatory challenges is crucial in the realm of product management for machine learning. This area encompasses a multitude of considerations that are not merely legal obligations but also moral imperatives that can shape the success and sustainability of machine learning products. As data becomes increasingly integral to the performance of models, understanding how to manage ethical implications is no longer optional—it’s a necessity.
A critical aspect of this challenge lies in addressing data privacy laws. These laws are in place to protect individuals’ information, requiring product managers to develop a keen awareness of what’s involved in collecting, storing, and utilizing data. Ignoring these regulations can not only lead to severe penalties but also damage the trust between companies and their customers.
Another significant area is the need to mitigate algorithmic bias. Without careful oversight and calibration, machine learning algorithms can inherit biases present in their training data or in the design of the models, leading to unintended and unfair outcomes—something that product managers must preemptively address.
Overall, these challenges impact how products are designed, prioritized, and deployed in the market. Thus, product managers need to embrace these nuances to create ethical, compliant, and forward-thinking machine learning applications.
Addressing Data Privacy Laws
Data privacy laws are a fundamental component in the product management lifecycle for machine learning. With regulations like the European General Data Protection Regulation (GDPR) and the California Consumer Privacy Act (CCPA), organizations are expected to adhere strictly to operational standards concerning user data. Here are some key considerations:
- Transparency: Companies must clearly inform users about what data is being collected, how it will be used, and ensure that consent is obtained.
- Data Minimization: It’s essential to collect only the data that is necessary for the intended functionality. This principle curbs unnecessary data gathering, which can lead to violations.
- User Rights: Understanding user rights under applicable laws, such as the right to access, correct, or delete personal data, is vital.
By implementing robust strategies for data governance, organizations can significantly reduce the risk of non-compliance while also building customer trust. A proactive stance on data privacy not only protects the organization but also positions it as a responsible player in the tech ecosystem.
Mitigating Algorithmic Bias
Mitigating algorithmic bias is another pressing regulatory challenge in machine learning product management. Bias can creep into algorithms through various avenues, such as biased training data or flawed assumptions made during model development. This can manifest in several detrimental ways:
- Unequal Treatment: Certain demographics may be unfairly treated if the models are not adequately representative of the population.
- Reinforcement of Stereotypes: Algorithms can unintentionally perpetuate harmful stereotypes if they reflect the biases found in the training data.
- Legal Ramifications: Companies might face backlash from regulatory bodies if their algorithms result in discriminatory outcomes.
To address these points effectively, product managers should:
- Audit Training Data: Regularly review and assess the data sets used in training to ensure they are diverse and representative.
- Incorporate Bias Detection Tools: Utilize tools and frameworks designed specifically to identify and analyze bias within algorithms before deployment.
- Engage in Continuous Learning: Stay informed about best practices in fairness and ethics in AI to adapt and refine strategies over time.
"Addressing bias in machine learning is not just about ethics; it's about guaranteeing the reliability and fairness of technology in our daily lives."
By adopting a comprehensive approach to mitigating algorithmic bias, organizations can pave the way for more inclusive and equitable machine learning products.
Future Trends in Machine Learning Product Management
In the ever-shifting landscape of technology, understanding future trends in machine learning product management isn’t just smart; it’s essential. This domain is not static; it evolves as new technologies emerge, user expectations shift, and ethical considerations become increasingly prominent. Adaptability is more than a buzzword; it's a necessity for product managers who aim to stay ahead of the curve. Recognizing these trends allows teams to harness innovations, optimize product offerings, and, ultimately, enhance user experience.
The Impact of Emerging Technologies
Emerging technologies shape the very foundation of machine learning products. Think about it—technologies like quantum computing, edge computing, and the evolution of AI algorithms can fundamentally change how products are developed and used. Quantum computing, for instance, offers computational power that could expedite complex data analysis, making machine learning models more sophisticated and faster. Similarly, edge computing allows data to be processed closer to where it’s generated, which can significantly reduce latency in applications like IoT devices.
These shifts impact product management by necessitating new strategies for integration and deployment. For example:
- Adopting Agile Methodologies: Using agile frameworks ensures teams remain flexible and can pivot quickly as new technologies come into play.
- Continuous Learning: Staying informed about upcoming tech trends can turn you from a reactive team into a proactive one. This is no small feat in a field defined by rapid change.
Taking these actions can result in not just improved efficiency in product development, but also a more engaged user base that feels the effects of these advancements. The key is balancing tech integration with user needs, ensuring that while we embrace novelty, we don’t lose sight of what the user actually requires.
"Emerging technologies are not just tools, but potential game-changers that can elevate product management practices to new heights."
Preparing for the Evolution of User Expectations
As machine learning technology advances, so do user expectations. Customers are becoming more knowledgeable and discerning about the products they use. They expect not only functionality but intuitiveness and seamless experiences. Product managers must prepare for this shift by understanding that:
- Simplicity is Key: Users are drawn to streamlined interfaces that require minimal effort to navigate. Consider products like Spotify or Netflix; their success lies in uncomplicated designs that allow for easy discovery.
- Personalization Matters: Machine learning products that leverage user data for tailored experiences have a competitive edge. For instance, recommendation algorithms that suggest content or features based on previous interactions significantly enhance user satisfaction.
- Transparency is Crucial: More users are questioning how their data is used. Acknowledging this concern by providing clear information on data practices builds trust.
This evolution affects how products are marketed and developed. Product managers need to start integrating user feedback loops that inform ongoing development. This can be achieved through:
- Utilizing surveys and interviews to gather user feedback.
- Analyzing usage data to identify pain points.
- Keeping abreast of competitor offerings to understand market expectations.
Ultimately, navigating these user expectations may require shifts in strategy, but the rewards are substantial—higher user retention, better market positioning, and a more robust relationship between the product and its users.
Finale
Recap of Key Insights
- Interdisciplinary Collaboration: The interplay between technical teams and product managers is pivotal. Constant communication fosters alignment on objectives, which is necessary for the successful delivery of machine learning products.
- User-Centric Approach: Understanding user expectations is key. Crafting an experience that resonates with the user not only enhances satisfaction but drives product adoption.
- Data Strategy and Quality: The importance of managing data effectively cannot be overstated. Analyzing data sources, ensuring quality, and adhering to governance are fundamental for building trustworthy models.
- Ethics and Regulation: In today's landscape, being aware of ethical considerations and regulatory requirements is crucial. Addressing algorithmic bias and comprehending data privacy laws can prevent missteps down the line.
- Performance Metrics: Establishing clear KPIs and continuously iterating based on user feedback solidifies a product's success trajectory.