In-depth Exploration of Healthcare Sample Data: Insights & Analysis
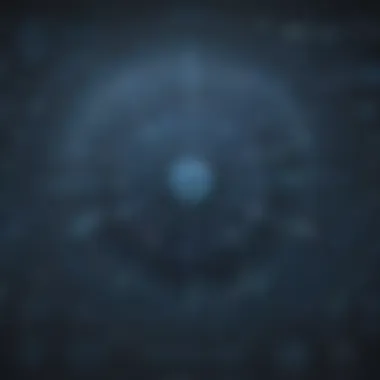
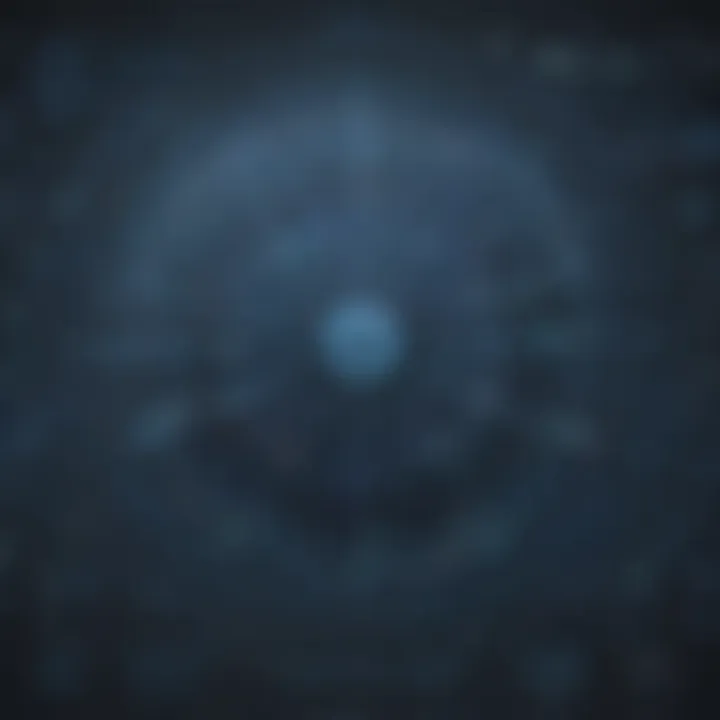
Tech Trend Analysis
Healthcare sample data analysis is currently at the forefront of tech trends, with professionals and enthusiasts eagerly exploring its implications. The insights gleaned from this data can revolutionize the industry, paving the way for improved services and outcomes. As we delve deeper into the realm of healthcare data analysis, it becomes evident that the current trend emphasizes the importance of accurate data collection methods and sophisticated statistical analysis techniques. This trend has significant implications for consumers, as it opens doors to personalized healthcare solutions and predictive analytics that can enhance patient care. Looking towards the future, the possibilities are endless, with advancements in AI and machine learning poised to transform how healthcare data is utilized and interpreted.
Product Reviews
When examining healthcare sample data, one must consider the intricacies of the products used for data collection and analysis. These products play a vital role in ensuring the accuracy and reliability of the insights derived from the data. Analyzing the features and specifications of these products is essential for understanding their capabilities and limitations. Performance analysis is key in determining the efficiency and effectiveness of these tools in processing and interpreting healthcare data. While these products offer a myriad of benefits, such as streamlined data processing and advanced analytical capabilities, they also come with their own set of drawbacks. It is imperative to weigh the pros and cons of each product carefully to make informed decisions regarding their utilization in healthcare data analysis. Based on the performance analysis and feature evaluation, recommendations can be made to guide professionals and enthusiasts in selecting the most suitable products for their specific analytical needs.
How-To Guides
For individuals looking to explore healthcare sample data analysis, a comprehensive guide is essential to navigate this intricate landscape successfully. An introduction to the topic should provide a clear overview of the importance and relevance of healthcare data analysis in today's healthcare industry. Step-by-step instructions are crucial in guiding readers through the process of data collection, analysis, and interpretation. Tips and tricks can offer valuable insights and enhance the efficiency of data analysis workflows. Additionally, troubleshooting guidelines can help address common challenges and errors that may arise during the data analysis process. By following this detailed how-to guide, both beginners and seasoned professionals can enhance their data analysis skills and extract meaningful insights from healthcare sample data.
Industry Updates
Staying informed about recent developments in the healthcare tech industry is paramount for professionals and enthusiasts engaged in healthcare sample data analysis. Analyzing market trends can provide valuable insights into the direction of the industry and potential opportunities for innovation. Keeping track of the impact of these developments on businesses and consumers is essential for making informed decisions and strategic planning. By staying up-to-date with industry updates, individuals can adapt their approaches to data analysis and leverage emerging technologies to gain a competitive edge in the healthcare sector.
Introduction to Healthcare Sample Data
Healthcare sample data analysis is a crucial element in understanding and improving the healthcare industry. By delving into the vast pool of data available, professionals can uncover trends, patterns, and insights that drive decision-making processes and enhance patient outcomes. Within the realm of healthcare data analysis, the importance of accurate data collection methods, robust statistical techniques, and insightful implications cannot be overstated. This article serves as a comprehensive guide to exploring healthcare sample data, shedding light on the intricate details that pave the way for industry advancements.
Understanding the Significance of Healthcare Data Analysis
Role of Data in Healthcare Decision-Making
Data plays a pivotal role in shaping healthcare decision-making processes. By harnessing the power of data, healthcare professionals can make informed choices regarding patient care, resource allocation, and policy formulation. The ability of data to provide evidence-based insights enhances the precision and effectiveness of decision-making within the healthcare domain. When navigating the landscape of healthcare sample data, understanding the role of data in decision-making is paramount for driving positive industry transformations.
Impact of Data Analysis on Patient Outcomes
Data analysis has a direct impact on patient outcomes, influencing the quality of care and treatment provided. Through sophisticated analytical techniques, healthcare providers can identify trends, predict potential risks, and personalize treatment approaches to suit individual patient needs. The utilization of data analysis in healthcare not only improves patient outcomes but also facilitates proactive and preventive measures that uphold the well-being of individuals. Exploring the impact of data analysis on patient outcomes is fundamental to comprehending the holistic benefits of leveraging data in healthcare settings.
Challenges in Handling Healthcare Sample Data
Data Privacy and Security Concerns
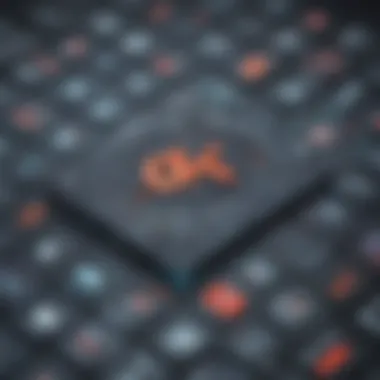
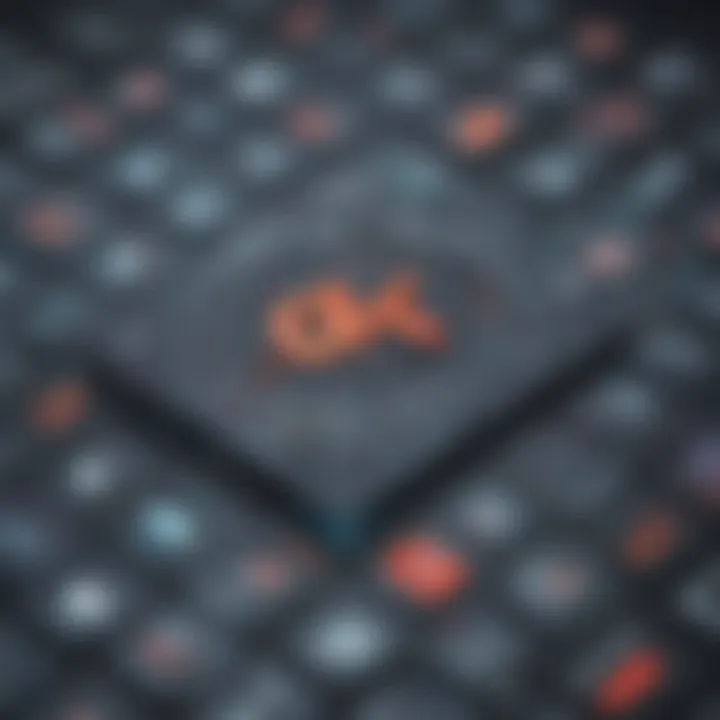
Ensuring data privacy and security in healthcare sample data is a significant challenge that demands meticulous attention. With the abundance of sensitive information stored in databases, protecting patient confidentiality and upholding data security standards are paramount. Striking a delicate balance between data accessibility and security measures poses a constant challenge for healthcare organizations striving to maintain the integrity of their data assets. Navigating the complexities of data privacy and security concerns requires a multi-faceted approach that safeguards information while allowing for meaningful data analysis.
Data Quality and Integrity Issues
Maintaining data quality and integrity is a critical aspect of healthcare sample data management. Issues such as data duplication, inaccuracies, and inconsistencies can impede the reliability and validity of analytical insights derived from the data. Addressing data quality and integrity concerns involves implementing stringent validation processes, data governance frameworks, and quality assurance protocols. By overcoming these challenges, healthcare professionals can harness the full potential of their data resources and drive impactful decision-making based on accurate and trustworthy information.
Methods of Collecting Healthcare Sample Data
Exploring the techniques for collecting healthcare sample data is a crucial aspect of this comprehensive analysis. Understanding the various methods of data collection is fundamental in ensuring the quality and relevance of the data being analyzed. By delving into primary and secondary data sources, professionals and enthusiasts can gain valuable insights into the intricacies of healthcare data. The process involves meticulous planning to gather accurate information that forms the basis of deriving meaningful conclusions and making informed decisions.
Primary Data Collection Techniques
When it comes to primary data collection, utilizing surveys and questionnaires plays a pivotal role in acquiring specific insights from healthcare samples. Surveys and questionnaires aid in gathering structured information directly from respondents, allowing researchers to analyze trends, preferences, and health-related behaviors. Their structured nature facilitates data comparison and statistical analysis, enhancing the credibility of research outcomes.
Surveys and questionnaires stand out as popular tools in the healthcare industry due to their versatility in capturing a wide range of data points efficiently. However, their reliance on self-reported information poses challenges related to respondent bias and data accuracy. Despite these limitations, their cost-effectiveness and ability to collect data from a large sample size make them a preferred choice in healthcare research.
In contrast, interviews and focus groups provide qualitative insights through direct interactions with participants. By engaging in in-depth conversations, researchers can explore nuanced perspectives and uncover underlying motivations behind healthcare decisions. The interactive nature of interviews and focus groups enables researchers to delve deeper into complex healthcare issues, fostering a comprehensive understanding of patient experiences and perceptions.
Interviews and focus groups excel in capturing rich, contextual data that may not be easily obtained through surveys alone. However, their resource-intensive nature and potential for interviewer bias necessitate stringent methodologies to ensure data validity and reliability. Despite these challenges, interviews and focus groups offer a profound depth of information essential for holistic healthcare analysis.
Secondary Data Sources
Secondary data sources such as Electronic Health Records (EHR) and Health Insurance Claims Data contribute significantly to enriching healthcare sample analysis. Leveraging EHR data provides a wealth of medical information, including patient history, treatment outcomes, and diagnostic details. This electronic repository simplifies data access and retrieval, offering researchers a comprehensive view of individual and population health trends.
Electronic Health Records stand out for their efficiency in providing real-time, longitudinal data for research purposes. The structured nature of EHR data enables complex analyses relevant to healthcare decision-making, clinical research, and policy development. However, challenges related to data interoperability and privacy concerns necessitate robust protocols to ensure data security and confidentiality.
On the other hand, Health Insurance Claims Data offer insights into healthcare utilization, costs, and service patterns within the insurance domain. Analyzing claims data helps identify trends in healthcare delivery, reimbursement practices, and disease prevalence, guiding evidence-based interventions and policy adjustments. Health Insurance Claims Data present opportunities for cross-sector collaborations and health system improvements through data-driven decision-making.
Health Insurance Claims Data serve as a valuable resource for examining healthcare economics, coverage gaps, and pattern analysis. Despite their utility in enhancing healthcare planning and resource allocation, issues related to data accuracy and completeness require vigilance to prevent erroneous conclusions. By combining primary and secondary data sources, healthcare professionals can paint a comprehensive picture of the industry landscape for informed decision-making and policy formulation.
Statistical Analysis of Healthcare Data
In the realm of healthcare data analysis, statistical analysis plays a vital role, serving as the cornerstone for extracting meaningful insights and patterns from vast datasets. Statistical analysis is instrumental in uncovering trends, relationships, and anomalies within healthcare data, offering invaluable information for decision-making processes. By utilizing statistical methods, healthcare professionals can make informed assessments, devise effective strategies, and drive improvements in patient care and outcomes.
Descriptive Statistics in Healthcare Research
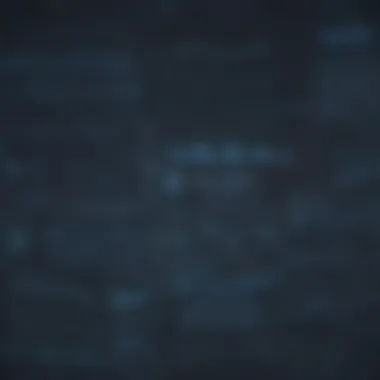
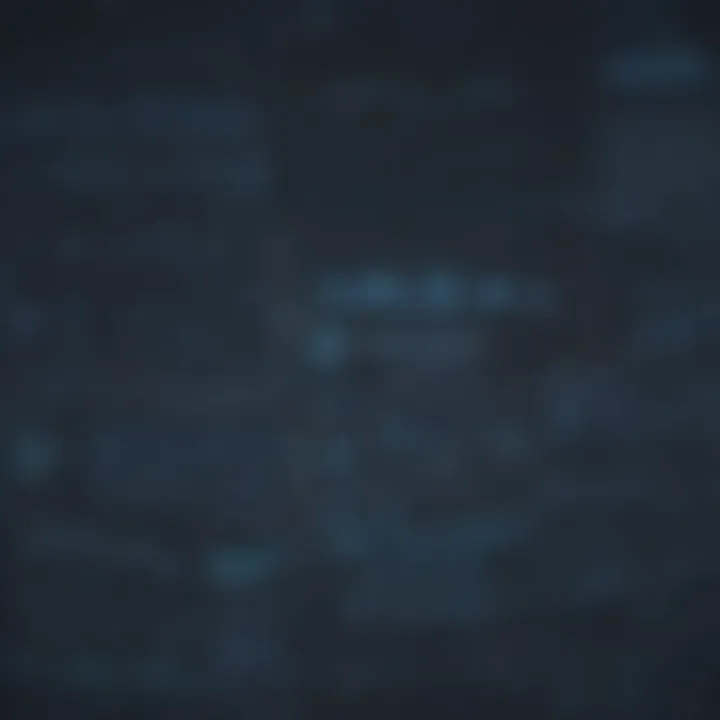
Mean, Median, and Mode Calculations
Mean, median, and mode calculations stand as fundamental tools in descriptive statistics, providing a summary of numerical data distribution. The mean represents the average of a dataset, offering a central tendency measure that is sensitive to extreme values. On the other hand, the median denotes the middle value when data is arranged in ascending order, offering a robust measure of central tendency that is unaffected by outliers. Additionally, the mode signifies the most frequently occurring value in a dataset, reflecting its peak occurrence. These calculations aid in understanding the typical values and distribution pattern of healthcare data, enabling researchers to grasp the data's central characteristics and variability effectively.
Frequency Distributions
Frequency distributions showcase the spread and dispersion of values within a dataset, presenting how frequently each value occurs. By organizing data into intervals or categories and depicting the frequency of observations within each segment, frequency distributions offer a comprehensive overview of data patterns and variability. This graphical representation allows researchers to visualize the distribution shape, central tendencies, and variability present in healthcare data. Understanding frequency distributions is crucial for interpreting data trends, identifying outliers, and determining the data's level of skewness or symmetry. Analysts can leverage frequency distributions to gain insights into the prevalence and distribution patterns of healthcare variables, facilitating informed decision-making and strategic planning.
Inferential Statistical Techniques
Hypothesis Testing
Hypothesis testing is a key inferential statistical technique used to assess the validity of assumptions or claims made about a population based on sample data. By formulating a null hypothesis and an alternative hypothesis, analysts can statistically evaluate whether the sample data provide sufficient evidence to support rejecting or failing to reject the null hypothesis. Hypothesis testing enables researchers to draw conclusions, make inferences, and generalize results from sample data to the broader population. This method aids in validating research hypotheses, drawing reliable conclusions, and establishing the statistical significance of findings in healthcare research.
Regression Analysis
Regression analysis encompasses a set of statistical methods utilized to model and analyze the relationship between variables. This technique aims to predict the impact of independent variables on a dependent variable, elucidating how changes in one variable affect the other. Regression analysis enables researchers to identify correlations, evaluate the strength and direction of relationships, and predict future outcomes based on historical data. By fitting regression models to healthcare datasets, analysts can uncover meaningful associations, identify influential factors, and forecast trends or outcomes with a degree of confidence. Regression analysis serves as a powerful tool for predictive modeling, outcome prediction, and decision support in healthcare data analysis.
Implications of Healthcare Data Analysis
In this section of the article, we will delve into the essential topic of Implications of Healthcare Data Analysis, which plays a pivotal role in revolutionizing the healthcare industry. By analyzing healthcare data, professionals can extract invaluable insights that pave the way for significant improvements in patient care, outcomes, and health policy decision-making. The meticulous analysis of healthcare data opens doors to personalized medicine approaches and predictive analytics for disease management, offering a tailored and proactive healthcare strategy.
Improving Patient Care and Outcomes
Personalized Medicine Approaches
Personalized medicine approaches are a groundbreaking aspect of healthcare that tailors medical treatment to individual patients based on their genetic makeup, lifestyle, and environmental factors. This customized approach ensures that patients receive treatments that are specifically designed to address their unique health needs. The key characteristic of personalized medicine is its focus on precision and individualization, moving away from traditional one-size-fits-all medical interventions.
This approach has gained popularity due to its ability to enhance treatment efficacy, minimize adverse effects, and optimize patient outcomes. By customizing treatments to suit each patient's genetic predispositions, personalized medicine offers targeted therapies that are more effective and less likely to cause complications, ushering in a new era of patient-centric healthcare.
Predictive Analytics for Disease Management
Predictive analytics for disease management harnesses the power of data to forecast potential health outcomes and identify high-risk individuals who may benefit from early intervention. By analyzing past medical records, lifestyle factors, and demographic information, predictive analytics can anticipate disease progression, enabling healthcare providers to intervene preemptively.
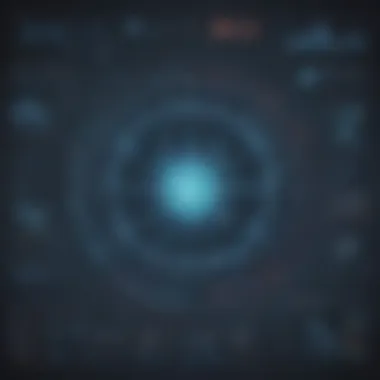
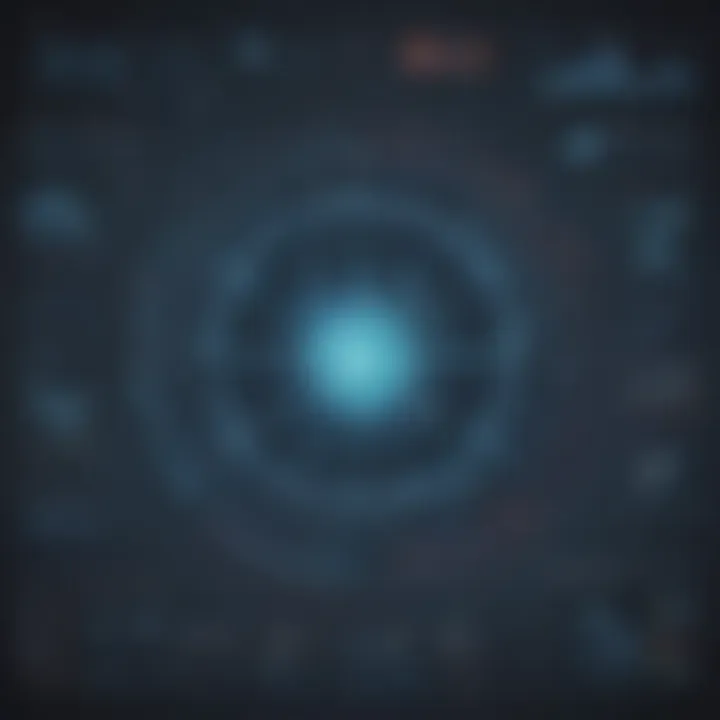
The key characteristic of predictive analytics lies in its ability to identify patterns and trends in data that can predict future health conditions accurately. This proactive approach allows healthcare professionals to offer preventive measures, personalized treatment plans, and targeted interventions to improve patient outcomes and quality of life.
Health Policy and Decision-Making
Evidence-Based Policy Formulation
Evidence-based policy formulation relies on empirical evidence and data insights to shape healthcare policies that are grounded in research and proven effectiveness. This approach ensures that policy decisions are driven by data-driven analysis, leading to strategies that are more likely to succeed in improving overall health outcomes.
The key characteristic of evidence-based policy formulation is its emphasis on measurable outcomes and scientific evidence to support policy decisions. By basing policies on rigorous research and empirical data, decision-makers can implement strategies that have been proven to enhance healthcare quality, effectiveness, and cost-efficiency.
Resource Allocation Strategies
Resource allocation strategies involve optimizing the distribution of healthcare resources to maximize efficiency, cost-effectiveness, and quality of care. By strategically allocating resources based on data-driven insights, healthcare providers can ensure that resources are allocated where they are most needed, minimizing waste and enhancing overall healthcare delivery.
The key characteristic of resource allocation strategies is their focus on equitable distribution and optimal utilization of resources to meet the diverse and evolving healthcare needs of communities. By prioritizing the allocation of resources based on data-driven assessments, healthcare decision-makers can foster sustainable and inclusive healthcare systems that prioritize patient well-being and equitable access to services.
Future Trends in Healthcare Data Analysis
In the realm of healthcare data analysis, the exploration of future trends holds significant importance for professionals and enthusiasts alike. Understanding and adapting to the evolving landscape of data analytics is crucial in maximizing the potential benefits for the healthcare industry. By delving into the incorporation of cutting-edge technologies such as Artificial Intelligence (AI) and Blockchain, experts can harness valuable insights, improve decision-making processes, and enhance patient outcomes. These trends not only represent the forefront of innovation but also pave the way for more efficient and effective healthcare practices that align with the demands of modern-day data-driven healthcare environments.
Integration of Artificial Intelligence
Machine Learning Applications
Exploring the realm of Machine Learning Applications within the context of healthcare data analysis unveils a myriad of opportunities and advancements. Machine learning, a subset of AI, empowers systems to learn from data, identify patterns, and make decisions with minimal human intervention. The utilisation of machine learning algorithms offers a sophisticated approach to analysing vast amounts of healthcare data swiftly and accurately, enabling healthcare professionals to extract valuable insights for enhancing patient care and optimizing healthcare services.
Natural Language Processing in Healthcare
An in-depth examination of Natural Language Processing (NLP) within healthcare data analysis reveals the transformative potential of linguistic data processing. NLP leverages AI algorithms to interpret and analyze human language, enabling systems to comprehend, interpret, and generate human-like text. In the healthcare domain, NLP facilitates the extraction of valuable insights from unstructured data sources such as medical notes, research articles, and patient records. This transformative technology accelerates the analysis process, improves data accuracy, and enhances decision-making capabilities within healthcare organizations.
Blockchain Technology in Healthcare Data Management
Within the realm of healthcare data management, the integration of Blockchain technology is heralded as a formidable solution to enhance data security and streamline interoperability. The architecture of Blockchain, characterized by its decentralized and tamper-evident nature, ensures the secure storage and transmission of healthcare data across interconnected networks. By leveraging Blockchain's immutable ledger and cryptographic algorithms, healthcare organizations can fortify data security, mitigate the risks of data breaches, and maintain the integrity of sensitive patient information.
Enhancing Data Security
Diving into the facet of Enhancing Data Security through Blockchain technology illuminates the proactive measures undertaken to safeguard healthcare data assets. The utilization of cryptographic techniques and distributed consensus mechanisms within Blockchain architecture fortifies data protection, minimizes the vulnerabilities of centralized data repositories, and safeguards against unauthorized access or manipulation. By embedding enhanced data security features, healthcare entities can bolster patient trust, uphold regulatory compliance, and fortify data integrity within their information ecosystems.
Streamlining Interoperability
Exploring the domain of Streamlining Interoperability in healthcare data management unveils the transformative impact of Blockchain technology in optimizing data exchange and accessibility. By establishing standardized data protocols, smart contracts, and decentralized data sharing mechanisms, Blockchain enhances interoperability among disparate healthcare systems and stakeholders. This seamless data integration fosters seamless communication, accelerates data transactions, and simplifies the interoperability challenges encountered in cross-institutional healthcare data exchange, ultimately improving the continuum of care and operational efficiency within the healthcare ecosystem.