Unlocking the Potential of Graphs in Statistical Analysis for Data Insights
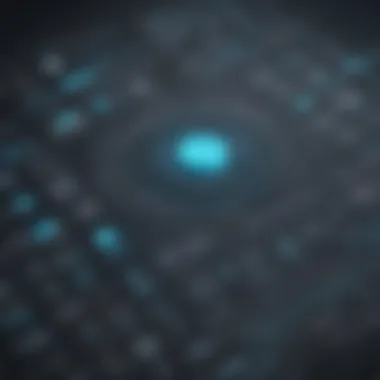
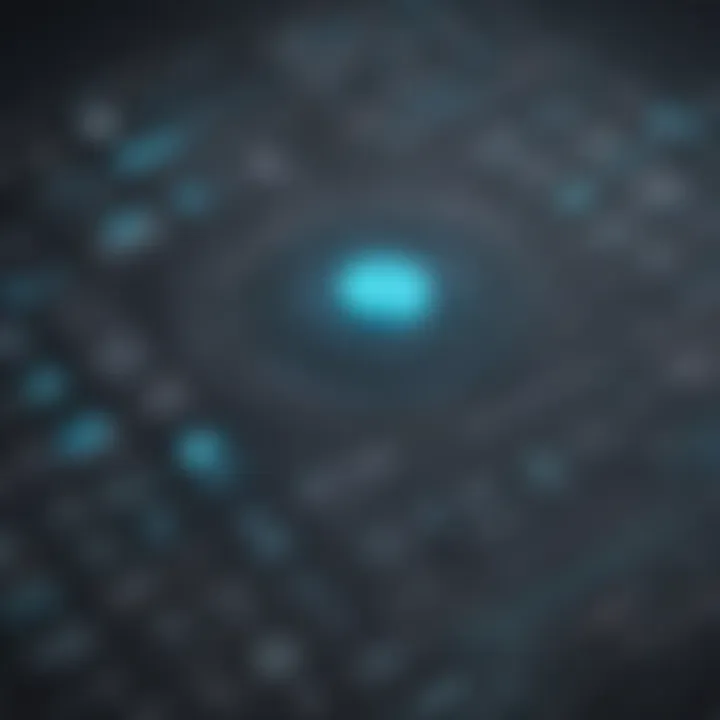
Tech Trend Analysis
Graphs have become indispensable tools in statistical analysis, revolutionizing the way data is visualized and interpreted. The current trend towards harnessing the power of graphs underscores the shift towards more dynamic and interactive data representation. This trend carries significant implications for consumers, as it promotes a deeper understanding of complex datasets and allows for more informed decision-making. Looking ahead, the future predictions and possibilities in graph utilization suggest continued advancements in data visualization technologies, opening new avenues for exploration and analysis.
How-To Guides
Introduction to the Topic:
Before delving into the intricacies of leveraging graphs for statistical analysis, it is essential to understand the fundamental principles behind graph creation and interpretation. By grasping the basics of graphing techniques, individuals can effectively convey information, identify trends, and draw valuable insights from data. This section serves as a primer for readers looking to enhance their statistical analysis skills through graph utilization.
Step-by-Step Instructions:
To effectively harness the power of graphs for statistical analysis, it is imperative to follow a systematic approach. Starting with data collection and organization, users can then proceed to choose the most appropriate graph type based on the nature of the data. Subsequent steps involve customizing the graph settings, labeling axes, and incorporating statistical markers to enhance visualization clarity. By adopting a structured methodology, individuals can streamline the graphing process and extract meaningful conclusions from their data.
Tips and Tricks:
Beyond the basic steps, there are several tips and tricks that can enhance the efficacy of graph utilization in statistical analysis. Leveraging color schemes to highlight key data points, utilizing interactive features for in-depth exploration, and incorporating annotations for clarity are just a few strategies to optimize graph interpretation. Additionally, users can experiment with different graph layouts and styles to find the most suitable format for conveying their statistical findings.
Troubleshooting:
Despite its utility, graph creation may present challenges such as data formatting errors, axis scaling issues, or misinterpretation of data trends. This section addresses common troubleshooting scenarios encountered during graph creation and interpretation, offering solutions to rectify potential discrepancies and ensure accurate representation of statistical insights. By familiarizing themselves with these troubleshooting strategies, individuals can navigate graph-related obstacles with confidence and precision.
Introduction
In the realm of statistical analysis, the utilization of graphs stands as a pivotal component, offering a visual gateway to complex data landscapes. Graphical representations not only enhance the accessibility of information but also streamline the interpretation and communication processes. This article embarks on a journey through the intricate network of graphs, shedding light on their significance in decoding statistical insights and fostering informed decision-making.
Significance of Statistical Analysis
Understanding the role of statistics in data analysis
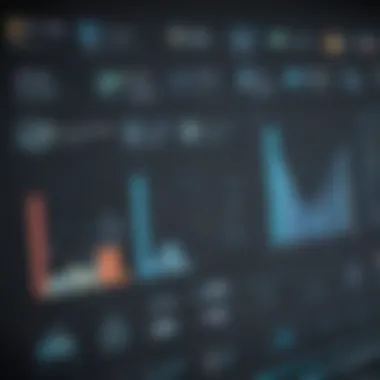
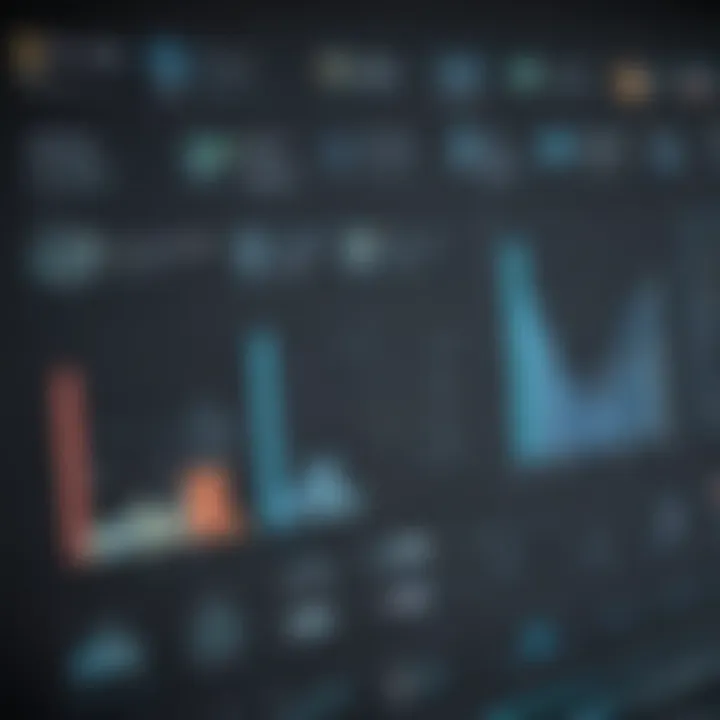
Statistical analysis serves as the backbone of data interpretation, providing a systematic approach to extracting meaningful insights from raw information. The essence of understanding the role of statistics lies in its ability to unravel patterns, distributions, and relationships within datasets. By employing statistical methodologies, analysts can uncover hidden trends and derive conclusive results from empirical observations. The detailed examination of statistical principles not only enriches analytical procedures but also fortifies the reliability and accuracy of data-driven conclusions.
Impact of statistical insights on decision-making processes
The impact of statistical insights reverberates across decision-making realms, guiding stakeholders towards informed choices and strategic directions. Statistical interpretations furnish decision-makers with evidence-based rationales, instilling confidence in the selected course of action. By harnessing statistical insights, businesses can adapt swiftly to market trends, optimize resource allocations, and mitigate risks effectively. The symbiotic relationship between statistical analysis and decision-making underscores the transformative potential of data-driven strategies in modern organizational landscapes.
Role of Graphs in Statistical Analysis
Enhancing data visualization through graphical representations
Graphs play a pivotal role in enriching data visualization, translating complex numerical data into intuitive visual constructs. The visual appeal of graphs not only captivates audiences but also simplifies data comprehension through graphical storytelling. By encapsulating statistical information in visual formats, graphs create a bridge between analytical depth and visual accessibility. The interactive nature of graphical representations fosters engagement and enhances the retention of statistical insights, making graphs indispensable tools in the analytical arsenal.
Facilitating the identification of patterns and trends
Graphs serve as dynamic canvases for uncovering patterns and trends ingrained within datasets, offering a holistic perspective on evolving data dynamics. The innate ability of graphs to highlight trends, cyclical variations, and outlier anomalies enables analysts to unveil crucial patterns that might escape tabular scrutiny. By facilitating pattern recognition, graphs empower analysts to make data-driven inferences, streamline decision-making processes, and forecast future trends with enhanced precision.
Purpose of the Article
Outlining the objective of exploring graph implementation in statistical analysis
The core objective of this article revolves around illuminating the transformative power of incorporating graphs into statistical analyses. By outlining the seamless integration of graphs in statistical frameworks, this article aims to underscore the multilayered benefits of leveraging visual representations for data interpretation and analysis. The strategic deployment of graphs in statistical analyses not only enhances analytical efficiency but also cultivates a deeper understanding of complex datasets. Through this exploration, readers will embark on a journey towards harnessing graphs as potent instruments for unlocking data-driven insights and enriching statistical undertakings.
Best Practices for Graph Creation
Simplicity and Clarity
Avoiding Visual Clutter for Better Comprehension
Visual clutter can impede the understanding of data insights, making it crucial to prioritize simplicity and clarity in graph creation. By streamlining visual elements and eliminating unnecessary distractions, graphs can convey information more effectively, facilitating insightful analysis and decision-making processes. The emphasis on decluttering visuals ensures that key data points stand out prominently, aiding in the comprehension of complex datasets.
Emphasizing Key Data Points for Clarity
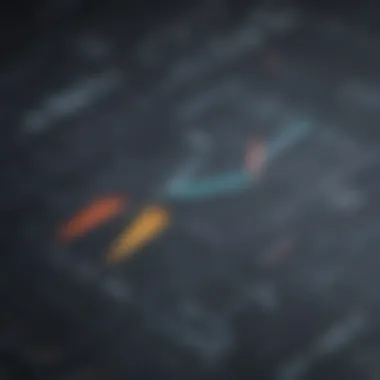
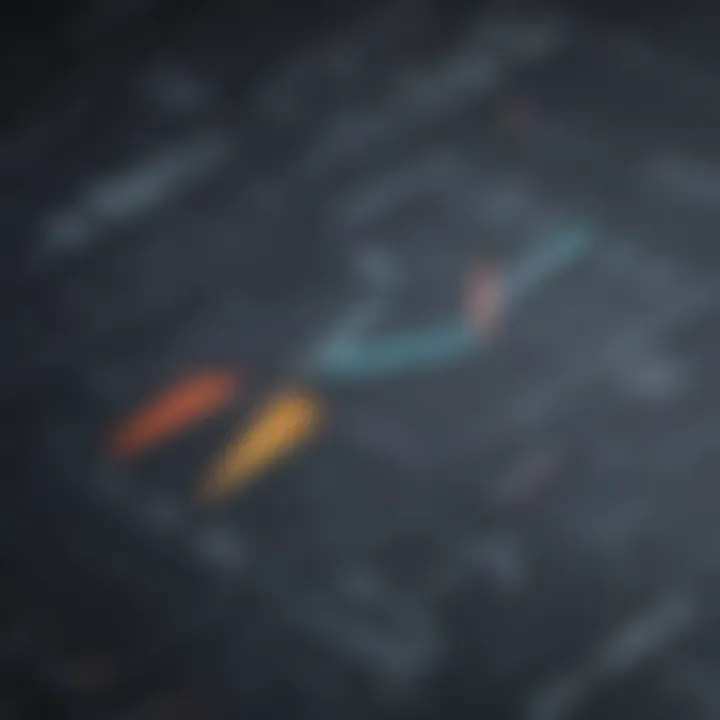
Highlighting key data points within graphs enhances clarity and interpretation, enabling viewers to grasp crucial information at a glance. By strategically accentuating important metrics or trends, graph creators provide audiences with a clear focus, directing attention to significant findings and patterns. This practice not only improves data readability but also fosters a deeper understanding of the insights presented, promoting informed decision-making and analysis.
Consistency in Design
Maintaining Uniform Color Schemes and Labeling Conventions
Consistency in color schemes and labeling conventions contributes to a harmonious visual experience across graphs, ensuring a cohesive presentation of data. By adhering to uniform design elements, such as color palettes and labeling styles, creators establish a sense of continuity and structure in their visualizations. This uniformity facilitates comparison between multiple graphs, fostering readability and coherence in analytical narratives.
Ensuring Coherence Across Multiple Graphs
Creating coherence across multiple graphs is essential for maintaining a seamless storytelling experience in statistical analysis. By aligning design elements, scales, and formatting choices across various visuals, creators establish a cohesive narrative flow that enhances data comprehension. Consistent graph design not only improves visual appeal but also aids in the seamless interpretation of complex statistical information.
Accuracy in Representation
Ensuring Proportional and Scaled Graph Elements
Accuracy in representing graph elements at proportional scales is fundamental for conveying data truthfully and informatively. By ensuring that graphical components such as bars or lines accurately reflect quantitative values, creators uphold the integrity of statistical insights. Proportional and scaled graph elements enable viewers to make precise comparisons and draw accurate conclusions, bolstering the credibility and reliability of data visualizations.
Verifying Data Accuracy and Integrity
Verifying data accuracy and integrity is a critical step in validating the reliability of statistical findings presented in graphs. By cross-referencing source data, conducting rigorous quality checks, and ensuring consistency between datasets and visual representations, creators confirm the authenticity of their insights. This practice not only safeguards against misinterpretations but also fosters trust in the statistical analysis process, elevating the impact and relevance of graph-driven conclusions.
Interpreting Graphs for Statistical Insights
In the realm of statistical analysis, interpreting graphs holds paramount significance. Graph interpretation serves as a visual gateway to understanding complex data patterns and relationships. By meticulously decoding graphs, analysts can extract valuable insights crucial for informed decision-making processes. This section delves deep into unraveling the cryptic language of graphs, emphasizing their role as indispensable tools in extracting meaningful statistical insights.
Identifying Trends and Patterns (250- words)
Recognizing upward or downward trends in data
The art of discerning upward or downward trends in data signifies a key aspect of statistical analysis. By identifying these trends, analysts can unveil crucial information about the underlying dynamics of a dataset, thereby enabling proactive measures or strategic interventions. Monitoring shifts in data trends allows for the anticipation of potential outcomes, aiding in forecasting and risk management strategies. Recognizing these trends empowers analysts to make data-driven decisions based on the observed patterns, enhancing the precision and accuracy of their analytical processes.
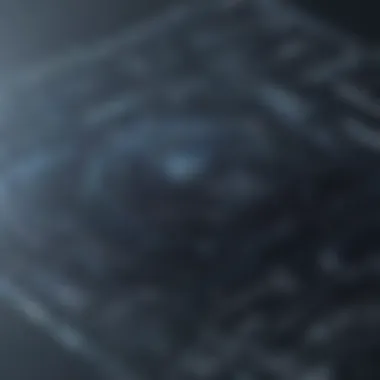
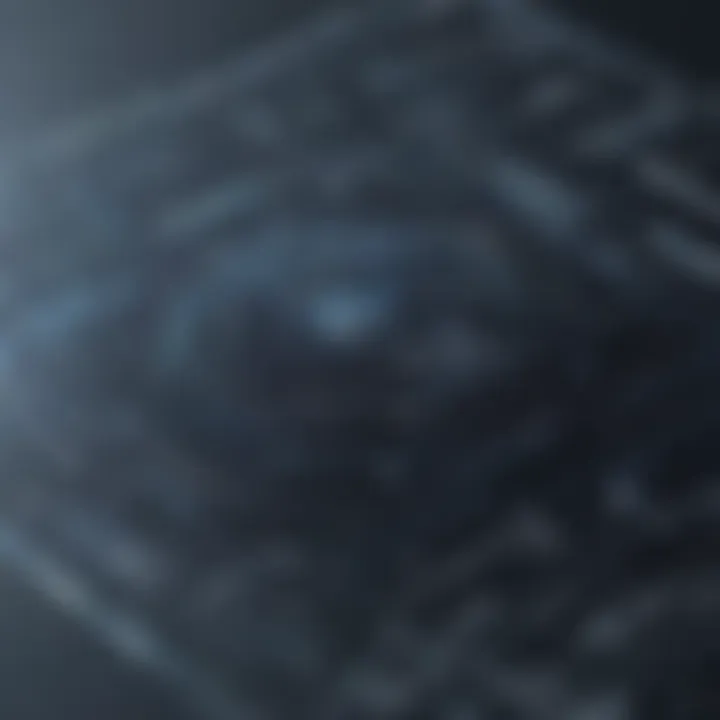
Detecting cyclical patterns through graph analysis
The ability to detect cyclical patterns through graph analysis adds another layer of sophistication to statistical interpretations. Cyclical patterns often reveal recurring trends or behaviors within a dataset, shedding light on seasonal variations or repetitive cycles that influence the data points. By deciphering these cyclical patterns, analysts can anticipate fluctuations and adapt their strategies accordingly to harness the predictive power embedded within the data. Understanding and exploiting cyclical patterns enable analysts to grasp the holistic picture portrayed by the data, enabling nuanced insights into the cyclical nature of the underlying phenomena.
Correlation Analysis (250- words)
Evaluating relationships between variables using scatter plots
Evaluating relationships between variables through scatter plots provides a visual illustration of the dependencies and connections present within a dataset. Scatter plots offer a tangible representation of the correlation between different variables, enabling analysts to gauge the strength and direction of these relationships. By examining the clustering or dispersion of data points on a scatter plot, analysts can infer the degree of association between variables, paving the way for deeper correlation analysis and predictive modeling. Leveraging scatter plots for correlation analysis enhances the precision of statistical interpretations, promoting data-informed decision-making processes.
Understanding the strength and direction of correlations
Understanding the strength and direction of correlations between variables is integral to unveiling the underlying dynamics driving a dataset. Quantifying the extent and nature of correlations provides insights into how changes in one variable affect another, offering valuable information for predictive analytics and trend forecasting. By unraveling the interplay between variables, analysts can discern causal relationships and identify potential drivers influencing the outcomes observed in the data. Grasping the nuances of correlations equips analysts with the arsenal needed to extract actionable insights from complex datasets, enhancing the efficacy of statistical analysis methodologies.
Outlier Detection (250- words)
Spotting anomalies and data discrepancies through graphs
Spotting anomalies and data discrepancies through graph analysis catapults outlier detection to the forefront of statistical analysis. By scrutinizing graphs for irregular patterns or outlier data points, analysts can identify data anomalies that deviate significantly from the norm. Detecting these outliers is essential for maintaining data integrity and ensuring the robustness of statistical interpretations. Addressing anomalies promptly allows analysts to mitigate their impact on the overall analysis, fostering a more accurate representation of the underlying dataset.
Addressing outlier impact on statistical interpretations
Addressing the impact of outliers on statistical interpretations involves delving into the implications of these anomalous data points on the analytical outcomes. Outliers have the potential to skew results and mislead interpretations if left unaddressed, underscoring the importance of diligent outlier management. By acknowledging and addressing the influence of outliers, analysts can refine their analytical models, improving the accuracy and reliability of their statistical inferences. Managing outlier impact through data validation and cleansing processes fortifies the statistical analysis against misleading conclusions, maintaining the integrity and credibility of the analytical outcomes.
Conclusion
Graphs play a pivotal role in enhancing statistical analysis by providing a visual representation of data, facilitating easier interpretation and communication of insights. In this article, we have discussed the various types of graphs used in statistical analysis, the best practices for creating them, and how to interpret them effectively. By harnessing the power of graphs, individuals can gain a deeper understanding of trends, correlations, and outliers within their data, leading to more informed decision-making processes. The inclusion of graphs in statistical analysis not only elevates the quality of insights but also enables clearer communication of complex data structures.
Harnessing Graphs for Statistical Success
Summarizing the role of graphs in enhancing statistical analysis
Graphs serve as indispensable tools in statistical analysis due to their ability to condense complex data sets into easily digestible visual representations. Their succinct nature allows for quick analysis of trends and patterns, thus significantly enhancing the efficiency of data interpretation. By summarizing pivotal data points within graphs, individuals can grasp key insights at a glance, streamlining the decision-making process and fostering a deeper understanding of the data landscape. Despite their efficiency, it's essential to recognize that graphs may oversimplify data at times, potentially leading to misinterpretations if not approached with caution.
Educating readers on leveraging graphs for data-driven insights
Educating readers on the utilization of graphs for deriving data-driven insights empowers individuals to make informed decisions based on quantitative evidence. By emphasizing the importance of graph interpretation in drawing meaningful conclusions, readers can enhance their analytical skills and cultivate a data-centric mindset. The ability to leverage graphs for data-driven insights not only enhances statistical literacy but also encourages critical thinking and a deeper exploration of data relationships. However, it's crucial to remember that graphs are tools that require a nuanced understanding to extract accurate and meaningful insights, underscoring the importance of interpretive skills in statistical analysis.