Exploring the Depths of Big Data: Trends and Insights
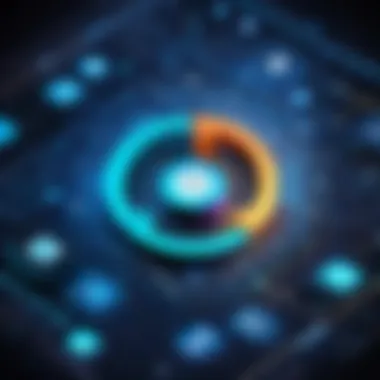

Intro
Big data has become a buzzword in today’s fast-paced digital world. It’s not just a passing fad but a transformative force reshaping industries and organizations. Defined simply, big data refers to the vast and complex datasets that are generated every minute, from social media interactions to sensor data from devices. The evolution of this term has been significant, moving from mere numbers to intricate layers of information that can be analyzed for decision-making, forecasting, and even predictive analytics.
In recent years, the importance of big data has skyrocketed. Companies across sectors are mining this information for insights, leading to improved services and strategies. For instance, healthcare organizations utilize big data to analyze patient records, enhance treatment plans, and predict outbreaks. Retailers use it to understand consumer behavior, stocking products that better meet demand. Yet, with its potential comes considerable challenges, such as security, privacy concerns, and the need for sophisticated analytical tools.
Let’s dig deeper into the trends emerging in this dynamic field.
Tech Trend Analysis
Overview of the current trend
Right now, various trends are making waves in the big data space. Technologies like artificial intelligence and machine learning are increasingly intertwined with big data analytics. They enable organizations to sift through enormous data sets and derive patterns that humans might miss. Moreover, cloud computing has transformed how data storage, access, and processing are handled, providing scalable solutions that were previously unattainable for many small entities.
Implications for consumers
Consumers are witnessing the effects of these trends firsthand. With personalized recommendations on platforms like Netflix and targeted advertising on Facebook, big data is shaping our daily experiences. While this convenience can be appealing, it also raises questions about data privacy. Users are often unaware of how their information is being collected and used, leading to a growing demand for transparency and ethical data practices.
Future predictions and possibilities
Looking ahead, one can imagine a landscape where predictive analytics becomes the norm rather than the exception. Companies may utilize big data to foresee market shifts and consumer trends more effectively. This synergy might also spur innovations in areas like autonomous vehicles and smart cities, as data from countless devices will inform decision-making on an unprecedented scale.
"The future of big data is not just about collecting data, but understanding it in meaningful ways."
As big data continues to evolve, the challenge will not solely lie in managing larger volumes of information, but in harnessing analytical prowess to turn raw data into actionable insights. This might also lead to the development of stricter regulations for data handling, ensuring that consumer privacy is held in high regard.
Industry Updates
Recent developments in the tech industry
Significant strides have been made in the tech industry related to big data, especially with advancements in data warehousing and real-time analytics. The introduction of platforms like Snowflake and Databricks has simplified the data handling processes, allowing for smoother operations and increased collaboration within teams.
Analysis of market trends
The market for big data is projected to expand rapidly, driven by the demand for data-driven strategies in businesses. The increasing recognition of data as a critical asset is prompting more organizations to invest heavily in analytics tools, big data technologies, and skilled professionals, creating a rich ecosystem centered around data utilization.
Impact on businesses and consumers
As businesses adapt to these changes, consumers can expect to experience enhanced services tailored to their preferences and needs. However, this comes with the responsibility of ensuring that all data practices remain ethical and transparent. The challenge will be to strike a balance between innovation and consumer rights, keeping trust intact in an age defined by data.
Closure
With big data shaping the future across multiple sectors, the need for understanding its complexities has never been more crucial. By exploring current trends and their implications, businesses and consumers alike can better navigate the evolving landscape of big data.
Understanding Big Data
In today's increasingly complex digital world, grappling with the concept of big data is more than just a challenge; it’s a necessity. Understanding big data prepares individuals and organizations to leverage a wealth of information that can facilitate smarter decision-making and foster innovation in a highly competitive landscape. Given the exponential growth of data generated daily, its potential applications stretch far and wide, impacting sectors such as healthcare, finance, and retail.
Big data isn't just about having massive volumes of information; it encompasses the very characteristics that differentiate it from traditional data systems. By delving into aspects like data volume, velocity, variety, and veracity, stakeholders can derive not only insights but also strategic advantages. Knowledge of big data also extends to interpreting trends and understanding its historical context, enabling a robust approach to future challenges and opportunities.
Definition and Scope
Big data, simply put, refers to datasets that are so vast, complex, and intricate that conventional data-processing applications cannot manage them effectively. It involves the collection, storage, analysis, and actionable insights drawn from data generated at high speeds from various sources.
The scope of big data goes beyond analytic functions. It encompasses:
- Data generation: Real-time inputs from sources such as IoT devices, social media, and online transactions contribute to an ever-expanding data pool.
- Data collaboration: The ability to amalgamate disparate datasets from various domains creates a richer context for analysis.
- Data insight: Employing sophisticated algorithms allows businesses to extract valuable patterns that inform products, services, and customer engagement.
Understanding the definition and scope of big data is paramount for emerging tech enthusiasts and industry professionals who aim to harness its transformative potential.
Historical Context
To truly appreciate where big data stands today, it is crucial to rewind the clock a bit. The history of big data finds its roots in the growing tide of information in the late 20th century, primarily attributed to the rise of the internet. As more devices got connected and online activities surged, organizations began recognizing the need to capture and analyze user-generated content.
In the early 2000s, companies like Google and Amazon started prioritizing vast amounts of consumer data, which led to the development of scalable data solutions like Hadoop—an open-source framework based on distributed storage and processing. This shift marked a significant turning point, pushing industries to adapt or risk obsolescence.
From the launch of Google's Bigtable to the evolution of NoSQL databases that catered to the varied structure of big data, the framework for its management has continuously developed. Companies have had to embrace technology and innovation to keep pace, shaping an ecosystem around big data tools and analytics that drive decision-making today.
Understanding this historical backdrop is crucial, as it highlights the evolution of big data technologies and sets the stage for future advancements. The interplay of innovation and necessity will only become more pronounced as we look ahead.
Key Characteristics of Big Data
The world is awash with data, a veritable ocean of bytes, and navigating this vast sea requires understanding its key characteristics. These five features—volume, velocity, variety, veracity, and the often-overlooked value—play a crucial role in how organizations approach big data. Ignoring these elements could mean sailing into rough waters without a compass.
Volume
The first of these characteristics is volume, which refers to the sheer amount of data generated and collected. In today's digital landscape, it's not uncommon for organizations to handle terabytes, or even petabytes, of information daily. This reflects a fundamental shift from previous decades when data remained relatively manageable.
Businesses can gain insights like never before due to this staggering volume. By analyzing large datasets, organizations can identify trends, make predictions, and drive strategic decisions. Yet, the challenge lies in processing this immense data load efficiently. Storing data is one hurdle, but extracting actionable information is where the magic happens.
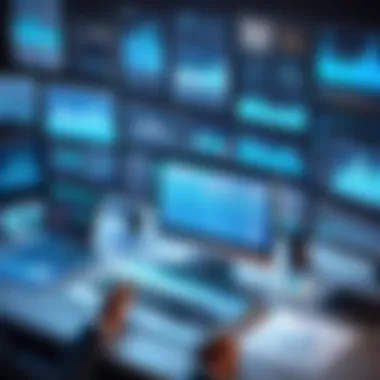
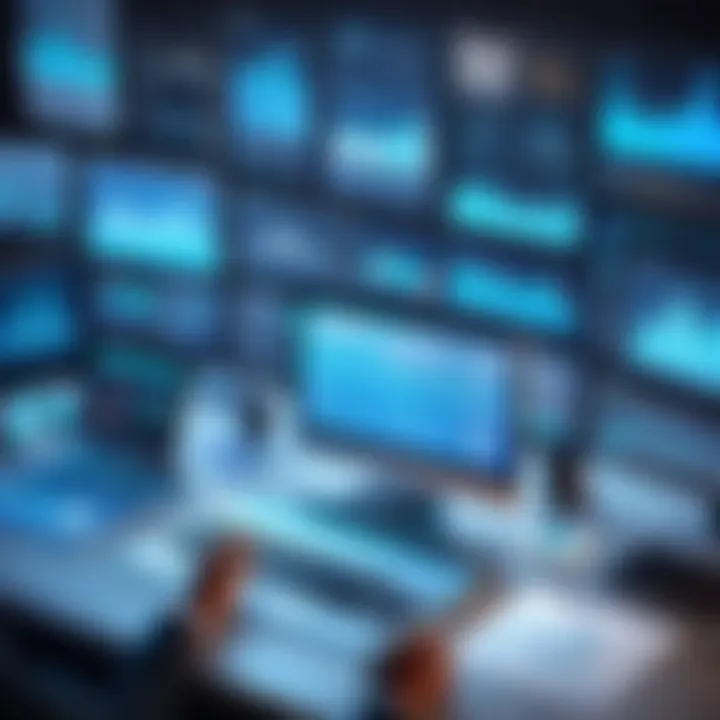
"Volume is not just about having data; it's about using that data to drive decisions in real-time."
Velocity
Next comes velocity, which pertains to the speed at which data is generated and processed. Data flows into organizations at a breakneck pace—from social media interactions, online transactions, to IoT devices constantly sending updates.
Real-time analytics have become crucial. Companies in industries like finance and e-commerce need to respond instantly to data streams. If a retailer can analyze customer purchase patterns as they happen, it can tailor recommendations and promotions in the blink of an eye.
This need for speed opens up new opportunities but also presents logistical challenges. Organizations must deploy robust data processing frameworks like Apache Kafka to keep up with the relentless pace.
Variety
The third characteristic, variety, highlights the diverse forms data can take. This encompasses structured, semi-structured, and unstructured data. Consider the disparities between traditional databases filled with numerical data and the treasure trove of unstructured data found in emails, images, and social media.
Embracing variety allows organizations to create a more holistic understanding of the data at hand. For instance, operational insights gleaned from sales numbers can be complemented by customer sentiment analysis derived from social media conversations. This multifaceted approach can lead to well-rounded strategies and more informed decisions.
Veracity
Veracity speaks to the reliability and accuracy of data. In the age of misinformation and data overload, ensuring that insights drawn from data are trustworthy is paramount.
Organizations must implement data governance frameworks to verify data integrity. Inaccurate data can lead companies astray, potentially resulting in misguided strategies based on faulty assumptions. By maintaining high standards for data quality, organizations can foster confidence in their analytics outcomes.
To illustrate, consider an organization using predictive analytics to forecast inventory needs. If the data feeding the algorithm is flawed, it could lead to overstock or stockouts, both of which are detrimental to business.
Finale
Each of these characteristics—volume, velocity, variety, and veracity—intertwine to form the backbone of what we call big data. Recognizing the significance of these traits enables organizations to strategically harness big data, transforming overwhelming information into valuable insights.
In a world where data is king, understanding these key characteristics is less of an option and more of a necessity for organizations aiming to thrive.
Technologies Behind Big Data
The significance of technologies in the big data realm cannot be overstated. They serve as the backbone, enabling organizations to collect, store, process, and analyze vast amounts of data efficiently. In the age where data flows like a raging river, effective technologies help channel it, making it comprehensible and usable for diverse applications.
Data Storage Solutions
Storing extensive amounts of data requires systems that not only manage size but also allow for rapid data retrieval and scalability. The following are pivotal storage solutions utilized across various industries:
Distributed File Systems
Distributed file systems play a crucial role in providing scalable storage that can spread data over multiple machines. One of the key characteristics is fault tolerance, which ensures that data remains available even if parts of the system fail. This benefit is particularly relevant for big data applications, where data is constantly growing.
A standout feature of distributed file systems is their ability to process large datasets in parallel, allowing businesses to harness data more effectively. However, one downside might be the initial complexity in setup and maintenance. High-level expertise is sometimes required to keep these systems running smoothly, making them a substantial, if valuable, investment in the long run.
NoSQL Databases
In a landscape where traditional databases struggle with flexibility, NoSQL databases arise as a breath of fresh air. These systems are designed for unstructured data and provide immersive capabilities for scaling out - meaning they can handle a lot of connections and queries simultaneously.
A unique advantage is their schema-less nature, which allows developers to store data with varied formats without extensive upfront design. This can be a great boon for projects that require agility and frequent changes. But the trade-off is usually a lack of some built-in functionality, such as complex queries, that traditional SQL databases naturally offer.
Data Lakes
Data lakes provide a centralized repository that allows you to store structured and unstructured data at scale. This characteristic is vital for organizations looking to manage data from various sources without the need for it to be processed first.
The unique feature of a data lake is its analytics capability, which lets organizations query raw untransformed data, paving the way for innovative analytics and machine learning applications. One disadvantage, however, is the risk of creating a 'data swamp'—a situation where poor data quality leads to unmanageable datasets that complicate analysis and recovery efforts.
Data Processing Frameworks
For processing big data, frameworks are essential. They help manage large data flows and execute sophisticated transformations that fuel insightful analytics and decisions. Here’s a look at key frameworks in this domain:
Hadoop
Hadoop stands out for its ability to distribute data across many computers, thus enabling high-speed data processing. Its primary strength lies in processing batch workloads effectively, making it ideal for tasks requiring extensive computation over large datasets.
A primary unique feature of Hadoop is its fault-tolerant architecture, which automatically replicates data to prevent losses. While this is crucial, one drawback is its reliance on batch processing, which might not be ideal for scenarios demanding real-time analytics.
Spark
Spark distinguishes itself with in-memory processing capabilities, allowing it to run operations significantly faster than systems revolving around disk-based processing. This is particularly useful for applications that rely on real-time data analysis or complex machine learning workflows.
The ability to process streaming data in addition to batch data makes Spark a flexible choice. However, managing memory can become tricky with large datasets if not monitored closely, potentially leading to performance hiccups.
Flink
Flink offers another layer of agile processing with its event-driven architecture. It’s well-suited for scenarios where analyzing streams of data in real time is paramount. One of its leading characteristics is precisely handling late data and potential outliers, which is central to maintaining accurate real-time analytics.
A unique aspect of Flink is its capability for stateful computations, allowing it to keep track of data over time and make decisions based on historical context. Yet, similar to other technologies, it might demand significant resources to run optimally, which needs consideration when planning implementations.
"In the world of big data, the right technology can be the difference between insight and infamy."
These technologies are shaping the landscape of big data management, each bringing its unique strengths and some quirks. Understanding the mechanics behind them proves essential for anyone looking to navigate the complexities of big data in today's technology-driven environment.
The Role of Analytics
Analytics is fundamental in the realm of big data, serving as the lens through which organizations can make sense of the overwhelming volume of information they generate and collect. Simply put, analytics transforms raw data into meaningful insights, enabling informed decision-making across various industries. The landscape of big data analytics can be classified into three primary categories: descriptive, predictive, and prescriptive analytics. Each plays a unique role, catering to different organizational needs and strategic goals.
Descriptive Analytics
Descriptive analytics is all about understanding the past. By utilizing various tools and techniques, organizations can sift through historical data to identify trends and patterns. It paints a clear picture of what has happened, allowing stakeholders to grasp the context of their data. This type of analysis is often accompanied by dashboards and data visualizations that make complex information digestible. For example, a retailer might analyze last year’s sales data to determine peak shopping seasons.
Here’s why descriptive analytics is essential:
- Informs Strategy: By understanding past behavior, businesses can tailor their strategies effectively.
- Operational Insights: It can highlight areas of inefficiency, prompting process improvements.
- Customer Understanding: Organizations gain deeper insights into customer preferences, aiding in segmentation and marketing strategies.
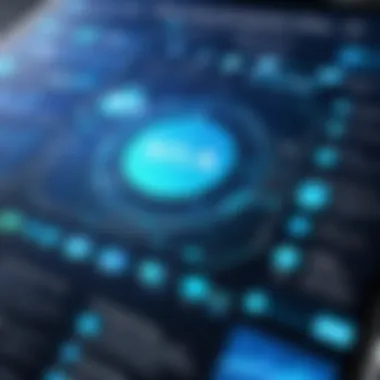
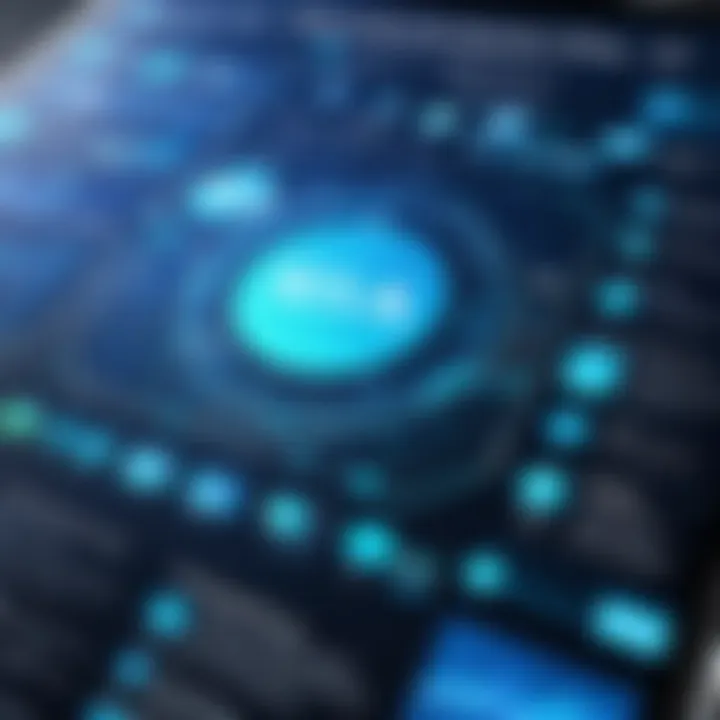
Predictive Analytics
Stepping into the future, predictive analytics takes the insights from descriptive analytics and uses them to forecast potential outcomes. It employs statistical algorithms and machine learning techniques to analyze current and historical data, making predictions about future events. It’s like a crystal ball—just more nerdy and scientific.
In practical terms, a bank might use predictive analytics to assess the creditworthiness of loan applicants by looking not just at their current financial status but also at their spending habits over time. Some key benefits include:
- Risk Management: Helps organizations identify potential risks before they occur, allowing for proactive measures.
- Revenue Forecasting: Assists in predicting future sales, making for better financial planning.
- Enhanced Personalization: Companies can tailor their marketing efforts by predicting customer behavior, leading to improved engagement and conversion rates.
Prescriptive Analytics
Prescriptive analytics is where it gets particularly interesting. This type goes beyond prediction to recommend actions based on the data analyzed. It leverages techniques from operations research and simulations to suggest the optimal course of action for any given situation.
Imagine a logistics company using prescriptive analytics to optimize delivery routes. By considering various factors—traffic patterns, delivery windows, and fuel costs—an algorithm might suggest the most efficient route to minimize costs and time. The potential advantages are numerous:
- Efficiency Improvement: Organizations can streamline operations, cutting down time and costs.
- Strategic Decision-Making: Provides actionable insights, elevating the effectiveness of leadership decisions.
- Resource Allocation: Helps in making the best use of available resources based on data-driven recommendations.
"Analytics is the golden key that unlocks the treasure chest of big data."
Challenges in Big Data Management
The .advent of big data has revolutionized how organizations process, analyze, and derive meaning from vast amounts of information. However, the journey is not without its hurdles. Managing big data can be a double-edged sword; while it's a goldmine of potential insights, it presents a plethora of challenges that can limit its effectiveness. Addressing these challenges in big data management is crucial for realizing the full potential of this technology across various fields.
Data Quality Issues
One of the foremost challenges is maintaining the quality of data. It's akin to building a house on a weak foundation; poor data quality undermines any analysis performed on it. Inaccurate, incomplete, or biased data can lead to faulty conclusions, which might affect critical decisions. Organizations must invest in data cleansing processes to ensure that the information they rely on is both accurate and reliable.
Every organization should consider implementing methods such as:
- Regular audits: Conducting periodic checks to identify and fix anomalies in data sets.
- Data validation: Establishing rules for data entry to minimize human error during collection.
- Source credibility: Prioritizing data from reputable sources can enhance reliability.
Privacy and Security Concerns
With great data comes great responsibility. The collection and management of substantial data pools raise significant privacy and security concerns. Companies often find themselves walking a tightrope, balancing between harnessing data for innovation and protecting individual privacy. Data breaches can lead to severe consequences, triggering not only financial losses but also reputational damage that could take years to mend.
To mitigate these risks, organizations are encouraged to:
- Develop a strong cybersecurity strategy: This includes firewalls, encryption, and regular security updates to protect sensitive information.
- Implement access controls: Limiting data access to authorized individuals only can help minimize the risk of internal leaks.
- Educate employees: Conducting training sessions on best practices for data handling is essential; even a single lapse can lead to vulnerabilities.
"In today’s digital world, being vigilant about data security isn't just an option, it's a necessity."
Integration and Interoperability
Another pressing challenge in big data management lies in integration and interoperability of various data sources. Companies often use disparate systems, which can make it difficult to amalgamate data from different platforms effectively. This fragmentation can lead to inefficiencies and hinder the ability to glean actionable insights from the data.
To tackle integration issues, organizations may consider:
- Adopting standardized formats: Using common data formats can simplify data exchanges between systems.
- Investing in integration tools: Middleware solutions can bridge gaps between different database systems and applications.
- Fostering collaboration across departments: Encouraging different teams to work together can facilitate a smoother data flow and break down silos.
By recognizing these challenges and actively seeking solutions, organizations can position themselves to not only manage big data more effectively but also leverage its capabilities for greater innovation and improvement.
Applications Across Industries
Big data has become a buzzword that has transcended various sectors, reshaping how businesses operate and make strategic decisions. The colossal quantities of data available today can be harnessed to uncover valuable insights, optimize operations, and improve customer experiences. It’s a pivotal element in enabling companies to stay ahead of the competition and adapt to ever-changing market dynamics. The exploration of its applications across multiple industries reveals both the possibilities and challenges that organizations encounter in their data journey.
Healthcare
In the healthcare sector, big data is nothing short of a game changer. With the vast amount of patient data generated from electronic health records (EHR), wearable devices, and clinical trials, healthcare providers can leverage this information to enhance patient care.
By analyzing data, practitioners can identify trends in patient health, predict outbreaks, and tailor treatments to individual patients based on their medical history. For example, predictive analytics allows hospitals to foresee patient admissions and manage resources effectively, ensuring that they are not caught off guard during peak times. Moreover, big data can facilitate personalized medicine—treatments specifically designed for the genetic makeup of patients have shown promising results.
"The next era of healthcare is marked by insights driven by data, which can lead to more effective care and better outcomes."
Finance
The finance industry is another area where big data plays a crucial role. With the ability to process vast datasets in real time, financial institutions can make more informed decisions while managing risks efficiently. Big data technologies allow for the analysis of customer behaviors, market trends, and economic indicators.
For example, banks utilize data analytics to detect fraudulent transactions by identifying patterns that differ from normal behavior. Risk assessment models, powered by big data, help lenders determine the creditworthiness of borrowers with impressive accuracy, leading to better loan approval processes. The impact of big data in finance is profound, enabling better customer service, precise targeting for marketing campaigns, and overall improved operational efficiency.
Retail
In the retail world, understanding customer preferences and behaviors has never been more critical. Big data equips retailers with insights that drive inventory management, pricing strategies, and marketing efforts. With data collected from customer interactions, purchase patterns, and even social media, retailers can create more tailored shopping experiences.
For instance, companies like Amazon utilize recommendation engines that analyze browsing and purchasing history to suggest products that customers are likely to buy. Additionally, big data allows retailers to enhance supply chain efficiency by predicting demand and adjusting inventory levels accordingly, resulting in reduced costs and increased customer satisfaction.
Ultimately, the seamless integration of big data across these industries amplifies operational capabilities, offering organizations a distinct edge in their respective markets. As we navigate through the complexities of this data-centric world, embracing these applications is integral to unlocking not just efficiency, but also innovation.
Case Studies of Successful Implementations
Examining case studies of successful implementations is not just an exercise in showcasing success; it is about drawing meaningful lessons from real-world applications of big data. These case studies provide keen insights into how organizations leverage big data to drive innovation, improve efficiency, and gain competitive advantage. They serve as blueprints for best practices while also shedding light on common pitfalls to avoid. Understanding these success stories helps both seasoned professionals and newcomers to navigate the rich landscape of big data.
Tech Giants
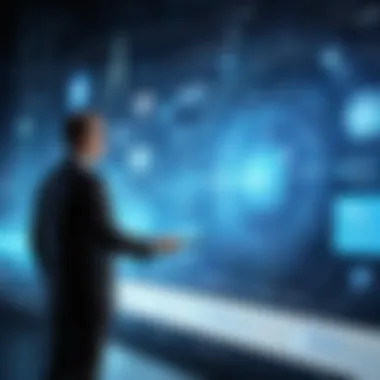
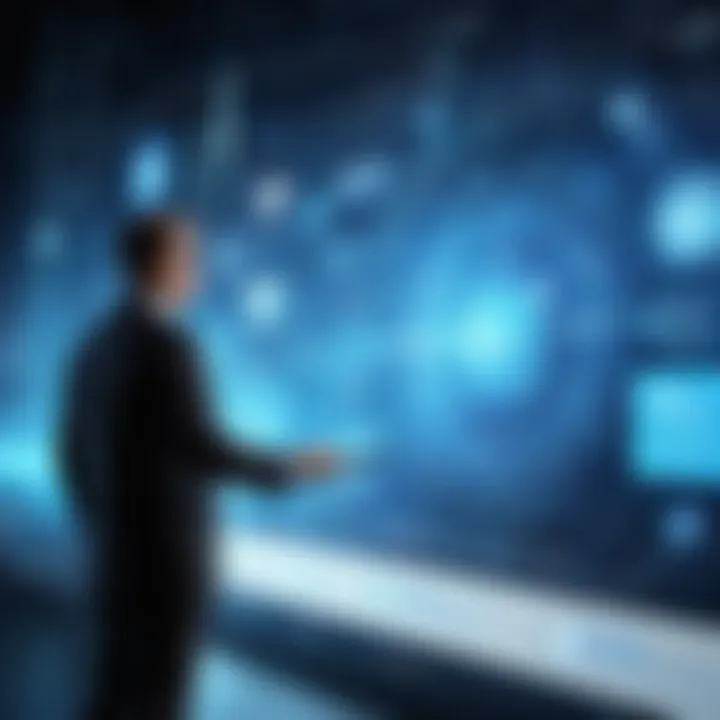
When we look at the titans of the tech world, big data is often the backbone of their operations. Companies like Google, Amazon, and Facebook have harnessed massive datasets to improve services, enhance user experiences, and generate substantial revenue. For instance, Google's search algorithms are finely tuned through the analysis of user data. By studying patterns in search queries, Google constantly refines its algorithms. This iterative process ensures that users receive the most relevant results—essentially marrying data analysis with customer satisfaction.
Moreover, Amazon has managed to leap ahead of competitors thanks to its personalized recommendation system. Through comprehensive data analysis, Amazon not only understands individual customer preferences but also predicts future purchases. This capability hinges on mining data from millions of transactions, thereby creating an ecosystem where customers are compelled to keep coming back.
While the advantages are clear, these giants also face challenges. Privacy issues often arise. Facebook, for instance, has grappled with concerns regarding user data protection. The balance they attempt to strike between personalization and user privacy is a nuanced dance, reflecting broader ethical discussions in the data landscape.
Startups and Innovators
Startups are shaking up the big data scene by adopting nimble and innovative approaches. Many emerging companies focus on niche sectors, delivering customized solutions that target unique challenges. For example, Zylo, a startup focused on SaaS management, uses big data analytics to help businesses gain visibility into their software usage. It enables firms to monitor and optimize their software expenditures, which is vital in today's economy where every penny counts.
Another innovative player is DataRobot, which automates the process of building and deploying machine learning models. By offering an accessible platform for businesses to invest in analytics without the need for rich resources or established infrastructure, DataRobot democratizes data science. Startups like these show that you don’t have to be a tech giant to harness the power of big data; smaller players can made significant strides through creativity and responsiveness to market needs.
To highlight the importance of these case studies, consider this:
"Real-world implementations not only affirm the viability of big data strategies but also inspire and guide others who aim to integrate such systems."
These examples underscore how both established and emerging entities can thrive using big data. As industries evolve, staying abreast of these successful implementations can aid organizations in molding their data strategies, fostering an atmosphere of continuous learning and adaptation.
Future Trends in Big Data
The landscape of big data is moving faster than a jackrabbit on a date. The innovations and technologies shaping the future hold great potential, but they come with their own set of challenges. Understanding these trends is not merely academic; it's practical, since they’ll likely influence decision-making and strategies in various sectors. The direction in which big data is headed can redefine operations, enhance efficiencies, and create new pathways to insights that can change the game.
Edge Computing
Edge computing is fundamentally about processing data close to where it is generated rather than relying on a centralized data center. As the IoT devices become ubiquitous, the volume of data they generate is staggering. Yet, sending all of this data to a far-off server for analysis can lead to bottlenecks. Imagine a smart car; it collects data in real-time about obstacles, traffic, and even road conditions. If that data needs to travel hundreds of miles for processing, it's not going to be much help when you’re already on the road.
By leveraging edge computing, responses can be instantaneous. This leads to better performance, lower latency, and, importantly, improved data privacy. Data processed on the edge means there's less personal information whisked away to the cloud, reducing vulnerabilities.
As businesses embrace edge computing, they're finding new ways to analyze data in real-time, adapt swiftly to changing conditions, and ultimately drive innovation while cutting costs.
AI and Machine Learning
Artificial intelligence and machine learning are not just buzzwords; they are the engines behind the next wave of big data applications. These technologies help to sift through staggering amounts of data, extracting meaningful patterns and insights that would be next to impossible for a human to identify alone.
For instance, in healthcare, machine learning algorithms can analyze patient data, identifying risks and suggesting treatments that are hyper-personalized. This isn't just pie-in-the-sky thinking; it's already happening in the real world, allowing practitioners to make more informed decisions.
Moreover, the integration of AI with big data allows businesses to forecast sales trends, optimize supply chain management, and even enhance customer service through chatbots that learn and improve over time. The implications for industry efficiency, customer satisfaction, and ultimately profitability are massive.
As AI and machine learning continue to evolve, the precise combination of big data analytics and these technologies might create transformational opportunities across various domains, positioning organizations to be more proactive rather than reactive.
Data Democratization
Data democratization aims to make data accessible to all levels of an organization, not just the data scientists or tech gurus. This approach is essential as it enables employees to leverage data insights for informed decision-making right from the get-go.
Consider a company that encourages its marketers to analyze customer data directly: they can identify trends, adjust strategies on the fly, and personalize campaigns without waiting for data teams. The faster teams can act, the quicker they can adapt to changes in customer demands or market conditions.
Moreover, when businesses democratize data, they foster a culture of data literacy, encouraging all employees to engage with data responsibly. This not only empowers staff but also enhances collaboration. When everyone has insights at their fingertips, it builds a unified direction across departments.
However, this trend does come with caution: as data access broadens, so does the potential for misuse. Ensuring the right checks and balances is vital to maximize the benefits while mitigating risks associated with unauthorized data use.
"Data-driven organizations are likely to see a competitive edge as they harness the power of big data, advancing their capabilities in ways that were once considered impossible."
Big data is poised for tremendous shifts that will significantly affect how industries operate. Organizations that adapt to these trends will better navigate the complex terrain ahead.
Ethical Considerations
The rise of big data has not only brought about exciting possibilities but also raised significant ethical concerns. In this section, we will delve into the various dimensions of these ethical considerations. It's imperative to recognize that as organizations continue to harness vast amounts of data, they must tread carefully to avoid the pitfalls that can arise from misuse or misinterpretation of this information.
Bias in Data
Data is often hailed as the new oil, but much like oil, its value comes from refining it correctly. Bias in data is a hot topic nowadays because it can lead to skewed results and unfair practices. For instance, if the data collected does not accurately represent the demographic it’s meant to reflect, then the outcomes derived from that data can be misleading.
To put it simply, imagine developing a hiring tool using historical data that favors certain groups over others. If the dataset is primarily composed of applicants from a certain background, there’s a high chance that the resulting algorithm will continue to favor that group.
This raises questions.
- Who gets to decide what data is relevant?
- Are we inadvertently perpetuating stereotypes?
Addressing bias involves a proactive approach. Organizations must actively seek out diverse data sources and continuously evaluate their data for inclusivity. It’s not just about gathering data anymore; it’s about gathering the right data.
"The key to ethical data usage is not merely compliance with regulations but a commitment to fairness and accuracy that inspires trust."
Transparency and Accountability
When we talk about transparency and accountability in the realm of big data, we step into a realm that isn’t just about adhering to legal standards, but fostering a culture of openness. In many industries, there’s a trend towards requiring companies to be transparent about how they collect, store, and utilize data. For instance, the GDPR in Europe emphasizes the importance of clear communication with users regarding data practices.
A company's accountability also extends to how it responds to data breaches or misuse. Being upfront about incidents and outlining steps to mitigate risks fosters trust among consumers.
Some essential aspects include:
- Clear data usage policies
- Regular audits and assessments
- Open channels for feedback from data subjects
Organizations must recognize that achieving transparency is not just about avoiding scandals. It’s about building relationships with stakeholders who feel valued and informed. Accountability can therefore become a competitive advantage in retaining customer loyalty.
In the big picture, ethical considerations in big data are not merely obstacles to navigate but integral aspects that shape a fair and responsible data ecosystem. As this landscape continues to evolve, companies that prioritize these ethical dimensions are likely to lead the pack, paving the way for a more balanced interaction between technology and society.
The End
In the grand tapestry of big data, the conclusion serves as both a reflective summary and a springboard for future exploration. It draws together the various threads of the discussion, highlighting the significance of understanding big data's diverse aspects and its implications in today’s world. Big Data is not just a collection of information; it is a powerful asset that shapes strategies across industries, influencing decisions that impact millions.
Summary of Key Points
- Understanding Big Data: It starts with grasping its core definition and historical context, reinforcing how it has transformed from mere analytics to a cornerstone of strategic business practices.
- Key Characteristics: The four Vs—Volume, Velocity, Variety, and Veracity—are critical elements that encapsulate the complexity and richness of data today.
- Technologies Behind Big Data: An exploration of the modern storage solutions and processing frameworks reveals the tools that enable businesses to harness their data effectively.
- Analytical Core: The role of analytics cannot be overlooked, where descriptive, predictive, and prescriptive methodologies help organizations glean insights from data.
- Challenges and Solutions: The issues surrounding data quality, privacy, and interoperability highlight that the big data journey is fraught with obstacles that need addressing to gain full value.
- Applications in Diverse Fields: From healthcare to finance, big data applications are manifold, showcasing how various sectors leverage this resource for growth and innovation.
- Future Trends: Finally, keeping an eye on trends such as edge computing and AI ensures that organizations remain ahead of the curve, adapting robustly to an evolving landscape.
"Data is often seen as the new oil; it must be refined to be truly valuable."
Final Thoughts on Big Data's Impact
As we look ahead, the impact of big data is likely to deepen, shaping not just business models but also societal norms. Monitoring the ethical implications, like data bias or security concerns, is as vital as understanding the technical frameworks. Tech enthusiasts, industry professionals, and policymakers alike must collaborate to craft a future where data serves humanity effectively.
In essence, big data is not a fleeting trend; it is the foundation of informed decision-making and innovation across myriad disciplines. Embracing its complexities while navigating the challenges can offer vast potentials for growth and intelligence. The conversations around big data will only gain momentum, making it essential for all stakeholders to engage consciously and thoughtfully.
Thus, as we close this chapter, the ongoing dialogue about big data's uses, challenges, and ethical concerns will remain pivotal in shaping how we interact with knowledge and technology in years to come.