The Evolution of Artificial Intelligence: A Comprehensive Review
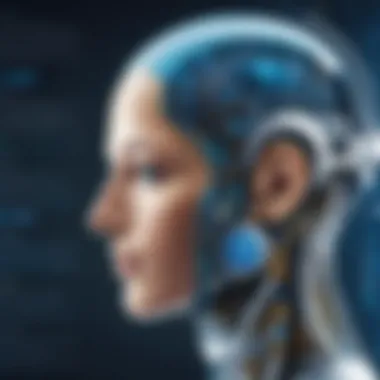
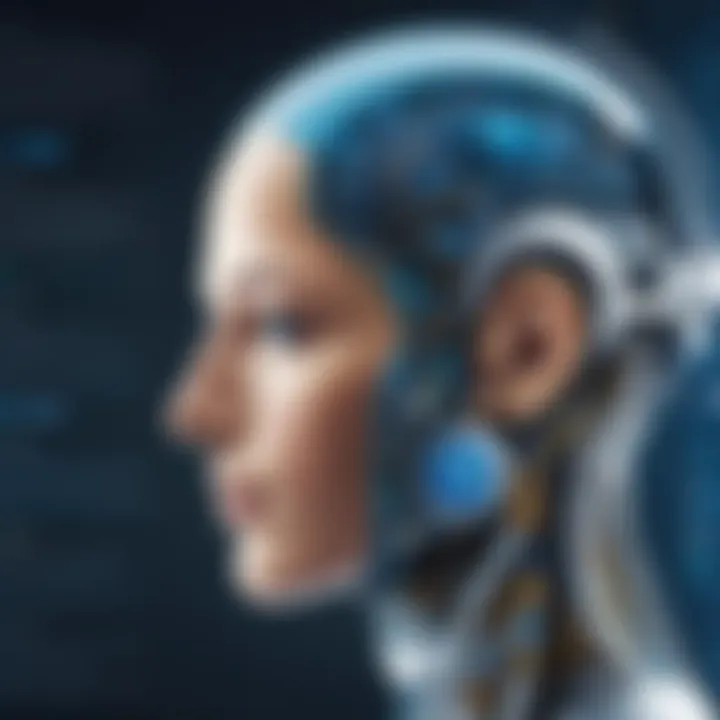
Intro
Artificial intelligence has become a buzzword, yet its journey is steeped in history, technology, and ethical considerations. From the first stirrings of machine intelligence to the sophisticated systems we see today, this evolution is both fascinating and complex. In this exploration, we will dissect the various phases AI has traversed, spotlighting crucial milestones and innovations that have molded modern AI tools. We will also probe the ethical quandaries that have emerged as technology raced ahead, asking what the implications mean for individuals and industry alike.
By delving into each era of AI's evolution, from its embryonic stages to present-day advancements, we draw a map of the technological landscape that informs not only our understanding but also our reaction to this ubiquitous tool. As we move forward, the discussion will encompass the current trends, recent breakthroughs, and predictions that paint a picture of what lies ahead in the AI realm.
Tech Trend Analysis
Overview of the current trend
AI technologies are pervasive, interwoven into various sectors such as healthcare, finance, and entertainment. The rise of machine learning and deep learning has transformed how we interact with technology, resulting in smarter algorithms that can predict trends, analyze behaviors, and even create content. Tools such as chatbots, recommendation systems, and automated processes are just the tip of the iceberg. Today, AI is not merely a concept; it’s working behind the scenes, improving efficiency and altering our daily lives.
Implications for consumers
The implications of these advancements are profound. For consumers, the benefits range from personalized shopping experiences to enhanced medical diagnostics. However, there are also challenges—data privacy remains a pressing concern, especially as companies harness vast amounts of information to deliver tailored experiences.
Future predictions and possibilities
Looking ahead, the potential applications of artificial intelligence seem boundless. As machine learning continues to evolve, we can expect AI to penetrate deeper into sectors like autonomous vehicles and smart cities. Predictions suggest that AI could revolutionize industries by fostering innovation and offering unprecedented solutions to complex problems, making it a tool for transformative change.
"The real race is not about technology itself but who controls it and how it's integrated into society."
Industry Updates
Recent developments in the tech industry
Recent months have seen significant leaps in AI capabilities, particularly with natural language processing models that enable smoother human-computer interactions. Major tech firms are investing heavily in research, aiming to enhance AI performance and expand its applications. For example, machine learning models used in voice recognition systems become more accessible and accurate, reshaping customer service.
Analysis of market trends
The market trends clearly indicate an upward trajectory for AI adoption across various fields, fueled by increased investment and the ongoing digitization of businesses. As organizations realize the potential productivity gains from AI integration, the push to adopt these technologies will likely accelerate.
Impact on businesses and consumers
For businesses, embracing AI could mean operational efficiencies and enhanced decision-making capabilities. However, it also raises the stakes for skill upgrades to keep pace with technological change. For consumers, while the benefits of AI are visible in enhanced products and services, the challenge remains to navigate the complexities of a digital world where privacy and security come into play.
Historical Context of Artificial Intelligence
Understanding the historical context of artificial intelligence is like holding a compass in the tangled woods of its evolution. This context not only sheds light on how far we've come but also influences our approach to future developments, giving insight into the successes and missteps along the way. The interplay between ancient philosophies and the advent of modern computing lays a foundational canvas where today's AI marvels paint their potential. By exploring this context, we discern patterns and principles that could guide responsible advancements in technology.
Ancient Philosophies and Early Concepts
Long before silicon chips and neural networks, thinkers were grappling with the essence of intelligence and the possibility of crafting machines that could mimic human thought. Ancient philosophies laid the groundwork with ideas that still resonate today.
Take for instance the ancient Greeks, who pondered the concept of logic and reasoning. Aristotle's syllogistic logic formed the kernel of what later became the basis for algorithms—sets of rules or processes to follow in calculations and problem-solving.
In different corners of the world, Chinese philosophers were articulating notions of reasoning and decision-making, which also contributed to early AI concepts. The connection of these ancient insights to modern computing might seem a stretch, but they highlight humanity's long-standing curiosity about replicating thought.
- Early myths and stories often featured automata, reflecting humanity's fascination with creating life-like agents. These narratives acted like breadcrumbs leading to future endeavors in artificial intelligence.
- Additionally, Al-Khwarizmi, the mathematician from the Islamic Golden Age, introduced algorithms through his work, setting a precedent for computing that would eventually mesh with AI.
The significance of these early concepts cannot be overstated; they planted the seeds of curiosity that would, centuries later, spur the digital revolution.
The Birth of Modern Computing
The mid-20th century marked a pivotal shift as theoretical musings began to crystallize into tangible technology. The birth of modern computing, spurred by conscientious innovators, laid the tracks for AI to emerge as we know it today.
Alan Turing is often hailed as the father of computer science and the concept of a machine that could simulate human intelligence. His formulation of the Turing Machine provided a blueprint for building computational models, the principles of which are still foundational in AI research today.
In the 1940s and 1950s, the development of the first electronic computers initiated a new wave of possibilities. Researchers began to explore machine learning and intelligence, leading to early experiments that were, at times, trials and errors akin to a ship navigating uncharted waters.
- Issues such as memory, processing power, and programming languages were vital in shaping early frameworks for machine learning.
- Enter the Dartmouth Conference in 1956, often referred to as the spark that ignited the AI revolution. This gathering brought together brilliant minds who shared hopes and ambitions of imbuing machines with cognitive abilities. It laid the groundwork for a field that would expand rapidly, although not without its growing pains.
As we trace this historic thread, the interplay between philosophy and computing is unmistakable. The ideation phase fueled invention, creating a tapestry of knowledge that continues to weave through the fabric of today's sophisticated AI systems.
"Computer science is no more about computers than astronomy is about telescopes." - Edsger W. Dijkstra
In summary, the historical context of AI reflects a journey enriched with intellectual curiosity, technological metamorphoses, and vital explorations of what it means to be intelligent. Understanding this trajectory is crucial not just for tech enthusiasts and professionals but for anyone intrigued by the enigma of artificial intelligence.
Key Developments in AI Research
The journey of artificial intelligence is marked by significant progress that has shaped its current landscape. Understanding key developments in AI research allows us to contextualize modern applications and recognize the complex factors driving its evolution. The importance of this topic lies not only in outlining major milestones but also in acknowledging the collaborative efforts in academia and industry that led to breakthroughs. Each development serves as a stepping stone, paving the way for innovative technologies that now permeate various sectors.
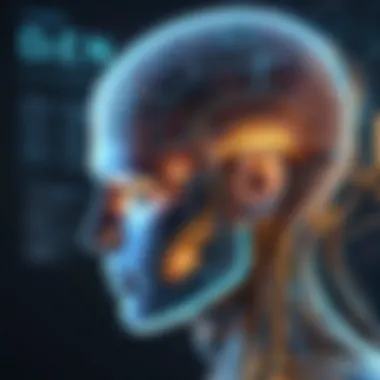
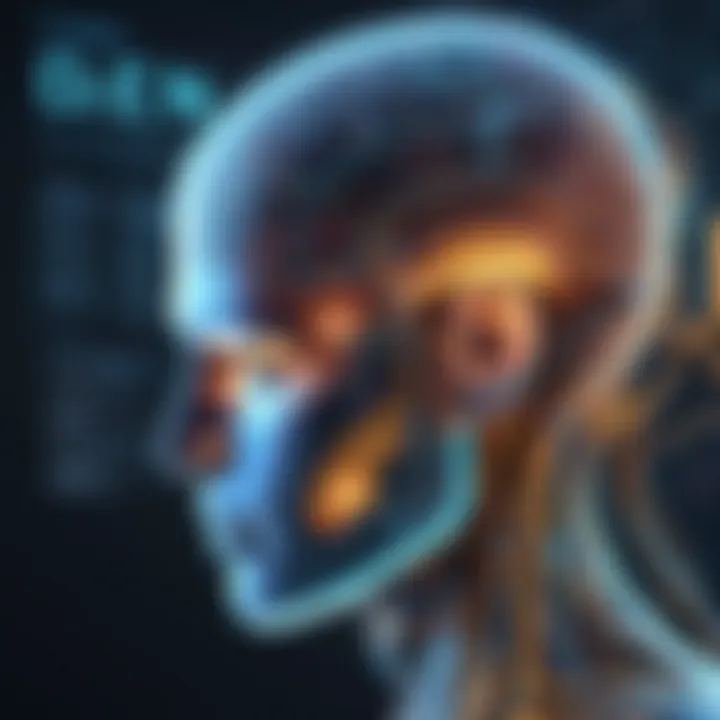
The Dartmouth Conference
The Dartmouth Conference of 1956 is often heralded as the birth of artificial intelligence as a distinct field of study. Participants included notable scholars like John McCarthy, Marvin Minsky, Nathaniel Rochester, and Claude Shannon. The aim was ambitious: to explore whether machines could be made to simulate human intelligence.
The conference was seminal for a few reasons. Firstly, it put AI research on the academic map, drawing attention to the potential of machines to "think" and prompting further investment in the field. Secondly, it catalyzed the formation of distinct branches within AI research—such as cognitive science and machine learning—that continue to evolve. In many ways, the outputs of this conference shaped the frameworks through which AI is understood today.
"The Dartmouth Conference was the first time that people sat down to deliberate on the profound implications of machines mimicking human cognition. It was a gathering that ignited a gravitational pull for future research."
The legacy of this gathering cannot be overstated. It laid the groundwork for foundational projects, funding endeavors, and research collaborations between institutions that were dedicated to cultivating a pragmatic approach to AI challenges.
The Rise of Machine Learning
As the decades rolled on, the AI landscape expanded to accommodate new methodologies, chief among them being machine learning. This transformation marked a significant shift in focus from rule-based systems to algorithms that enable systems to learn from data. In essence, rather than being programmed with explicit instructions, machines began to derive patterns and make decisions based on past experiences.
Machine learning gained traction due to several factors:
- Increased Computational Power: The evolution from vacuum tubes to transistors, and eventually microchips, made powerful computation more accessible.
- Data Proliferation: The surge of digital data available on the internet and through various sensors provided the fuel needed for sophisticated algorithms to operate effectively.
- Algorithmic Innovations: Breakthroughs like Support Vector Machines, decision trees, and, later, ensemble methods opened up new avenues for analysis.
Some notable applications of machine learning include:
- Predictive Analytics: Used across industries to forecast trends and behaviors, enhancing decision-making processes.
- Recommendation Systems: Platforms like Netflix and Amazon leverage machine learning to personalize user experiences, showcasing its commercial viability.
- Computer Vision: Detect and interpret visual information in ways that enable functionalities like facial recognition and object detection.
The rise of machine learning has not just transformed AI but has also sparked discussions around the ethical implications of technology, resulting in varied stances on its implementation across sectors.
Breakthroughs in AI Technologies
The landscape of artificial intelligence has undergone remarkable transformations over the decades. These advancements have not only pushed the boundaries of what machines can achieve but have also redefined our expectations of technology. Breakthroughs in AI technologies are vital to understanding how we have reached today's capabilities and what the future holds.
Neural Networks and Deep Learning
Neural networks are often touted as one of AI's most revolutionary breakthroughs. They mimic the structure of the human brain, comprising layers of interconnected nodes or neurons. This design allows neural networks to learn from large amounts of data, adapting and improving their predictions over time.
One critical development in this area has been the emergence of deep learning, a subset of neural networks featuring multiple layers. With these extra layers, deep learning systems can identify intricate patterns in complex datasets. For instance, consider a weather forecasting AI. A deep learning model processes massive datasets from various sensors, recognizing subtle relationships between atmospheric conditions far beyond what a traditional model could identify.
Moreover, the impact of deep learning spans various sectors. In healthcare, algorithms now assist in diagnosing diseases by analyzing medical images with remarkable accuracy. In finance, they predict stock market trends based on historical data. The key takeaway is that these technologies enable more sophisticated and precise analyses, paving the way for smarter applications.
Natural Language Processing
Natural Language Processing (NLP) represents another watershed moment in AI technologies. This domain focuses on the interaction between computers and human language, aiming to bridge the comprehension gap between machines and humans. At its core, NLP allows machines to understand, interpret, and generate human language in a useful manner.
The significance of NLP extends into everyday technology. For instance, when you use your smartphone's virtual assistant, that's NLP at work, interpreting your spoken commands and providing responses. Applications like Google Translate have expanded cross-linguistic communication, allowing diverse populations to connect.
However, NLP is not without challenges. Understanding context, sarcasm, and idiomatic expressions in language presents significant obstacles. Despite these hurdles, the transformative implications of NLP technologies are crystal clear. They enhance accessibility to information and streamline human-computer interaction, making technology more intuitive as time goes on.
Robotics and Autonomous Systems
Robotics and autonomous systems have changed the way we think about automation and labor. The technological advancements in these areas have led to machines that can perform tasks independently, from manufacturing assembly lines to household chores. The rise of sophisticated robotics designed for specific tasks is nothing short of fascinating.
Consider the world of autonomous vehicles, which have sparked considerable attention in recent years. These vehicles utilize a combination of sensors, machine learning algorithms, and mapping data to navigate effectively without human intervention. For example, companies like Tesla have made significant strides in their self-driving technology, illustrating concrete applications of AI in the real world.
On another front, robots are being deployed in healthcare settings, assisting in surgeries or helping with patient care. Their precision and ability to work tirelessly can contribute significantly to efficiency in these sectors, though ethical concerns about their reliance remain.
In essence, robotics and autonomous systems not only showcase AI's capabilities but also raise questions about the future of work and ethics in society.
"The advancements in AI technology highlight how interconnected and multifaceted the future will be, challenging our perspectives on work, communication, and even ethics as we integrate these systems into daily life."
Applications of Artificial Intelligence
The role of Artificial Intelligence has evolved significantly over the years, permeating various sectors and reshaping how we live and work. Applications of AI are not just a passing trend; they serve essential functions that address real-world challenges, enhance efficiency, and offer innovative solutions across industries. Through these applications, AI has proven its value in optimizing processes and improving outcomes in ways we once only dreamed of.
AI in Healthcare
In the healthcare sector, AI acts like a serious game changer. It has revolutionized how diseases are diagnosed, treatments are devised, and how personalized care is prioritized.
Patient Diagnostics
Focusing on patient diagnostics, AI systems streamline the identification of conditions at an early stage, increasing the likelihood of effective interventions. A key characteristic here is the ability to analyze vast amounts of medical data rapidly. For instance, algorithms trained on extensive historical patient data can recognize patterns in symptoms that might be overlooked by a human eye.
One unique feature of AI in this realm is its predictive capabilities, predicting disease risks based on genetic and environmental factors. However, while it offers accuracy and speed, there are challenges concerning the interpretation of data; algorithms need constant updates and validation to remain effective.
Drug Discovery
Turning to drug discovery, AI speeds up the painstaking process of finding viable compounds for new medications. By using machine learning to predict how different chemicals will behave in the body, researchers gain valuable insights much faster than traditional methods.
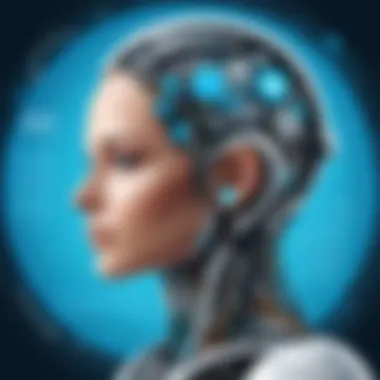
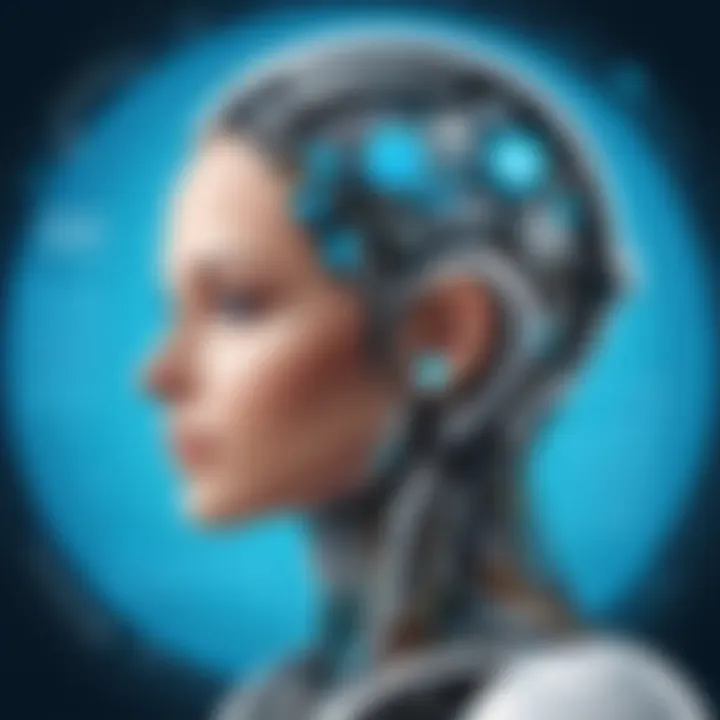
Its unique feature is the ability to prioritize potential candidates based on predicted efficacy and safety, which can drastically reduce the time and cost of bringing new drugs to market. Yet, there's a drawback; the reliance on existing data can sometimes limit the discovery of entirely new drug profiles, hence requiring a balance of computational and experimental studies.
Personalized Medicine
Personalized medicine is about tailoring treatment to individual patients based on their unique genetic makeup and lifestyle. AI contributes here through analyzing data from genetic tests, medical histories, and even lifestyle data to craft treatment plans that are uniquely suited to each patient.
The biggest advantage of personalized medicine is the potential for better patient outcomes and minimized side effects. However, issues pertaining to data privacy and the accessibility of personalized treatments raise concerns that need addressing.
AI in Finance
In the realm of finance, AI is not merely about automation; it enhances decision-making processes and helps mitigate risks associated with financial investments.
Algorithmic Trading
Algorithmic trading uses AI algorithms to execute trades at lightning speeds based on market data analysis, making split-second decisions. The approach relies heavily on historical data and real-time market analysis, delivering significant advantages in speed and efficiency.
Its key characteristic is the ability to trade on a scale and speed that human traders would find challenging. However, it’s essential to consider potential downsides like market volatility wherein algorithms may respond too aggressively to market movements, potentially exacerbating fluctuations.
Risk Management
Risk management utilizes AI to analyze and predict financial risks associated with market fluctuations and borrower defaults. AI tools can sift through data to pinpoint weaknesses and suggest mitigation strategies.
By using sophisticated modeling techniques, financial institutions can assess risks faster and with greater accuracy. But reliance on AI models carries its risks; inaccurate data or flawed algorithms can lead to misleading conclusions, making ongoing oversight essential.
Fraud Detection
Fraud detection harnesses AI to identify unusual patterns that might indicate fraudulent activity. With machine learning technologies that continuously adapt to new fraud strategies, businesses can detect threats almost in real-time.
Its strength lies in its ability to analyze massive datasets and flag anomalies that a traditional system might miss. There are, however, ongoing concerns regarding privacy and the potential for false positives, necessitating a finely tuned balance between vigilance and customer trust.
AI in Transportation
In transportation, AI offers considerable advancements, optimizing everything from vehicle performance to predictive maintenance and traffic management.
Autonomous Vehicles
Autonomous vehicles represent one of the most ambitious applications of AI. Here, AI systems integrate real-time data from multiple sensors to navigate, recognize obstacles, and make driving decisions. The key characteristic of autonomous vehicles is their ability to operate without human intervention.
While they present the promise of reducing accidents and improving traffic flow, the technology is not without its challenges. Legal frameworks and safety assurances must evolve alongside technology to ensure public trust and systemic safety.
Traffic Management
AI-driven traffic management systems leverage real-time data to minimize congestion, making city navigation smoother. By analyzing traffic patterns, these systems can optimize signal timings and provide route recommendations to avoid bottlenecks.
The advantage here is a substantial reduction in travel time and emissions, yet it raises questions about data privacy and the reliance on technology to manage public infrastructure effectively.
Logistics Optimization
Logistics optimization employs AI to enhance supply chain processes, ensuring goods reach their destinations with minimal delay. This application highlights the ability of AI to forecast demand patterns and adjust logistics accordingly.
A standout feature is the integration of AI with IoT devices to provide real-time tracking of shipments. But it’s not all rosy; unforeseen events like natural disasters can complicate logistics, and businesses need a robust strategy to adapt to such challenges.
Overall, these applications showcase the monumental impact of AI on different fields, leading to enhanced efficiency and effectiveness. As AI technology continues to advance, the potential for further applications is limitless.
Ethical Considerations in AI Development
As artificial intelligence continues to advance and integrate into various aspects of daily life, the importance of ethical considerations around its development becomes increasingly paramount. This section explores key elements that shape the ethical landscape of AI, shedding light on crucial topics like bias, privacy, and the legal frameworks needed to manage this powerful technology. These ethical deliberations not only influence public perception but also dictate the standards and regulations that govern AI, ensuring its benefits are shared fairly.
Bias and Fairness
One pressing concern in AI is the presence of bias, which can creep into systems through data and algorithms. Bias can be unintentional or a reflection of historical prejudices. For instance, if an AI used in hiring is trained primarily on data from a specific demographic, it may inadvertently favor candidates from that group, excluding talented individuals from varied backgrounds. Such occurrences raise the question of fairness in AI applications: Are algorithms perpetuating societal biases?
To add to this dilemma, fairness in AI needs to be defined clearly. It’s often subjective, and what’s considered fair can vary across cultures and contexts. A study conducted by a group at Stanford University revealed significant racial bias in facial recognition algorithms, underscoring the need for robust audits of such technologies. In response, researchers are seeking to develop techniques that minimize bias, like diverse training datasets and fairness-aware algorithms.
Privacy Concerns
Privacy is another incredibly salient issue in the realm of AI. With data being the lifeblood of AI systems, the question arises: how is this data being collected and whether individuals' rights are being safeguarded?
Many AI systems—like those used in smart home devices or personal assistants—rely on the constant collection of user data to function effectively. This raises concerns about data misuse and user consent. For example, a significant breach in 2020 involving a popular fitness tracker exposed millions of users' sensitive information.
Public trust hinges on transparent practices regarding data collection and usage. Striking the balance between harnessing data for AI's potential while ensuring privacy is not breached is a tricky endeavor. New regulatory frameworks are being suggested to safeguard user information, but their implementation is fraught with challenges.
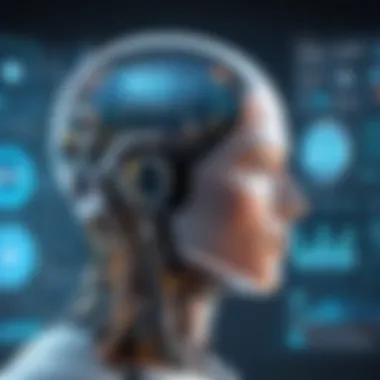

Regulatory Challenges
The rapid evolution of AI technologies poses a significant challenge to regulatory bodies, which often lag behind the pace of innovation. Creating appropriate regulations can feel like throwing darts in the dark.
One challenge is the need for global cooperation; AI technology does not acknowledge borders. Issues such as data privacy and usage often require international guidelines, which can be hard to establish and enforce. For example, the General Data Protection Regulation (GDPR) in Europe sets strict rules on data usage, but its effects may be felt differently in countries without similar regulations.
Furthermore, there’s the challenge of coming up with regulations that do not stifle innovation. Striking the right balance might seem like walking on a tightrope. What regulations are necessary without hindering the further advancement of AI?
Regulatory bodies are left grappling with these questions, developing frameworks that take into account the intricate relationship between ethical AI development and advancing technology. This ongoing dialogue will shape the future of AI and its role in society, underscoring the need for collaboration among technologists, ethicists, and lawmakers to foster a responsible AI landscape.
The Future of Artificial Intelligence
Understanding the future of artificial intelligence is like peering into a crystal ball of endless potential. It holds pivotal importance in our discourse. AI is not just a trend; it’s slowly but surely weaving itself into the very fabric of our daily lives. With each leap forward, it reshapes industries, redefines job roles, and alters how we interact with technology. The stakes are high, not just for individuals but also for corporations and society at large.
In this section, we delve into different components that signify what is brewing in the field of AI. The interplay of emerging trends and the looming challenges will not only influence technical advancements but also the ethical dimensions of this remarkable evolution.
Emerging Trends in AI
Explainable AI
In a world increasingly influenced by algorithms, explainable AI stands out. It emphasizes the necessity for transparency. What sets it apart is its focus on understanding how decisions are made by machines. This characteristic is increasingly essential as more sectors integrate AI. When users can grasp why a system arrived at a certain conclusion, it builds trust.
However, while explainable AI offers clarity, it can also present challenges. Creating models that are both complex enough to perform well and simple enough to understand can be a tightrope walk. Balancing performance with interpretability is an ongoing challenge.
AI in Creative Fields
AI's entry into creative domains is reshaping what we consider art. Think about AI-generated paintings or music compositions. The remarkable ability of algorithms to synthesize styles breaks traditional barriers. Yet, there’s a nuanced discussion about originality. Many favor AI for its potential to inspire, but others worry about the dilution of human creativity.
The unique feature here is AI’s ability to analyze vast datasets to produce new forms of creativity. On one hand, it can enhance efficiency, allowing artists to explore more. On the other, it raises questions about the authenticity of AI-created work.
AI and Climate Change
AI technology is emerging as a hopeful ally in the battle against climate change. It’s harnessing data for smarter decisions about resource utilization and emissions management. The characteristic that makes this exciting is the real-time data analysis that AI can provide, which allows for quick responses to environmental challenges.
This effort isn’t without complications. For instance, the energy consumption by AI systems themselves poses a contradiction. While AI can optimize resources, it also requires significant computational power. Understanding this irony is crucial as we navigate forward.
Challenges Ahead
Job Displacement
Job displacement is becoming an unavoidable consequence of AI ingenuity. Automation is replacing repetitive tasks, leading to a workforce that is scrambling to adapt. The key characteristic of this trend lies in the sheer scale of job roles being affected. While some positions might disappear, new ones will undoubtedly arise—but only if workers are equipped with the necessary skills.
The unique aspect here is the speed of change. Many workers find themselves in a tough spot: adapt quickly or risk being left behind. This leads to serious questions around retraining and educational systems.
Security Threats
With the advancement of AI comes heightened security risks. AI technologies are not just tools; they can also be weapons. The potential misuse of AI for malicious purposes—notably cyber attacks—becomes a pressing concern. This characteristic of AI creates a double-edged sword, where it can enhance security measures yet simultaneously threaten them.
Recognizing this threat is vital for developing countermeasures. Organizations must be proactive, investing in stronger security infrastructures that incorporate AI solutions to protect against potential abuses.
Global Competition
The race to dominate AI technology underscores the growing global competition. Major players, such as the United States and China, are jostling for position, each keen on harnessing AI for myriad applications. This situation’s key characteristic is the drive for innovations that can propel economies forward.
However, with competition comes the risk of unethical practices. Countries may resort to lax regulations to hasten progress, potentially endangering ethical standards. Keeping a keen eye on this trend is tantamount to ensuring that the evolution of AI benefits all sectors evenly.
Culmination
Summarizing AI's Trajectory
To put it simply, AI's progression has been nothing short of a rollercoaster. From the theorists of the mid-20th century, who proposed ideas that often seemed far-fetched, to today's algorithms that drive complex decision-making processes, each phase in AI's evolution has been marked by significant milestones.
"The development of AI parallels not just technological evolutions, but also societal shifts."
Some key phases include:
- The rise of computational theory in the 1950s, where the groundwork was laid by pioneers like Alan Turing and John von Neumann.
- Algorithmic advancements in the 1980s, through which neural networks gained popularity, leading to early prototypes of what we now refer to as machine learning.
- Integrating AI into everyday applications, such as virtual assistants and recommendation systems, really gained momentum in the 2000s.
Overall, this trajectory highlights a consistent pattern of increasing sophistication and application scope. As researchers demystified complex problems, industries began adopting AI to refine efficiencies and open doors to innovative prospects.
Final Thoughts on Future Directions
Looking ahead, the future of artificial intelligence holds a myriad of paths ripe for exploration and development. While the promise of explainable AI sets the stage for clearer, more transparent decision-making processes, the creative realms beckon technologists to push boundaries further.
The ongoing challenges must also be acknowledged, especially in areas like:
- Job displacement resulting from automation.
- Security threats that arise as AI systems grow more sophisticated.
- Global competition among nations aiming to lead in technology.
For professionals and enthusiasts alike, staying on top of these trends isn't just about participating in tech evolution; it's about preparing for a society where AI intertwines further with human experiences. As tech aficionados, understanding these complexities, along with being aware of ethical implications, is crucial for shaping a sustainable and beneficial AI-integrated future.