Enterprise Data Risk Management Strategies in a Digital World
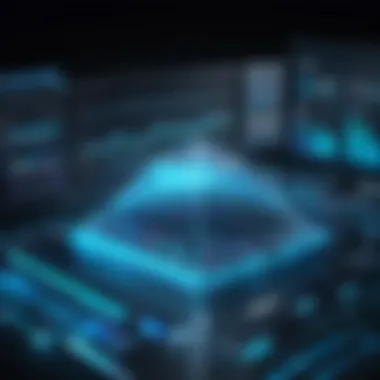
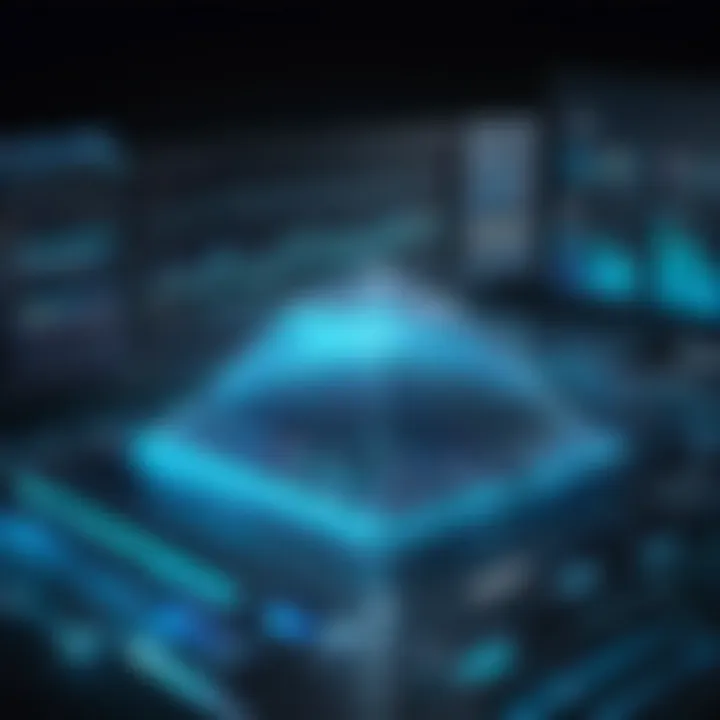
Intro
In todayâs fast-paced digital world, the landscape of enterprise data management is constantly evolving. As organizations increasingly rely on digital tools and platforms, the potential for data-related risks has become a significant concern. Every day, vast amounts of sensitive information flow through various systems, making data security a top priority for businesses of all sizes. Data breaches and compliance failures not only cause financial losses but can also severely damage an organization's reputation.
The importance of enterprise data risk management cannot be overstated. It involves a strategic approach to identifying, assessing, and mitigating risks associated with data. By understanding the nuanced nature of these risks, businesses can navigate the complexities of digital environments more effectively. Furthermore, as regulatory requirements grow stricter, having a robust data risk management framework in place isn't just advisable; it's essential.
This article serves as a conduit for tech professionals and organization leaders to grasp the critical elements of data risk management. It will explore the multifaceted nature of data risks, delve into methodologies and strategies for effective management, and highlight the role of technology in bolstering data security. Moreover, lessons learned from past incidents will pave the way for better practices in safeguarding information in today's digital age.
Understanding Data in Enterprises
In todayâs digital age, understanding data within enterprises is more than just a necessity; it is a foundational pillar for success. Organizations are flooded with data from diverse sources, and grasping its nuances is crucial. The importance of this topic lies in how data influences decision-making, operational efficiency, and ultimately, profitability.
Data is often referred to as the new oil, indicating its value when refined and managed correctly. By analyzing data effectively, businesses can anticipate market trends, improve customer experiences, and mitigate risks. Without a proper understanding of data, organizations risk making misinformed choices that can lead to operational inefficiencies and financial losses.
Moreover, companies these days also face several regulations that mandate proper data handling and management. These regulations can vary from industry to industry, yet the underlying principle remains the same: data is key to operational integrity and compliance. Thus, understanding data not only includes its value proposition but also includes the challenges posed by legal frameworks.
Definition of Data Risk
Data risk can be characterized as the possibility of losing information or compromising its integrity. This encompasses anything from data breaches to data loss caused by natural disasters, human error, or cyberattacks. The implications of these risks are profound, given the sensitive nature of information organizations process, from personal data to proprietary business information. Understanding data risk is vital for developing strategies that can minimize vulnerabilities and protect data assets.
Types of Data within Organizations
When delving into the realm of data within organizations, it is helpful to distinguish between various types. Here we examine three distinct categories of data:
Structured Data
Structured data refers to highly organized information that is easily searchable. Typically found in databases, it adheres to a pre-defined data model. Common examples include customer information stored in a CRM system or financial data kept in ERP systems. The key characteristic of structured data is its consistency, allowing companies to run queries efficiently. This organized nature makes it the preferred choice for analysis, as insights can be gleaned through straightforward data manipulation. One unique feature of structured data is its compatibility with SQL, a standardized programming language used for managing and querying databases. However, while structured data is efficient, it can be restrictive due to its rigid format, requiring significant effort to adapt to new types of information as they arise.
Unstructured Data
Unstructured data presents a contrasting situation. Unlike structured data, this type does not have a defined format, making it more challenging to analyze. Examples include social media posts, emails, or multimedia files. The key feature of unstructured data is its variety and volume; organizations may possess vast amounts of unstructured data but struggle to extract actionable insights from it. This type of data is increasingly popular, as it fuels valuable customer insights through sentiment analysis and behavioral tracking. However, the disadvantage lies in its complexity and the technology required to analyze it effectively, often needing advanced tools to derive value.
Metadata
Metadata serves as data about data. It provides context for content and can include details such as file size, creation date, and authorship. By enhancing our understanding of data assets, metadata proves beneficial for efficient data management. It helps organizations classify and retrieve data swiftly, effectively reducing time spent on data-related tasks. One unique feature of metadata is its ability to facilitate data governance through better tracking and accountability. However, if poorly managed, metadata can quickly become a double-edged sword, leading to confusion and inefficiencies.
The Importance of Data Management
In an environment saturated with data, proper data management becomes indispensable. Organizations that prioritize data management stand a better chance of safeguarding their assets against violations or unexpected losses. Effective data management not only ensures compliance with various regulations but also fosters a culture of data-driven decision-making.
Data management policies should be robust and adaptable, accommodating shifts in data volume and diversity. The clarity of these policies extends to conducting regular audits, maintaining data integrity, and ensuring that all stakeholders are aligned with the organization's data strategy. By reinforcing the importance of data management, enterprises can lay the groundwork for resilient data governance and risk management frameworks.
The Landscape of Data Risks
In todayâs rapidly evolving digital ecosystem, understanding the landscape of data risks is more crucial than ever. Organizations face a myriad of threats that can compromise data integrity, leading to substantial financial and reputational damages. This section aims to dissect these risks into manageable components, shedding light on how organizations can successfully navigate these treacherous waters.
Identifying Potential Data Risks
Identifying potential data risks is the cornerstone of effective data risk management. These risks typically come from three major categories: internal, external, and technological risks.
Internal Risks
Internally, organizations can find themselves facing data risks that stem from employee actions, whether intentional or accidental. For instance, an employee inadvertently sharing sensitive information or mishandling company data can lead to significant liabilities. The key characteristic here is the proximity of these risks; they arise within the company's own operations, making them sometimes overlooked. Internal risks are often a popular choice for this discussion because they point to a critical aspect often ignoredâorganizational culture and employee training.
One unique feature about internal risks is that they can often be mitigated through improved training and awareness programs. The advantage of addressing them proactively is that it fosters a culture of responsibility among employees, potentially reducing incidents overall. However, a disadvantage could be the fact that no matter how stringent the measures are, human error remains unpredictable.
External Risks
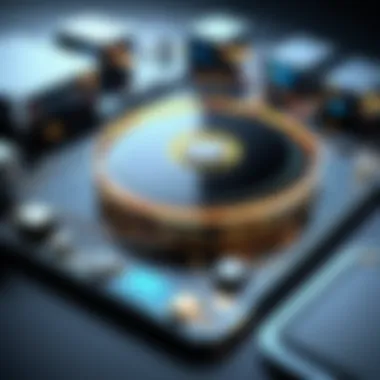
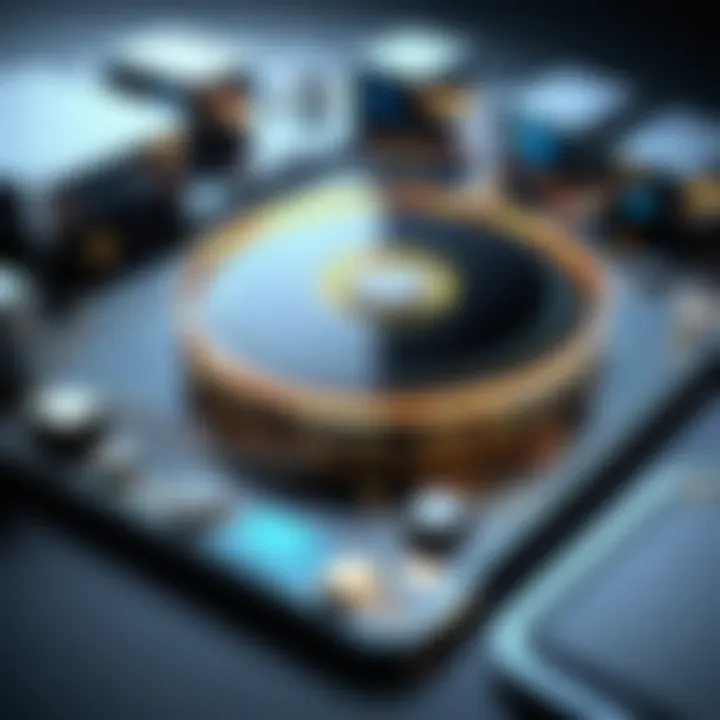
External risks, on the other hand, derive from factors outside the companyâs control. Cyberattacks, often from malicious entities, fall into this category, and their contribution to data risk management is immense. A key characteristic of external threats is their relentless nature. They are often ongoing and come in various forms, such as phishing attacks or ransomware. This makes them a key point of focus in any comprehensive data risk strategy.
What sets external risks apart is their manifestation through various sophisticated techniques, continually evolving to evade traditional security measures. The advantage of understanding these risks lies in the ability to employ advanced security protocols. On the flip side, the downside is that being caught off guard can lead to catastrophic breaches of data integrity, which could result in massive financial repercussions.
Technological Risks
Technological risks present another layer of complexity when managing enterprise data. As reliance on technology grows, so do vulnerabilities associated with it. These risks include outdated software systems, misconfigured cloud settings, and even unpatched security flaws. A key characteristic of technological risks is their rapidly changing landscapeâjust when you think you've secured one vulnerability, another arises.
These risks are frequently highlighted as beneficial for this discussion because they underscore the need for ongoing vigilance and adaptability in technology management. Their unique feature is that technological risks can often be systematically monitored and potentially automated, offering organizations the ability to respond quickly. However, they come with the disadvantage of requiring constant resources and attention to prevent lapses in security.
The Impact of Data Breaches
Data breaches can have a ripple effect through organizations, causing more than just immediate financial loss. They can erode customer trust, lead to legal challenges, and damage reputations irreparably. In a world where news travels fast, maintaining the trust of your clientele is pivotal. Organizations need to recognize that the time spent addressing a data breach can be costly not only in finances but also in customer relationships.
Regulatory Compliance Challenges
Navigating regulatory compliance is another critical aspect of the data risk landscape. With regulations like GDPR and CCPA on the rise, companies face enormous hurdles in ensuring they meet the evolving standards set forth by governing bodies.
GDPR
The General Data Protection Regulation, or GDPR, represents a comprehensive framework for data protection in Europe. It emphasizes the need for organizations to grant individuals enhanced control over their personal data. As a regulatory standard, GDPR has a key characteristic of holding organizations accountable for how they collect, process, and store data. Its benefit lies in fostering trust in data management, but the challenge is its stringent compliance requirements, which can be daunting for organizations.
CCPA
The California Consumer Privacy Act, or CCPA, mirrors GDPR but is specific to California residents. The main aspect of CCPA is its focus on consumer privacy rights, highlighting consumersâ ability to request the deletion of their information. Its popularity as a regulatory model stems from its straightforward approach to consumer rights. However, businesses may find it cumbersome to restructure their data management practices to comply fully.
Industry-Specific Regulations
Various industries face their own sets of regulations, adding yet another layer of complexity for data risk management. For example, healthcare organizations must comply with HIPAA regulations, which safeguards sensitive patient information. Each industry-specific regulation has unique features tailored to address the particular concerns inherent to that field. While compliance can enhance data protection and consumer trust, the downside is the operational burden placed on organizations to maintain adherenceâoften requiring significant resources.
Effective data risk management requires understanding not just the existing risks but also anticipating future challenges and regulations.
Frameworks for Data Risk Management
In today's digital landscape, having a robust framework for data risk management is not just a good idea; it's a necessity. Organizations are navigating through a maze of complex data challenges, from compliance with regulations to the safeguarding of sensitive information. A well-structured framework serves as a guiding compass for navigating these turbulent waters. It outlines clear roles, responsibilities, and processes while aligning with the organizationâs overall objectives. This coherence ensures that data risks are managed proactively, rather than reactively, which can save an organization a world of trouble down the line.
Establishing a Data Governance Structure
Creating a data governance structure is akin to laying down the foundation of a sturdy building. You wouldn't construct a skyscraper without a solid base, right? Likewise, without a well-defined governance structure, data risk management initiatives are likely to crumble under pressure.
A strong data governance framework involves the establishment of roles such as data stewards and data custodians, who are responsible for maintaining data integrity and security. This structure not only enforces accountability but also streamlines data management processes across departments. The active participation of various stakeholders ensures that every angle is covered.
Risk Assessment Methodologies
Methodologies for assessing data risks can be grouped mainly into two types: qualitative and quantitative. Both methodologies aim to identify vulnerabilities and their potential impact on the organization, but they approach this task in distinct ways.
Qualitative Assessments
Qualitative assessments dive into the subjective side of risk management. They rely on expert judgment, interviews, and heuristic techniques to gauge the level of risk associated with various data assets. This methodology emphasizes understanding the context of risks rather than just calculating probabilities. One key characteristic of qualitative assessments is their ability to identify risks that may not be easily quantifiable.
This approach proves beneficial, especially in organizations that deal with intricate systems or where data has unique contextual significance. For instance, in healthcare settings, the risk of data loss can involve not just financial implications but also potential harm to patients. The unique feature here is the deep qualitative insights it provides, though it can sometimes leave gaps where hard data may offer better clarity.
Quantitative Assessments
On the flip side, quantitative assessments lean more on the numbersâstatistics, incident reports, and mathematical models form the cornerstone of this methodology. It aims to provide measurable data that can justify decisions. A key characteristic is its ability to deliver concrete, data-driven insights, which can often be persuasive for upper management. For instance, allocating budget based on the projected cost of potential data breaches can guide strategic funding decisions. The unique feature of this approach is its emphasis on numerical data, linking risks directly to financial impacts. However, organizations must tread carefully; an over-reliance on numbers can obscure the nuanced understanding of risks that qualitative assessments capture.
Creating a Risk Management Plan
Drafting a risk management plan can be thought of as creating a roadmap. It outlines how an enterprise will address identified risks and response strategies. This plan should be iterative, evolving with the changing data landscape. An effective plan includes prioritization of risks, clearly defined response strategies, and consistent documentation. Additionally, testing and revising the plan should be standard procedure, ensuring readiness in the face of unforeseen data threats.
In summation, a well-structured framework for data risk management not only ensures compliance but aids in maintaining stakeholder trust. Navigating the pitfalls of data risks demands vigilance, and organizations should not undertake this responsibility lightly.
"Strong governance is not merely a box-ticking exercise; it's a powerful strategy that aligns risk management with business objectives and cultivates trust."
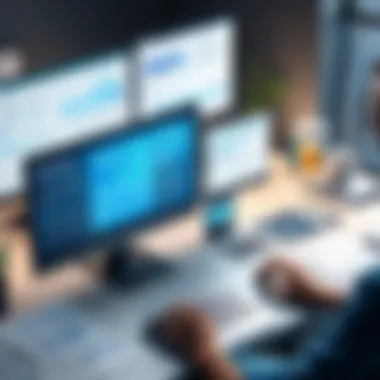
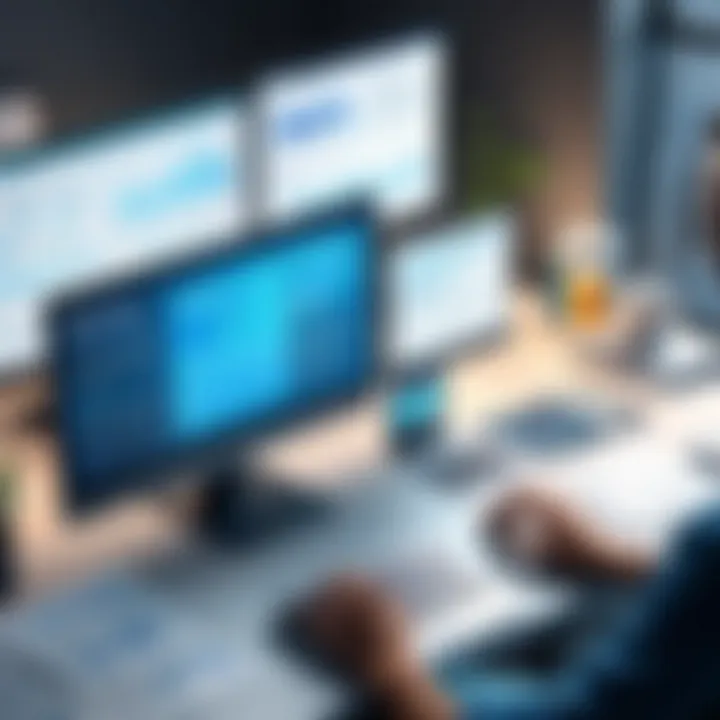
Leveraging qualitative and quantitative assessments helps provide a comprehensive understanding of risks while crafting a risk management plan will fortify the organizationâs defenses against data threats.
Technological Solutions for Data Risk Management
In todayâs digital world, where data is the new currency, managing the associated risks demands a tech-savvy approach. Technological solutions are key to enhancing the security and integrity of organizational data. They not only help in identifying vulnerabilities but also establish defenses against potential threats. Technologies can streamline processes, offer advanced security measures, and foster a culture of proactive risk management.
Data Encryption Techniques
Data encryption techniques serve as a cornerstone in safeguarding sensitive information. By converting data into a coded format that is unreadable to unauthorized users, organizations can protect their assets from prying eyes.
Key benefits of employing encryption include:
- Data protection during transmission and storage.
- Compliance with regulations, as encryption often satisfies legal requirements for data protection strategies, such as GDPR.
- Versatile applications, whether for email, database, or cloud storage protection.
However, companies must also consider the trade-offs involved. For instance, urgent access to sensitive information can be delayed if a complex encryption key management system is in place. Furthermore, if encryption is not accompanied by robust access controls, it can create a false sense of security.
Utilizing Machine Learning in Risk Prevention
Machine learning harnesses algorithms that can learn from and make predictions based on data. It provides organizations with an edge in recognizing patterns and anomalies that typical security measures might miss. By analyzing historic data breaches or unusual access patterns, machine learning can enhance predictive capabilities and strengthen defenses against potential threats.
The benefits of integrating machine learning for risk prevention include:
- Automated threat detection that operates in real-time.
- The ability to analyze massive datasets, identifying risks that human analysts might overlook.
- Continuous learning systems that adapt and evolve as threats change.
On the flip side, reliance on machine learning can lead to overfittingâwhere the system becomes too specialized and misses broader threats. Organizations should maintain a balance, incorporating human oversight to validate findings and manage risks judiciously.
Cloud Security Measures
With more companies migrating to cloud-based systems, robust cloud security measures have become indispensable. These strategies help to protect data not just during storage but throughout its lifecycle, from creation to deletion.
Some effective cloud security measures include:
- Access control policies that define who can view or modify data.
- Data loss prevention protocols to safeguard against accidental or malicious data breaches.
- Encrypted storage and network connections to secure data integrity in transit.
Nevertheless, companies should be mindful that cloud providers often have varying levels of security. Choosing the right partner with strong compliance and security certifications is crucial. Oversight of the shared responsibility model between the provider and user ensures that neither side inadvertently exposes sensitive information.
"Technology is the backbone of data risk managementâwithout it, organizations are left exposed to a multitude of threats."
Adopting these technological solutions isnât just about patching holes; itâs about building a resilient framework that anticipates and adapts to new threats. Organizations equipped with the right technologies will be much better positioned in their fight against data risk.
Crisis Management and Recovery
In the realm of enterprise data management, the shadow of crisis looms large. Whether it's a minor data mishap or a full-blown breach, the consequences can be severe and far-reaching. Consequently, having a robust crisis management and recovery strategy isn't just nice to have; it's a necessity. This section examines the essential components of crisis management and recovery, spotlighting how organizations can safeguard their data, protect their reputation, and ensure continuity in operations.
Developing an Incident Response Plan
An effective incident response plan is the cornerstone of any data risk management strategy. This plan serves as a playbook, outlining clear steps to follow once an incident occurs. It's crucial to establish roles and responsibilities among team members to eliminate confusion when the clock is ticking.
Consider the various types of incidents you might face, from data breaches to system failures. Each type will require tailored responses that your plan should address. Include elements such as:
- Detection and Analysis: Timely identification of an incident can minimize damage. Train your team to recognize early warning signs.
- Containment Strategies: Quickly isolate affected systems to prevent the breach from spreading.
- Eradication and Recovery Methods: Identify the root cause and clean up the system to restore normality.
- Post-Incident Review: Conduct a thorough analysis after an incident to learn what went right and wrong.
Ultimately, your incident response plan should be a living document, consistently updated to reflect evolving threats and operational adjustments.
The Role of Culture in Data Risk Management
When it comes to safeguarding enterprise data, one aspect that deserves close attention is the culture within the organization. It's like the backdrop against which all data management policies play out. Without a strong, risk-aware culture, even the most sophisticated data security measures can falter. Organizations need to understand that in this digital age, protecting information isn't solely a technical concern but also a matter of ideology and behavior.
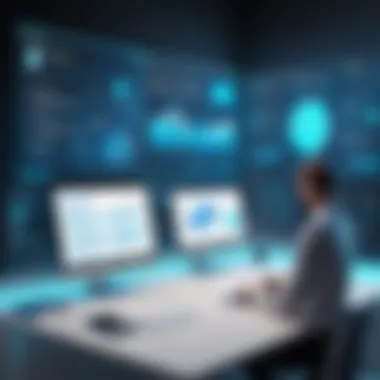
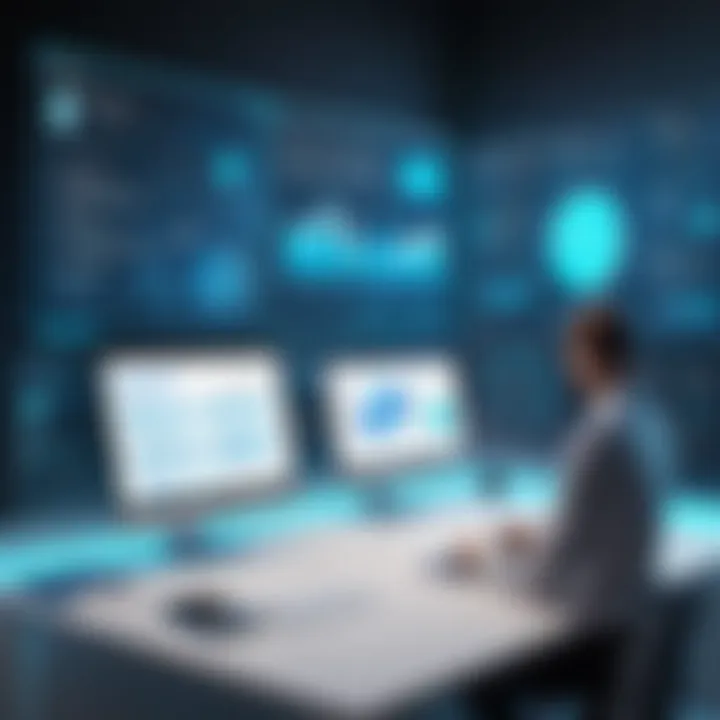
A cohesive cultural framework encourages employees to recognize their role in data security, shaping how they interact with sensitive information. It emphasizes that data risk management isnât just the IT department's job; itâs a collective responsibility. This cultural shift can lead not only to better compliance with regulations but also to a more resilient organization capable of adapting to data risks that evolve daily.
Training and Awareness Programs
Training and awareness programs are the bedrock of a risk-aware culture. Itâs crucial for organizations to offer regular training sessions that donât just cover compliance checks but engage employees deeply with the principles of data security and risk management.
These sessions should incorporate:
- Real-world Case Studies: Discussing actual breaches and how they could have been prevented can paint a vivid picture for employees. It can be shocking to realize how easily a small misstep may lead to major consequences.
- Interactive Scenarios: Engaging staff in role-playing exercises allows them to navigate potential data risk situations and think critically about their actions and decisions.
- Regular Updates: Given the fast-paced nature of technological advancements and changing regulations, periodic training sessions should be mandatory. Employees must stay abreast of emerging threats and the latest best practices.
Implementing these programs not only equips employees with knowledge but instills a sense of ownership related to data protection.
Encouraging a Risk-Aware Culture
To encourage a risk-aware culture, leadership plays a pivotal role. Executives should model desired behaviors. If decision-makers prioritize data risk management and demonstrate a commitment in their actions, employees are likely to mirror this attitude.
Several strategies can help foster this environment:
- Open Communication Channels: Create systems where employees feel comfortable reporting potential data risks or breaches. A culture of transparency can dismantle the idea that speaking out is punitive.
- Recognition and Rewards: Acknowledge and reward proactive behavior among employees that contributes to data protection. Highlighting individual contributions can boost morale and motivation.
- Collaborative Environment: Encourage cross-departmental collaboration to share responsibilities in managing data risks. Different teams, such as IT, HR, and Sales, need to work together to understand how their specific processes relate to seamless data protection.
Future Trends in Data Risk Management
In the ever-changing landscape of data, organizations must remain vigilant and proactive. Future trends in data risk management unveil a fascinating interplay between emerging technologies, evolving regulatory frameworks, and adaptive organizational cultures. As companies continue to grapple with the risks associated with their data, understanding these trends becomes crucial for securing sensitive information and ensuring long-term success.
Organizations that keep their finger on the pulse of data risk management will find themselves better equipped to face new challenges and exploit opportunities in the digital domain. This section will shed light on the significance of these trends, while highlighting specific elements that are gaining traction.
Emerging Technologies and Their Impact
Emerging technologies play a pivotal role in shaping the future of data risk management. From artificial intelligence to blockchain, these innovations offer unique advantages that can significantly enhance data security.
- Artificial Intelligence (AI): AI is not just for smart assistants anymore. In the context of data risk management, it can analyze data patterns to identify anomalies and potential threats. By automating risk detection, companies can respond swiftly before a minor issue escalates into a major crisis.
- Blockchain Technology: This decentralized approach to data storage and transactions ensures greater transparency and accountability. It can reduce the risk of unauthorized data tampering, making it an appealing option for organizations looking to bolster their data integrity.
- Internet of Things (IoT): With more devices connected to the Internet, the potential attack surfaces are expanding. IoT devices must be safeguarded with layers of security measures. Implementing robust data governance practices becomes vital here, as every connected device can potentially serve as a gateway for cyber threats.
Emerging technologies can empower organizations to not only mitigate risks but also streamline operations. However, itâs important to remember that these advancements also come with challenges. For instance, integrating AI solutions may require upskilling staff, while balancing security with user convenience continues to be a fine line to tread.
The Evolution of Regulatory Expectations
The regulatory landscape is not one to overlook when discussing future trends. As data privacy concerns escalate, so too do the expectations placed on businesses. Regulators are tightening the screws, making compliance not just a checklist item but an essential part of corporate strategy.
- GDPR and Beyond: The General Data Protection Regulation (GDPR) turned heads worldwide when it came into effect. This standard isnât just about penalties; itâs about reshaping organizational attitudes toward data protection. Companies must now prioritize their data practices, ensuring transparency and accountability.
- Increasing Global Standards: Following GDPR, other regions and countries are also enacting stringent data protection laws. The California Consumer Privacy Act (CCPA) is a prime example. As compliance becomes a global phenomenon, organizations must tailor their strategies to meet diverse regulatory demands.
- Proactive Adaptation: Future trends indicate a shift from reactive compliance to a more proactive approach. Businesses that anticipate regulatory changes, instead of scrambling after the fact, are likely to build stronger reputations and engender trust with clients and stakeholders.
"In this digital age, compliance isnât merely about avoiding fines; itâs a testament to an organizationâs integrity and commitment to safeguarding user data."
As organizations look to the future of data risk management, integrating emerging technologies while embracing evolving regulations can create a resilient framework. Itâs about more than just keeping data safe; itâs about fostering a culture of proactive risk awareness and adjustment.
In this arena, those who stay ahead of the curve will not only protect their interests but will also earn the trust of their clients and stakeholders, ensuring a competitive advantage in a data-driven marketplace.
Ending and Recommendations
In an era where data flows like water, the capacity to manage data risks effectively is not just an option but a necessity for organizations. As we draw insights from earlier parts of this article, it becomes clear that data risk management encompasses a range of factors, from identifying potential risks to utilizing technology for data protection. The inclusion of clear strategies and best practices allows enterprises to bolster their defense against inevitable data challenges, ensuring that sensitive information remains secure.
Effective data risk management not only protects an organizationâs critical assets but also cultivates trust with stakeholders. This trust is paramount in fostering a reliable business reputation and, by extension, driving growth. So, effective measures can create a protective buffer that strengthens an organizationâs operational resilience.
Summary of Key Points
- Comprehensive Understanding: Organizations must grasp various data types and their associated risks â both internal and external.
- Governance Framework: Establishing structured data governance can address regulatory compliance issues and enhance risk mitigation protocols.
- Technological Integration: Utilizing cutting-edge tools such as encryption and machine learning aids significantly in recognizing and preventing data breaches before they escalate.
- Culture Shift: Developing a risk-aware culture is essential; education and training programs equip employees with the knowledge to recognize and respond to data threats.
"Data isnât just numbers; itâs a reflection of trust, potential, and responsibility. "
The future of a data-driven enterprise hinges on how well it can pivot and adapt to changes in the data ecosystem.
Next Steps for Organizations
Organizations should take a proactive stance in data risk management by following these actionable steps:
- Conduct Comprehensive Risk Assessments: Regularly evaluate potential data risks within both internal and external environments. This should include an analysis of past breach incidents for learning purposes.
- Enhance Data Governance and Compliance: Implement robust frameworks that adhere to various regulatory standards, which not only ensure compliance but also instill a culture of accountability and transparency among employees.
- Invest in Latest Technologies: Leverage advancements in technology such as AI and cloud security tools for efficient management and protection of data assets.
- Build a Risk-Aware Culture: Foster an environment where every employee understands their role in data protection. Provide ongoing training and awareness programs to help them stay vigilant.
- Establish Incident Response Protocols: Prepare for the worst. This includes having well-documented plans that detail the steps to take when a data breach occurs, ensuring a swift and controlled response.