Unraveling the Enigma of Deep Learning: An Insightful Discourse
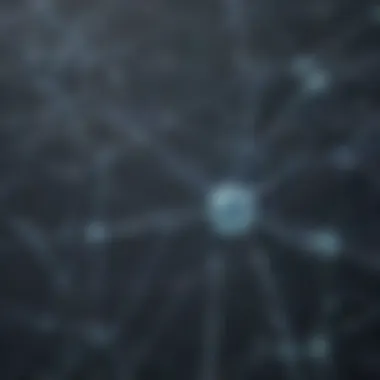

Tech Trend Analysis
In the realm of technological advancements, deep learning stands out as a pivotal trend, revolutionizing how machines process and analyze data. The current trend showcases a growing reliance on neural networks to tackle complex tasks that were once exclusive to human intelligence. Consumers are gradually witnessing the practical implications of deep learning through the seamless automation of processes, predictive analytics, and enhanced machine capabilities. Looking ahead, the future holds exciting possibilities, with deep learning poised to further blur the lines between artificial and human intelligence.
Product Reviews
While deep learning itself is not a tangible product per se, its applications manifest in various innovative tools and technologies. These developments boast an array of features and specifications tailored to deliver efficient and accurate results. Through meticulous performance analysis, it becomes evident that deep learning systems exhibit unparalleled potential in handling vast datasets and intricate algorithms. As with any technological advancement, there exist both pros and cons, which must be weighed carefully to determine the best use cases. Ultimately, a thoughtful recommendation is crucial to guide users towards leveraging deep learning effectively.
How-To Guides
Embarking on a journey to understand deep learning requires a foundational introduction to the topic. Breaking down the concepts and mechanisms behind neural networks and layered algorithms sets the stage for a comprehensive grasp of this sophisticated field. Step-by-step instructions unravel the complexities of training models, recognizing patterns, and optimizing algorithms. In this technological voyage, useful tips and tricks enhance the learning process, offering insights into refining model performance and troubleshooting common challenges along the way.
Industry Updates
Keeping abreast of recent developments in the tech industry sheds light on the continuous evolution of deep learning applications. Analyzing market trends unveils the shifting landscape of artificial intelligence and its impact on businesses and consumers alike. These updates offer invaluable insights into the expanding role of deep learning in reshaping industries and driving innovation. By understanding these trends and implications, industry professionals can adapt their strategies to harness the full potential of deep learning technologies.
Introduction
In the realm of modern technological advancements, where artificial intelligence reigns as a pinnacle of innovation, the essence of deep learning emerges as a powerful force shaping the landscape of data analysis and decision-making processes. As we embark on a journey to demystify the complexities entwined within deep learning, it is crucial to grasp the fundamental concepts that underpin this intricate domain. Through a meticulous exploration of neural networks, algorithmic layers, and pattern recognition mechanisms, this article serves as a beacon of comprehension amid the intricate web of artificial intelligence.
Defining Deep Learning
Understanding the Basics
Delving into the core essence of deep learning unveils a realm where neural networks converge to mimic human cognitive processes through intricate layers of algorithms. The inherent prowess lies in the adeptness of these networks to discern intricate patterns within vast datasets, enabling them to make informed decisions akin to human intelligence. Embracing 'Understanding the Basics' illuminates the foundational principles that steer deep learning towards unveiling the true potential of artificial intelligence.
Distinguishing Deep Learning from Machine Learning
Drawing a distinct line between deep learning and machine learning delineates their unique paradigms in the realm of artificial intelligence. Unlike machine learning, deep learning thrives on complex hierarchical architectures that enhance the learning capacity of neural networks. The quintessence of 'Distinguishing Deep Learning from Machine Learning' lies in the intricate web of neural connectivity that empowers deep learning models to decipher intricate patterns with unparalleled precision, setting them apart in the realm of advanced data analytics.
Significance of Deep Learning
Revolutionizing Artificial Intelligence
The transformative impact of deep learning on the landscape of artificial intelligence resonates with unrivaled potential, reshaping conventional paradigms with its adaptive learning mechanisms. 'Revolutionizing Artificial Intelligence' epitomizes the paradigm shift ushered in by deep learning, where neural networks harness vast datasets to perform tasks hitherto deemed exclusive to human cognition. Embracing this facet underscores the monumental leap towards a future driven by intelligent data analytics.
Applications Across Industries
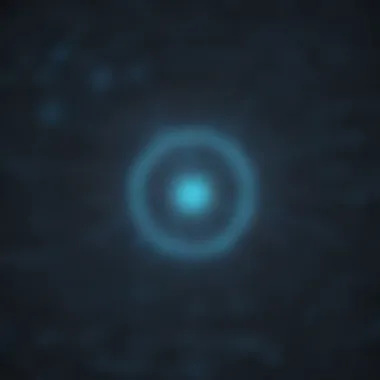
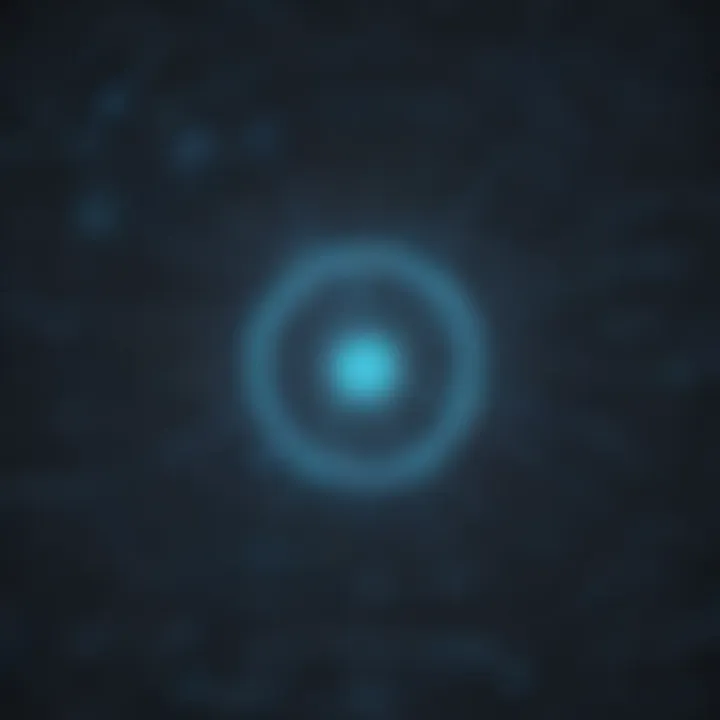
Nurturing deep learning's expansive reach across diverse industries heralds a new era of innovation and efficiency, transcending conventional boundaries to empower sectors with unprecedented analytical capabilities. 'Applications Across Industries' delineate the symbiotic relationship between deep learning and multifaceted sectors, showcasing its versatility in revolutionizing healthcare, finance, e-commerce, and beyond. The myriad applications underscore the adaptability and scalability of deep learning algorithms, paving the way for bespoke solutions in varied domains.
Evolution of Deep Learning
Historical Context
Tracing the lineage of deep learning unveils a rich tapestry of evolution, echoing the endeavors of pioneering researchers who laid the groundwork for contemporary advancements in artificial intelligence. 'Historical Context' elucidates the seminal moments and breakthroughs that culminated in the birth of deep learning, underscoring the iterative progression towards sophisticated neural networks. Delving into this chronological narrative illuminates the transformative journey that shapes our understanding of artificial intelligence.
Advancements Leading to Current Capabilities
The narrative of deep learning's evolution is punctuated by a series of breakthroughs and innovations that have propelled neural networks towards unprecedented capabilities. 'Advancements Leading to Current Capabilities' encapsulate the culmination of research endeavors and technological innovations that have augmented the efficacy and efficiency of deep learning models. Exploring these advancements offers a glimpse into the dynamic landscape of artificial intelligence, where constant refinement and innovation converge to redefine the boundaries of intelligent data analysis.
Foundations of Deep Learning
Deep learning is a fundamental aspect of modern artificial intelligence (AI). This section delves into the core concepts and functionalities that underpin the intricate processes of deep learning. Understanding the foundations of deep learning is crucial as it forms the backbone of neural network training and pattern recognition. By exploring neural networks, activation functions, and backpropagation, one can grasp the inner workings of AI algorithms and their applications in various industries. Building a strong foundation in deep learning sets the stage for advancements and innovations in technology.
Neural Networks
Basic Structure
Neural networks consist of interconnected nodes that mimic the human brain's neurons. These nodes are organized in layers, with each layer processing specific information. The basic structure of neural networks includes input, hidden, and output layers, each playing a vital role in information processing. The interconnected nature of nodes allows for complex data analysis and pattern recognition, making neural networks a powerful tool in AI development. Despite their effectiveness, neural networks require extensive computational resources to train and optimize, posing a challenge for scalable implementation.
Functionality in Deep Learning
Within deep learning, neural networks serve as the primary architecture for data processing and decision-making. The functionality of neural networks lies in their ability to learn from large datasets and adapt to changing patterns. This adaptive nature enables neural networks to improve their accuracy over time, making them ideal for tasks that involve complex data structures. However, the flexibility of neural networks can also lead to overfitting, where the model performs well on training data but fails to generalize to new instances. Balancing model complexity and generalization is essential in maximizing the performance of neural networks within deep learning applications.
Activation Functions
Role in Information Processing
Activation functions play a crucial role in neural networks by introducing non-linearities into the model. These functions control the output of individual nodes based on the input received, allowing for complex decision-making processes. In deep learning, activation functions enable neural networks to model intricate patterns and relationships within data, enhancing the model's capacity to learn and adapt. The choice of activation function impacts the network's ability to converge during training, influencing both computational efficiency and predictive accuracy.
Popular Activation Functions
Within the realm of deep learning, popular activation functions such as ReLU (Rectified Linear Unit) and Sigmoid function are widely used for their computational efficiency and interpretability. ReLU, in particular, offers simplicity and effectiveness in capturing non-linear transformations within data, making it a popular choice for many neural network architectures. On the other hand, the Sigmoid function provides smooth gradients that aid in stable training and convergence. Understanding the characteristics and nuances of popular activation functions is essential in designing neural networks that balance performance and interpretability.
Backpropagation
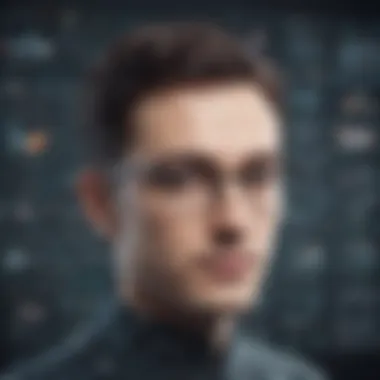
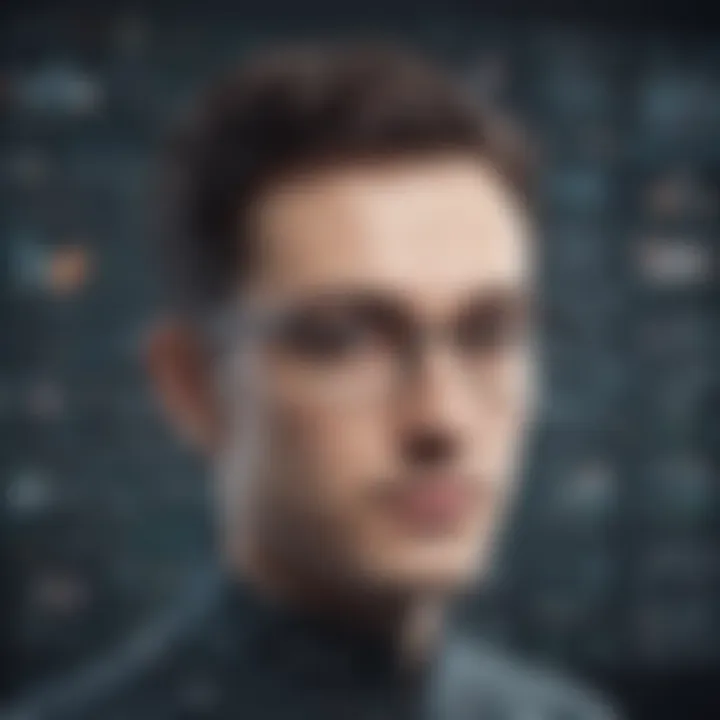
Training Neural Networks
Backpropagation is a key algorithm in deep learning that enables neural networks to learn from their mistakes. By propagating errors backwards through the network, backpropagation adjusts the model's weights and biases to minimize prediction errors. This iterative process of updating parameters based on gradient descent is essential for improving model accuracy and convergence. However, backpropagation requires careful tuning of hyperparameters to prevent issues such as vanishing or exploding gradients, which can hinder training progress.
Optimizing Model Parameters
Optimizing model parameters is a critical step in deep learning to enhance model performance and efficiency. By fine-tuning parameters such as learning rate, batch size, and regularization techniques, practitioners can improve the generalization and robustness of neural networks. Regularization methods like L1 and L2 regularization help prevent overfitting by adding penalty terms to the loss function, guiding the model towards simpler and more generalized solutions. Understanding the intricacies of optimizing model parameters is essential for achieving optimal performance in deep learning tasks.
Deep Learning Models
Deep learning models play a pivotal role in our exploration of the intricacies of deep learning. These models serve as the backbone of many artificial intelligence applications and are engineered to replicate the human brain's ability to analyze data and make informed decisions. Deep learning models hold the key to unlocking the potential of neural networks, enabling them to discern complex patterns and extract meaningful insights.
Convolutional Neural Networks (CNNs)
In the realm of deep learning, Convolutional Neural Networks (CNNs) stand out as an essential tool for tasks requiring image recognition. The unique architecture of CNNs allows them to efficiently process visual data by convolving input images with learnable filters or kernels. This process enables them to recognize intricate patterns and shapes within images, making them indispensable for applications like object detection, facial recognition, and medical image analysis.
Image Recognition
Image recognition is the core functionality of CNNs, enabling machines to identify and classify objects within digital images with remarkable precision. By leveraging convolutional layers, pooling layers, and activation functions, CNNs can detect features at various levels of abstraction, facilitating accurate image analysis and interpretation. The ability of CNNs to automate the extraction of features from images simplifies tasks that require understanding visual content, revolutionizing industries like healthcare, autonomous vehicles, and security systems.
Applications in Computer Vision
The applications of CNNs in computer vision are vast and varied, revolutionizing how machines interpret visual data and interact with the environment. From enhancing surveillance systems to facilitating augmented reality experiences, CNNs empower machines to perceive and comprehend their surroundings with human-like acuity. By leveraging intricate architectures and sophisticated algorithms, CNNs pave the way for innovations across sectors, changing the landscape of technology and human-machine interaction.
Recurrent Neural Networks (RNNs)
Recurrent Neural Networks (RNNs) stand at the forefront of sequential data analysis in deep learning, playing a crucial role in processing time-series data and sequential information. The unique recurrent connections within RNNs enable them to retain memory and contextual information, making them adept at tasks involving natural language processing, speech recognition, and sentiment analysis.
Sequential Data Analysis
RNNs excel in analyzing sequences of data by capturing dependencies and relationships between elements across time steps. This ability is invaluable in tasks requiring an understanding of temporal patterns and context, such as predicting stock market trends, generating text, and recognizing speech patterns. RNNs' recursive nature allows them to iterate over sequences dynamically, making them suitable for dynamic and evolving datasets.
Language Processing
Language processing is a fundamental application of RNNs, empowering machines to understand, generate, and translate human languages. By processing text sequentially and learning from the context of previous words, RNNs can generate coherent sentences, detect sentiment in reviews, and power language translation tools. The versatility of RNNs in handling sequential data makes them indispensable for various natural language processing tasks, enriching applications in communication, content analysis, and information retrieval.
Generative Adversarial Networks (GANs)
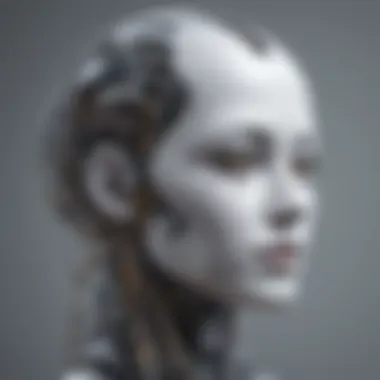
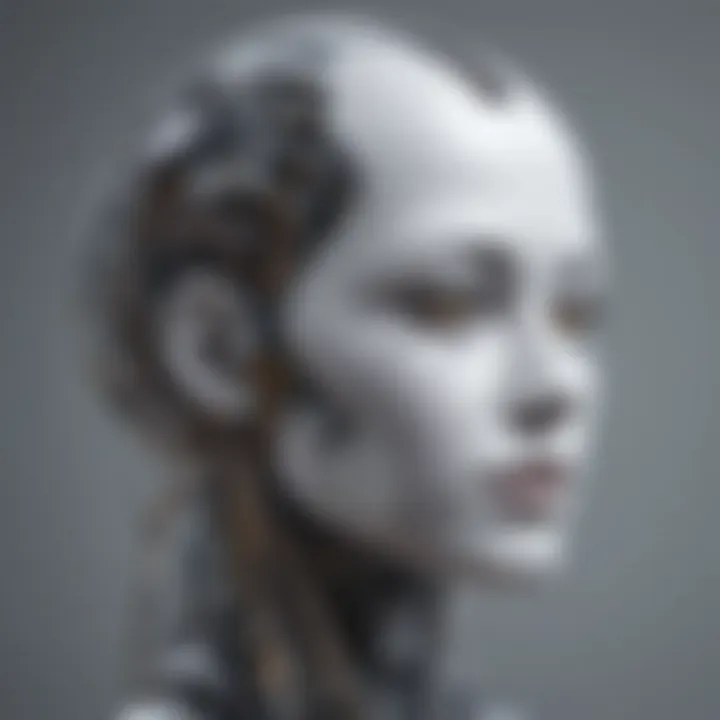
Generative Adversarial Networks (GANs) revolutionize the creation of synthetic data and fuel advancements in creative applications like image generation, style transfer, and content creation. Comprising two neural networks - a generator and a discriminator - GANs engage in a competitive learning process, driving creativity and innovation in the realm of artificial intelligence.
Creating Synthetic Data
GANs excel in generating realistic synthetic data by learning the underlying patterns and distributions of training data. This capability is instrumental in scenarios where labeled data is scarce or costly to obtain, enabling researchers and practitioners to augment datasets for training machine learning models effectively. By producing diverse and authentic synthetic data, GANs enrich the learning process, enhancing model performance and robustness.
Use in Creative Applications
The utilization of GANs in creative applications opens up a world of possibilities for artists, designers, and innovators seeking to explore new avenues of expression. From generating lifelike artworks to transforming visual styles, GANs provide a playground for creativity and experimentation. By harnessing the power of adversarial training, GANs stimulate imagination and redefine the boundaries of AI-driven creativity, fostering novel solutions and artistic endeavors.
Challenges and Future Trends
In the realm of deep learning, understanding challenges and future trends plays a pivotal role in shaping the landscape of artificial intelligence. Delving into the complexities inherent in optimizing neural networks, practitioners are confronted with the pervasive issue of overfitting. Overfitting, a common hurdle, occurs when a model performs exceptionally well on training data but fails to generalize to unseen data. To counter this challenge, mitigation strategies are essential. These strategies encompass a spectrum of techniques aimed at enhancing a model's ability to perform well on new data by preventing it from excessively fitting to the training set.
Mitigation Strategies
Mitigation strategies form a critical component of deep learning practices as they focus on rectifying overfitting issues. By implementing regularization methods such as L1 or L2 regularization, dropout, and early stopping, practitioners can curb the model's tendency to overfit. Regularization techniques impose penalties on the model's parameters, preventing them from becoming too large and leading to overfitting. Dropout, a widely used technique, randomly deactivates neurons during training, thus fostering robustness and preventing the reliance on specific neurons. Early stopping, a strategic approach, halts the training process once the model starts overfitting, thereby preserving its generalization capability.
Balancing Complexity and Generalization
Achieving a balance between complexity and generalization is a nuanced art in deep learning. Models that are overly complex risk overfitting due to their capacity to memorize training data. On the contrary, overly simplistic models may struggle to capture the intricacies of the underlying patterns, leading to poor generalization. The crux lies in navigating this delicate equilibrium, where models need to be sophisticated enough to grasp intricate patterns yet not overly complex to memorize data. By striking this equilibrium, practitioners can ensure that their models generalize effectively while retaining the capacity to discern complex patterns.
Interpretable AI
Interpretable AI stands at the forefront of ethical and practical considerations within the deep learning domain. Comprehending how models arrive at decisions is crucial, particularly in sensitive applications like healthcare or finance. Understanding model decisions entails unraveling the 'black box' nature of deep learning models to decipher the factors influencing their outputs. By elucidating the decision-making process, stakeholders can gain insights into why a model makes specific predictions, enhancing transparency and accountability.
Understanding Model Decisions
Understanding model decisions involves deciphering the intricate mechanisms driving a model's output. By visualizing feature attributions or creating decision trees, practitioners can gain visibility into the factors contributing to a model's predictions. Techniques such as SHAP (SHapley Additive exPlanations) or LIME (Local Interpretable Model-agnostic Explanations) facilitate the interpretation of complex models by highlighting the relevance of input features. This interpretability not only fosters trust in the model but also enables practitioners to identify biases or erroneous correlations that may impact decision-making.
Ethical Implications
Exploring the ethical dimensions of AI is imperative to mitigate potential harms and biases embedded within deep learning systems. Ethical implications encompass a spectrum of considerations, ranging from data privacy and security to fairness and accountability. Scrutinizing the ethical ramifications of AI algorithms entails evaluating the societal impacts of automated decision-making processes and the potential amplification of existing biases. By proactively addressing these ethical quandaries, stakeholders can uphold ethical standards and promote the responsible deployment of AI technologies.
Advancements in Deep Learning
The realm of deep learning is continually evolving, spurred by groundbreaking advancements that push the boundaries of artificial intelligence. These advancements herald a new era of interpretability and autonomy within AI systems, reshaping the paradigms of traditional machine learning approaches.
Explainable AI
Explainable AI epitomizes the quest for transparency and understanding in complex machine learning models. By elucidating the decision-making processes of AI models, explainable AI enhances trust and interpretability. Through visualizations, feature importance scores, and attention mechanisms, practitioners can navigate the inner workings of black-box models, unveiling the rationale behind specific predictions.
Self-supervised Learning
Self-supervised learning represents a paradigm shift in how models are trained, emphasizing autonomous learning without the need for annotated labels. By leveraging the intrinsic structure of data, self-supervised learning enables models to discern meaningful relationships and patterns independently. This unsupervised learning paradigm not only reduces dependency on labeled data but also fosters adaptability and generalization across diverse domains.