The Essential Role of Data Scientists in Oil and Gas
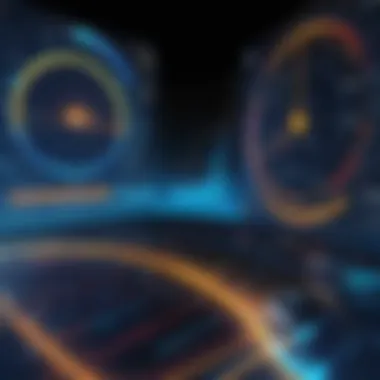
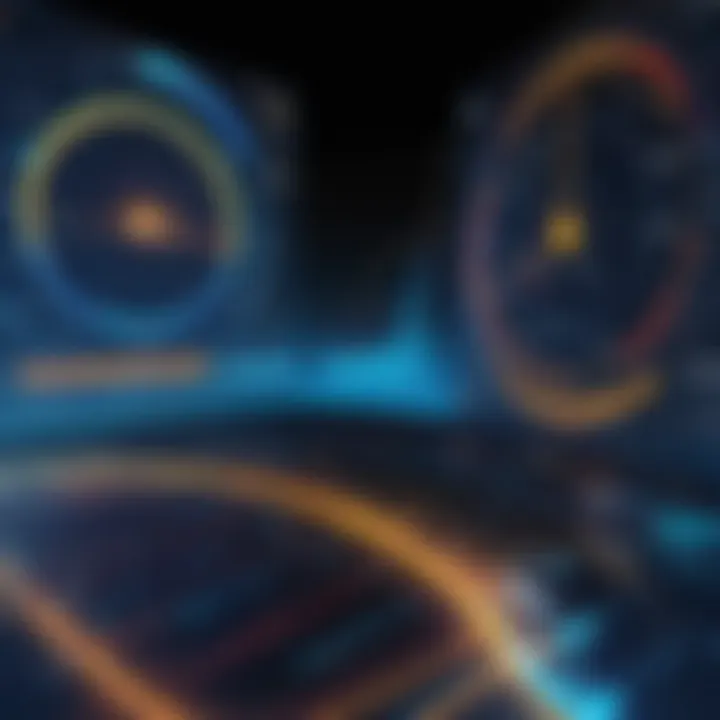
Intro
The oil and gas industry, a backbone of the global economy, is experiencing a significant transformation, driven by the advent of data science. As this sector grapples with the complexities of modern energy demands and environmental concerns, data scientists emerge as pivotal players. They analyze vast amounts of data, from exploration and drilling to production and distribution, to enhance operational efficiency, mitigate risks, and foster innovation. This article takes a closer look at the role of data scientists in oil and gas, documenting their methodologies, challenges, and the implications for decision-making. Understanding this evolving role is critical not just for industry insiders but for anyone interested in the intersection of technology and energy.
Tech Trend Analysis
Overview of the Current Trend
In the realm of oil and gas, the integration of data science has become increasingly prominent. With the rise of big data, companies are leveraging advanced analytics and machine learning techniques to optimize exploration and production processes. Data scientists are tasked with the analysis of geological data, seismic readings, and operational statistics to uncover patterns that human analysts might overlook. This shift is not just about making tasks easier; it’s about smart, data-driven decision making that can ultimately save time and money.
Implications for Consumers
As data scientists refine processes through data analytics, consumers stand to gain significantly. Improved efficiency in extraction and production translates to a more stable supply chain, which can lead to lower fuel prices. Moreover, embracing predictive analytics can help companies anticipate market demands, ensuring that consumers have access to resources when needed. This upshot not only supports economic stability but also enhances transparency in how resources are managed.
Future Predictions and Possibilities
Looking ahead, the future of data science in the oil and gas industry is considerably bright. We can expect increased reliance on artificial intelligence to fine-tune financial estimations and regulatory compliance while simultaneously managing environmental impacts. Virtual reality and augmented reality also hold potential for training purposes, offering immersive experiences for new employees, enhancing safety and efficiency.
"The use of data science is not merely a trend; it's a paradigm shift in how the oil and gas industry operates."
Methodologies in Data Science
Common Techniques Employed
Data scientists utilize various methodologies, including:
- Statistical Analysis: To determine relationships between variables, making sense of historical data trends.
- Machine Learning: For predictive modeling and automating decision-makin processes, which can adapt over time as more data becomes available.
- Natural Language Processing: Used for sifting through unstructured data, such as reports and operational texts, to extract actionable insights.
Each method plays a unique role in informing the company's strategy, ensuring adaptability in a volatile market.
Challenges in Data Integration
One cannot ignore the myriad of challenges that accompany data integration in oil and gas. These can include:
- Data Silos: Often, departments operate in isolation, which prevents sharing of valuable insights.
- Data Quality: Ensuring accurate, clean data is essential; otherwise, flawed analyses may lead to poor decision making.
- Skill Gaps: There's a pressing need for professionals who not only understand data but also have the expertise in oil and gas operations.
Overcoming these hurdles requires coordinated efforts across departments and ongoing education.
End
Preamble to Data Science in Oil and Gas
The integration of data science into the oil and gas industry isn't just a passing trend; it's reshaping the entire landscape. As companies face pressures to enhance productivity, minimize costs, and adhere to stricter environmental standards, the need for precise data analytics becomes essential. Data scientists play a pivotal role in this transition, providing insights that drive innovation and operational efficiency.
By harnessing the power of data, these professionals equip organizations to make informed decisions based on real-time information, leading to enhanced performance overall. This introduction serves as a gateway to understanding not just what data scientists do, but why their work is crucial to the future of oil and gas operations.
Defining Data Science
At its core, data science combines scientific methods, processes, algorithms, and systems to extract knowledge and insights from structured or unstructured data. In the oil and gas sector, this means analyzing vast datasets related to exploration, production, and market performance. The role of a data scientist is multifaceted; these individuals utilize statistics, machine learning, and data visualization techniques to interpret data effectively.
For example, when analyzing geological data for potential drilling sites, a data scientist must first clean and organize the data, then apply statistical models to predict the likelihood of finding oil or gas. This scientific approach allows companies to reduce risks and invest in projects with the best chance for success.
Importance in Industry
The significance of data science in the oil and gas industry cannot be overstated. As the sector becomes increasingly competitive, the integration of data analytics can propel a company ahead of its competitors. Here are some key reasons why data science is vital:
- Enhanced Decision-Making: With accurate data, companies can make strategic decisions that affect drilling locations, production rates, and market engagement more effectively.
- Cost Efficiency: Leveraging data science can lead to significant reductions in operational costs by predicting failures and optimizing maintenance schedules.
- Improved Safety: Data analytics contributes to the identification of potential hazards before they become incidents, fostering safer working environments.
- Sustainability Practices: As more emphasis is placed on environmental stewardship, data science aids in monitoring and reducing the ecological impact of oil and gas extraction.
"Data is the new oil; it has to be refined to extract value."
The Data Landscape in Oil and Gas
Understanding the data landscape within the oil and gas sector is like mapping out a treasure map. It allows industry professionals to uncover valuable insights that can enhance operations, drive profitability, and mitigate risks. As oil and gas companies increasingly rely on data-driven strategies, recognizing the diverse types of data collected becomes essential. This section delves deep into the types of data gathered—focusing on geological, operational, and market data—alongside the sources that supply these crucial insights.
Types of Data Collected
Geological Data
Geological data is the bread and butter of exploration and production in the oil and gas industry. It consists of information derived from various geological sources and studies, including seismic surveys, core samples, and well logs. This kind of data is paramount for determining where to drill and how to optimize recovery rates. The key characteristic of geological data lies in its predictive capability. It allows geoscientists to construct models that can forecast the presence of hydrocarbons beneath the earth's surface.
One unique feature of geological data is its integration of multiple datasets to create a comprehensive picture of subsurface formations. However, this integration can be quite complex and labor-intensive. Data quality becomes a vital factor since any misinterpretation can lead to costly drilling decisions. Thus, while geological data is indispensable, it comes with its own set of challenges that users must navigate carefully.
Operational Data

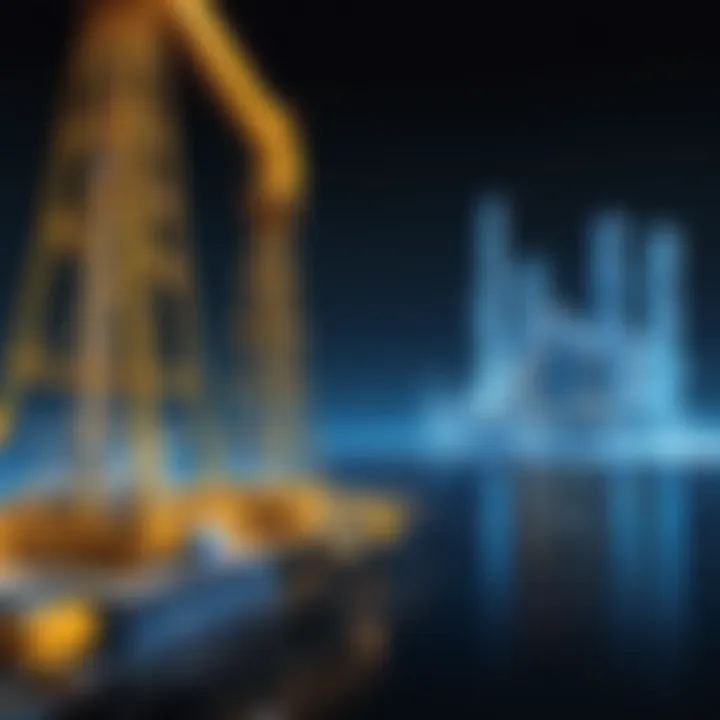
Operational data in the oil and gas sector encapsulates a wide range of information regarding day-to-day activities, production metrics, and equipment performance. This data is often collected through various monitoring systems and sensors installed in rigs, pipelines, and refineries. Operational data plays a critical role in understanding efficiency and reliability in operations. The highlight of this type of data is its real-time nature, allowing for immediate feedback and adjustments to processes.
What sets operational data apart is its focus on the intricacies of everyday workflows. This detailed insight provides a chance to pinpoint inefficiencies and enhance overall productivity. However, it can also be overwhelming. The sheer volume of data might lead to analysis paralysis, where decision-makers get bogged down by information overload. Thus, while operational data is beneficial, finding ways to effectively analyze and utilize it is essential.
Market Data
Market data consists of financial information, such as prices of crude oil, natural gas statistics, and economic indicators tied to global supply and demand. This data can influence strategic decisions, resource allocation, and business forecasting in the oil and gas industry. One key characteristic of market data is its volatility; oil prices, for example, can fluctuate based on a myriad of geopolitical factors and market forces.
A distinctive feature of market data is its capacity to inform risk management strategies. By analyzing market trends, companies can anticipate downturns and adjust production levels accordingly. Yet, its reliance on external factors can also make market data unpredictable. This unpredictability can lead to hasty decisions if not approached cautiously.
Data Sources
Sensor Networks
Sensor networks are an essential component of data collection in the oil and gas industry. These networks comprise numerous sensors placed throughout drilling sites, pipelines, and processing plants to monitor a myriad of parameters like pressure, temperature, and flow rates. The primary benefit of sensor networks is their continuous data collection capabilities, providing insights into operational efficiencies or detecting issues proactively.
One unique attribute of sensor networks is their ability to create an interconnected system where the data collected feeds into a central repository for holistic analysis. Yet, issues such as sensor malfunction or network outages can pose significant challenges, leading to data losses and increased downtime, ultimately impacting operations.
Remote Sensing Technology
Remote sensing technology employs aerial and satellite imagery to gather data from a distance, which can include land use, environmental monitoring, and potential drilling sites. A significant benefit of remote sensing is its ability to cover vast areas that would be impractical or cost-prohibitive to inspect physically. This method is valuable for initial site assessments and environmental impact studies.
However, remote sensing does have its drawbacks. The data acquired can sometimes be limited in resolution and accuracy, requiring ground-truth verification to ensure reliability in interpretations. This added step can slow down the decision-making process if it is not integrated effectively.
Transactional Databases
Transactional databases manage all transactional data from various operations, such as purchasing, sales, and inventory records. This information is crucial for maintaining operational integrity and providing a clear audit trail for financial decisions. The primary characteristic of transactional databases lies in their structured nature, enabling efficient data retrieval and processing.
However, the challenge with transactional databases is efficiency during peak operations. If the database systems are not well-optimized, they may become bottlenecks, slowing down access to real-time data. Thus, while they provide critical insights into day-to-day transactions, companies must invest in robust database management systems to leverage their full potential.
"In the world of oil and gas, data isn’t just king; it’s the entire kingdom."
As we proceed through this exploration of data science within the oil and gas sector, understanding the data landscape becomes integral to unlocking greater efficiency and profitability.
Key Roles of Data Scientists
Data scientists play a pivotal role in the oil and gas industry, bridging the gap between data and actionable insights. Their expertise allows the industry to delve deeper into the data landscape, ensuring decision-making is not only faster but also more informed. The importance of their role can't be overstated, especially as oil and gas companies look for ways to enhance efficiency, reduce costs, and better manage risks. Understanding their contributions enhances the overall appreciation of how data science is reshaping traditional workflows in this sector.
Predictive Analytics
Predictive analytics has become a cornerstone of data science applications in oil and gas. This technique employs data, statistical algorithms, and machine learning to identify the likelihood of future outcomes based on historical data. Companies leverage predictive analytics primarily for two application areas: equipment failure prediction and production optimization.
Equipment Failure Prediction
In the oil and gas sector, unplanned equipment failures can result in substantial downtime and financial loss. Data scientists utilize predictive analytics to forecast when equipment is likely to fail, allowing for proactive maintenance. A key characteristic of equipment failure prediction is its reliance on real-time data from sensors placed on machinery, transforming raw data into actionable insights. This method stands out as a beneficial choice for the industry as it minimizes costly interruptions.
The unique feature of this predictive approach is its ability to adapt and learn with time. By employing machine learning techniques, data scientists can refine models using past failure data, which can result in higher accuracy. However, a disadvantage exists when maintaining the models, as they need constant updates to remain relevant with evolving technology use. Failure to do so could lead to misleading predictions.
Production Optimization
Another critical facet of predictive analytics in the oil and gas industry is production optimization. This process involves analyzing various data sources to maximize production while minimizing resources. Here, the key characteristic of production optimization lies in its ability to simulate different scenarios, helping companies assess the best plans of action.
Production optimization shines brightly in its capacity to offer practical, data-driven solutions to enhance output. A unique feature of this methodology is the integration of various datasets, including geological and market data, which presents a more holistic view of potential production outcomes. Still, a drawback of production optimization is the intricacy involved in aligning numerous datasets. Data scientists must often untangle various sources and formats, which can consume considerable time and resources.
Data Visualization
Data visualization is another crucial role fulfilled by data scientists. In the oil and gas sector, the ability to present complex data in a digestible format is vital. Through visualization, data becomes more accessible, enabling decision-makers to grasp insights quickly and accurately. This transformation often involves the use of charts, graphs, and dashboards that visually communicate trends, forecasts, and anomalies in data. Utilizing tools like Tableau or Power BI, data scientists craft visual stories that simplify the intricacies of data analysis and open pathways for more effective discussions around strategy and operations.
Machine Learning Applications
Machine learning applications are expansive, but within the oil and gas sector, they find unique power in drilling optimization and resource identification. These applications are poised to revolutionize how companies approach challenges inherent in the exploration and extraction processes.
Drilling Optimization
Drilling optimization focuses on improving the efficacy of drilling operations. By analyzing historical drilling data and performance metrics, data scientists can develop algorithms that suggest the most efficient methods and technologies for specific drilling projects. The essence of drilling optimization is its significant impact on reducing costs and time, ensuring that companies can rapidly deliver results without compromising quality.
The appealing aspect of this optimization is its iterative nature, continuously learning from past operations to enhance future drilling strategies. However, machine learning adoption in drilling does come with challenges, particularly regarding the integration of data from various rigs and wells, which can present obstacles to achieving consistently optimal results.
Resource Identification
In the vast and complex landscape of oil and gas, resource identification is a powerful machine learning application. It involves leveraging geological data and technologies such as seismic imaging to predict the location and quality of underground resources. This capability is invaluable as it can significantly decrease the risk associated with exploration projects.
One notable feature of resource identification is its reliance on sophisticated algorithms that can analyze vast datasets from varied sources. While this brings undeniable advantages, a downside may include substantial initial investments in technology and training, which can be barriers for some companies.
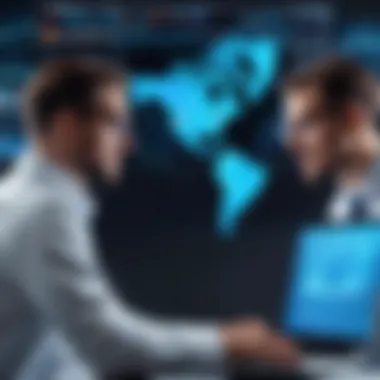
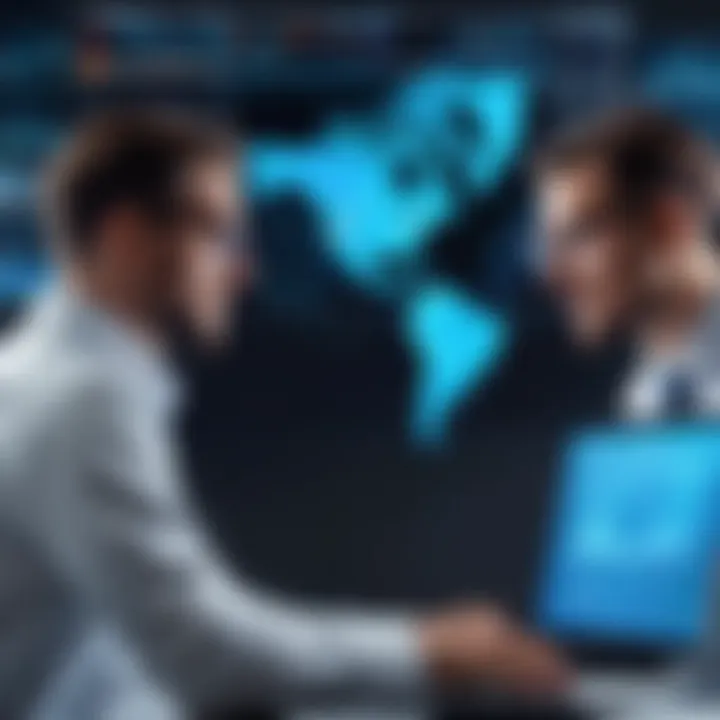
Predictive analytics, data visualization, and machine learning not only empower data scientists but also illuminate paths for decision-makers to navigate complex challenges efficiently.
In sum, the roles of data scientists in predictive analytics, data visualization, and machine learning applications form the backbone of innovations within the oil and gas industry, driving it toward greater efficiency and effectiveness.
Methodologies Used in Data Science
In the oil and gas industry, the application of data science methodologies leads to improved decision-making and operational efficiency. These methodologies allow data scientists to extract meaningful insights from raw data, enabling companies to optimize resources and enhance productivity. As organizations face stiff competition and rising costs, understanding these methodologies isn't just useful—it's vital.
Statistical Analysis Techniques
Statistical analysis techniques play a crucial role in the interpretation of data. By applying various statistical methods, data scientists can identify trends and patterns that may not be immediately evident. This can range from simple descriptive statistics, like calculating the mean and median of production rates, to more complex inferential statistics which help in predicting future outcomes based on current data.
In the oil and gas sector, statistical techniques can aid in everything from estimating reserves to predicting equipment failures. These insights can therefore lead to more informed decision-making, reducing downtime and enhancing overall operational performance. By rigorously verifying hypotheses with statistical tests, companies can avoid costly investments in false leads.
Machine Learning Algorithms
Machine learning is a subset of data science that involves algorithms learning from data to make predictions or decisions without being explicitly programmed.
Supervised Learning
Supervised learning is predicated on the use of labeled datasets to train algorithms. This means every input has a corresponding output. For example, predicting drilling success based on past performance data is an area where supervised learning shines. Its key characteristic is its reliance on these predefined labels, making it a favored choice for predictive tasks in the oil and gas industry.
The unique feature of supervised learning is that it can provide accurate predictions when there's sufficient quality data. Its main advantages include high accuracy and the ability to understand complex data relationships. However, the downside is that it requires a significant amount of labeled data, which can sometimes be difficult to source.
Unsupervised Learning
In contrast, unsupervised learning does not operate on labeled data. This methodology is employed to discover hidden patterns or intrinsic structures within the data. For instance, clustering algorithms can be utilized to segment market data for targeted strategies in exploration or production.
The appeal of unsupervised learning lies in its flexibility; it can handle various data types and doesn’t require labeled responses. The unique advantage is that it can uncover unexpected connections and trends that might aid strategic decision-making. Nonetheless, its downside is that the absence of labels makes it harder to evaluate the performance of the model.
Big Data Technologies
Big data technologies have become essential in managing the enormous volumes of data in the oil and gas industry. These technologies enable the efficient storage, processing, and analysis of vast datasets that are characteristic of exploration and production activities.
Hadoop Ecosystem
The Hadoop Ecosystem is a powerful framework that allows organizations to store and process large datasets in a distributed computing environment. One of its strong suits is its scalability, which makes it a valuable choice for companies looking to leverage big data in their operations.
What sets Hadoop apart is its ability to handle both structured and unstructured data. This characteristic is particularly advantageous in the oil and gas sector, where data may come from varied sources such as sensors, remote monitoring, and historical records. However, the complexity of setting up and managing a Hadoop cluster can be seen as a drawback for smaller organizations lacking technical expertise.
Cloud Computing Solutions
Cloud computing offers oil and gas companies flexible and scalable resources for data storage and processing needs. The on-demand accessibility of cloud solutions makes them a strategic choice for firms looking for cost-effective and efficient data management.
The unique aspect of cloud computing is its ability to provide collaborative tools and real-time data access across various locations. This facilitates better sharing of insights and enables quick response to operational changes. Nonetheless, concerns related to data security and privacy remain significant, necessitating a careful approach when opting for cloud solutions.
Challenges Facing Data Scientists
Data scientists in the oil and gas sector operate in a landscape that can be as challenging as it is rewarding. With the industry continuously evolving, data professionals face a unique set of hurdles that can significantly impact their work and the overall success of projects. Understanding these challenges is essential for grasping the broader implications of data science in this domain. Let's delve into the specific issues that stand out in this complex field.
Data Quality Issues
One of the foremost concerns data scientists encounter is the issue of data quality. In the oil and gas industry, data comes from various sources — sensors, satellite imagery, and field reports, to name a few. When data is collected, it can often be incomplete or riddled with inaccuracies.
Poor quality data not only skews analysis but can also lead to misguided decisions, resulting in lost time and resources. For instance, if geological models are based on faulty data, the implications for drilling operations can be costly and far-reaching.
To mitigate these issues, companies must invest in rigorous data cleansing processes. Data scientists play a key role in developing and maintaining standards to assess data quality, as without clean and consistent data, the reliability of predictive models is severely compromised.
Integration of Diverse Datasets
In the oil and gas industry, data comes from a plethora of sources, often formatted differently and generated under various conditions. Integrating these datasets into a unified model poses a significant challenge for data scientists. For instance, blending operational data from machinery with geological information requires advanced understanding of both the geological context and technical parameters.
This integration is crucial because it enables more comprehensive analyses. Failure to effectively combine diverse datasets can lead to silos of information where opportunities for insights are lost. Data scientists must adeptly use tools and frameworks that facilitate this integration, fostering collaboration among IT and operational teams.
Interdisciplinary Collaboration
Finally, the oil and gas sector often requires data scientists to work alongside experts from different fields — geologists, engineers, and business analysts, to name a few. This interdisciplinary collaboration is vital for translating data insights into actionable strategies. Yet, it does not always come easy.
Different disciplines bring their own jargon and perspectives, which can lead to misunderstandings or misaligned objectives. For example, while a data scientist might focus on statistical trends, an engineer might be concerned with real-world applications. Without clear communication and a shared understanding, projects can falter.
To navigate these complexities, fostering a culture of openness within teams can help. Regular meetings, cross-disciplinary workshops, and collaborative tools should be encouraged to bridge the gaps between disciplines, ensuring everyone is on the same page.
Effective collaboration unleashes creative potential, which can lead to innovative solutions in oil and gas operations.
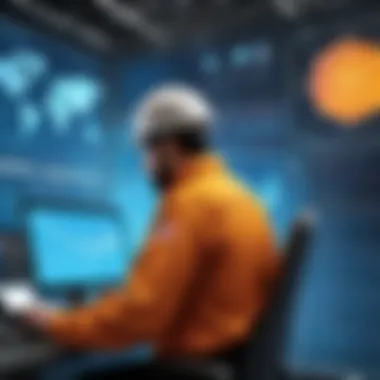
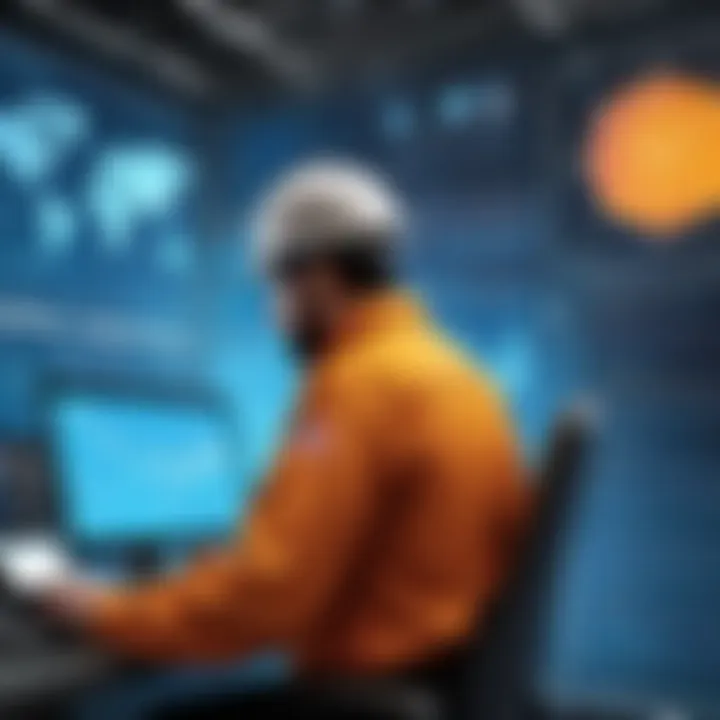
Impact on Decision-Making
Data scientists play a pivotal role in transforming raw data into actionable insights within the oil and gas industry. Their expertise not only enhances operational processes but also underpins strategic decisions. Ensuring that decision-makers can rely on robust analyses aligns with the industry's ongoing quest for efficiency and risk management. The interplay of data science and decision-making can lead to significant benefits, shaping overall business models and long-term strategies.
Enhancing Operational Efficiency
Operational efficiency is at the heart of maximizing production and minimizing costs in oil and gas operations. Data scientists employ advanced analytical techniques to dissect complex datasets, identifying patterns that could easily go unnoticed. For instance, monitoring drilling operations and analyzing equipment performance in real-time can lead to timely interventions, reducing downtime.
- Optimization of Resources: Data scientists can analyze historical performance data, understanding which equipment or drilling techniques yield the best results under specific conditions.
- Improving Maintenance Protocols: Predictive maintenance models help anticipate when equipment might fail, allowing for preemptive repairs rather than unplanned outages. This not only optimizes the operational workflow but also extends the life cycle of expensive machinery.
As a result, companies that adeptly harness their data can significantly decrease their operational costs and improve their overall productivity.
Cost Reduction Strategies
Recognizing that every penny counts in the oil and gas sector, strategies pioneered by data scientists can lead to considerable cost savings.
- Asset Management: Using analytics to manage physical assets effectively, companies can avoid unnecessary expenditures on maintenance and replacements by ensuring that resources are used wisely.
- Resource Scheduling: Data analysis allows for improved scheduling of drilling rigs and workers, ensuring that resources are allocated efficiently, thereby cutting labor costs and maximizing output.
Moreover, data-driven insights can guide firms in evaluating their supply chain strategies. When companies leverage data analytics to assess market trends, they can make informed decisions on inventory management, avoiding surplus or shortages that might incur additional costs.
Risk Management Approaches
The oil and gas industry is inherently risky, characterized by fluctuating market conditions and environmental concerns. Here, data scientists come to the forefront with their approach to risk management.
- Environmental Impact Analysis: Data modeling can predict potential environmental hazards, guiding operational adjustments that minimize ecological risks. This is increasingly vital, not just for compliance but also for maintaining corporate responsibility.
- Market Volatility Assessment: Analyzing market trends, external data, and historical performance helps companies better anticipate price swings and demand fluctuations. Through robust predictive models, firms can create contingency plans that mitigate financial risks.
"In today's dynamic environment, data-driven risk management strategies can make the difference between thriving and merely surviving."
Through these lenses, it becomes clear that the impact of data scientists on decision-making carries multifaceted benefits—enhancing efficiency, reducing costs, and managing risks in a commercially viable manner. By aligning data insights with organizational goals, oil and gas companies are better positioned to navigate the complexities of the industry.
Future Trends in Data Science
The future trends in data science are pivotal to understanding how the oil and gas industry will evolve in the coming years. As the sector increasingly adopts advanced data techniques, staying ahead of emerging trends is crucial for data scientists. This helps them not only to enhance their analytical capabilities but also to drive innovation within their organizations. The integration of cutting-edge technologies leads to improved processes, sustainability, and profitability. Thus, exploring these future trends isn't just an academic exercise; it is a necessity for professionals who are keen on maintaining a competitive edge.
Artificial Intelligence Integration
Artificial intelligence (AI) is beginning to reshape the operational landscape of the oil and gas sector. From drilling to production optimization, the incorporation of AI algorithms into workflows unlocks new levels of efficiency.
- Predictive Maintenance: AI allows for the predictive maintenance of equipment, minimizing unplanned downtimes. By analyzing sensor data, AI can foresee potential failures and suggest interventions.
- Enhanced Decision-Making: AI systems can provide data-driven insights faster than human counterparts, allowing for timely strategic decisions. This step enhances the agility of organizations in responding to market changes.
- Analytical Models: Integrating AI with existing analytical frameworks drives the development of more sophisticated models, giving organizations deeper insights into operational dynamics.
AI holds the promise of revolutionizing both decision-making and operational efficiency in the oil and gas industry.
Real-Time Data Processing
Real-time data processing represents another significant trend driving the field forward. Given that decisions in the oil and gas sector often need to be made quickly, the ability to process data in real-time is invaluable. This technology enables companies to:
- Monitor Operations Continuously: Sensor data can be processed on-the-fly, which helps operators in detecting issues as they happen, rather than after the fact. This shift can prevent small hiccups from escalating into larger problems.
- Adjust Processes Dynamically: Real-time analytics allows for immediate adjustments to drilling and production processes, enhancing resource utilization and operational response times.
- Facilitate Better Field Management: Companies can optimize field operations using live data feeds, leading to enhanced recovery rates and lower operational costs.
This trend not only boosts performance but also lowers risk, making it integral to modern strategies in the oil and gas industry.
Sustainability and Data-Driven Solutions
The sustainability requirements in today's world are more pressing than ever, and data-driven solutions are critical to meeting these demands within the oil and gas industry. With increasing scrutiny on environmental impact, organizations must adopt:
- Data Analytics for Emissions Reduction: Using data to analyze emissions at every stage of the oil production process aids in identifying areas for reduction, thereby promoting greener practices.
- Resource Optimization Solutions: Advanced analytics can help in minimizing waste and optimizing the use of non-renewable resources, ensuring that operations are not just efficient but also ethical.
- Integration of Renewable Energy Sources: Data science can assist in understanding the potential for integrating renewables into traditional oil and gas operations. For instance, companies can evaluate the feasibility of solar or wind projects alongside existing infrastructures.
In this context, the role of data scientists becomes critical. They will not only facilitate compliance with regulations but can also position their organizations as leaders in sustainable practices.
"As organizations pivot toward sustainability, data scientists will be the architects of the intelligent systems that drive energy efficiency."
Each of these trends highlights the transformative power of data science. Professionals who can leverage these advancements will be at the forefront of an industry poised for significant developments.
Finale
One of the key points to underscore is the transformation brought about by advanced methodologies. Data scientists employ predictive analytics, machine learning, and real-time data processing to tackle existing challenges. This integration not only identifies anomalies in equipment operations but also helps in optimizing production flows, ultimately leading to cost reductions and improved risk management strategies.
Recap of Key Points
- Transformative Impact: Data scientists are changing how oil and gas companies approach operations by analyzing geological, operational, and market data.
- Predictive Analytics: This plays a crucial role in anticipating equipment failures and optimizing production, thereby maximizing output while minimizing downtime.
- Interdisciplinary Collaboration: It's not just about numbers; effective data science in this sector requires collaboration across various departments, ensuring a holistic view.
- Future Innovations: The article highlights emerging trends such as the integration of AI and sustainability-focused data-driven solutions.
The Path Ahead for Data Scientists
Looking forward, the trajectory for data scientists in the oil and gas sector is promising yet challenging. The rapid pace of technological advancements necessitates continuous learning and adaptability. As the industry increasingly focuses on sustainability, data-driven insights will provide crucial support for eco-friendliness and regulatory adherence.
Furthermore, the demand for real-time data processing solutions will likely increase. This could lead to enhanced decision-making that is not only faster but also more accurate, allowing companies to adjust operations and strategies swiftly in response to evolving market conditions.
As every challenge presents an opportunity, data scientists must build on their skills related to AI and machine learning to stay ahead of the curve. Those who commit to understanding and refining these emerging technologies will find themselves at the forefront of a transformational period in the oil and gas industry.
"The future is not something we enter. The future is something we create.” – Leonard I. Sweet
In summary, the continued evolution of the data scientist's role in the oil and gas industry is a reflection of the sector’s broader shifts towards innovation. As we conclude this article, it is evident that the blend of tradition with cutting-edge technology will shape the path ahead, making skilled data scientists invaluable assets in navigating this complex landscape.