Harnessing Data Analytics for Business Success
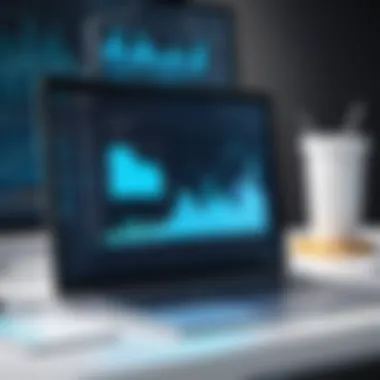
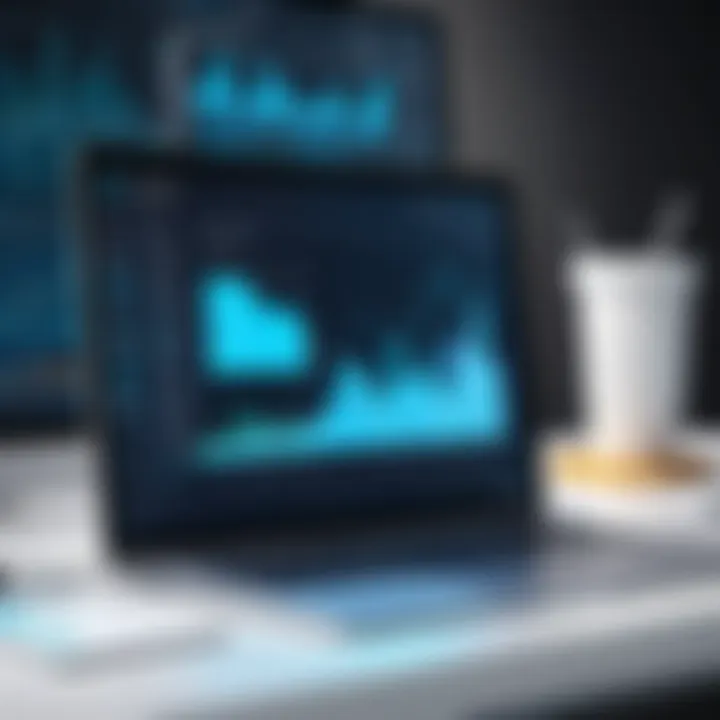
Intro
In today's dynamic business landscape, the role of data analytics cannot be overstated. It has evolved from being a niche interest to becoming a core component of strategic decision-making. Organizations across various industries are embracing data-driven practices to gain insights that significantly impact their performance. This article explores how data analytics is integrated into business practices, enhancing efficiency, informing strategies, and transforming customer experiences.
The ability to analyze vast amounts of data allows companies to identify trends, optimize operations, and create actionable strategies. As the demand for quicker and more informed decision-making grows, data analytics stands at the forefront. This article will cover the key aspects of data analytics integration, including trends, challenges, and future predictions in this rapidly changing field.
Preface to Data Analytics in Business
Understanding data analytics is critical in today’s business environment. Data analytics enables companies to turn raw data into useful insights, fostering better decision-making and operational efficiency. Organizations that effectively integrate data analytics into their practices can gain a significant competitive edge. This introduction will explore the various aspects that make data analytics essential in modern business.
Definition of Data Analytics
Data analytics refers to the systematic computational analysis of data. It involves applying statistical tools and techniques to examine, model, and transform data, resulting in actionable insights. The process typically encompasses various stages, including data collection, data cleaning, analysis, and interpretation.
Importance of Data Analytics in the Modern Economy
The role of data analytics has grown substantially in the modern economy. Organizations leverage data analytics for multiple reasons:
- Informed Decision-Making: Businesses use data analytics to understand trends, customer preferences, and market conditions. This insight helps in making informed strategic decisions.
- Operational Efficiency: By analyzing operations data, companies can identify inefficiencies and areas for improvement, thus optimizing processes.
- Customer Insights: Data analytics aids in understanding customer behavior and preferences, leading to better-targeted marketing efforts.
- Risk Management: Identifying potential risks through data analysis enables businesses to mitigate issues before they become significant problems.
"In the realm of business, the capacity to analyze data effectively translates to power in decision-making."
Companies also face challenges in adopting data analytics, such as choosing the right tools and managing data privacy concerns. Nonetheless, the benefits often outweigh the obstacles, making the integration of data analytics an essential focus for businesses hoping to thrive in competitive industries.
Types of Data Analytics
In the realm of business, data analytics serves as a critical element for driving decision-making and operational improvement. Several types of data analytics exist, each providing distinct insights and benefits. Understanding these types is crucial for organizations aiming to leverage data effectively. This section outlines the four main types: descriptive, diagnostic, predictive, and prescriptive analytics. Each type serves a unique purpose in the analytics journey and can lead to informed business strategies.
Descriptive Analytics
Descriptive analytics focuses on summarizing historical data. It provides insights based on past performance and is often the first step in the data analysis process. By using statistical techniques and data aggregation, descriptive analytics helps businesses understand what has happened in their operations.
Key components of descriptive analytics include:
- Data Collection: Businesses gather data from various sources such as sales records, customer feedback, or web traffic.
- Data Processing: The collected data is then organized and processed to generate meaningful insights.
- Reporting: Results are often displayed through reports, charts, and dashboards for easier interpretation.
Descriptive analytics answers questions like: "What were our sales last quarter?" or "How many customers visited our store last month?" This type plays a vital role in establishing benchmarks for performance tracking.
Diagnostic Analytics
Diagnostic analytics builds on descriptive analytics by analyzing data to understand the reasons behind past performance. It seeks to establish cause-and-effect relationships through deeper exploration of data. Businesses can use it to identify patterns that explain certain outcomes, helping them adjust future strategies.
The main features include:
- Root Cause Analysis: This identifies the underlying reasons for a performance gap or trend.
- Comparative Analysis: It compares different datasets to find correlations and insights.
- Drill-Down Analysis: This involves examining data at more granular levels to find more detailed insights.
Diagnostic analytics addresses questions like: "Why did sales drop during the last quarter?" Understanding these reasons allows businesses to implement corrective measures.
Predictive Analytics
Predictive analytics takes an advanced approach by applying statistical models and machine learning techniques to predict future events. It utilizes historical data along with external factors to estimate future outcomes. Organizations leverage predictive analytics to make informed decisions based on expected trends.
The process includes:
- Data Mining: Exploring large datasets to discover patterns.
- Model Building: Using algorithms to create models that can forecast future results.
- Validation: Testing the models with new data to improve accuracy.
Predictive analytics is beneficial for assessing potential risks and opportunities. For instance, it can answer questions like: "What is the likelihood of customer churn?" or "Which products are expected to sell well next season?" It enables proactive decision-making.
Prescriptive Analytics
Prescriptive analytics is the most advanced form, focusing on providing recommendations for actions. Using complex algorithms and simulations, it evaluates different scenarios and suggests optimal outcomes. This type helps businesses determine the best course of action based on predictive insights.
Its components include:
- Optimization Techniques: These identify the best possible decision amid constraints and uncertainties.
- Scenario Analysis: This examines various possible future scenarios to find the best action plan.
- Decision Analysis: This involves assessing the implications of different choices.
For example, prescriptive analytics can help answer, "What actions should we take to reduce costs?" or "How can we optimize our supply chain?" By providing actionable insights, businesses can not only anticipate changes but also strategically plan their responses.
In summary, each type of data analytics plays a crucial role in the holistic understanding of data. They contribute to informed decision-making and drive organizations towards success by exploring past performance, understanding causes, predicting future outcomes, and recommending actions.
Understanding these types and their applications allows businesses to connect their data efforts with strategic goals effectively.
Applications of Data Analytics in Business
The integration of data analytics into business practices is crucial. It enables organizations to make informed decisions and enhance overall effectiveness. By employing various applications of data analytics, businesses gain insights that help them optimize processes and strategies. These applications lead to improved customer experiences, streamlined operations, and enhanced ability to compete in the market. The relevance of data analytics extends across different sectors, making it a transformative element in contemporary business.
Customer Relationship Management
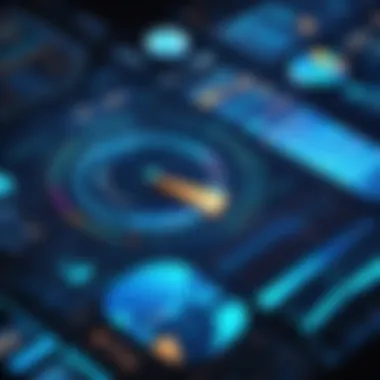
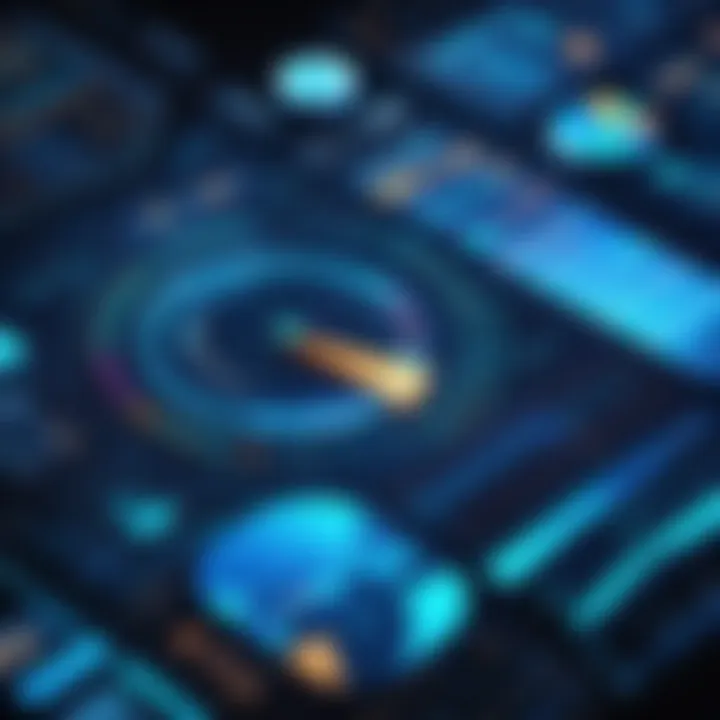
Enhancing Customer Experience
Enhancing customer experience is a vital area where data analytics plays an important role. Analyzing customer interactions and feedback leads to a better understanding of their preferences and needs. By leveraging customer data, companies can create more targeted communication strategies. A significant feature of this approach is its ability to increase customer satisfaction.
The advantages of enhancing customer experience through data analytics include improved customer loyalty and increased retention rates. However, over-reliance on data could potentially overlook personalized interactions, which are critical in service industries.
Personalization Strategies
Personalization strategies further enhance customer engagement. These strategies pinpoint individual customer behaviors, allowing businesses to customize their offerings. By using analytics to track customer habits, businesses can develop personalized marketing messages and product recommendations. This tailored approach is becoming increasingly preferred, as it resonates with consumers.
The unique feature of personalization strategies lies in their adaptability; they can evolve with customer preferences. The disadvantage could be the complexity in implementing these systems, as they require ongoing maintenance and robust data management.
Supply Chain Optimization
Inventory Management
Inventory management benefits significantly from data analytics. By analyzing patterns in product movement, companies can minimize stockouts and overstock situations. The key characteristic of effective inventory management is the ability to respond quickly to demand changes. This adaptability can save costs and optimize warehouse operations.
An advantage of using data analytics in inventory management is improved efficiency in stock levels. Nonetheless, one must consider data accuracy issues that can arise if the underlying data is flawed.
Demand Forecasting
Data analytics plays a pivotal role in demand forecasting as well. It enables organizations to predict future demand with greater accuracy. This predictive capability helps in planning resources and mitigating risks related to product shortages or excess.
The key advantage of demand forecasting is its impact on financial performance. Yet, if models are not continuously updated, they can lead to erroneous predictions, which may negatively affect operations.
Market Analysis and Strategy Development
Competitive Analysis
Competitive analysis is another crucial application of data analytics in business. By monitoring competitors' metrics, businesses can identify strengths and weaknesses in their own strategies. This practice allows companies to adjust their market positioning effectively. The strategic insights gained are vital for staying ahead.
One key characteristic of competitive analysis is its focus on data-driven decisions. The downside is that it can be resource-intensive and requires constant monitoring.
Trend Identification
Data analytics facilitates trend identification, which provides businesses with critical insights into changing market dynamics. It assists in understanding consumer preferences and new market conditions. This proactive identification of trends allows organizations to adjust their strategies timely.
The advantage of recognizing trends early is the potential for high returns on investments. However, organizations must be careful as trends can sometimes be fleeting, making it essential to discern which trends are worth pursuing.
Financial Analytics
Risk Assessment
Risk assessment is crucial in guiding financial decisions and ensuring businesses withstand market volatility. Through data analytics, organizations can identify potential risks by analyzing historical data and market trends. This proactive approach helps in safeguarding against unexpected downturns.
One notable advantage of risk assessment analytics is greater peace of mind for decision-makers. However, the predictive models used may have limitations if based on incomplete data.
Investment Analysis
Investment analysis also utilizes data analytics to evaluate the viability of potential investments. By examining market conditions and historical performance, companies can make better investment decisions. The key characteristic of investment analysis is its reliance on quantitative data for forecasting.
The benefit of this analysis includes informed decision-making that can lead to higher profitability. However, it is crucial to recognize that data alone should not be the sole factor in making investment decisions; qualitative analysis remains essential.
The Role of Big Data in Analytics
In today’s data-driven environment, the intersection of big data and analytics has emerged as a crucial element in understanding business dynamics. As organizations increasingly rely on vast amounts of information, the role of big data becomes paramount. It shapes how businesses derive insights, inform decisions, and create strategies. Big data encompasses the extensive volume, variety, and velocity of data generated every second across diverse channels. This article aims to showcase the significance of big data in analytics and its relevance to business practices.
Definition and Scope of Big Data
Big data refers to datasets that are so large or complex that traditional data processing applications are inadequate to deal with them. It includes data generated from social media interactions, online transactions, sensors, and IoT devices, among others. The primary characteristics of big data can be summarized as the three Vs:
- Volume: The sheer amount of data being generated.
- Velocity: The speed at which data is created and processed.
- Variety: The different formats and sources of data.
Understanding the definition and scope of big data is essential, as it underpins the analytics processes that businesses employ to uncover actionable insights. The increasing growth of big data highlights its profound impact on the decision-making landscape.
Challenges of Managing Big Data
While big data presents incredible opportunities, it also poses several challenges for organizations attempting to harness its potential. The following sections delve into two key challenges that frequently arise in managing big data: data quality issues and data privacy concerns.
Data Quality Issues
Data quality issues refer to the various problems that can compromise the reliability and accuracy of data used in analytics. Inaccuracies can stem from several factors, including inconsistent data entry, outdated information, and lack of standardization across sources. Ensuring that quality data is used in analytics is crucial because poor data can lead to misguided business decisions.
- Key Characteristic: The key characteristic of data quality issues is their ability to skewer analytics outcomes. Often, organizations may analyze what they think is quality data, only to find that the results do not align with actual trends.
- Unique Feature: Data quality issues are particularly challenging because they can be systematic and hard to identify. Data cleansing processes must be in place to mitigate these problems, which require time and resources but ultimately pays off in the form of accurate insights.
- Advantages/Disadvantages: Addressing data quality issues enhances reliability but can incur costs related to time and labor. In the competitive business landscape, investing in data quality can lead to robust analytics solutions that streamline operations and promote data-driven decision-making.
Data Privacy Concerns
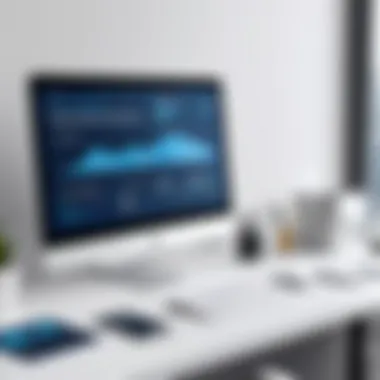
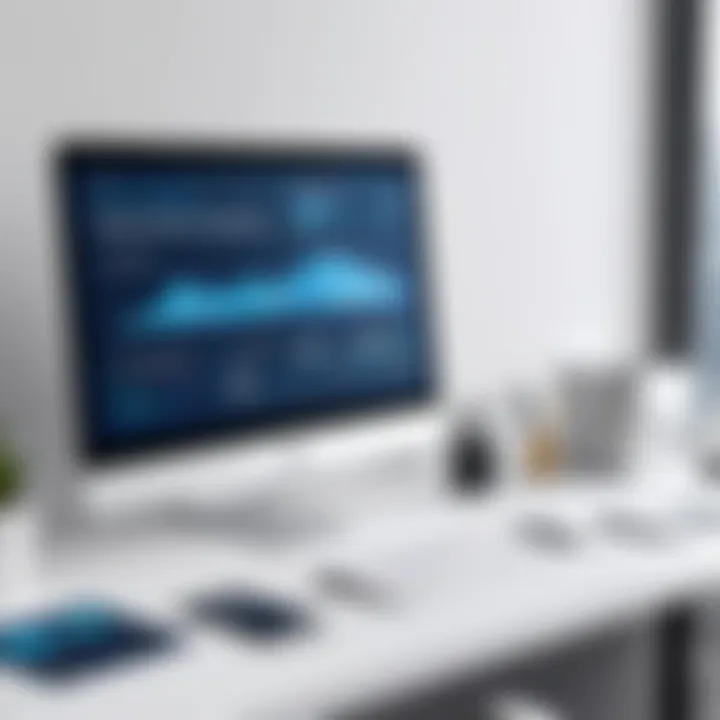
Data privacy concerns encompass the ethical and legal aspects of collecting, storing, and processing personal data. As organizations amass vast datasets, they face the responsibility of ensuring that they comply with regulations and maintain the trust of their customers. Privacy issues can impact brand reputation and legal standing.
- Key Characteristic: These concerns revolve around the potential for data misuse. Many consumers are wary of how their data is used, which complicates the relationship between businesses and their clients.
- Unique Feature: A unique attribute of data privacy concerns is the evolving nature of regulations, such as the General Data Protection Regulation (GDPR) in Europe, which mandates strict data management practices. Businesses must stay vigilant about compliance requirements to avoid hefty fines and lawsuits.
- Advantages/Disadvantages: While addressing data privacy can enhance customer trust and loyalty, it requires significant investment in technologies and protocols to securely manage data. Balancing governance with analytics capabilities is a critical challenge.
"In the age of big data, the challenge is not just the high volumes of data but ensuring its quality and safeguarding privacy."
To summarize, the integration of big data in analytics brings both significant potential and substantial challenges. Companies that understand and navigate these challenges can leverage data to inform strategic decisions effectively, cultivating a competitive edge in their respective markets.
Tools and Technologies for Data Analytics
The integration of data analytics in modern business practices hinges greatly on the tools and technologies utilized. These elements form the backbone of analytical processes, enabling companies to extract insights from complex datasets. The effectiveness of data analytics is significantly enhanced through the use of these specialized tools, which not only streamline operations but also foster informed decision-making.
Data Visualization Tools
Data visualization tools are crucial for presenting complex data in a simplified manner. These tools transform raw data into graphs, charts, and dashboards, allowing businesses to communicate findings effectively. Popular options like Tableau and Power BI provide interactive environments, enabling users to customize their views based on specific metrics. The use of such tools enhances comprehension and helps stakeholders quickly grasp key insights.
Benefits of effective data visualization include:
- Improved comprehension: Visual representations highlight trends and patterns.
- Enhanced decision-making: Stakeholders can make data-driven decisions with visualized insights.
- Increased engagement: Effective visuals attract attention and retain audience interest.
Machine Learning Algorithms
Machine learning algorithms play an integral role in predicting outcomes based on historical data. By analyzing vast datasets, these algorithms identify patterns that traditional methods may overlook. Algorithms such as regression analysis or neural networks enable businesses to forecast trends, customer preferences, and even potential risks. The predictive capabilities of machine learning are indispensable in various sectors, from finance to healthcare.
Key considerations in using machine learning include:
- Data quality: High-quality data is necessary for more accurate predictions.
- Model selection: Choosing the right algorithm greatly impacts outcomes.
- Continuous learning: Algorithms must be updated with new data to maintain efficacy.
Cloud-based Analytics Solutions
Cloud-based analytics solutions are revolutionizing how businesses manage and analyze data. Solutions like Google Cloud and Microsoft Azure enable organizations to store and process vast amounts of data effortlessly. The cloud offers scalability, allowing businesses to adjust their data needs without significant infrastructure changes.
Important benefits of cloud-based analytics include:
- Accessibility: Teams can access analytics tools from anywhere in the world.
- Cost-effectiveness: Companies save on hardware costs and maintenance fees.
- Collaboration: Cloud solutions foster collaboration, as multiple users can work on datasets simultaneously.
Cloud-based analytics solutions are shaping the future of data analytics by providing flexibility and enhancing collaboration among teams.
In summary, the selection and application of the right tools and technologies for data analytics are essential for organizations aiming to thrive in today's data-centric environment. Appropriate data visualization tools, machine learning algorithms, and cloud-based solutions collectively enhance a company's ability to harness data for strategic advantage.
Case Studies of Successful Data Analytics Implementation
Case studies serve as vital pieces of evidence that demonstrate the real-world applications and effectiveness of data analytics in business practices. They provide illustrative examples of how organizations have successfully integrated data analytics to drive growth, improve performance, and enhance customer experiences. Understanding these case studies is essential for grasping the potential benefits that businesses can realize through analytics, as they highlight best practices, results achieved, and valuable lessons learned.
Retail Sector Innovations
In the retail sector, data analytics has fundamentally transformed how businesses operate. Many retailers utilize customer purchase data to analyze shopping patterns, leading to innovative marketing strategies. For instance, Target Corporation famously gained insights through purchase histories that allowed them to identify trends and behaviors, thus enabling them to tailor promotions effectively.
Using predictive analytics, retailers forecast demand for different products. This anticipation helps manage inventory efficiently. As a result, excess stock is minimized, reducing waste and storage costs. Implementing analytics tools such as Tableau or Google Analytics also provides visualization capabilities that reveal trends and insights quickly.
Some retailers are now experimenting with real-time analytics. This technique enables them to respond instantly to consumer behavior changes, which can significantly influence sales outcomes. Overall, the integration of data analytics promotes a proactive approach to retail management, enhancing customer satisfaction and revenue growth.
Healthcare Industry Advances
The healthcare industry presents a unique landscape for data analytics application. With vast amounts of data generated from patient records, medical histories, and treatment outcomes, organizations can derive insightful analytics to improve patient care.
One notable example is Mount Sinai Health System, which implements a data-driven approach for patient management. By utilizing predictive analytics, Mount Sinai has successfully reduced hospital readmission rates. The system identifies patients at high risk through sophisticated algorithms that analyze electronic health records. Interventions can then be tailored to meet these patients' specific needs, improving overall health outcomes.
Additionally, analytics aids in streamlining operations within healthcare facilities. By analyzing resource utilization and patient flow metrics, hospitals can optimize staffing and reduce wait times. This has been critical in managing costs while maintaining high-quality patient care.
Finance Sector Transformations
In the finance sector, data analytics is instrumental in risk management and fraud detection. Leading firms like JPMorgan Chase utilize advanced analytics models to assess loan applications. By analyzing a vast range of data points, they can evaluate creditworthiness more accurately and efficiently than traditional methods.
Moreover, investment firms integrate real-time analytics to monitor market trends and develop strategies that respond to volatility. For example, Goldman Sachs uses machine learning algorithms to analyze large datasets from various markets, providing insights that inform investment decisions. This not only enhances decision-making accuracy but also offers a competitive advantage.
Fraud detection has also seen advancements through data analytics. By employing sophisticated algorithms, financial institutions can monitor transactions in real-time, identifying suspicious activities before significant losses occur. This integration of analytics safeguards assets and reinforces trust among customers.
"Data analytics is reshaping the fundamental strategies of modern businesses, with high impact across sectors. Understanding these transformations is essential for leadership today."
Challenges in Implementing Data Analytics
Implementing data analytics in business practices comes with a set of unique challenges that organizations must navigate. Understanding these challenges is crucial for effective integration. The need for data analytics has surged, but without addressing obstacles, the potential benefits remain elusive. From workforce readiness to data security, every aspect plays a role in the successful adoption of analytics.
Skill Gaps and Workforce Readiness
One significant hurdle in implementing data analytics is the skill gap within the workforce. Many employees may not possess the necessary skills to work with data effectively. Training programs must be established to bridge this gap. Companies should invest in continuous learning opportunities for their staff. This not only covers technical skills related to data analysis tools but also promotes a data-driven culture within the organization. Fostering an environment where analytical thinking is encouraged can enhance overall workforce readiness.
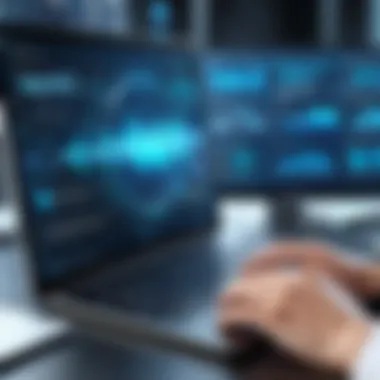
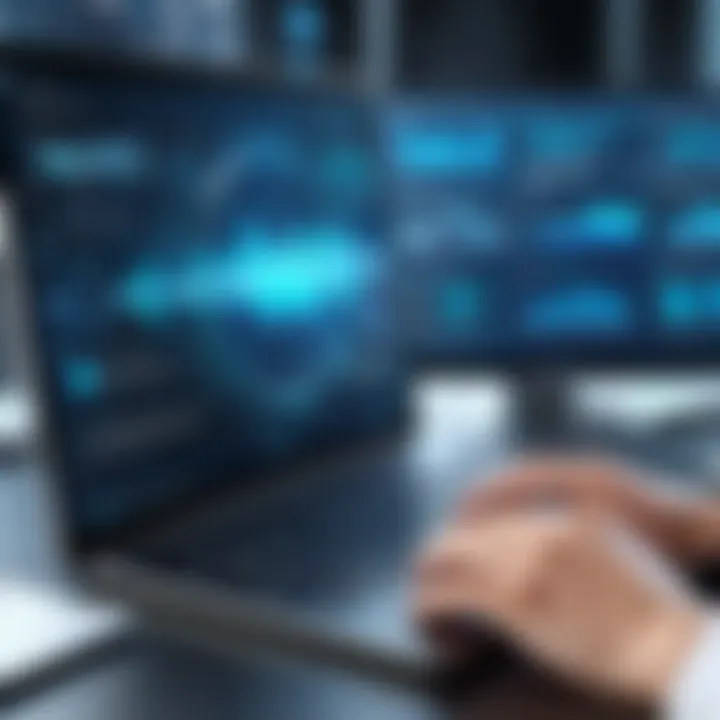
- Key strategies include:
- Providing workshops on data tools like Tableau, Power BI, or Python.
- Encouraging collaboration with data specialists.
- Developing mentorship programs focusing on data literacy.
Integration Within Existing Systems
Many organizations face challenges when integrating new data analytics solutions with existing systems. Legacy systems can be incompatible with modern analytics tools. This creates barriers in data flow and accessibility. To successfully integrate analytics, businesses need a clear strategy that includes evaluating their current technology infrastructure.
- Considerations should include:
- Conducting a thorough analysis of existing IT frameworks.
- Ensuring compatibility of analytics tools with current software.
- Gradually implementing solutions to minimize disruption.
Creating a phased approach can alleviate integration issues and ensure smoother transitions, avoiding operational headaches.
Data Security and Compliance Issues
Data security and compliance stand as major concerns for companies venturing into data analytics. With increasing regulations, such as GDPR and CCPA, organizations must be vigilant about how they manage personal data. A single breach can have dire consequences, both financially and reputationally.
Organizations should focus on establishing robust data governance frameworks. This involves putting in place policies that dictate how data is collected, stored, and processed.
- Key actions can include:
- Regular audits to identify potential vulnerabilities.
- Training staff on data security best practices.
- Implementing encryption for sensitive information.
"Data security cannot be an afterthought. It must be at the forefront of analytics strategy."
Addressing these compliance and security challenges is not just about risk mitigation. It builds trust with consumers and stakeholders, ultimately strengthening the organization's reputation. Navigating these challenges is essential to harnessing the full transformative power of data analytics.
Future Trends in Data Analytics
The landscape of data analytics is evolving rapidly, driven by technological advancements and changing business needs. Understanding these future trends is crucial for organizations aiming to maintain competitiveness in their respective sectors. Companies must recognize that data analytics is not a one-time investment but rather an ongoing commitment that can yield significant benefits if executed well. This section highlights key trends that are likely to shape the future of data analytics, including automated analytics, enhanced AI integration, and real-time analytics adoption.
The Rise of Automated Analytics
Automated analytics represents a significant shift in how businesses handle data. This approach minimizes reliance on manual data processing, which can be time-consuming and prone to errors. Automation tools allow for faster data collection and analysis, offering insights to decision-makers at unprecedented speeds.
- Benefits of automated analytics include:
- Increased efficiency in data processing.
- Reduction of human error.
- Ability to handle larger data sets seamlessly.
As companies adopt this technology, they can free up valuable resources and redirect focus toward strategic initiatives rather than mundane data tasks. Furthermore, the integration of automated reporting dashboards can offer real-time insights tailored to different team members' needs, ultimately aiding in better-informed decision-making.
Enhanced AI Integration
The incorporation of artificial intelligence into data analytics practices is transforming the field. AI technologies, particularly machine learning algorithms, can uncover patterns and trends that human analysts might overlook. Through enhanced predictive capabilities, businesses can not only analyze past events but also anticipate future developments.
- Considerations for AI implementation:
- Ethical concerns regarding data usage.
- Continuous model training to maintain accuracy.
AI-powered analytics can enable more sophisticated business intelligence tools that adapt to changes in data flows and user interactions. This results in a more agile response to market demands and consumer behavior, affording businesses a competitive edge.
Real-time Analytics Adoption
The demand for immediate insights is growing. Real-time analytics enables organizations to process data as it is generated, thus allowing for instant decision-making. Businesses across various sectors are increasingly investing in technologies that support real-time data analysis to respond quickly to market shifts.
- Advantages include:
- Improved customer service through timely responses.
- Greater operational agility to adapt strategies as needed.
Implementing real-time analytics requires robust infrastructure but can significantly enhance a company's operational capabilities. As more organizations appreciate the benefits of immediate insights, the shift towards real-time analytics is expected to grow significantly in the coming years.
"The future of data analytics lies in the ability to act quickly and efficiently, using the most advanced technological tools available."
Overall, these trends signify a transformative period for data analytics. Companies that prioritize these advancements can expect to drive innovation and achieve sustained growth without overlooking the challenges that come with each transition.
Finale
In this article, we examined the comprehensive impact of data analytics on business practices. Understanding this topic is crucial for organizations that aim to leverage data for growth and competitive advantage. The integration of analytics helps businesses identify trends, optimize processes, and make informed decisions. Each business sector, from retail to healthcare, benefits by tailoring strategies based on tangible data insights.
Summary of Key Points
Several key points have been discussed throughout this article:
- Role of Data Analytics: Data analytics is pivotal for understanding customer behavior and market trends. Organizations that utilize these insights can enhance their value propositions.
- Challenges: Despite its advantages, businesses face obstacles in implementing analytics. Issues like skill gaps and data privacy are significant hurdles that need attention.
- Tools and Technologies: Various tools, such as machine learning algorithms and data visualization software, enable organizations to analyze data effectively.
- Future Trends: Emphasis has been placed on automated analytics and the increased integration of AI, promising more streamlined processes.
"Data-driven decision-making not only informs strategy but also drives innovation across sectors."
The Future Landscape of Data Analytics
As we look towards the future, the landscape of data analytics appears promising. The rise of automated analytics tools suggests a shift towards more efficient data processing. AI will likely enhance the capacity for predictive modeling, enabling organizations to stay ahead of competitors.
Real-time analytics adoption will allow swift responses to market changes, fostering agility within businesses. Additionally, the dialogue surrounding data ethics and privacy will become increasingly important as organizations navigate data rights in the modern era. Thus, understanding these future trends will be vital for businesses seeking to remain relevant and competitive in an ever-evolving marketplace.