Understanding AI: Its Current Trends and Future Perspectives
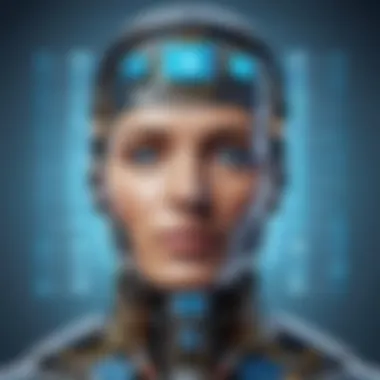
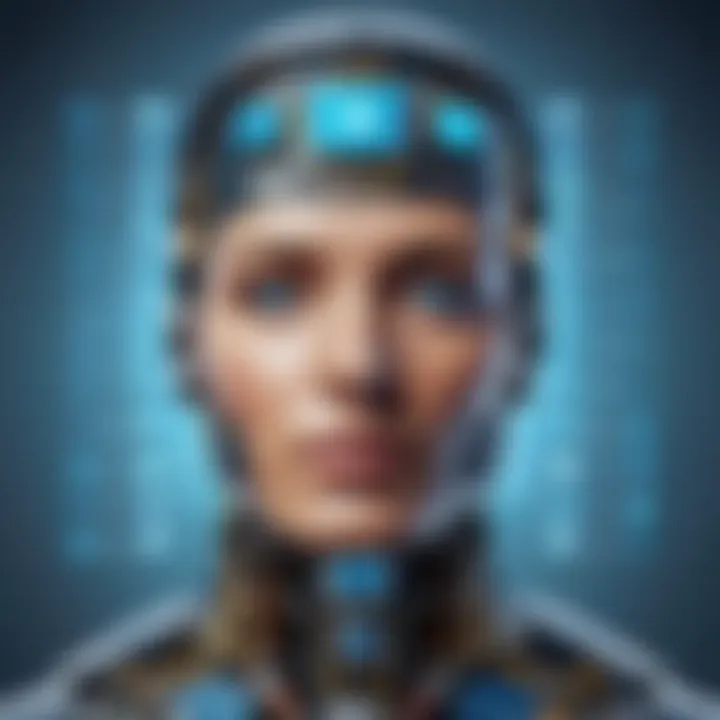
Intro
Artificial intelligence (AI) has become an unavoidable topic in today's tech-driven conversations. This vast field covers a range of technologies that allow machines to perform tasks typically requiring human intelligence. These tasks include understanding natural language, recognizing patterns, and making decisions through learning from data.
In recent years, AI has transitioned from theoretical exploration to practical integration across various industries. This shift brings significant implications that affect not just technology professionals but also the wider public. As a society, we must comprehend its evolving nature to navigate its many facets responsibly.
The aim of this article is to provide an informed perspective on AI, emphasizing its historical context, current utilizations, and potential future directions.
Tech Trend Analysis
Overview of the current trend
The current trend in AI is characterized by rapid advancements and increasing applications in real-world scenarios. Technologies such as machine learning, neural networks, and natural language processing have made significant strides. For instance, companies like OpenAI and Google are leveraging these technologies for language models, image recognition, and predictive analytics.
Implications for consumers
The influence of AI extends to consumer experiences. Online shopping, social media feeds, and even personal assistants like Siri and Alexa utilize AI algorithms to enhance user engagement. Consumers benefit through personalized recommendations, which can streamline decision-making processes. However, this also raises concerns about privacy and data security.
Future predictions and possibilities
Looking ahead, the AI landscape is likely to shift further. Predictions indicate that AI will become more integrated into daily life. Autonomous vehicles, smart home systems, and enhanced healthcare diagnostics could soon become commonplace. Yet, the ethical considerations surrounding these advancements cannot be overlooked. It is essential to strike a balance between innovation and ethical responsibility.
"AI is not just the future; it is now. How we handle its integration will shape the world." - Tech Analyst
Industry Updates
Recent developments in the tech industry
Various tech companies have announced breakthroughs in AI research. For example, NVIDIA has unveiled new hardware optimized for AI workloads. This reflects a growing recognition of AI’s role in shaping the next generation of computing. Similarly, enterprises are beginning to adopt AI solutions for enhancing operational efficiency.
Analysis of market trends
Market trends indicate an increasing investment in AI startups, which aim to provide innovative solutions across sectors such as healthcare, finance, and marketing. This surge highlights a robust belief in AI's transformative potential.
Impact on businesses and consumers
For businesses, the integration of AI can lead to improved productivity and cost savings. Consumers may experience enhanced services as AI drives better customer experiences and operational efficiencies. However, job displacement fears remain a pressing concern, necessitating discussions on the future workforce.
Epilogue
A comprehensive understanding of AI involves recognizing its rapid evolution and the profound implications it holds for society. As we analyze the present and anticipate the future, it is clear that AI is set to maintain its trajectory as a cornerstone of technological innovation. This article seeks to equip readers with the insights necessary to navigate this complex landscape responsibly.
Understanding Artificial Intelligence
Understanding Artificial Intelligence (AI) is crucial in today's technology-driven world. It represents a significant progression in how machines can process information, learn from it, and make decisions. This section aims to provide the best understanding of AI by breaking down its definition and exploring its historical context. It is not just a technical concept; it influences various aspects of daily life, industry, and economy. Knowing AI's basics helps people see its potential and limitations.
Defining Artificial Intelligence
AI can be defined as the capability of a machine to imitate intelligent human behavior. It encompasses various components, including problem-solving, learning from experiences, and adapting to new data. More specifically, AI can be categorized into two main types: narrow AI and general AI. Narrow AI focuses on performing specific tasks, such as facial recognition or language translation, while general AI refers to systems that have the ability to understand or learn any intellectual task that a human can.
This concept provides the foundational framework for understanding various technologies that utilize AI today.
"Artificial Intelligence is the simulation of human intelligence processes by machines, especially computer systems."
Historical Context of AI Development
The history of AI development is both rich and complex. Its roots can be traced back to ancient history, but formal research began in the mid-20th century. Here are some key milestones:
- 1956: The Dartmouth Conference, where John McCarthy coined the term "artificial intelligence."
- 1960s: The development of early AI programs like ELIZA, which simulated conversation.
- 1980s: Expert systems emerge, allowing for decision-making based on a set of rules.
- 1997: IBM's Deep Blue defeats chess champion Garry Kasparov, showcasing AI's capabilities.
- 2010s: The rise of machine learning and advancements in data processing capabilities drive AI into numerous applications, from healthcare to finance.
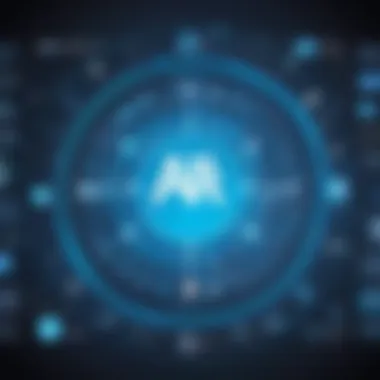
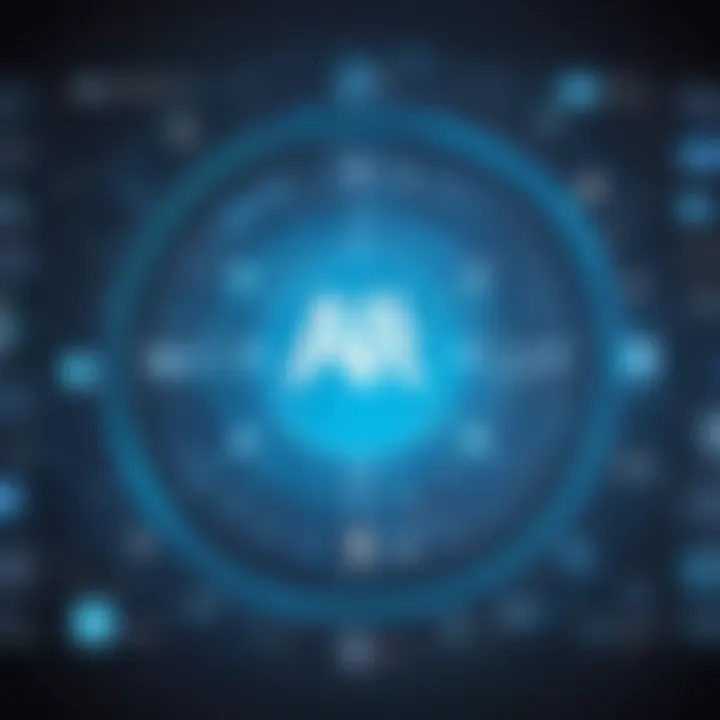
Understanding this historical context helps clarify current AI capabilities and shapes its future trajectory. It also illustrates the long-standing human desire to create machines that can think and act autonomously.
Current Applications of AI
The advent of artificial intelligence has ushered in significant changes across numerous industries. Understanding the current applications of AI offers insight into its transformative potential. AI technologies are not merely theoretical concepts; they are actively reshaping practices in various fields. The importance of this topic lies in recognizing the tangible benefits that AI brings, as well as the considerations that must be addressed as these technologies evolve.
AI in Healthcare
Artificial Intelligence is revolutionizing healthcare by enhancing diagnostics, personalizing treatment plans, and streamlining operations. AI algorithms analyze medical images faster and often more accurately than human radiologists. This capability leads to earlier detection of conditions such as cancer, subsequently improving patient outcomes. Furthermore, AI-driven platforms like IBM Watson Health assist in managing patient records and prescribing customized medications based on individual profiles and genetic information.
The potential for predictive analytics within healthcare is also noteworthy. By evaluating large data sets of patient histories, AI can predict an individual's health risks, enabling proactive interventions that could save lives. However, while implementing AI in healthcare generates immense benefits, it also brings challenges. Data privacy is particularly pressing, as patients' sensitive information must be safeguarded against breaches.
AI in Finance
The finance industry is experiencing profound changes due to AI technologies. From algorithmic trading to fraud detection, AI systems analyze patterns and behaviors far more efficiently than traditional methods. For example, banks employ AI to assess credit risk quicker than conventional approaches, allowing for faster loan approvals. Moreover, firms like PayPal use machine learning algorithms to monitor transactions in real-time, identifying potentially fraudulent activity almost instantaneously.
AI chatbots are becoming common in customer interactions, providing immediate responses to customer queries. These applications improve user experience while significantly reducing operational costs. Nonetheless, as reliance on AI increases, the potential for biased AI systems becomes a concern, particularly in lending and credit scoring. Ensuring fairness and transparency will be vital as the industry continues to evolve.
AI in Transportation
Transportation is another sector reaping the benefits of AI applications. Notable advancements include autonomous vehicles and smart traffic management systems. Companies like Tesla and Waymo are leading the charge in developing self-driving technology that aims to reduce accidents and traffic congestion. These vehicles use vast amounts of data from sensors and cameras to make real-time decisions on the road.
Moreover, AI is being integrated into public transport systems to optimize routes and schedules, ensuring efficiency and reducing wait times. However, as with healthcare and finance, ethical considerations arise. The debate on liability in cases of accidents involving autonomous vehicles poses questions that society will need to address in the near future.
AI in Customer Service
AI's role in customer service is increasingly vital. Modern businesses utilize AI-enhanced tools to improve customer interactions and satisfaction. Virtual assistants and chatbots are designed to understand customer inquiries and provide precise solutions. These applications can handle numerous requests simultaneously, which allows human agents to focus on more complex tasks. Notable platforms like Zendesk and Intercom are leading this effort by integrating AI capabilities into their services.
Additionally, sentiment analysis through AI algorithms helps businesses gauge customer feelings towards their products or services. This information can inform marketing strategies and product improvements. Nevertheless, organizations must navigate the balance between automation and maintaining the human touch in customer interactions. The challenge remains to use AI in a manner that enhances, rather than replaces, personal engagement with customers.
Machine Learning: The Core of AI
Machine learning, as a subset of artificial intelligence, is extremely relevant in the context of this article. It is essential for enabling systems to learn from data, improve accuracy, and make informed decisions. The ability of machine learning to analyze patterns within large datasets can directly impact a range of sectors including healthcare, finance, and transportation. It allows AI to work efficiently and make predictions based on historical information or learned experiences.
Types of Machine Learning
Supervised Learning
Supervised learning is a fundamental approach where models are trained using labeled data. This means that the input data is paired with the correct output, guiding the model toward accurate results. The key characteristic here is the presence of clear guidance during the learning process. This makes supervised learning a popular choice in many applications, such as image recognition and spam detection.
The unique feature of supervised learning is its ability to provide specific feedback through a loss function, which helps in minimizing errors of prediction. It accelerates the learning process, enhancing model accuracy. However, it does require substantial amounts of labeled data, which can be time-consuming and costly to compile.
Unsupervised Learning
In contrast, unsupervised learning involves training on data without explicit labels or guidance. The system explores the data to find hidden patterns or structure. This characteristic makes unsupervised learning particularly beneficial for tasks like clustering and dimensionality reduction. It is well-suited for analyzing large datasets where labeling is impractical.
A unique feature of unsupervised learning is its ability to discover novel insights without prior expectations. It can efficiently categorize information into clusters. However, the resulting patterns may not always be easily interpretable, leading to challenges in actionable insights.
Reinforcement Learning
Reinforcement learning is a distinct paradigm where an agent learns by interacting with an environment. This method uses feedback from actions taken to learn the best strategies over time. Its key characteristic is the use of rewards and penalties, guiding the agent toward optimal behavior. This makes it suitable for complex decision-making scenarios, such as game playing and robotics.
The unique feature of reinforcement learning is its focus on long-term reward optimization. It adapts through a trial-and-error process, continuously improving through experiences. However, it can be computationally intensive and require substantial time to converge on optimal solutions.
The Role of Data in AI
Data serves as the lifeblood of machine learning and AI. The quality and quantity of data directly influence the performance of AI models. With a sufficient amount of relevant data, machine learning algorithms can capture intricate patterns and deliver precise predictions, ultimately enhancing the effectiveness of AI applications.
Moreover, managing data effectively is crucial. Poor data management can lead to inaccurate conclusions, undermining the potential of AI systems. This highlights the importance of data curation and preprocessing in the AI development lifecycle.
"In the age of information, the role of data in artificial intelligence cannot be overstated. It dictates the success and applicability of AI across various domains."
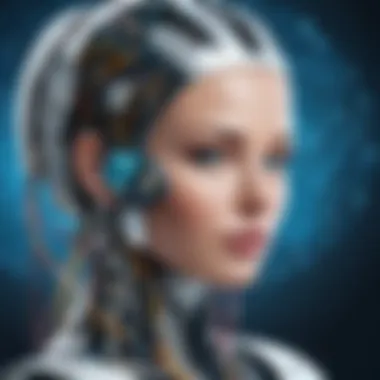
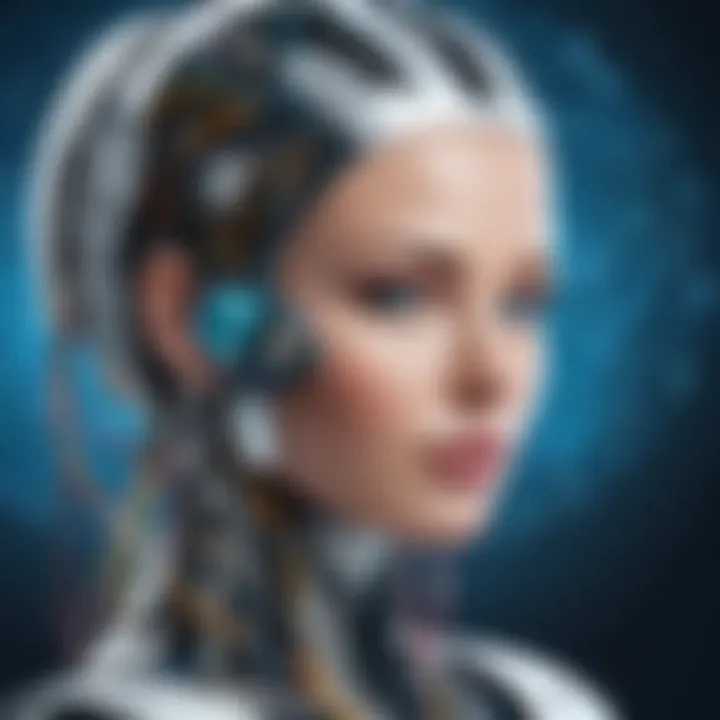
The relationship between data and machine learning is complex and forms the backbone of modern AI capabilities. Understanding this relationship enables various industries to harness AI effectively and ethically.
Challenges and Limitations of AI
Artificial Intelligence (AI) has made significant strides in recent years, transforming industries and reshaping daily interactions. However, the integration of AI into various sectors has not been without its challenges and limitations. Understanding these issues is crucial for stakeholders in this domain, as they must navigate potential pitfalls while embracing the technology.
Technical Limitations
AI systems operate on complex algorithms and models, which are susceptible to various technical limitations. One prominent issue is computational power. Many AI applications require extensive data processing, but limitations in hardware can hinder performance. For instance, deep learning algorithms may require GPU acceleration, which can be costly and not always accessible.
Moreover, the design of AI systems can lead to overfitting. This occurs when a model learns the training data too well, failing to generalize to new inputs. As a result, performance may degrade in real-world applications where data can vary significantly from training datasets. This challenge underscores the need for balance in model complexity and generalization capacity.
A relevant facet of technical limitations relates to interoperability. Many AI systems are built on proprietary technologies. This lack of standardization can impede seamless integration across platforms, creating siloed solutions that do not communicate effectively. Addressing these limitations is essential for creating robust and effective AI solutions.
Data Privacy Concerns
Data privacy is a critical concern in AI development and deployment. AI systems often rely on large datasets that may include personal data, raising questions about consent and data security. Mismanagement or breaches of sensitive information can lead to severe repercussions for individuals and organizations alike.
The General Data Protection Regulation (GDPR) in Europe sets strict guidelines on data usage, emphasizing the importance of transparency and user control. As AI technologies continue to evolve, compliance with such regulations becomes paramount. It is crucial for AI developers to incorporate privacy by design principles, ensuring that user data is safeguarded at every stage of the AI lifecycle.
Additionally, public tension regarding surveillance technology has heightened awareness of privacy concerns. There is often fear that AI will be used to monitor individuals without consent, leading to a loss of autonomy. Organizations must address these fears by adopting ethical practices and maintaining open lines of communication with users about data handling practices.
Bias in AI Systems
Bias in AI systems represents one of the most pressing challenges. AI models are only as good as the data used to train them. If the training data is flawed or unrepresentative, the AI system may perpetuate existing biases and inequalities. This is especially concerning in applications like hiring, lending, and law enforcement, where decisions can significantly affect people's lives.
For instance, facial recognition systems have been shown to exhibit bias against various demographic groups, leading to misidentification. This is a serious issue that not only undermines trust in AI technologies but can also amplify social injustices.
"Addressing bias is not only a technical problem; it requires a cultural shift within organizations to prioritize fairness and equality in AI outcomes."
To mitigate bias, organizations need to implement diverse and representative datasets alongside ongoing monitoring of AI performance. Additionally, fostering an inclusive approach during the design phase can help identify potential biases before they translate into harmful outcomes. Ensuring that teams involved in AI development are diverse can bring varied perspectives and reduce the likelihood of biased systems.
The challenges and limitations of AI are intricately linked to its successful integration into society. By addressing technical limitations, prioritizing data privacy, and combating bias, stakeholders can harness the transformative power of AI while safeguarding against its potential pitfalls.
Ethics in Artificial Intelligence
Ethics in artificial intelligence has emerged as a pivotal topic amidst the rapid evolution of this technology. As AI systems increasingly influence our daily lives, ethical considerations become imperative. Understanding the implications of AI involves scrutinizing not just technical prowess but also the moral compass guiding its development and application. Ethical discussions in AI define the principles that ensure technology serves society positively while minimizing harms.
The importance of ethics in AI lies in several critical elements:
- Accountability: Developers and organizations must take responsibility for their AI systems. Clear guidelines can determine who is accountable when AI makes decisions that affect human lives.
- Transparency: The inner workings of AI, particularly in algorithms, should be understandable. Users need access to information about how decisions are made to trust the technology.
- Fairness: AI systems must be designed to avoid bias. Ensuring that algorithms treat all individuals equitably is fundamental to building a just society.
- Privacy: With vast data collection, AI raises significant privacy concerns. Ethical considerations must prioritize user consent and data protection.
These elements showcase the balance between innovation and ethical responsibility, emphasizing that technological progress cannot disregard societal values.
Defining Ethical AI
Defining "ethical AI" entails establishing frameworks that govern the development and use of artificial intelligence in ways that align with societal norms and values. Such definitions often encompass fundamental principles like fairness, accountability, and transparency, alongside obligations to minimize harm and promote benefits for all stakeholders. Ethical AI necessitates a commitment from all actors involved, from researchers to policymakers, to prioritize human wellbeing over mere technological advancement.
An example of integrating ethics into AI is the development of ethical guidelines by organizations like the European Commission. These guidelines stress the importance of human oversight and the impact of AI on societal values.
Regulatory Frameworks
Regulatory frameworks for AI are critical in guiding the ethical deployment of technology. As AI penetrates various sectors, clear regulations can ensure that AI technologies are used responsibly. Governments and international bodies are beginning to create regulations that focus on the outlined ethical principles.
For instance, the GDPR in Europe sets strict guidelines on data protection and privacy. It influences how AI handles personal data, emphasizing consent and transparency. As AI matures, these frameworks are evolving to address emerging technologies, including machine learning systems and autonomous agents.
Some notable regulatory approaches include:
- Mandatory AI Ethics Training: Requiring developers to undergo training in ethics can help them understand the societal implications of AI systems.
- Audit Requirements: Implementing regular audits of AI systems can ensure compliance with ethical standards and provide accountability.
- Public Engagement: Involving communities in discussions regarding the use of AI can help tailor regulations to meet societal needs and concerns.
Public Perception of AI Ethics
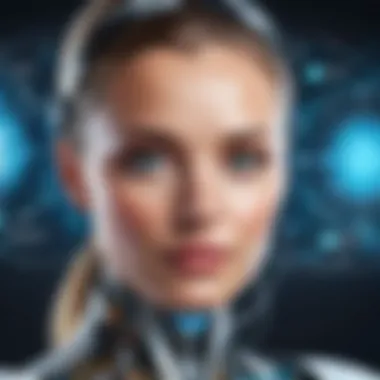
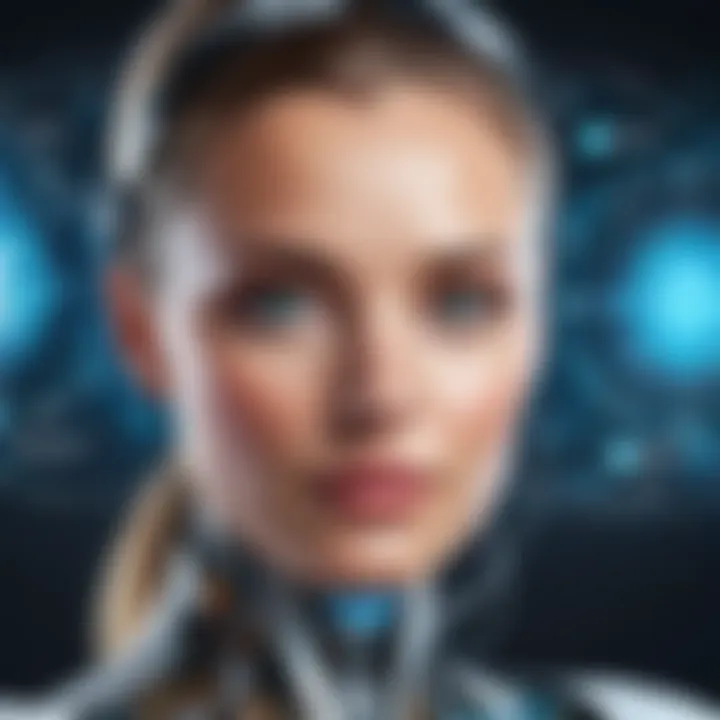
Public perception significantly impacts the adoption and trust in AI technologies. As AI's footprint grows, awareness and understanding of ethical implications play a critical role in shaping opinions. Many individuals express concerns about how AI might lead to bias, invade privacy, or diminish human agency.
Surveys often indicate that while people appreciate the benefits of AI, there remains skepticism about fairness and ethical use. This duality prompts a need for enhanced communication strategies to educate the public about the safeguards in place.
Some findings on public perception include:
- Concerns over Job Displacement: Many fear that AI will take over jobs, creating economic instability.
- Trust Issues: A lack of transparency in how AI operates can breed distrust.
- Desire for Regulations: A significant portion of the public supports stricter regulations to ensure ethical AI use.
"AI ethics is not just about preventing harm but also about fostering trust and safeguarding human dignity in the digital age."
Engagement with the public through transparency and education can help alleviate fears and build trust in AI technologies.
The Future of Artificial Intelligence
The importance of examining the future of artificial intelligence lies in its profound implications for society, industry, and individual lives. AI is not static; it evolves rapidly, fueled by advancements in technology, increasing data availability, and emerging paradigms. Understanding its future trajectory can guide stakeholders in making informed decisions and adapting to inevitable changes.
One critical aspect is the potential for increased efficiency across multiple sectors. As AI systems become more sophisticated, they will improve decision-making processes, optimize operations, and enhance user experiences. On the other hand, ethical concerns and regulatory challenges will persist. Evaluating the future landscape of AI allows us to foresee these obstacles and implement necessary measures to mitigate risks.
Predictions for AI Advancements
Predictions for AI advancements suggest an era where AI systems become integral to daily operations and personal lives. Experts forecast that by 2030, AI could contribute up to $15.7 trillion to the global economy. This number is indicative of the transformative shift expected in various sectors. Key areas of advancement include:
- Enhanced natural language processing
- More accurate computer vision capabilities
- Increased automation in routine tasks
- Development of general AI, though this remains a debated subject.
Among these, improved natural language processing will likely redefine human-AI interactions, making them more seamless and intuitive. As computers better understand context and emotion, applications will emerge that enhance communication, especially in customer service and mental health.
AI and Employment Trends
The relationship between AI and employment is complex. While AI systems will automate numerous tasks, they will also create new job categories that demand high levels of creativity, problem-solving, and emotional intelligence. Some estimates speculate that AI might displace 75 million jobs by 2022 but could create 133 million new roles that focus on uniquely human skills.
Concerns will continue about redundancies in industries such as manufacturing, retail, and transport. Organizations will need to prioritize retraining and upskilling their workforce to address the skills gap. Public and private sectors must work collaboratively to form training programs that prepare individuals for the changing job landscape.
The Intersection of AI and Other Technologies
The future of AI will not exist in isolation. Its intersection with technologies such as blockchain, Internet of Things (IoT), and quantum computing will produce novel applications and solutions.
- Blockchain and AI: Combining blockchain with AI can enhance data security and ensure traceability and accountability in AI systems.
- IoT and AI: The synergy between IoT devices generating vast data and AI algorithms analyzing these data will lead to smarter cities, automated homes, and improved resource management.
- Quantum computing and AI: Quantum computers can process complex calculations rapidly, opening new possibilities for AI applications that require advanced analytical capabilities.
"By harnessing the power of AI with complementary technologies, we can potentially unlock new solutions that are currently unimaginable."
Finale: Navigating the AI Landscape
Understanding the current state and future potential of artificial intelligence is essential for various stakeholders. This conclusion aims to summarize the outcomes of this exploration while highlighting the importance of navigating the AI landscape with careful consideration.
The impact of AI is vast and multifaceted. From revolutionizing healthcare to reshaping financial markets, the applications of AI technologies are extensive. As businesses increasingly integrate AI into their operations, it is crucial to assess the benefits and challenges involved.
Summarizing the Impact of AI
Artificial intelligence has already begun to transform multiple sectors in significant ways. Key impacts include:
- Efficiency Improvements: AI enables systems to analyze large datasets with speed and accuracy, exceeding human capabilities.
- Innovations in Product Development: AI tools assist in designing better products faster, which gives companies a competitive edge.
- Customer Insights: Organizations leverage AI to analyze consumer behavior, enabling personalized marketing strategies and enhanced customer service experiences.
While the benefits are clear, challenges also accompany these advancements. Concerns around data privacy, bias in AI algorithms, and ethical implications are prominent. Addressing these challenges is essential for creating a responsible AI environment that fosters trust among users.
Future Considerations for Stakeholders
As AI continues to evolve, stakeholders must remain vigilant about its future trajectory. Several considerations need attention:
- Continuous Learning: Companies and individuals should embrace lifelong learning to keep pace with rapid developments in AI technology. Staying updated on emerging trends can provide a competitive advantage.
- Ethical Responsibility: Organizations must take proactive steps to mitigate bias and ensure transparent AI practices. This responsibility aligns with public expectations for ethically sound technology.
- Collaborative Efforts: Collaboration among governments, industry leaders, and academic institutions is crucial for creating effective regulatory frameworks. Such partnerships can enable a well-balanced approach to AI deployment.
- Public Perception: Mraining public perceptions about AI's implications is vital. Misinformation can lead to fear or resistance towards AI technologies, hindering progress. Engaging with communities through education initiatives can improve understanding and acceptance.
In summary, navigating the AI landscape requires careful assessment of its impact, proactive measures to address challenges, and a focus on ethical considerations. Stakeholders must work collaboratively to harness AI's potential responsibly and create a future that benefits society as a whole.
"Understanding and addressing the challenges of AI will determine how effectively society can harness its benefits."
For further reading on AI's implications and resources, visit the articles on Wikipedia and Britannica.
Ultimately, by fostering a culture of ethical AI deployment and encouraging open dialogue, stakeholders can influence a landscape that values innovation while prioritizing social responsibility.
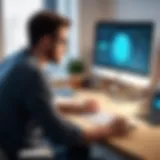
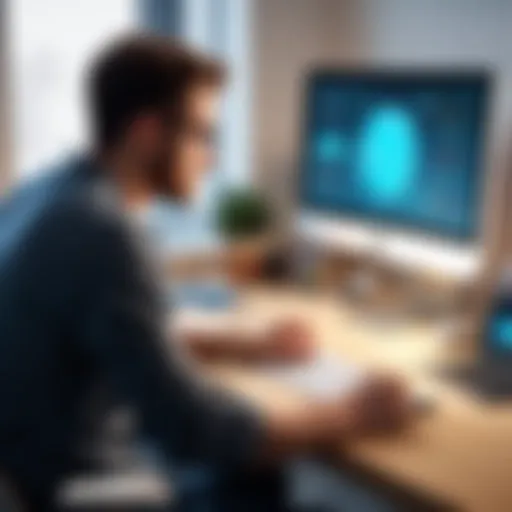