Big Data Analysis: Practical Applications and Insights
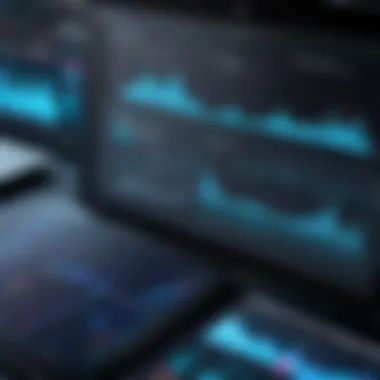
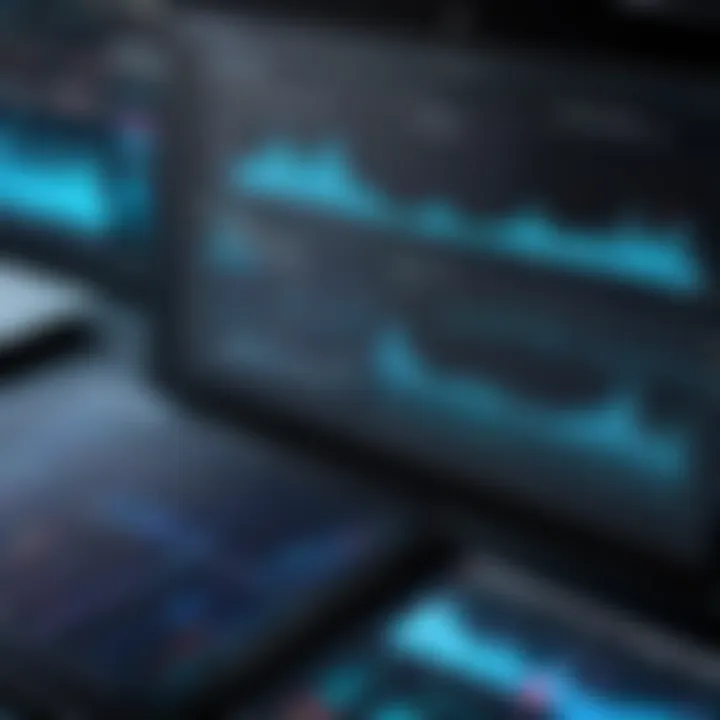
Intro
In the expanding landscape of technology, big data analysis has emerged as a critical component for businesses aiming to leverage vast amounts of information. This article elucidates how various industries utilize big data, showcasing practical examples and methodologies that elucidate its significance.
By exploring several case studies, we seek to uncover the immense potential big data holds in decision-making, optimizing operations, and guiding strategic initiatives. Understanding these facets is essential for tech enthusiasts, industry professionals, and anyone intrigued by the ever-evolving tech environment.
Tech Trend Analysis
Overview of the Current Trend
Currently, industries across the globe are increasingly relying on big data analysis to enhance their decisions and processes. Organizations such as Amazon and Google have paved the way by utilizing extensive data to predict consumer behavior and personalize their services. The integration of artificial intelligence and machine learning further amplifies these capabilities, making it feasible to analyze and interpret large datasets swiftly.
Implications for Consumers
For consumers, the implications of big data analysis are significant. Personalized marketing strategies tailored to individual preferences are one immediate impact. Businesses can now recognize trends in consumer behavior, shaping product development and marketing approaches accordingly. Furthermore, advancements in recommendation systems enable consumers to discover products that are more suited to their needs, enhancing their overall experience.
Future Predictions and Possibilities
Looking ahead, the role of big data is expected to expand even further. Emerging trends such as real-time analytics and the growing importance of data privacy will shape the future of big data analysis. Companies that adapt to these trends will likely have a competitive edge. The use of predictive analytics will become more prevalent, allowing businesses to foresee market shifts and adjust their strategies proactively.
Industry Updates
Recent Developments in the Tech Industry
Recent advancements include improvements in data warehousing solutions and cloud computing. These innovations facilitate the storage and processing of big data. For instance, platforms like Google Cloud and AWS have gained traction, providing scalable solutions for organizations.
Analysis of Market Trends
In the market, we observe a shift toward data democratization, allowing non-technical staff to extract insights from data. Tools designed for users without a technical background are on the rise, promoting wider engagement with data analysis within companies.
Impact on Businesses and Consumers
The impact of these developments cannot be understated. Businesses are able to operate with greater agility, responding to real-time data insights. For consumers, this translates to more refined products and services, ultimately improving satisfaction levels and engagement.
"Big data is not just about the data itself; it's about using that data effectively."
The ongoing evolution of big data analysis highlights its critical relevance across industries. As we delve deeper into practical applications and real-world examples, it will become clear how big data not only informs choices but drives innovations.
Prelims to Big Data Analysis
Big data analysis is a vital domain that assists organizations and individuals in understanding large volumes of data. This section aims to establish a foundation for comprehending the array of methodologies and applications pertinent to big data. As industries increasingly rely on data-driven decisions, understanding the intricacies of big data analysis becomes paramount. The benefits include enhanced decision-making, increased operational efficiencies, and improved customer experiences.
Definition of Big Data
Big data refers to datasets that are so large and complex that traditional data processing software cannot manage them efficiently. The characteristics of big data are often summarized by the three Vs: Volume, Velocity, and Variety.
- Volume denotes the massive amount of data generated every second, from social media to sensor outputs.
- Velocity speaks to the speed at which these data are generated and processed, necessitating real-time analysis.
- Variety refers to the diverse formats and types of data, including structured, semi-structured, and unstructured data.
In essence, big data encapsulates more than just the size of the datasets. It entails sophisticated methodologies for collection, analysis, and interpretation, ultimately transforming raw data into meaningful insights.
Importance in Today's World
The significance of big data analysis in today’s context cannot be understated. Businesses can harness data to gain insights that influence their strategic planning. For example, in retail, data analysis helps in understanding consumer behaviors, preferences, and trends. This leads to better inventory management and targeted marketing efforts.
Moreover, sectors like healthcare benefit greatly from big data. Predictive health analytics assists in anticipating patient needs and outcomes, ensuring resources are allocated efficiently. The financial services industry also utilizes big data to combat fraud, assess risk, and conduct real-time transaction monitoring.
"Data is the new oil. It’s valuable, but if unrefined it cannot really be used."
To summarize, the growing importance of big data analysis is evident across various sectors, driving efficiency and innovation. In the following sections, we will delve into the process of big data analysis, highlighting practical examples and insights that demonstrate its impactful role in today's world.
The Process of Big Data Analysis
The process of big data analysis is crucial for extracting insights and value from large volumes of data. It encompasses various stages, from collecting data to interpreting the results. Each stage requires careful attention to detail to ensure that the data analyzed is clean and relevant. Without proper methods, analysis results can be misleading or inaccurate. Understanding the nuances of this process allows organizations to make informed decisions that can lead to operational efficiencies and improved strategies.
Data Collection Techniques
In any big data analysis, data collection serves as the foundational step. There are different methods available to gather data, each with its distinct characteristics and uses.
Surveys and Questionnaires
Surveys and questionnaires are common tools for gathering data directly from individuals. They allow for targeted data collection that responds to specific research questions. One key characteristic of surveys is their ability to reach a wide audience quickly. This makes them a beneficial choice for collecting insights in fields like market research or customer satisfaction.
The uniqueness of surveys lies in their adaptability. They can be structured, semi-structured, or unstructured, catering to different types of information collection. However, the disadvantage is that surveys rely heavily on participant honesty and comprehension. Any misunderstanding or bias can distort the results.
Web Scraping
Web scraping refers to the automated collection of data from websites. It has become increasingly popular for its efficiency in obtaining vast amounts of data quickly across different platforms. The primary characteristic of web scraping is that it can harvest information that is publicly available, which can be invaluable for businesses looking to analyze competitor strategies or market trends.
One unique trait of web scraping is its capability to collect real-time data, making it suitable for time-sensitive projects. Yet, it may not always comply with legal guidelines, leading to ethical considerations. Additionally, the data quality can vary significantly because not all online sources are credible.
Sensor Data Generation
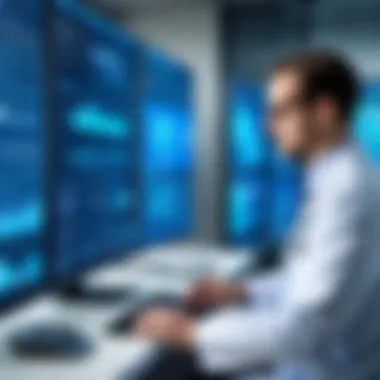
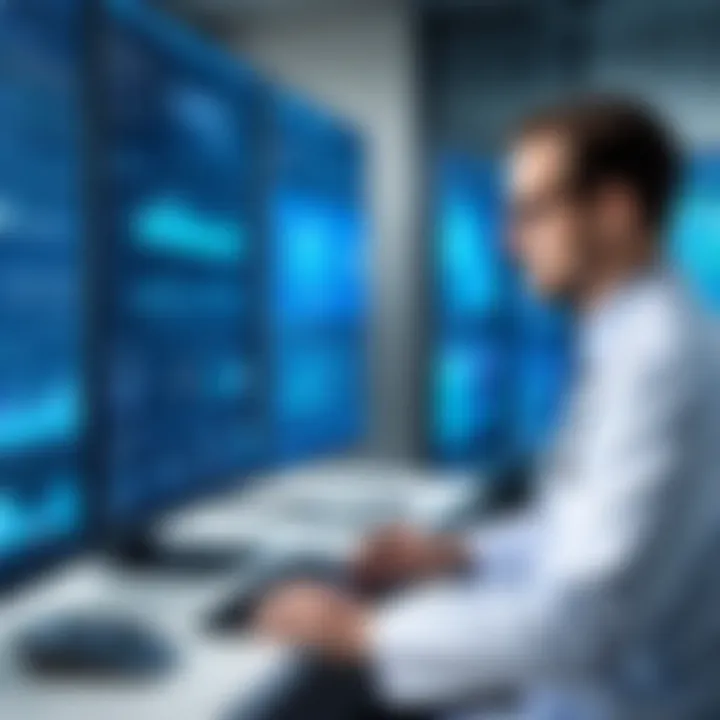
Sensor data generation involves collecting information through electronic sensors that monitor environments or systems. This technique is increasingly used in sectors like manufacturing, transportation, and healthcare. The key characteristic of sensor data is its ability to provide real-time insights based on physical interactions, enabling proactive decision-making.
A unique feature of sensor data generation is its high accuracy and granularity, which makes it suitable for detailed analytics. However, the disadvantages include the need for significant infrastructure investment and potential issues related to data privacy.
Data Processing and Cleaning
After data collection, the next step is processing and cleaning the data. This stage is essential for ensuring the accuracy and relevance of the subsequent analysis.
Eliminating Noise
Eliminating noise from data refers to the process of removing irrelevant or erroneous information that can distort analysis results. This aspect is crucial because noise can significantly influence outcomes. The key aspect of noise reduction is its potential to enhance clarity and focus on the relevant data.
The unique feature of this process is that it can involve various techniques, like filtering or aggregation. The downside is that excessive noise reduction can lead to loss of valuable data, so a balance must be maintained.
Normalization Processes
Normalization involves adjusting the data to ensure uniformity and comparability across datasets. Its importance lies in minimizing discrepancies that arise from varying scales or units of measurement. This makes the data easier to analyze and interpret. A key characteristic of normalization is its ability to create a common ground for disparate data sources.
Normalization can enhance the quality of insights drawn from the analysis. However, the process can be time-consuming and may require advanced understanding of statistical methods.
Handling Missing Values
Handling missing values is a crucial aspect of data cleaning. It addresses gaps in data that can occur due to various reasons, such as non-responses in surveys. The core contribution of resolving missing values lies in preventing skewed analysis. A significant characteristic of this task is the implementation of various techniques, such as imputation or exclusion.
Unique to this aspect is that it can involve complex statistical approaches. However, not addressing missing values may lead to biased results and poor decision-making.
Data Analysis Methods
Analyzing the collected and processed data is where insights begin to materialize. Different methods offer diverse ways of interpreting data.
Descriptive Analytics
Descriptive analytics focuses on summarizing past data to identify patterns and trends. This method is characterized by its use of historical information, making it a vital initial step in understanding data. Its role is fundamental for providing context, which then informs future analysis.
The unique feature of descriptive analytics is its reliance on visualization techniques, which can enhance comprehension. However, it does not predict future outcomes, limiting its use to descriptive purposes.
Predictive Analytics
Predictive analytics takes a step further by using historical data to forecast future events. Its key characteristic is its ability to employ statistical models and machine learning techniques to predict trends. This approach is beneficial for organizations that want to anticipate market changes.
The unique aspect of predictive analytics lies in how it can provide actionable insights for strategic planning. Nonetheless, predictions depend heavily on the quality of historical data, which poses a risk if the data has biases.
Prescriptive Analytics
Finally, prescriptive analytics guides decision-making by suggesting actions based on data analysis. The primary characteristic of this method is its ability to recommend optimal choices. It represents the culmination of descriptive and predictive analytics, providing a comprehensive view of what actions should be taken.
Its uniqueness lies in the use of algorithms to evaluate various scenarios, but the complexity can make it challenging to implement effectively, requiring sophisticated tools.
Case Studies in Big Data Analysis
Case studies in big data analysis illustrate how organizations across different industries leverage data to enhance decision-making and optimize performance. These real-world examples provide crucial insights that underline the practical implications of big data analytics. They showcase tangible results and benefits that can be achieved through the effective use of data. Furthermore, case studies reveal unique applications and challenges that organizations face while implementing big data solutions, serving as a reference for others seeking to harness such technologies.
Retail Sector Example
Consumer Behavior Insights
Understanding consumer behavior is pivotal in shaping marketing strategies. In data analysis, it involves examining patterns in purchasing habits and preferences. Retailers utilize this analysis to personalize the shopping experience, increasing customer satisfaction and loyalty. The key characteristic of consumer behavior insights is their capacity to predict future buying decisions based on historical data. This makes it a popular choice for companies seeking a competitive advantage in the market. However, the unique feature of this approach is the need for access to extensive datasets, which might raise concerns related to data privacy and compliance.
Inventory Management Optimization
Inventory management is vital for maintaining operational efficiency. By analyzing big data, businesses can predict stock levels and optimize supply chains. The critical element of inventory management optimization is its ability to reduce costs while meeting customer demand effectively. This makes it a beneficial approach for retailers facing supply chain pressures and fluctuating consumer demands. A unique advantage is the integration of real-time data analysis, which helps in making informed decisions. Nevertheless, it can be challenging to implement due to the complexity of existing systems.
Personalized Marketing Strategies
Personalized marketing strategies focus on tailoring advertisements to individual consumer preferences. Insights gleaned from big data allow retailers to create targeted campaigns that resonate with customers on a personal level. The key characteristic of personalized marketing is its effectiveness in improving engagement rates. This is why many businesses consider it a favorable approach. A standout feature of personalized marketing strategies is their capacity to enhance customer experience, leading to increased sales. However, the challenge lies in the accurate interpretation of data to avoid miscommunication with consumers.
Healthcare Sector Example
Predictive Health Analytics
Predictive health analytics involves analyzing trends to forecast patient outcomes. This is crucial in managing healthcare resources efficiently. A significant benefit of this approach is the reduction of healthcare costs through early intervention. The key characteristic here is the predictive capacity to identify at-risk patients based on data patterns. This makes predictive health analytics a compelling strategy in contemporary healthcare systems. However, unique challenges exist in acquiring clean data and ensuring interoperability among systems.
Improving Patient Outcomes
Improving patient outcomes relies heavily on data-driven insights from healthcare providers. This strategy enhances treatment protocols and enhances patient care pathways. The vital aspect is patient safety, which can be significantly elevated through informed decisions backed by data. This makes improving patient outcomes a necessary focus for healthcare organizations. A unique feature of this approach is multidisciplinary integration of data across departments. Nonetheless, the implementation might be constrained by varying data standards and practices.
Resource Allocation Strategies
Resource allocation in healthcare is about optimizing the use of available resources to meet patient needs effectively. Big data facilitates informed decisions regarding staff allocation, equipment usage, and facility management. The key characteristic is its focus on efficiency, enabling better service delivery. It is a beneficial choice for healthcare institutions grappling with resource constraints. A notable feature is the analytical ability to simulate different scenarios for effective planning. However, resource allocation can be complicated by rapidly changing healthcare demands and legislative requirements.
Financial Services Example
Fraud Detection Mechanisms
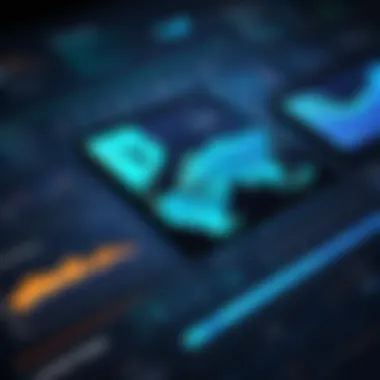
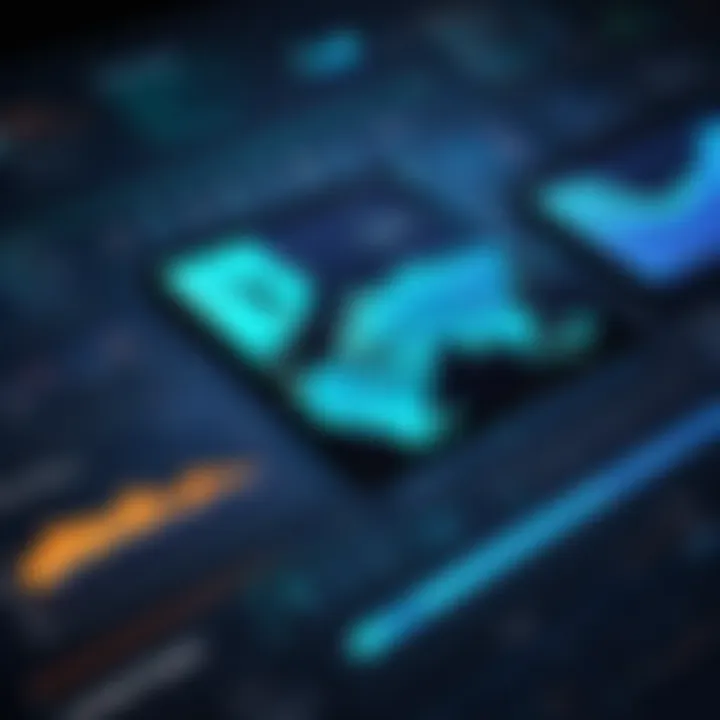
Fraud detection mechanisms in finance are essential for ensuring the integrity of transactions. Utilizing big data allows for real-time analysis of transaction patterns to identify fraudulent activities. The main advantage is the speed at which anomalies can be discovered, protecting both customers and institutions. This characteristic makes fraud detection a critical area of focus for financial institutions. The unique feature is the use of advanced algorithms to continuously learn and adapt to new fraudulent schemes. However, the challenge lies in managing false positives and ensuring customer trust.
Customer Risk Profiling
Customer risk profiling involves assessing the risk levels associated with clients. This helps financial institutions tailor services and mitigate potential losses. The key aspect here is the ability to categorize clients based on their financial behaviors and histories. This makes risk profiling a popular choice for banks and investment firms. A unique feature is the integration of external data sources, which can enhance the accuracy of risk assessments. Nonetheless, privacy concerns regarding personal data usage can pose significant challenges in this field.
Real-time Transaction Processing
Real-time transaction processing is crucial in maintaining customer satisfaction and trust in financial services. By analyzing data instantaneously, institutions can provide immediate responses to client requests. The primary benefit is enhanced user experience, leading to increased customer retention. A key characteristic of this approach is its reliance on advanced computing platforms capable of handling large data flows. However, systems must be robust enough to handle spikes in transaction volumes without compromising speed or safety.
Transportation Example
Route Optimization
Route optimization focuses on finding the most efficient paths for vehicles, which can lead to significant cost savings and improved service delivery. With big data analysis, transportation companies can assess traffic patterns and predict delays. The key characteristic here is the efficiency gained through data-driven decision-making. This makes route optimization an indispensable strategy for logistics companies. A unique feature is the incorporation of real-time data, enabling dynamic rerouting. Challenges might include the integration of diverse data sources and technology infrastructure requirements.
Traffic Pattern Analysis
Traffic pattern analysis involves studying movement trends in urban areas to manage congestion. By utilizing big data, city planners can design better traffic flows and enhance public transport systems. The main advantage is improved urban mobility and safety. The key characteristic of this analysis is its predictive capability, often leading to informed infrastructure development. This makes traffic pattern analysis a relevant focus area in urban planning. However, the complexity of urban environments may complicate data collection and analysis.
Predictive Maintenance of Vehicles
Predictive maintenance utilizes big data to anticipate vehicle failures before they occur. This approach prolongs vehicle life and reduces operational downtime. The critical aspect is safety, which can be significantly enhanced through proactive maintenance strategies. It is a beneficial choice for fleet operators aiming to minimize costs. A unique feature is the analysis of sensor data, allowing for detailed assessments of vehicle health. However, investment in the necessary technology and training can be demanding for organizations.
Tools and Technologies for Big Data Analysis
The realm of big data analysis relies heavily on suitable tools and technologies. These tools enhance the ability to collect, store, process, and analyze vast quantities of data generated daily. Selecting the right tools can significantly affect the efficiency and effectiveness of analysis operations. Common considerations include ease of use, scalability, cost, and integration capabilities with existing systems.
Big Data Frameworks
Hadoop Ecosystem
Hadoop Ecosystem has emerged as a cornerstone in big data processing. Its ability to handle large datasets efficiently is its key characteristic. The ecosystem encompasses various tools that support data storage, processing, and analysis. Hadoop's distributed file system allows data to be stored across multiple servers. This feature adds resilience and ensures data is accessible even in case of hardware failure.
One of the unique advantages of using Hadoop is its scalability. This framework can start with small amounts of data and expand as needs grow. However, its complexity can be a drawback for newcomers to big data analysis.
Spark Framework
Spark Framework is notable for its speed and ease of use in processing big data. Unlike Hadoop's batch processing, Spark enables real-time data processing, which is advantageous for applications requiring immediate insights. Spark's in-memory computing capability accelerates data computation significantly.
This immediacy makes Spark a popular choice among organizations looking for quick analysis. Yet, the requirement for more memory can lead to increased costs, depending on the deployment.
Kafka for Stream Processing
Kafka focuses on stream processing, allowing the handling of real-time data feeds. It provides a robust platform for building real-time applications. One notable characteristic is its ability to handle large volumes of streams, making it highly scalable and reliable.
Kafka's unique feature is the publish-subscribe model, enhancing data communication between different parts of an application. Though beneficial for real-time analytics, the configuration can be complicated for less experienced users.
Data Visualization Tools
Tableau
Tableau stands out as a powerful data visualization tool. Its focus on transforming data into interactive, visual representations is crucial for understanding complex datasets easily. Users can create dashboards and reports that help convey insights effectively.
The primary feature of Tableau is its drag-and-drop interface, which simplifies visualization creation. However, while Tableau is user-friendly, it can become costly as data needs expand over time.
Power BI
Power BI provides comprehensive business analytics solutions. Its integration capabilities with Microsoft products enhance its appeal. Power BI is known for its affordability and allows users to create detailed reports and dashboards.
One notable advantage is the ease with which users can share insights across an organization. However, some users may find its advanced features less intuitive, requiring additional training.
QlikView
QlikView is distinguished by its associative data model, which allows users to explore data in a more natural way. This model helps link data from different sources without needing to structure data beforehand. QlikView’s focus on user-driven analytics makes it a favored tool among analysts looking for flexibility.
A potential downside is that while QlikView provides deep analytics capabilities, the initial setup can be complex and may require a steep learning curve for new users.
Machine Learning Algorithms
Supervised Learning Techniques
Supervised learning techniques play a crucial role in big data analysis by enabling systems to learn from labeled datasets. This method helps in making predictions based on historical data. The accuracy of predictions in various applications, such as customer behavior and market trends, can greatly benefit from these techniques.
A key characteristic is the reliance on future data being similar to past data. However, the model might perform poorly with truly new data patterns, making it vital to regularly update training datasets.
Unsupervised Learning Techniques
Unsupervised learning techniques focus on discovering patterns in data. This is essential when labels are not available. Clustering and association are some common methods used here. Unsupervised learning can reveal hidden insights, making it powerful for exploration.
The main advantage is its ability to handle a variety of data types and structures. However, interpreting results can sometimes be subjective, which may mislead decision-making if not approached carefully.
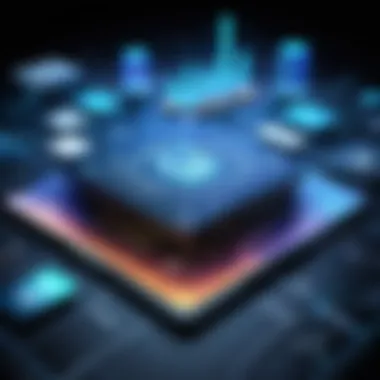
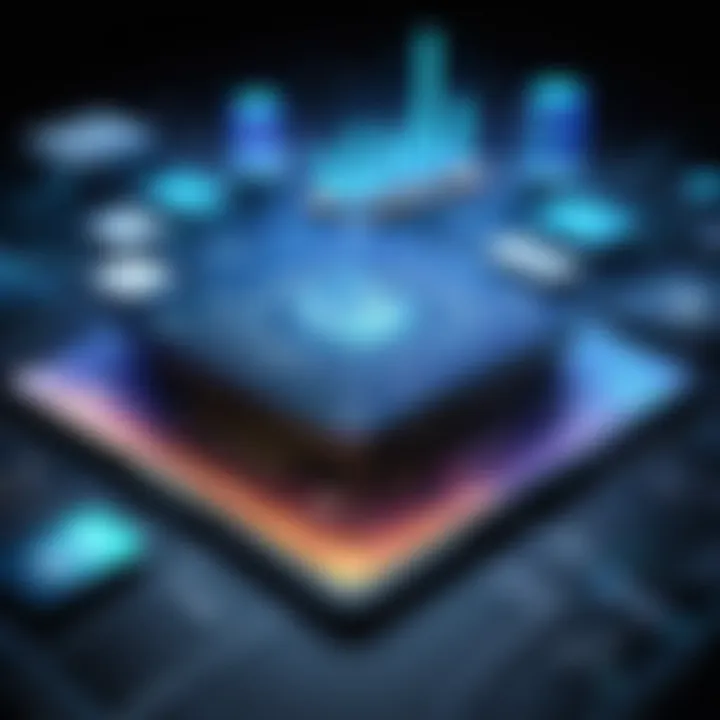
Reinforcement Learning Approaches
Reinforcement learning approaches allow for decision-making based on interactions with the environment. This method is particularly useful in settings where continuous feedback is essential, such as in robotics or adaptive learning systems. A key benefit includes optimizing actions to achieve the best outcomes over time.
Nonetheless, the training process can be resource-intensive and time-consuming. Organizations must be prepared for the investment in both computational power and time to reap the benefits.
Challenges in Big Data Analysis
Big data analysis involves significant hurdles that need consideration to fully harness its potential. Understanding these challenges is crucial because they directly impact the accuracy, effectiveness, and overall success of data-driven initiatives. By addressing these difficulties, organizations can improve their analytical capabilities and make better business decisions based on reliable insights.
Data Privacy Issues
Regulatory Compliance
Regulatory compliance is a critical aspect for organizations interacting with big data. Laws such as the General Data Protection Regulation (GDPR) impose strict guidelines on how personal data is collected and stored. Adhering to these regulations helps ensure consumer trust and avoids legal penalties. One key characteristic of regulatory compliance is its dynamic nature, as regulations can evolve, necessitating continual updates to data practices. While compliance may seem burdensome, its benefits include improved data management and respecting user privacy, vital in today's digital landscape.
Ethical Considerations
Ethical considerations in big data analysis go beyond legal obligations. Organizations must grapple with the morality of data usage, especially regarding sensitive information. This topic highlights the imperative of responsible data handling and informed consent. Ethical practices foster a culture of transparency and accountability, positioning an organization as a leader in its field. Despite the advantages of ethically sound practices, challenges arise in defining ethical boundaries and ensuring all stakeholders understand them.
Data Quality Concerns
Accuracy and Consistency
Accuracy and consistency are foundational to effective data analysis. If data is flawed, insights drawn from it will be misleading, potentially leading to poor decision-making. A notable characteristic of accuracy is that it often requires ongoing validation processes. Regular checks on data collection methods help maintain its integrity. However, prioritizing accuracy can be resource-intensive, posing a challenge for many organizations.
Data Integration Challenges
Data integration encompasses merging data from various sources into a coherent dataset. This presents a challenge due to the disparity in data formats and quality. A critical aspect of data integration is ensuring that the merged data retains its original context, which may involve complex mapping processes. While effective integration enhances the comprehensiveness of analysis, it may introduce complexity and require significant time investment.
Scalability Issues
Managing Large Datasets
Managing large datasets is a significant challenge, especially as organizations scale operations and data volumes increase. The focus here is on maintaining performance. A key characteristic of scalable data management is its reliance on advanced technologies, such as distributed computing. While these technologies offer solutions to managing volume, they may require substantial investment both financially and in terms of expertise.
Infrastructure Requirements
Infrastructure requirements play a pivotal role in the success of big data initiatives. Organizations must ensure they have adequate systems that can handle large-scale data processing. This includes high storage capacities and powerful computational resources. A unique feature of robust infrastructure is its flexibility to adapt as data needs grow. However, establishing a strong infrastructure may come with high upfront costs and ongoing maintenance challenges.
Future Trends in Big Data Analysis
The landscape of big data analysis is rapidly changing. Understanding future trends is essential for businesses and individuals aiming to leverage data effectively. Several trends are likely to shape the industry, enhancing the capabilities of big data analysis and refining its applications across various sectors.
Artificial Intelligence and Big Data
Artificial Intelligence (AI) is revolutionizing how we approach big data. By integrating machine learning algorithms, businesses can extract insights from data that were previously considered too complex. AI enhances predictive analytics, allowing companies to forecast trends and make informed decisions.
The combination of big data and AI can lead to significant improvements in several domains.
- Automated Insights: AI tools can analyze large datasets quickly, providing insights that would take human analysts much longer.
- Enhanced Accuracy: Algorithms can improve their performance over time, resulting in increasingly reliable predictions.
- Process Optimization: Organizations can fine-tune their operations based on data-driven insights generated through AI.
As AI continues to evolve, its integration with big data will open new avenues for innovation across industries.
Real-Time Analytics
Real-time analytics is another critical trend in big data analysis. The ability to process and analyze data instantaneously can significantly impact decision-making processes. Businesses can respond faster to market changes, consumer behaviors, and operational challenges.
Key benefits of real-time analytics include:
- Better Customer Experiences: Companies can adjust their offerings or services in real time based on consumer feedback.
- Competitive Advantage: Adapting quickly to trends can help businesses stay ahead of their competitors.
- Improved Operations: Organizations can monitor their operations continuously, allowing for proactive problem-solving.
In industries like finance and e-commerce, real-time analytics can be the difference between success and failure.
Cloud-Based Solutions
Cloud technology is becoming a dominant force in big data analysis. By utilizing cloud-based solutions, organizations can store vast amounts of data without the hefty investment in physical infrastructure. This trend provides several advantages:
- Scalability: Businesses can easily scale their data processing capabilities up or down based on their needs.
- Cost-Effectiveness: With a pay-as-you-go model, companies can manage expenses more effectively.
- Collaboration: Cloud environments promote collaboration across teams and departments, enabling seamless sharing of data and insights.
As businesses move towards more flexible cloud solutions, the ability to conduct big data analysis will become more widespread and accessible.
Ending
The conclusion of this article is critical to understanding the significance of big data analysis in today's business environment. Recapping the key points discussed throughout the article emphasizes how substantial this field has become. Big data analysis is not just a technical endeavor; it is a foundation for generating insights that directly influence strategic decision-making in various industries. Each method of data collection, processing, and analysis reveals layers of information that can alter business trajectories.
Recap of Big Data Analysis Importance
Big data analysis serves as a powerful tool for organizations aiming to leverage data for better outcomes. It equips businesses with the capability to:
- Understand consumer behavior through analytics.
- Enhance operational efficiencies by identifying bottlenecks in processes.
- Improve risk management, especially in sectors like finance.
Furthermore, the importance of big data is underscored by its role in maintaining competitive advantage. Companies that harness the power of big data analysis are often better positioned to anticipate market trends and adapt to changes swiftly. This adaptability can lead to increased market share and improved customer satisfaction.
Final Thoughts on Practical Applications
The practical applications of big data analysis are vast and varied, transcending industry boundaries. From healthcare improvements to optimizing retail strategies, the insights derived from data can transform the way organizations operate. Key considerations include:
- Integration with existing systems: Ensuring that big data tools complement current business processes is vital.
- Continuous learning: The landscape of data analysis evolves quickly. Keeping abreast of emerging technologies is essential.
- Ethical data use: As organizations delve deeper into data collection, they must prioritize ethical considerations to foster trust.