Unveiling the Profound World of Discovery Data Analysts
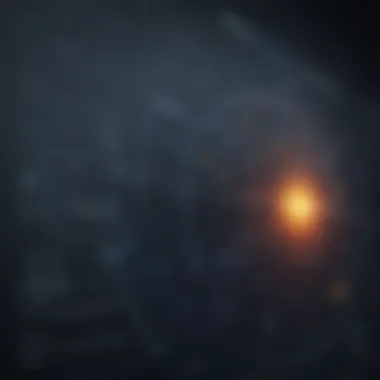
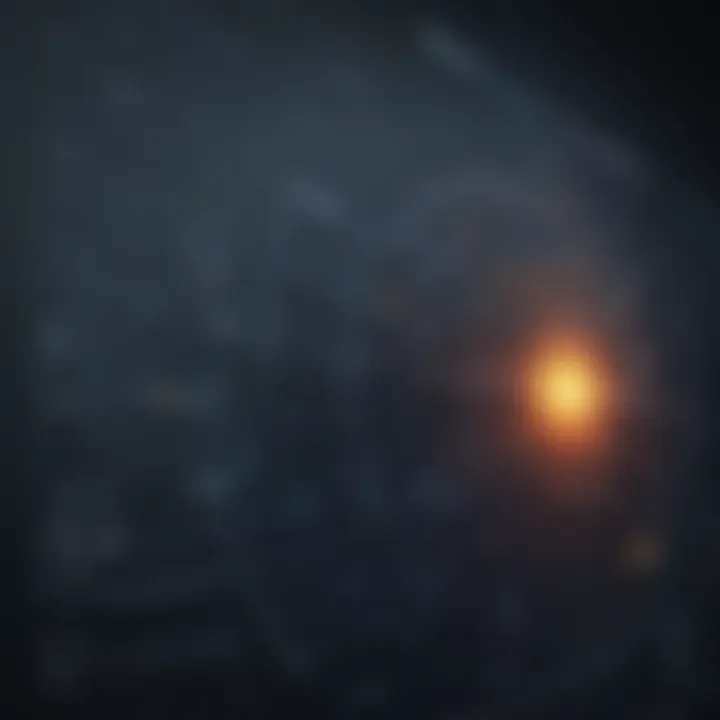
Tech Trend Analysis
In the dynamic realm of discovery data analysis, understanding tech trends is paramount. With data driving decisions and strategies, being abreast of the current trends is crucial for success. Tap into the vast ocean of data insights by analyzing trends that shape the landscape.
Unraveling the implications for consumers unveils a deeper understanding of their behavior and preferences. By deciphering trends, data analysts can anticipate consumer needs and create targeted solutions. It's not just about data; it's about the human touch in data interpretation.
Predicting future possibilities based on current trends sets the foundation for strategic planning. Data analysts play a pivotal role in forecasting the direction of the industry, enabling businesses to adapt proactively. By leveraging data to predict future scenarios, organizations gain a competitive edge in the ever-evolving market.
Product Reviews
When delving into the world of discovery data analysis, a critical aspect is evaluating the tools and products that aid in data interpretation. A detailed overview of products available in the market provides insights into their functionalities and utility.
Exploring the features and specifications of data analysis tools offers a comprehensive view of their capabilities. From data visualization to predictive analytics, each feature plays a crucial role in simplifying complex data sets.
Conducting performance analysis of these products sheds light on their efficiency and reliability. Understanding how well they process and interpret data enables data analysts to choose the most suitable tool for their needs.
Highlighting the pros and cons of each product ensures a balanced view for prospective users. By weighing the advantages against the limitations, analysts can make informed decisions regarding tool selection. Based on performance and versatility, recommendations can be tailored to suit specific data analysis requirements.
How-To Guides
Introducing aspiring data analysts to the intricacies of their craft is essential in nurturing their skills. A step-by-step guide elucidates the process from data collection to interpretation, providing a structured approach to analysis.
Incorporating tips and tricks enhances the learning experience, offering valuable insights that streamline the data analysis process. By sharing best practices and efficient methods, novices can expedite their learning curve and hone their analytical skills.
Troubleshooting common challenges faced during data analysis equips analysts with problem-solving skills. Identifying and resolving data discrepancies ensures the accuracy and reliability of insights generated. By addressing potential roadblocks proactively, analysts can navigate through complex data sets with confidence.
Industry Updates
Keeping abreast of the latest developments in the tech industry is instrumental for data analysts, as technologies evolve rapidly. Analyzing market trends and the impact on businesses provides a holistic view of the industry landscape.
Recent advancements in data analytics tools and techniques offer insights into the evolving best practices. Understanding these developments equips analysts with the knowledge to adapt to industry changes and leverage new opportunities.
The impact on businesses and consumers showcases the ripple effect of tech industry updates. From enhanced user experiences to data security measures, these updates influence decision-making processes across sectors. By comprehensively analyzing these updates, data analysts can stay ahead of the curve and drive innovation within their organizations.
Introduction
In the ever-evolving landscape of data analytics, the role of a Discovery Data Analyst holds profound significance. This introductory section sets the stage for a deep dive into the realm of extracting invaluable insights from data. By shedding light on the core responsibilities and essential skills required, this article serves as a beacon for aspiring data analysts venturing into the captivating world of discovery.
Understanding the Role of a Discovery Data Analyst
Analyzing Data Trends
Delving into the intricate realm of Analyzing Data Trends is akin to deciphering the language of data itself. This crucial aspect of a Discovery Data Analyst's role involves unraveling patterns and anomalies that are pivotal for the overall data interpretation process. Analyzing Data Trends acts as the compass guiding analysts through the vast ocean of information, enabling them to derive actionable insights that drive informed decision-making. Its robust analytical nature equips analysts with the prowess to identify emerging patterns and foresee market trends, making it an indispensable tool in the arsenal of data analysis.
Identifying Patterns
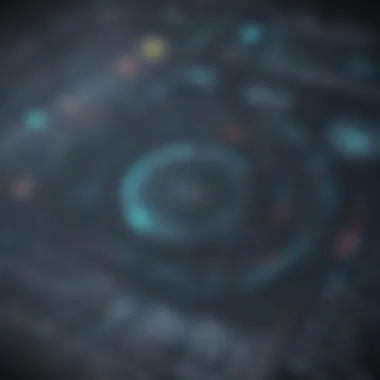
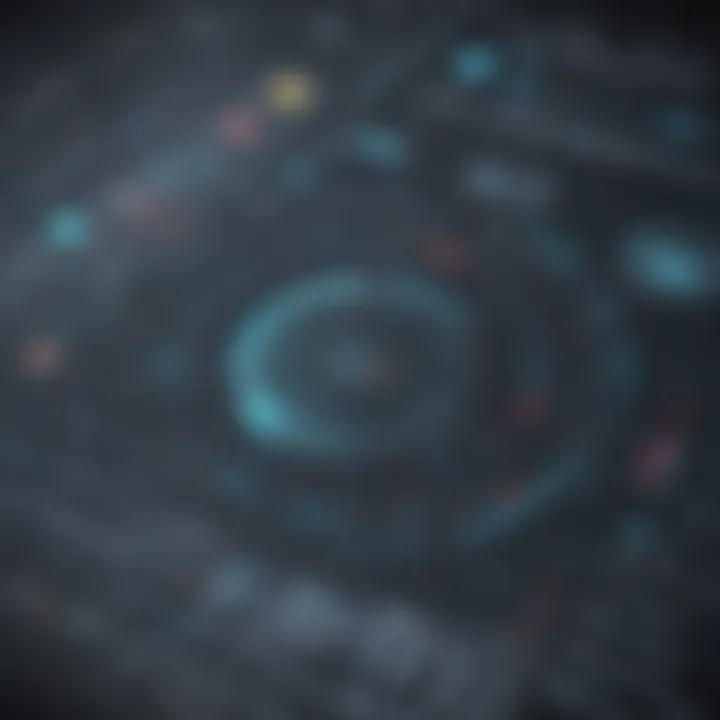
Identifying Patterns serves as the cornerstone of data interpretation, allowing Discovery Data Analysts to unearth hidden correlations within complex datasets. This skill involves recognizing repetitive structures or anomalies that provide a roadmap to understanding underlying phenomena or behaviors. The keen eye for detail required in Identifying Patterns empowers analysts to make data-driven decisions with unparalleled precision, making it a fundamental element in the palette of a skilled data practitioner.
Extracting Insights
At the heart of the data analysis process lies the essence of Extracting Insights, where raw data is transformed into tangible value for organizations. This pivotal aspect focuses on distilling complex information into actionable intelligence, enabling stakeholders to gain a competitive edge in their respective industries. While the process of Extracting Insights demands meticulous attention to detail and analytical prowess, the rewards of unlocking transformative revelations from data make it a highly sought-after skill in the arena of discovery data analysis.
Significance of Data Analysis in Modern Industries
Driving Decision-Making Processes
The art of Driving Decision-Making Processes through data analysis revolutionizes how organizations navigate the complexities of the modern business landscape. By leveraging data to inform strategic choices, companies gain a holistic view of market dynamics, customer preferences, and operational efficiency. This strategic approach not only enhances the decision-making acumen of businesses but also propels them towards sustainable growth and competitive advantage.
Enhancing Business Strategies
The process of Enhancing Business Strategies through data analysis injects a data-driven model into the traditional frameworks of strategic planning. By harnessing insights derived from data, organizations can fine-tune their strategies, optimize resource allocation, and capitalize on emerging market opportunities. This transformative approach not only augments an organization's agility but also fortifies its position in the market, fostering a culture of innovation and adaptability.
Optimizing Operations
Optimizing Operations through data analysis ushers in a new era of operational efficiency and cost-effectiveness for modern industries. By scrutinizing operational data streams, organizations can identify bottlenecks, streamline processes, and enhance overall productivity. The systematic approach of optimizing operations not only reduces wastage and redundancies but also elevates the scalability and sustainability of businesses in a dynamically evolving market landscape.
Core Responsibilities
In the enthralling world of Discovery Data Analysts, the core responsibilities play a pivotal role in extracting essential insights from voluminous datasets. As the gatekeepers of valuable information, data analysts are tasked with a multitude of critical functions that shape the decision-making processes of modern industries. Data Collection and Preparation stand at the forefront of these responsibilities, encompassing Data Cleaning, Data Integration, and Data Transformation. Without efficient and meticulous handling of data at this stage, the subsequent analytical processes cannot yield accurate results.
Data Collection and Preparation
Data Cleaning
Data cleaning is the meticulous process of identifying and rectifying errors within datasets to ensure accuracy and reliability in analytical outcomes. By scrubbing datasets of inconsistencies, duplicates, and missing values, data analysts guarantee the integrity of the data for downstream analysis. The key characteristic of Data Cleaning lies in its ability to enhance the quality of data, enabling analysts to derive meaningful insights with confidence. Although resource-intensive, this step is imperative in guaranteeing the validity of analytical results in the context of Discovery Data Analysis, where the accuracy of insights is paramount.
Data Integration
Data Integration refers to the consolidation of disparate data sources into a unified format for seamless analysis. By combining information from various origins, analysts gain a comprehensive view of the data landscape, enabling more informed decision-making processes. The key characteristic of Data Integration is its ability to unlock hidden patterns and relationships that may remain obscured in individual datasets. While enhancing the comprehensiveness of data, this process may introduce challenges related to data compatibility and merging methodologies, requiring skilled navigation for optimal outcomes.
Data Transformation
Data Transformation involves converting raw data into a standardized format that is conducive to analysis and interpretation. By reshaping data structures, aggregating information, and applying necessary computations, analysts prepare the data for statistical analysis and modeling. The unique feature of Data Transformation lies in its role as a bridge between raw data and actionable insights, shaping the data in a manner that aligns with analytical objectives. While enabling enhanced data usability, this process demands careful consideration of transformation methodologies to avoid distortions in the analytical outcomes.
Statistical Analysis
Hypothesis Testing
In the domain of statistical analysis, Hypothesis Testing serves as a fundamental tool for validating assumptions and drawing statistical inferences from data. By formulating null and alternative hypotheses, analysts assess the significance of relationships and effects within datasets. The key characteristic of Hypothesis Testing is its role in determining the validity of analytical conclusions, providing a structured framework for decision-making processes. Despite its analytical rigor, this approach may encounter challenges related to assumptions and sample sizes, influencing the robustness of conclusions in an analytical context.
Regression Analysis
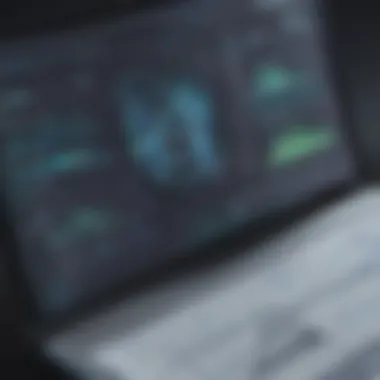
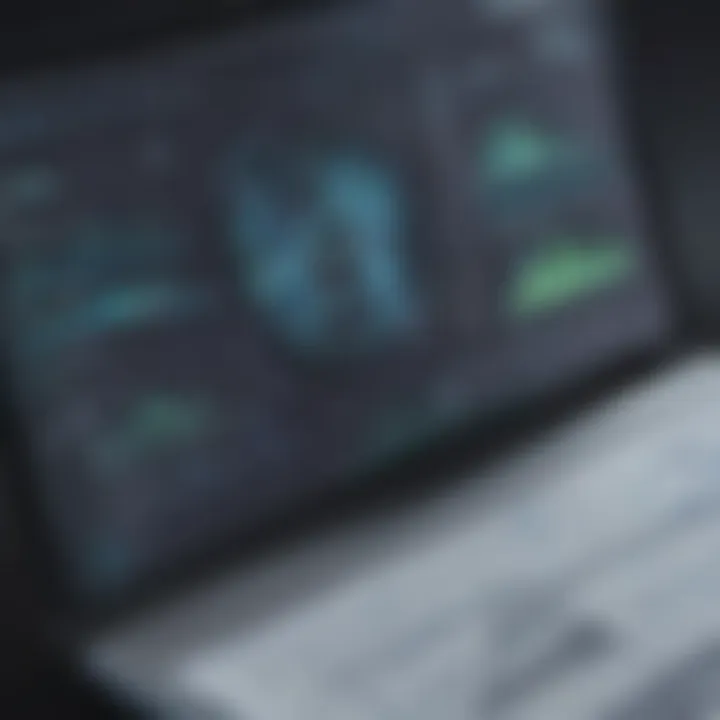
Regression Analysis is a statistical technique employed to model and analyze the relationships between variables within datasets. By identifying patterns and dependencies, analysts can make predictions and infer causal relationships based on empirical evidence. The key characteristic of Regression Analysis lies in its versatility and applicability across diverse data types, offering insights into the predictive trends within datasets. While providing valuable insights, this approach may present complexities in model interpretation and assumption validations, requiring a comprehensive understanding of statistical principles.
Cluster Analysis
Cluster Analysis involves segmenting data points into homogenous groups based on inherent similarities or patterns, enabling analysts to uncover hidden structures within datasets. By identifying clusters, analysts can discern trends, anomalies, or consumer preferences, facilitating targeted decision-making processes. The key characteristic of Cluster Analysis is its ability to elucidate data patterns without prior assumptions, fostering organic discovery within datasets. Despite its efficacy in pattern recognition, this approach may encounter challenges related to scalability and cluster validation, necessitating robust methodologies for reliable insights in analytical contexts.
Essential Skills
Understanding the Essential Skills required for a Discovery Data Analyst is crucial for excelling in this field. These skills serve as the foundation for performing tasks efficiently and effectively. A combination of Technical Proficiency and Analytical Mindset is essential for a data analyst to navigate through complex datasets and derive meaningful insights that drive strategic decisions. Without mastering these competencies, a data analyst may struggle to interpret data accurately and provide valuable recommendations for businesses. Therefore, a deep dive into Essential Skills is imperative in this article to equip aspiring data analysts with the necessary tools for success.
Technical Proficiency
Technical Proficiency stands out as a key pillar in the skill set of a Discovery Data Analyst. It encompasses various technical skills that are vital for handling data effectively. Within this domain, three crucial elements play a significant role:
Programming Languages
Programming Languages play a crucial role in data analysis, offering diverse functionalities to manipulate, clean, and analyze data efficiently. Python, R, and SQL are some popular programming languages utilized by data analysts for their versatility and robust capabilities. Python, renowned for its simplicity and extensive libraries, is highly favored for its data manipulation and visualization abilities. R, on the other hand, caters more towards statistical analysis and complex data modeling. SQL, a standard database querying language, is indispensable for accessing and managing database information. Each language has unique features that cater to distinct aspects of data analysis, providing analysts with a wide array of tools to handle different data-related tasks effectively.
Database Management
Database Management is another critical aspect of Technical Proficiency for data analysts. The ability to efficiently organize and retrieve data from databases is essential for conducting thorough analyses. Skills in database management enable data analysts to manipulate large datasets effectively and ensure data integrity throughout the analysis process. Understanding database structures, queries, and optimization techniques is essential for streamlining data access and enhancing analytical processes.
Data Visualization Tools
Data Visualization Tools play a significant role in transforming complex data into visually appealing and comprehensive representations. Tools like Tableau, Power BI, and D3.js enable data analysts to create interactive visualizations that facilitate better understanding and interpretation of data insights by stakeholders. These tools offer diverse visualization options, including charts, graphs, and dashboards, allowing analysts to present data in a visually engaging manner. The use of data visualization tools enhances communication of findings, aiding decision-makers in grasping key insights effectively.
Analytical Mindset
Apart from technical skills, an Analytical Mindset is paramount for a successful Discovery Data Analyst. It involves three critical components that shape the way data analysts approach and solve analytical challenges:
Critical Thinking
Critical Thinking plays a pivotal role in data analysis by enabling analysts to evaluate information objectively and make informed decisions based on evidence. It involves analyzing data systematically, questioning assumptions, and considering different perspectives to arrive at well-founded conclusions. Developing critical thinking skills equips data analysts with the ability to identify patterns, anomalies, and potential insights within datasets, enhancing the quality and depth of their analysis.
Problem-Solving
Problem-Solving skills are essential for resolving complex data-related issues and designing effective analytical solutions. Data analysts encounter various challenges during the analysis process, ranging from data inconsistencies to algorithm selection. Proficiency in problem-solving enables analysts to identify the root causes of issues, devise innovative strategies to overcome obstacles, and optimize their analytical workflow. Strong problem-solving skills empower data analysts to tackle data complexities head-on and deliver accurate and actionable insights to stakeholders.
Logical Reasoning
Logical Reasoning forms the foundation of effective data analysis, enabling analysts to deduce conclusions based on logic and rationality. It involves systematically assessing data patterns, relationships, and implications to draw valid inferences and hypotheses. By applying logical reasoning skills, data analysts can validate their analysis methodologies, identify logical fallacies, and ensure the accuracy and reliability of their findings. The integration of logical reasoning in data analysis promotes precision and rigor in decision-making processes, strengthening the credibility and relevance of analytical outcomes.
Career Path
In this article, we delve into the crucial aspect of a Discovery Data Analyst's career path, shedding light on the journey to becoming a proficient professional in the field of data analysis. Understanding the career path is vital for individuals aiming to excel in this domain, as it outlines the educational and professional steps required to succeed. By exploring the career path of data analysts, we uncover the key milestones, challenges, and opportunities that individuals may encounter on this career trajectory. Considering the rapid evolution of industries reliant on data, navigating a well-defined career path is instrumental in achieving success and making significant contributions to the field.
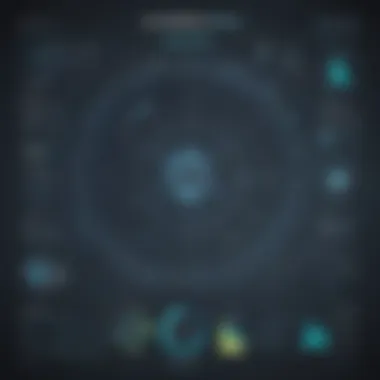
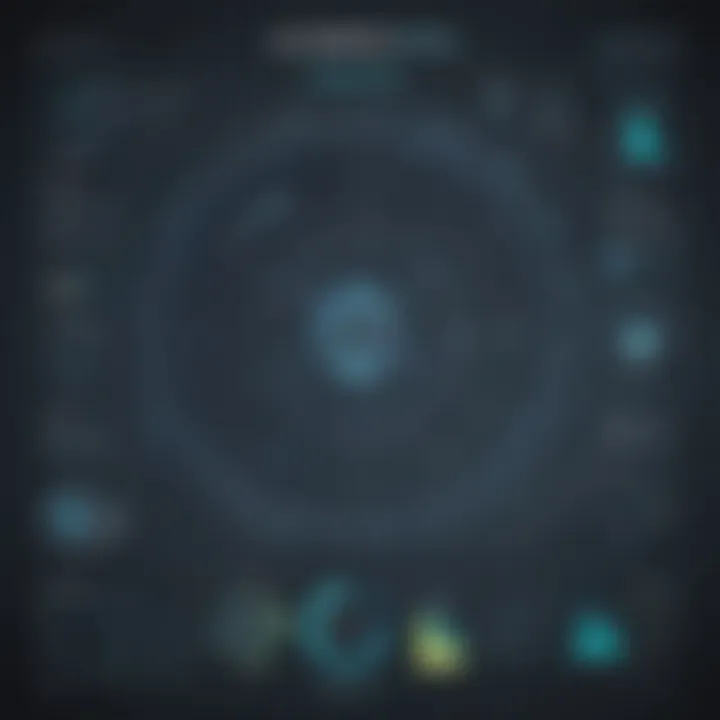
Educational Background
Degrees in Data Science
Delving into Degrees in Data Science is imperative for aspiring data analysts looking to garner specialized knowledge and skills. These degrees serve as foundational pillars that equip individuals with a comprehensive understanding of statistical methods, machine learning algorithms, and data mining techniques. The primary advantage of pursuing a degree in data science lies in the in-depth knowledge acquired in areas such as data visualization, data management, and predictive analytics. Despite the rigorous nature of these programs, the benefits of honing one's expertise through a data science degree significantly outweigh the challenges, making it a popular choice among individuals aspiring to carve a niche in the data analytics realm.
Certifications in Analytics
Exploring Certifications in Analytics proves to be a valuable asset for data analysts aiming to enhance their credibility and expertise in the field. These certifications validate an individual's proficiencies in data analysis, statistical modeling, and interpretation of complex data sets. An essential characteristic of these certifications is their industry relevance, providing practitioners with a competitive edge in the job market. While pursuing certifications in analytics demands dedication and commitment, the advantages of gaining industry-recognized credentials far outweigh the investment, making it a favorable option for individuals seeking career advancement in data analytics.
Specialized Courses
Embarking on Specialized Courses is instrumental in augmenting the skill set of data analysts, offering focused training in advanced data analysis techniques and tools. These courses cater to individuals looking to specialize in specific areas of data analytics such as big data management, business intelligence, or data-driven decision-making. A standout feature of specialized courses is their practical approach, enabling learners to apply theoretical concepts to real-world scenarios effectively. While opting for specialized courses necessitates a time and financial commitment, the benefits of acquiring specialized knowledge and proficiency in niche areas position individuals as invaluable assets in the competitive landscape of data analysis.
Professional Development
Internships
Exploring Internships opens doors for aspiring data analysts to gain hands-on experience, practical insights, and industry exposure. Internships offer a unique opportunity to apply theoretical knowledge to practical projects, collaborate with seasoned professionals, and cultivate essential soft skills. The key advantage of pursuing internships lies in the experiential learning and networking opportunities they provide, setting a strong foundation for a successful career in data analysis. Although internships require dedication and adaptability to varying work environments, the advantages of acquiring practical skills and industry insights make them a worthy investment in one's professional development.
Networking
Navigating the realm of Networking is integral for data analysts seeking to establish connections, leverage opportunities, and stay abreast of industry trends. Building a robust professional network enables individuals to access mentorship, career guidance, and potential job prospects within the data analytics landscape. The primary characteristic of effective networking is its reciprocity, where individuals share knowledge, resources, and support to foster mutually beneficial relationships. While active networking demands time and effort to cultivate meaningful connections, the advantages of expanding one's professional circle and gaining valuable insights far outweigh the initial investment, positioning individuals for career growth and success.
Continuous Learning
Embracing Continuous Learning is a cornerstone of professional growth for data analysts keen on staying competitive and relevant in a dynamic industry landscape. Continuous learning entails ongoing skill development, exploration of emerging trends, and engagement with evolving technologies in data analysis. The pivotal characteristic of continuous learning is its adaptability, allowing individuals to upskill, reskill, and innovate in response to changing industry demands. While committing to continuous learning demands discipline and dedication to knowledge acquisition, the advantages of remaining current, versatile, and future-ready in the data analytics field make it an essential practice for sustained career advancement and industry impact.
Industry Demand
In this segment, we delve into the critical aspect of Industry Demand within the broader context of discovery data analysis. Industry Demand holds immense significance as it directly influences the opportunities and growth potential for data analysts. Understanding the specific needs and requirements of various industries is crucial for data analysts to align their skills and expertise effectively. By focusing on Industry Demand, professionals can strategically position themselves to meet the evolving needs of different sectors, ensuring continued relevance and career advancement.
Growing Need for Data Analysts
Tech Companies
Tech Companies play a pivotal role in driving the growing demand for data analysts due to their heavy reliance on data-driven decision-making processes. These companies leverage data analysis to enhance product development, optimize user experiences, and streamline operations. The key characteristic of Tech Companies lies in their agile and dynamic work environment, which offers data analysts the opportunity to work on cutting-edge technologies and innovative projects. However, the fast-paced nature of Tech Companies may require data analysts to adapt quickly to changing requirements and priorities.
Financial Institutions
Financial Institutions represent another significant sector fueling the demand for data analysts, particularly in areas such as risk management, fraud detection, and customer analytics. The key characteristic of Financial Institutions is their focus on data security, regulatory compliance, and precision in decision-making processes. Working in Financial Institutions provides data analysts with exposure to complex financial data and the opportunity to contribute to critical business strategies. However, the highly regulated nature of the financial sector may necessitate data analysts to have a strong understanding of compliance standards and protocols.
Healthcare Sector
The Healthcare Sector is increasingly recognizing the value of data analysis in improving patient care, operational efficiency, and medical research. The key characteristic of the Healthcare Sector is its emphasis on data privacy, ethical considerations, and the direct impact of data analysis on patient outcomes. Data analysts in healthcare have the unique opportunity to work on projects that directly contribute to saving lives and enhancing healthcare services. However, the sensitive nature of healthcare data necessitates data analysts to adhere to strict privacy regulations and ethical guidelines to ensure the confidentiality and integrity of patient information.
Future Career Outlook
In this section, we explore the future career outlook for data analysts, analyzing key aspects such as job prospects, salary trends, and the evolution of roles within the industry. Understanding the evolving landscape of data analysis careers is essential for professionals looking to make informed decisions about their career paths and growth opportunities.
Job Prospects
The job prospects for data analysts remain strong across various industries, with a growing demand for skilled professionals to interpret and leverage data for strategic decision-making. Data analysts can explore diverse career opportunities in fields such as consulting, e-commerce, healthcare, and technology, allowing them to specialize in areas that align with their interests and expertise. The key characteristic of job prospects lies