Unleashing AutoML's Potential: A Comprehensive Guide to Its Diverse Use Cases
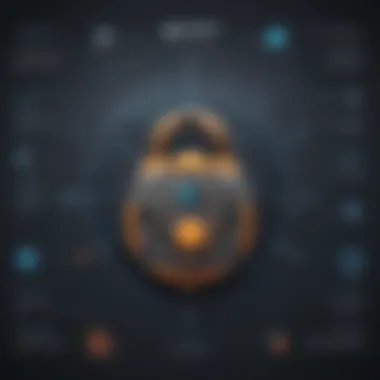
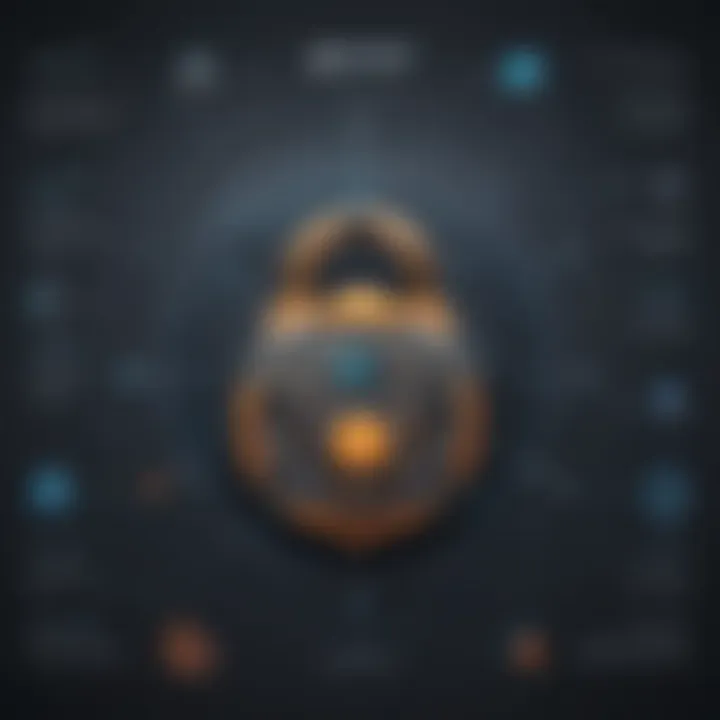
Tech Trend Analysis:
Automated Machine Learning (Auto ML) is at the forefront of reshaping the machine learning landscape, revolutionizing data analysis and predictive modeling by automating complex processes. This profound technology has far-reaching implications for consumers, enhancing decision-making processes, improving operational efficiencies, and driving unprecedented accuracy in predictive analytics. The current trend in the tech industry gravitates towards automation and efficiency, where AutoML stands as a game-changer. Future predictions suggest that AutoML will continue to evolve, offering more advanced algorithms and capabilities that will further streamline data analysis and modeling processes.
Product Reviews:
Delving into the realm of Auto ML products, one can find a plethora of options tailored to different industry needs. These products come equipped with advanced features and specifications aimed at simplifying the data modeling process for users. Performance analysis reveals that AutoML products significantly reduce the time and skill required for traditional machine learning tasks, allowing users to focus on extracting insights rather than fine-tuning algorithms. While the pros include efficiency, scalability, and accuracy, cons may relate to specific customization requirements or limitations in handling extremely complex data structures. Overall, AutoML products come highly recommended for organizations seeking to streamline their machine learning processes.
How-To Guides:
Embarking on the journey of Auto ML implementation requires a solid understanding of the fundamental concepts underlying automated machine learning. This step-by-step guide aims to provide novice users with the necessary instructions to harness the power of AutoML effectively. From an introduction to AutoML's architecture and functioning to detailed instructions on setting up workflows and interpreting results, this guide walks users through the entire process. Additionally, tips and tricks are shared to optimize performance and troubleshoot common issues that may arise during implementation, ensuring a smooth and seamless experience for users at every stage.
Industry Updates:
Recent developments in the tech industry highlight the increasing adoption of Auto ML across various sectors. As organizations realize the transformative power of automated machine learning, market trends indicate a shift towards automation and efficiency in data analytics and modeling. This wave of innovation has a significant impact on businesses and consumers, leading to improved decision-making, enhanced operational efficiencies, and higher predictive accuracy. The integration of AutoML solutions in diverse industries signifies a new era of streamlined data analysis and modeling, propelling organizations towards greater success in the digital age.
Introduction to AutoML
Automated Machine Learning (Auto ML) stands at the forefront of revolutionizing the way we approach machine learning tasks. In this detailed exposition of AutoML, we uncover its significance in streamlining complex processes and enhancing predictive capabilities. By automating the tedious aspects of machine learning, AutoML enables organizations to expedite their workflows, make more precise predictions, and optimize decision-making processes. Embracing AutoML not only saves time and resources but also empowers businesses to leverage the full potential of their data.
Understanding Automated Machine Learning
The Evolution of AutoML
Introducing the extraordinary evolution of Auto ML, a concept that has significantly altered the landscape of data science. The advancement in AutoML technologies has paved the way for efficient automation of complex machine learning tasks, offering unparalleled convenience and accuracy to users. Its ability to adapt and improve with each iteration makes it a preferred choice for businesses seeking rapid evolution and innovation in their data analytics processes. Despite some limitations, the evolution of AutoML proves instrumental in driving the progress of machine learning applications.
Key Components of AutoML
Exploring the fundamental components that form the essence of Auto ML, one encounters a myriad of intricate algorithms and functionalities designed to optimize machine learning workflows. These key components serve as the backbone of AutoML, enabling users to automate data preprocessing, model selection, and optimization seamlessly. Their unique features, such as algorithm selection and hyperparameter tuning, streamline the machine learning process, making it more accessible and efficient. While these components offer significant advantages in terms of scalability and performance, users must cautiously navigate potential drawbacks to maximize the benefits derived from AutoML applications.
Benefits of Auto
ML Implementation
Enhanced Efficiency
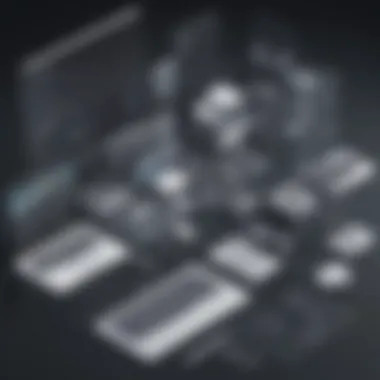
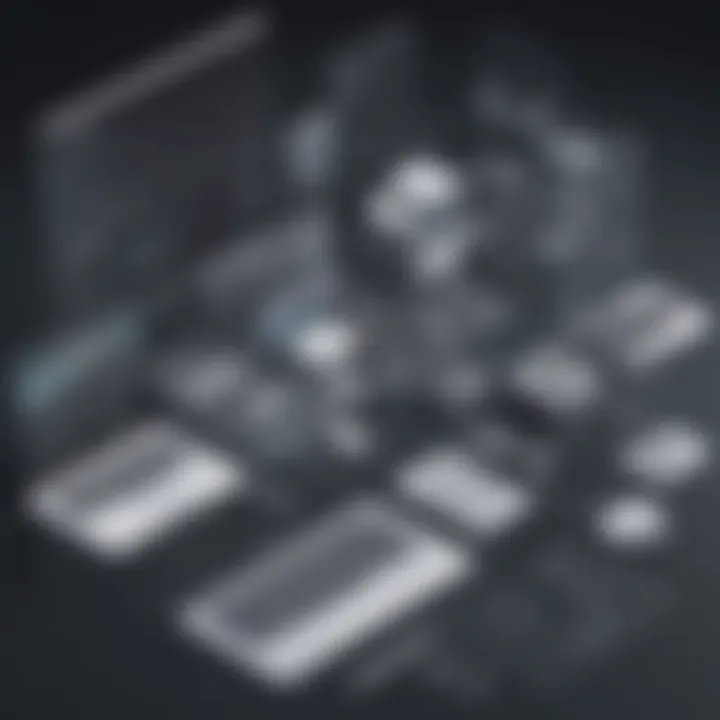
Delving into the realm of enhanced efficiency brought forth by Auto ML, we witness a transformation in how organizations approach data analysis and predictive modeling. By automating repetitive tasks and accelerating model development, enhanced efficiency empowers business users to tackle complex data challenges with ease and precision. Leveraging the time-saving capacities of AutoML not only boosts productivity but also fosters a culture of data-driven decision-making across industries.
Improved Accuracy
The pursuit of improved accuracy through Auto ML introduces a paradigm shift in the fidelity of predictive models and analytical insights. By leveraging advanced algorithms and robust data processing techniques, AutoML elevates the accuracy levels attained in machine learning applications, enabling more reliable and informed decision-making. This emphasis on accuracy not only enhances the trustworthiness of model predictions but also sets a new standard for precision in data analytics practices.
Reduced Time-to-Insight
Reducing the time-to-insight is a critical benefit that Auto ML brings to the table, enabling organizations to glean actionable intelligence from data in significantly less time. By automating complex processes and expediting model deployment, AutoML accelerates the pace at which insights are generated, optimizing decision-making timelines and enhancing strategic responsiveness. This reduction in the time-to-insight not only enhances operational efficiency but also allows businesses to stay agile and adaptive in a rapidly evolving data landscape.
AutoML in Data Analysis
Automated Machine Learning (Auto ML) in the realm of data analysis holds immense significance in illustrating the power of artificial intelligence in processing vast amounts of data efficiently to derive meaningful insights. It plays a pivotal role in automating traditionally time-consuming tasks like data preprocessing and model selection, leading to accelerated decision-making processes and improved outcomes. AutoML in data analysis revolutionizes how organizations approach data challenges by streamlining complex procedures and enhancing the overall data analysis workflow.
Automating Data Preprocessing
Feature Engineering
In the context of Auto ML, Feature Engineering stands out as a crucial step in data preprocessing, aiming to extract relevant features from raw data to enhance the predictive capability of machine learning models. Feature Engineering involves transforming the initial dataset into a format that is conducive to model training, often improving the model's accuracy and performance. This process plays a key role in optimizing the model's ability to understand patterns within the data, leading to more precise predictions and insights. Despite its advantages in enhancing model efficiency, Feature Engineering also presents challenges such as the risk of overfitting or creating irrelevant features if not executed meticulously.
Data Cleaning and Transformation
Data Cleaning and Transformation are integral components of data preprocessing within the Auto ML framework. Data cleaning involves identifying and rectifying errors, inconsistencies, or missing values in the dataset to ensure the accuracy and reliability of the predictive model. Transformation processes focus on reshaping the data to meet the requirements of specific machine learning algorithms, promoting optimal performance during model training. While Data Cleaning and Transformation enhance the quality of the dataset for analysis, inadequate preprocessing may introduce biases or inaccuracies that can impact the model's predictive capabilities, underscoring the importance of thorough data preparation in AutoML workflows.
Auto
ML in Predictive Modeling
Auto ML in Predictive Modeling holds a pivotal role in this discourse on AutoML applications. In the realm of predictive modeling, AutoML streamlines the process of model development by automating tasks that are traditionally time-consuming and intricate. By leveraging AutoML algorithms, businesses can expedite the model training phase and improve the overall efficiency and accuracy of predictive analytics. This section elucidates the specific elements that make AutoML indispensable in predictive modeling, emphasizing its benefits in delivering more precise insights in a fraction of the time typically required.
Automated Model Training
Regression Analysis
Delving into Regression Analysis within Automated Model Training, this method plays a crucial part in deriving valuable insights from data. Regression Analysis is characterized by its ability to establish relationships between variables, making it a fundamental tool in predictive modeling. Its utility lies in its capability to predict continuous outcomes based on input variables, providing a quantitative assessment of the relationships within the data. In the context of this article, Regression Analysis emerges as a prominent choice due to its accuracy in forecasting numerical values. However, it is imperative to consider the assumptions and limitations associated with this method, ensuring a nuanced understanding of its efficacy in various scenarios.
Classification Tasks
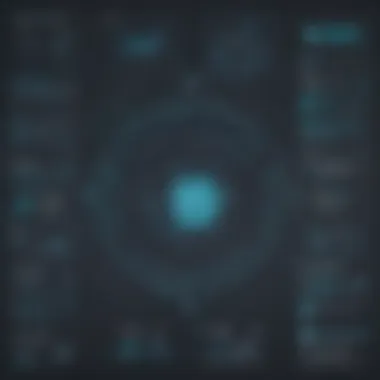
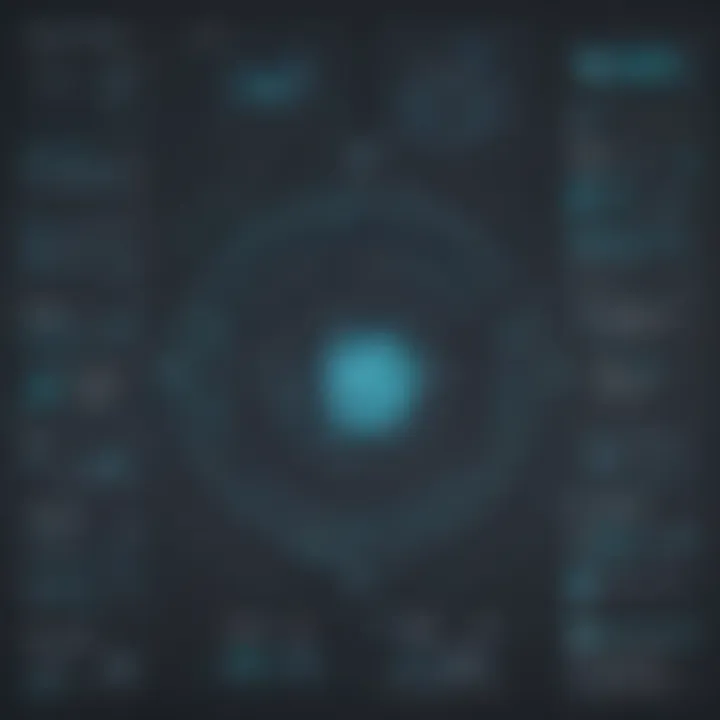
Regarding Classification Tasks within Automated Model Training, this facet contributes significantly to the overarching goal of accurate predictive modeling. Classification Tasks focus on segmenting data points into distinct categories based on specific attributes, enabling businesses to make informed decisions using historical data patterns. A key feature of Classification Tasks lies in their ability to classify unseen data accurately, hence enhancing the model's predictive capabilities. In the context of this article, the unique attribute of Classification Tasks lies in their versatility in handling diverse datasets, although considerations need to be made regarding the model's interpretability and potential biases.
Enabling Rapid Prototyping
Model Deployment
Model Deployment plays a vital role in enabling rapid prototyping in predictive modeling scenarios. This aspect involves the seamless transition of trained models into real-world applications, allowing organizations to capitalize on predictive insights efficiently. The key characteristic of Model Deployment lies in its capacity to facilitate the integration of machine learning models into existing systems, ensuring a seamless deployment process. In this article, the significance of Model Deployment is highlighted for its role in bridging the gap between predictive modeling outputs and actionable insights, underscoring its relevance in driving business value.
Scalability
Scalability is a critical aspect contributing to the efficiency of Auto ML in predictive modeling applications. By addressing scalability concerns, AutoML solutions can accommodate growing datasets and evolving business needs without compromising performance or accuracy. The key characteristic of Scalability is its ability to uphold model performance even with larger datasets, ensuring consistent predictive accuracy over time. In the context of this article, the unique feature of Scalability is its adaptability to dynamic business environments, although considerations must be made regarding computational costs and resource allocation.
AutoML in Healthcare
Auto ML in Healthcare is an integral aspect of automated machine learning explored in this article. It plays a crucial role in revolutionizing the medical industry by leveraging the power of AutoML to enhance diagnostics, treatment, and medical research. AutoML in healthcare offers a plethora of benefits, including improved accuracy in disease prediction, personalized medicine, streamlined drug discovery, and optimized clinical trials.
Enhancing Diagnostics and Treatment
Disease Prediction
Disease Prediction is a vital component of Auto ML in healthcare as it focuses on utilizing machine learning algorithms to forecast diseases in patients. The key characteristic of Disease Prediction lies in its ability to analyze complex medical data and patterns, leading to early detection and preventive measures. This approach is instrumental in improving patient outcomes and reducing healthcare costs. However, a challenge with Disease Prediction is the need for high-quality and diverse healthcare data for accurate predictions.
Personalized Medicine
Personalized Medicine is another critical aspect of Auto ML in healthcare, aiming to tailor medical treatment to individual characteristics. The key characteristic of Personalized Medicine is its ability to utilize patient-specific data, such as genetics and lifestyle factors, to create targeted treatment plans. This personalized approach enhances treatment efficacy, minimizes side effects, and ultimately improves patient satisfaction. However, the implementation of Personalized Medicine requires advanced algorithms and robust data privacy measures to ensure the security of sensitive patient information.
Streamlining Medical Research
Drug Discovery
Drug Discovery stands out as a significant advancement in medical research facilitated by Auto ML. This process involves leveraging machine learning models to identify potential drug candidates and accelerate the drug development timeline. The key characteristic of Drug Discovery is its ability to analyze vast amounts of chemical and biological data efficiently, leading to the discovery of novel therapies for various diseases. While Drug Discovery expedites the identification of promising drug compounds, it also presents challenges such as the validation of virtual screening results in real-world experiments.
Clinical Trials Optimization
Clinical Trials Optimization is a fundamental application of Auto ML in healthcare that aims to streamline the process of conducting clinical trials. The key characteristic of Clinical Trials Optimization is its capability to optimize patient selection criteria, trial design, and data analysis, resulting in more cost-effective and efficient clinical studies. By leveraging AutoML in clinical trials, researchers can identify suitable patient cohorts, reduce trial duration, and enhance decision-making processes. However, challenges such as regulatory compliance and the interpretability of machine learning models need to be addressed to ensure the validity and ethical conduct of optimized clinical trials.
AutoML in Finance
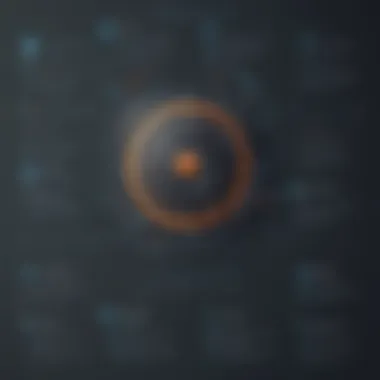
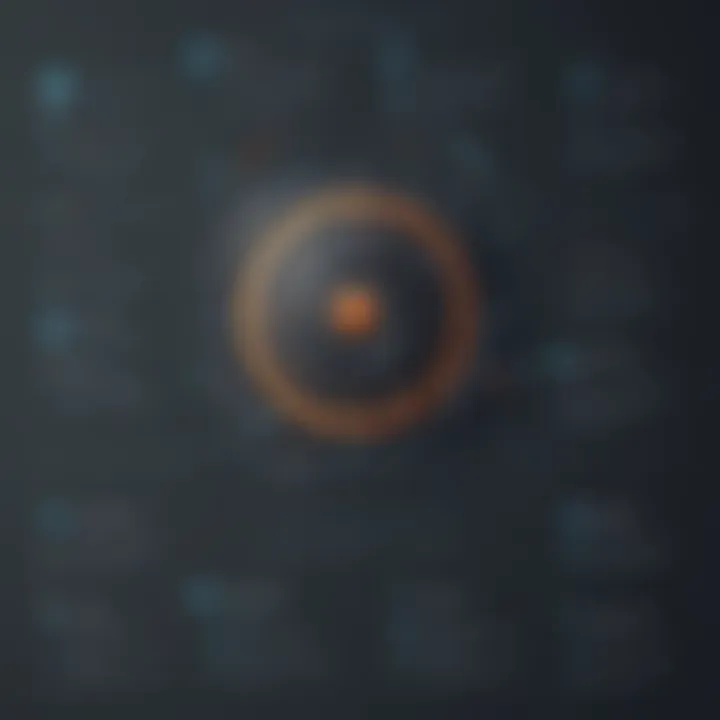
Auto ML in Finance is a pivotal aspect of this comprehensive guide, shedding light on the specific applications, benefits, and considerations within the financial sector. The integration of Automated Machine Learning (AutoML) in finance holds immense importance due to its ability to streamline operations, enhance decision-making processes, and mitigate risks effectively. By leveraging AutoML in finance, institutions can automate critical tasks like risk management and fraud detection, leading to improved efficiency and accuracy in their operations.
Risk Management and Fraud Detection
Credit Scoring
Credit Scoring emerges as a vital component within the realm of Auto ML in Finance, playing a significant role in assessing the creditworthiness of individuals or entities. The key characteristic of Credit Scoring lies in its predictive power, enabling financial institutions to evaluate the likelihood of default accurately. This aspect of Credit Scoring is particularly beneficial for this article as it showcases how AutoML can optimize lending processes and minimize credit risks. However, one potential disadvantage of Credit Scoring through AutoML is the reliance on historical data, which may not always capture current economic conditions accurately.
Anomaly Detection
Anomaly Detection stands out as another crucial element in the domain of Auto ML in Finance, offering advanced capabilities to identify irregularities or fraudulent activities within financial systems. The key characteristic of Anomaly Detection is its ability to detect deviations from normal behavior patterns, enabling swift intervention to prevent financial losses. This aspect of Anomaly Detection is highly valuable for this article as it highlights how AutoML can bolster fraud detection mechanisms and safeguard financial institutions. Nevertheless, a challenge of Anomaly Detection through AutoML is the potential for false positives, which might lead to unnecessary investigations and operational disruptions.
Enhancing Investment Strategies
Investment Strategies are augmented significantly through the incorporation of Auto ML in Finance, revolutionizing how financial decisions are made and optimizing portfolio performance. Portfolio Optimization emerges as a critical aspect, emphasizing the importance of diversification, risk management, and return maximization in investment portfolios. The key characteristic of Portfolio Optimization lies in its ability to generate optimal asset allocations based on predefined objectives, thereby enhancing overall portfolio efficiency and performance. This facet of Portfolio Optimization is particularly advantageous for this article as it elucidates how AutoML can enhance investment outcomes while reducing systematic risks. However, a potential limitation of Portfolio Optimization through AutoML is the complexity of implementation and the need for robust data inputs and model validation processes.
Market Forecasting
Market Forecasting plays a pivotal role in shaping investment strategies within the financial domain, providing valuable insights into future market trends and optimal trading decisions. The key characteristic of Market Forecasting is its predictive accuracy, enabling investors to anticipate market movements and adjust their strategies proactively. This element of Market Forecasting is highly relevant for this article as it underscores how Auto ML can empower investors with actionable market intelligence and improve decision-making processes. Nonetheless, a challenge of Market Forecasting through AutoML is the inherent unpredictability of financial markets, which may pose risks in relying solely on automated forecasting models.
Overall, Auto ML in Finance offers a transformative approach to decision-making processes, risk management, and investment strategies within the financial sector. By harnessing the power of Automated Machine Learning, financial institutions can gain a competitive edge, optimize operational efficiency, and drive sustainable growth in a dynamic economic landscape.
AutoML in E-Commerce
Auto ML plays a pivotal role in revolutionizing the landscape of E-Commerce, offering a wide array of benefits and opportunities for businesses operating in the online retail sector. By leveraging AutoML, E-Commerce companies can enhance their customer experiences, streamline operations, and drive personalized marketing strategies. One of the key aspects of AutoML in E-Commerce is its ability to analyze vast amounts of customer data efficiently, allowing businesses to gain valuable insights into consumer behavior and preferences. This enables E-Commerce platforms to offer tailored product recommendations, personalized marketing campaigns, and optimized supply chain management. Implementing AutoML in E-Commerce can lead to increased customer engagement, higher conversion rates, and overall improved business performance.
Personalizing Customer Experiences
Recommendation Systems
Recommendation Systems are a crucial component of Auto ML in E-Commerce, playing a pivotal role in enhancing user engagement and driving sales. These systems utilize machine learning algorithms to analyze customer preferences, browsing patterns, and purchase history to provide personalized product recommendations to users. One of the key characteristics of Recommendation Systems is their ability to continuously learn and adapt based on user interactions, ensuring that the recommendations remain relevant and effective over time. In this article, Recommendation Systems are highlighted for their capability to significantly improve the shopping experience for customers, leading to higher customer satisfaction and increased sales. However, a potential disadvantage of Recommendation Systems is the challenge of balancing personalized recommendations with user privacy concerns, which requires careful handling and transparency from E-Commerce businesses.
Targeted Marketing
Targeted Marketing is another essential aspect of Auto ML in E-Commerce, focusing on tailoring marketing campaigns to specific customer segments based on their preferences, behavior, and demographics. The key characteristic of Targeted Marketing is its ability to deliver personalized messages and promotional offers to customers, increasing the likelihood of conversion and engagement. In this article, Targeted Marketing is highlighted for its effectiveness in reaching the right audience with relevant content, thereby maximizing marketing ROI and driving customer loyalty. One unique feature of Targeted Marketing is its capability to analyze real-time data and adjust marketing strategies in response to changing market dynamics, ensuring proactive and adaptive marketing campaigns. However, a potential disadvantage of Targeted Marketing is the risk of excluding certain customer segments from broader marketing initiatives, which could impact brand perception and reach.
Optimizing Supply Chain Management
Auto ML plays a crucial role in optimizing supply chain management for E-Commerce businesses, offering advanced forecasting and predictive capabilities to streamline inventory management and demand planning. Through AutoML-driven Inventory Forecasting, E-Commerce companies can accurately predict product demand, optimize inventory levels, and minimize stockouts or overstock situations. The key characteristic of Inventory Forecasting is its ability to analyze historical sales data, market trends, and external factors to generate precise demand forecasts, aiding businesses in efficient inventory planning and replenishment. In this article, Inventory Forecasting is highlighted for its contribution to cost reduction, operational efficiency, and improved customer satisfaction by ensuring product availability and timely deliveries. While Inventory Forecasting brings significant advantages to E-Commerce businesses, a potential challenge lies in managing demand variability and unforeseen disruptions which may affect forecasting accuracy.
Demand Prediction
Demand Prediction is a critical aspect of Auto ML in E-Commerce, enabling businesses to anticipate and plan for future customer demand with greater accuracy and insight. By leveraging machine learning algorithms, Demand Prediction models can analyze customer behavior, market trends, and seasonal patterns to forecast demand for products or services. The key characteristic of Demand Prediction is its ability to help E-Commerce businesses optimize inventory levels, pricing strategies, and marketing campaigns based on forecasted demand, driving operational efficiency and revenue growth. In this article, Demand Prediction is emphasized for its role in proactive decision-making, resource allocation, and risk mitigation by providing actionable insights into future demand trends. While Demand Prediction offers significant benefits in terms of improving supply chain efficiency and customer satisfaction, potential disadvantages may include reliance on historical data accuracy and the need for continuous model retraining to maintain predictive performance.