Understanding Data Visualization in Data Analytics
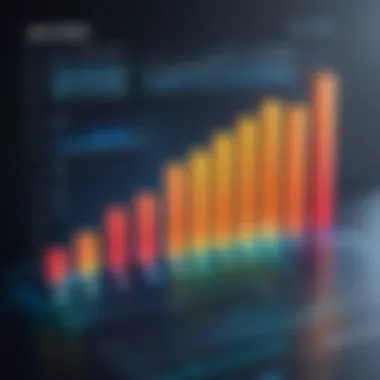
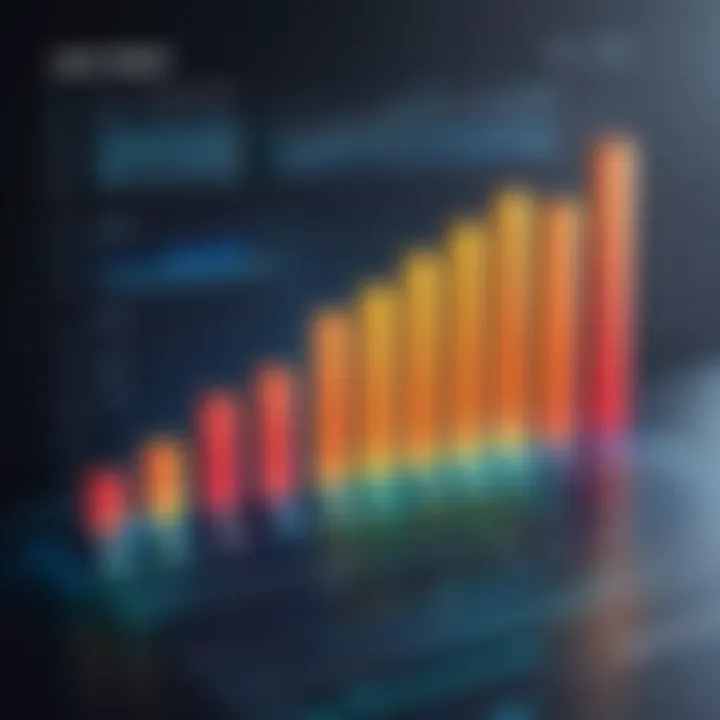
Intro
Data visualization is a fundamental component of data analytics. It transforms raw data into accessible visual formats, allowing people to derive insights quickly. This transformation is crucial in guiding decision-making processes. By presenting complex datasets graphically, it enables analysts and stakeholders to recognize patterns and trends that might otherwise go unnoticed.
In todayโs fast-paced environment, effective data visualization has gained increased relevance. Companies across various industries are investing in tools and techniques that convert data into meaningful visuals. This is not merely a trend; it is an essential practice that enhances strategic planning and operational efficiency.
Tech Trend Analysis
Overview of the current trend
Data visualization is evolving as technology advances. Current trends include the integration of artificial intelligence in analytical tools, which aids in generating more accurate visuals. Tools like Tableau and Microsoft Power BI are providing user-friendly interfaces that enable users to create complex visualizations without extensive technical knowledge. These platforms are growing rapidly in adoption due to their ability to democratize data.
Implications for consumers
The shift towards user-centered data visualization means that consumers are better equipped to interpret data. This enhanced accessibility allows for informed decision-making not just for businesses, but also for individuals. For example, consumers can visualize their spending habits through budget apps, making smarter financial choices.
Future predictions and possibilities
Looking ahead, the future of data visualization appears promising. As datasets grow larger and more complex, the need for effective visualization is set to increase. Innovations in augmented reality and virtual reality could revolutionize how we interact with data. Imagine a future where one can explore datasets in a three-dimensional space.
"Effective data visualization is not merely about aesthetic appeal; it's about clarifying data to improve insights and decision-making."
Industry Updates
Recent developments in the tech industry
The rise of machine learning has significantly impacted data visualization. Machine learning algorithms analyze data faster and more efficiently, leading to more accurate visualization outputs. New data visualization packages are emerging that focus on storytelling through data. Companies are increasingly recognizing the value of narrative in analytics, and tools are adjusting accordingly.
Analysis of market trends
The demand for skilled data visualization professionals is on the rise. Many companies are seeking individuals who can bridge the gap between complex analytical data and engaging visuals. This demand is not limited to tech companies; industries like healthcare and finance are also investing heavily in data visualization capabilities.
Impact on businesses and consumers
With better visualization tools, businesses can gain insights more readily than before. This impact is not isolated to the internal stakeholders; consumers benefit too. As companies adopt these practices, the end-users can enjoy improved services, as businesses can adjust operations based on visualized data trends.
Culmination
Understanding data visualization in analytics is critical for both professionals and consumers today. The trends show a robust movement towards integrating advanced technology and making data accessible. As we navigate this landscape, the importance of visual clarity cannot be overstated. The ability to interpret data effectively will not only drive individual decisions but will also steer business strategies in the future.
Defining Data Visualization
Data visualization plays a crucial role in data analytics. It refers to the graphical representation of information and data. Through visuals like charts, graphs, and maps, complex data sets become easier to digest and analyze. The ability to represent data visually is essential for deriving insights and making informed decisions.
Foreword to Data Visualization
Data visualization simplifies complexity. With vast amounts of data generated daily, visual tools help in identifying patterns, trends, and outliers. Instead of combing through spreadsheets filled with numbers, analysts can observe visual data representations. This makes it simpler to grasp information at a glance. For example, a line graph can show trends in sales over time, allowing businesses to make quicker adjustments to strategies.
Moreover, effective data visualization can highlight the relationships between variables. This understanding can guide businesses in predicting future outcomes. A good visual can communicate what numbers cannot explain in detail, enhancing comprehension and engagement.
The Role of Visualization in Data Analytics
Visualization is not merely about aesthetics; it fundamentally changes how data is interpreted. In the field of data analytics, the role is expansive and multifaceted.
First, visualization serves as a communication tool. It transforms findings into stories that resonate with stakeholders. Complex subjects can be distilled into visuals that are interpretative and impactful. For instance, a dashboard displaying key performance indicators gives executives an overview in real-time, facilitating strategic planning.
Second, visualization aids in data exploration. Analysts use data visualization to explore vast datasets and uncover hidden insights. By utilizing scatter plots or heat maps, they can analyze correlations or anomalies, leading to data-driven decisions.
Lastly, it enhances retention. People are more likely to remember information presented visually compared to raw data alone. Therefore, visualization plays a critical part in conveying messages and facilitating long-term understanding. The blend of clarity and visual engagement vastly improves the analytical process, paving the way for better results.
Importance of Data Visualization
Data visualization plays a crucial role in data analytics. It simplifies complex data sets, making them more accessible and understandable. In today's data-driven world, the ability to visualize information effectively is indispensable.
Facilitating Data Understanding
Visualization transforms raw data into graphical formats, allowing easier interpretation. For instance, a well-constructed bar chart can highlight differences in sales performance across regions quickly. Graphical representation of data reduces cognitive load, enabling stakeholders to grasp insights without overwhelming detail. Simple charts or graphs can illuminate trends that might be obscured in vast tables of numeric data.
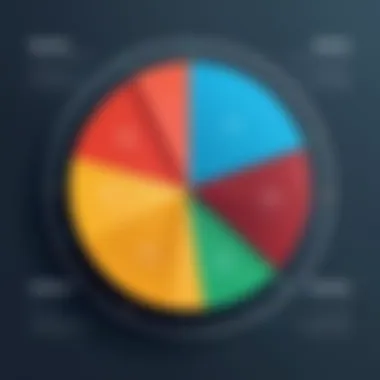
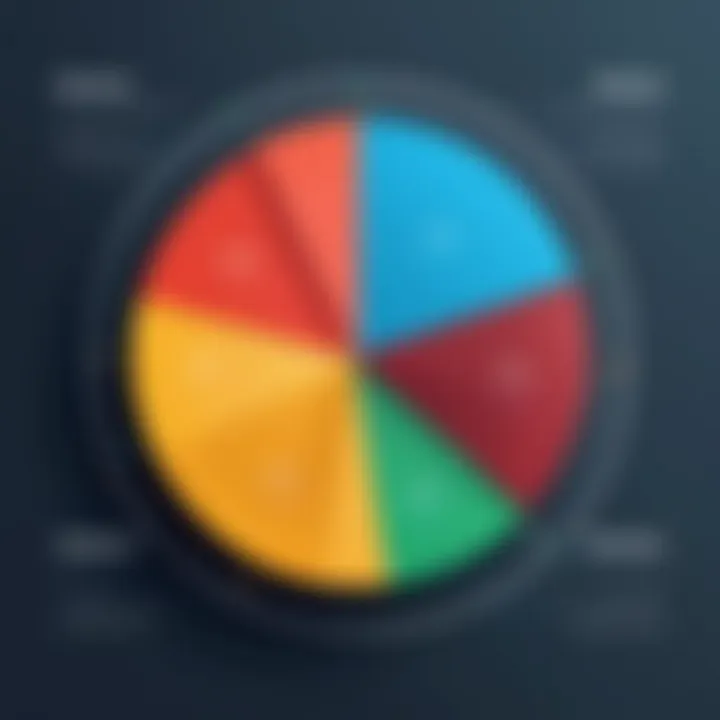
Enhancing Communication of Insights
Communication is vital in any analytical process. Data visualization bridges the gap between analysts and decision-makers. By presenting data visually, analysts can convey messages more effectively. A clear heat map can reveal concentration areas, while a concise line graph can show progress over time. This clarity fosters discussions that lead to informed actions. In team meetings, visual evidence can provoke thoughtful dialogue, facilitating a shared understanding of data-driven narratives.
Driving Data-Driven Decision Making
Organizations increasingly rely on data to guide their decisions. Visualization not only presents data but also highlights patterns, correlations, and anomalies. This information drives strategic initiatives. For example, a scatter plot can illustrate the relationship between marketing spend and customer acquisition. By interpreting such visuals, decision-makers can allocate resources wisely, corroborate hypotheses, and support initiatives with solid data.
"Data visualization is the difference between knowing what happens and understanding why it happens."
Embracing effective data visualization enhances overall organizational performance. Employees at different levels benefit from clarity. Those in management can utilize visuals to evaluate KPIs, while teams can track project statuses through dashboards. The impact of data visualization on decision-making processes is profound, emphasizing its essential nature in the analytical journey.
Common Data Visualization Techniques
Data visualization is paramount in the realm of data analytics, facilitating clear communication and understanding of complex data. Different techniques serve unique purposes and target various data types. Each visualization method has distinct strengths. Selecting the appropriate technique can significantly impact how information is perceived and interpreted.
Bar Charts and Histograms
Bar charts and histograms are fundamental types of data visualizations. While both use bars to represent data, they serve slightly different functions. Bar charts are effective for comparing discrete categories. They illustrate differences in values across categories, making trends easily recognizable. For instance, a bar chart showing sales figures for different products can help identify which product performs best.
In contrast, histograms are used for depicting distributions of continuous data. They show frequency, indicating how often data falls within certain ranges. This visualization is essential in statistics for understanding data distribution, skewness, or normality.
Considerations for use:
- Data type: Is the data categorical or continuous?
- Comparison: Are you comparing values of categories or distributions?
Line Graphs
Line graphs are significant when observing trends over time. They are ideal for showing continuous data, particularly in time series analysis. The linearity of line graphs allows viewers to easily perceive momentum and changes over periods. For example, one might track annual sales growth, leading to insights regarding market trends.
Furthermore, line graphs can compare multiple datasets concurrently by overlaying different lines on the same graph. This comparison can uncover relationships or divergences between datasets, yielding critical insights about behavior or patterns.
Benefits include:
- Trend identification: Quickly highlights trends and outliers.
- Multi-series capability: Easily overlay multiple datasets for comparison.
Pie Charts
Pie charts offer a very different approach to data visualization. They display proportions of a whole, with slices representing different categories. While visually appealing, they are often criticized for being less effective in delivering precise value comparisons. Pie charts suit specific instances, especially when illustrating parts of a whole.
Use them sparingly, as they may become unclear when dealing with many categories. The overall readability is decreased as slices become smaller. Ensure clarity in labeling and consider alternative visualizations for more nuanced datasets.
When to use:
- When representing simple proportions.
- When the number of categories is limited.
Heat Maps
Heat maps employ color gradients to represent data values across two dimensions. This visualization is particularly powerful for showcasing density or intensity. They can illustrate variations in data values comprehensively, often focusing on geographical representations or large datasets.
For example, a heat map could visualize population density by geographic area, allowing quick insights into concentration and regional trends. The gradation of color effectively communicates complex information without overwhelming the viewer.
Advantages:
- Immediate visual impact: Quickly reveals areas of intensity.
- Excellent for big data visualizations.
Scatter Plots
Scatter plots deliver a graphical representation of data points along two axes. This method illustrates relationships between variables, enabling viewers to search for correlations or trends. By plotting individual data points, one can gain insights regarding strength, direction, and possible outliers in relationships.
They are particularly useful in fields like economics, biology, and health analytics. For instance, plotting income against expenditure can reveal correlations crucial for economic studies.
Highlight:
Scatter plots can indicate trends, like positive, negative, or no correlation.
In summary, each visualization technique serves distinct purposes that are vital for effective data analysis and communication. Understanding these nuances allows practitioners to choose the most appropriate method based on their specific needs.
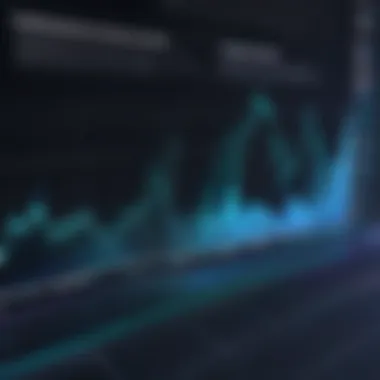
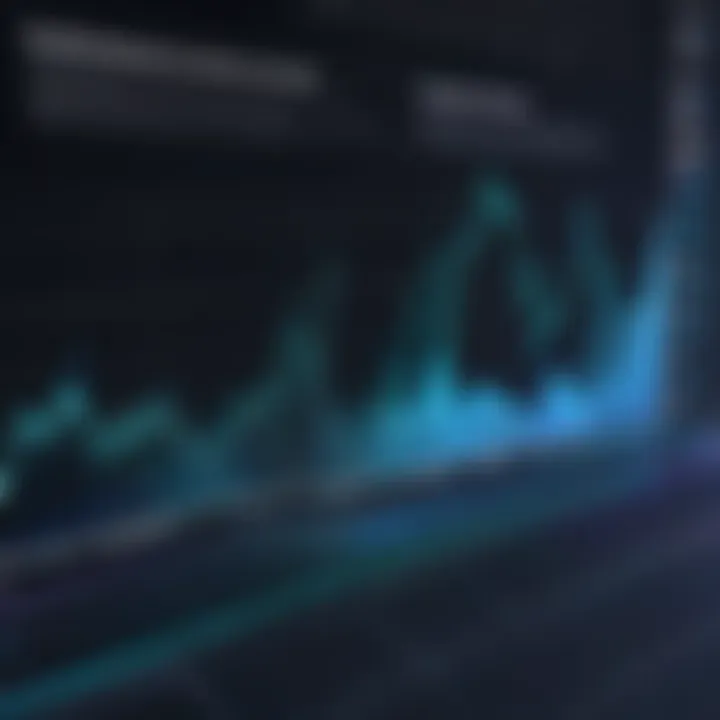
Choosing the Right Visualization Tools
Choosing the right visualization tools is a critical factor in effective data analytics. Tools serve as the medium through which data is translated into visuals that can be easily interpreted and understood. The selection of suitable tools can influence not only the clarity of the presentation but also the effectiveness of insights derived from data. Thus, understanding the various options available and their unique features is paramount in enhancing data comprehension and communication.
Overview of Popular Visualization Tools
Numerous visualization tools exist, each with its own strengths and functionalities. Here are some of the notable options:
- Tableau: Highly regarded for its interactive and user-friendly interface, Tableau allows users to create detailed and visually appealing dashboards.
- Microsoft Power BI: This tool integrates seamlessly with other Microsoft products, making it a favored choice among organizations already using Microsoft applications.
- QlikView: Known for its in-memory data processing, QlikView allows users to analyze data in real-time, facilitating swift insights.
- Google Data Studio: A free tool that provides a simple way to connect data sources and create shareable reports, enhancing collaboration.
- D3.js: A JavaScript library for producing dynamic and interactive data visualizations in web browsers, ideal for developers looking for flexibility.
Best Practices for Data Visualization
Data visualization is more than simply creating charts or graphs. It is about conveying information clearly and effectively. Best practices in data visualization are essential to ensure that visual representation of data is accurate, informative, and accessible. They serve as guidelines that enhance the viewerโs understanding and lead to more informed decisions.
Maintaining Clarity and Simplicity
Clarity is the cornerstone of effective data visualization. When a visualization is cluttered or overly complex, it can confuse the audience rather than inform them. To maintain clarity, it's crucial to focus on essential data points. Eliminate any unnecessary details that do not contribute to the main message.
- Keep your visual design simple. Use minimal text and avoid excessive embellishments.
- Label axes and data points clearly. This helps guide the viewer's understanding without requiring them to decipher complex patterns.
- Choose the appropriate type of chart to represent your data. For example, use line graphs for trends over time, and bar charts for comparing different categories.
"Clarity is about focusing on the relevant parts of the data to enhance understanding."
Using Color Effectively
Color plays a significant role in visual perception. When used effectively, it can highlight key data points, indicate trends, or categorize information. However, poor color choices can mislead the viewer. Here are some considerations for using color in data visualization:
- Utilize a limited color palette. Too many colors can overwhelm viewers. Aim for consistency and coherence in your color choices.
- Consider color blindness. Ensure that visualizations are accessible to those who may have difficulty distinguishing certain colors. Tools like color blindness simulators can help in this regard.
- Use contrasting colors to draw attention to critical data points. This can signal changes, anomalies, or any other aspects that deserve focus.
Incorporating Interactive Elements
Interactive elements can transform a static visualization into an engaging experience. They enable users to explore data from different angles, discovering insights at their own pace. Consider the following when incorporating interactive features:
- Allow users to hover over data points for additional details. This provides context without cluttering the visualization.
- Implement filtering options. Viewers should be able to isolate specific data sets that matter to them.
- Utilize tools like dashboards to combine multiple visual elements. This creates a comprehensive view that users can interact with and manipulate.
In the fast-evolving realm of data analytics, adhering to best practices in data visualization not only enhances the quality of the visualizations themselves but also enriches the decision-making process across various sectors. These practices ensure that the visualized data resonates with the intended audience, facilitating deeper insights and informed actions.
Case Studies in Data Visualization
Case studies in data visualization are critical because they offer real-world examples of how visualization techniques can translate complex data into actionable insights. Each case demonstrates the effectiveness of various visualization methods in a myriad of industries, highlighting the benefits and potential pitfalls when interpreting data. These studies not only reinforce the importance of thoughtful design in visualizations but also provide lessons on how to avoid common mistakes.
Business Applications
In the business sector, data visualization serves as a powerful tool in transforming raw data into insights that drive performance. Companies such as Amazon and Netflix utilize intricate visual styles to analyze customer preferences and behaviors. For instance, Amazon employs heat maps and user flow diagrams to optimize their website interface and tailor recommendations based on user interactions. This alignment of visualization with user data enhances customer satisfaction and fuels sales growth.
Moreover, organizations can identify market trends via scatter plots and bar charts. These tools simplify the information, allowing businesses to make informed decisions swiftly. When visualizations are clear and relevant, they minimize misunderstandings that could lead to poor strategic choices.
Healthcare Insights
In the healthcare industry, visualization of patient data has emerged as a cornerstone of efficient care delivery. Hospitals increasingly utilize dashboards that consolidate data regarding treatment effectiveness, patient wait times, and overall health trends. These tools allow for immediate insights into potential health issues.
For example, hospitals often turn to line graphs to monitor patient outcomes over time, which is vital for assessing treatment protocols. Success stories have surfaced where hospitals have significantly reduced readmission rates as a result of analyzing patient data visually. Furthermore, GIS mapping helps locate health resources geographically, illustrating disparities in healthcare access across different community areas.
"Data visualization in healthcare not only aids in clinical decisions but also empowers patients with information about their health."
Education Metrics
Education metrics benefit significantly from data visualization, as the interpretation of student performance data can impact curriculum design and educational strategies. Schools and educational bodies increasingly rely on dashboards and visual reports to track student attendance, grades, and engagement levels.
An illustrative example is the use of bar charts and population pyramids that represent demographic data, helping educators understand trends among various student cohorts. This approach allows stakeholders to identify areas needing improvement, facilitating targeted interventions. Moreover, interactive data visualizations can engage students in their learning process, making complex data more digestible.
Thus, case studies in education reveal that effective visualization not only improves educational outcomes but also enhances institutional decision making.
Future Trends in Data Visualization
In an era where data is becoming more abundant and integral to decision-making, future trends in data visualization are critical. They reflect how technology is evolving and shaping our interactions with data. This section explores emerging trends, particularly the roles of Artificial Intelligence (AI) and machine learning and advancements in personalization.
Trends in data visualization not only enhance how analysts interpret data but also improve the accessibility of insights for broader audiences. The increasing complexity of data demands visualizations that can distill information into digestible formats. This need propels innovations that redefine user experience and engagement with data.
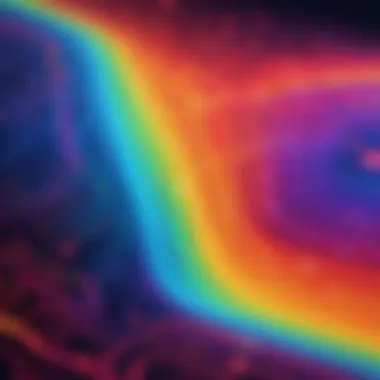
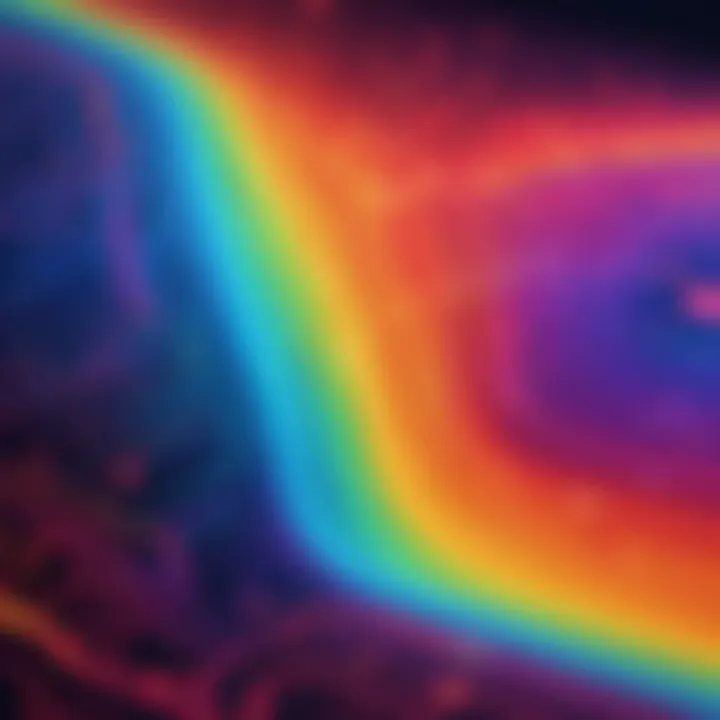
Emergence of AI and Machine Learning
The integration of AI and machine learning into data visualization represents a significant shift in how data is analyzed and presented. These technologies can automate the analysis process, offering predictive insights and real-time data updates.
For instance, AI algorithms can identify patterns and trends in vast datasets that may not be apparent to human analysts. These insights can be transformed into visual formats that are easy to understand. AI can also personalize visualizations based on user behavior, improving user engagement.
Some specific benefits of incorporating AI into data visualization include:
- Automation of Data Preparation: AI can preprocess data, ensuring accuracy and reliability, allowing analysts to focus on interpretation.
- Dynamic Visuals: As new data enters the system, visualizations can adapt instantaneously, providing up-to-date insights.
- Enhanced Predictive Capabilities: Machine learning models can forecast trends, which can then be visualized to guide strategic decisions.
Overall, the emergence of AI and machine learning in data visualization empowers businesses to leverage data more effectively, ultimately leading to informed decision-making.
Advancements in Personalization
Personalization in data visualization is gaining traction. Customization of visuals based on user preferences enhances the interaction with data. This trend acknowledges that different stakeholders may require various insights, impacting how they engage with visualizations.
Advancements in personalization may involve:
- User-Centric Designs: Tools that allow users to customize their dashboards or reports according to their needs.
- Adaptive Layouts: Visualizations that adjust based on user interactions or feedback, ensuring relevance and maintaining interest.
- Contextual Data Presentation: Providing users with data relevant to their specific roles or interests to increase the utility of the information presented.
Personalized data visualizations make information more accessible and actionable, facilitating better understanding and faster decision-making.
"As the landscape of data analytics evolves, the ability to present information in a user-friendly manner will determine the success of insights derived from data."
Ethical Considerations in Data Visualization
Ethics play a crucial role in ensuring that data visualizations are both truthful and respectful. In the age of big data, where information is rapidly proliferated and consumed, it becomes essential to maintain integrity in the way data is presented. Misleading or poorly constructed visualizations can distort reality, impacting decision-making processes, public opinion, and ultimately, trust.
Data visualizations can convey complex information at a glance. However, if they lead to misinterpretations, the consequences can be significant. As such, ethical considerations must be embedded in the data visualization process from the outset. This involves understanding not only the data set itself but also the potential implications of how that data is represented.
Avoiding Misrepresentation of Data
Misrepresentation occurs when visualizations distort facts and lead the audience to incorrect conclusions. This can happen through various means such as data cherry-picking, misleading scales, and inappropriate chart choices. For example, a bar chart showing a significant difference between two values can easily deceive if the scale of the y-axis is manipulated. This is why careful layout and scale settings are fundamental.
Critical steps to avoid misrepresentation include:
- Transparency: Always provide context and source for the data.
- Accuracy: Maintain fidelity to the underlying data, avoiding exaggeration of trends.
- Clarity: Opt for visualizations that best represent the message without distorting the values.
Adhering to these principles helps foster trust and credibility in data representation, ensuring that stakeholders can make well-informed decisions.
Data Privacy and Security
With the increasing reliance on data, the issue of privacy cannot be ignored. Data visualization efforts must prioritize the security of sensitive information. When visualizing data that includes personally identifiable information, it is essential to anonymize the data effectively. Failure to protect individual privacy can lead to severe legal repercussions and loss of stakeholder trust.
Incorporating data privacy strategies is vital. These may involve:
- Anonymization: Remove or alter identifying details before analysis and visualization.
- Data Minimization: Only use data relevant to the analysis, reducing the potential risk of exposure.
- Secure Tools: Utilize visualization tools that comply with security standards, protecting both data integrity and user privacy.
Ethical data visualization not only enhances understanding but also safeguards against harmful consequences that might arise from misinterpretation or privacy breaches.
End
The conclusion of this article is an essential part that wraps together the various threads we've explored regarding data visualization in data analytics. It reiterates the most critical points discussed and highlights why these concepts matter in todayโs technology-driven environment. Understanding data visualization not only enhances our ability to analyze complex data but also allows stakeholders to make informed and timely decisions. It reduces ambiguity and presents data in a more digestible format, thus leading to improved communication and collaboration among teams.
Summarizing Key Points
In summary, this article covers several key aspects:
- Defining Data Visualization: The fundamental concept and its importance in translating data into actionable insights.
- Importance of Data Visualization: How it facilitates understanding, enhances communication, and drives data-based decision-making.
- Common Techniques: Detailed examination of various visualization methods, including bar charts, line graphs, and scatter plots.
- Choosing Tools: Guidelines for selecting the right tools that match the needs of analysis and audience.
- Best Practices: Emphasis on clarity, effective use of color, and interactive elements.
- Case Studies: Real-world application of data visualization in business, healthcare, and education.
- Future Trends: Insights into how advancements in AI will shape data visualization.
- Ethics in Visualization: Importance of ethical practices in avoiding data misrepresentation and ensuring privacy.
These points enshrine the value and breadth of knowledge essential for anyone invested in data analytics.
The Continuing Relevance of Data Visualization
As we look ahead, the relevance of data visualization continues to grow. Rapid advancements in technology and an increase in data generation underline the need for more sophisticated and effective visualization tools. Industries across all sectors are dealing with vast amounts of data, making the ability to visualize and interpret this information crucial.
Moreover, as artificial intelligence becomes more integrated into analytics, the expectation of dynamic and interactive visualizations will rise. Stakeholders require not just any data, but a transformative approach that can present trends clearly and in real-time. An informed decision relies heavily on how well data is communicated.
In summary, the field of data visualization is not merely a passing trend; it is a critical aspect of data analytics that will continue to evolve.
"Data visualization is not just about making pretty pictures; it's about presenting data that informs and persuades."
The ongoing development of new tools and technologies augurs well for the future of data visualization. Its place in strategic decision-making is solidified more than ever.
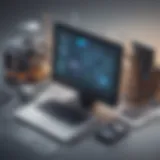
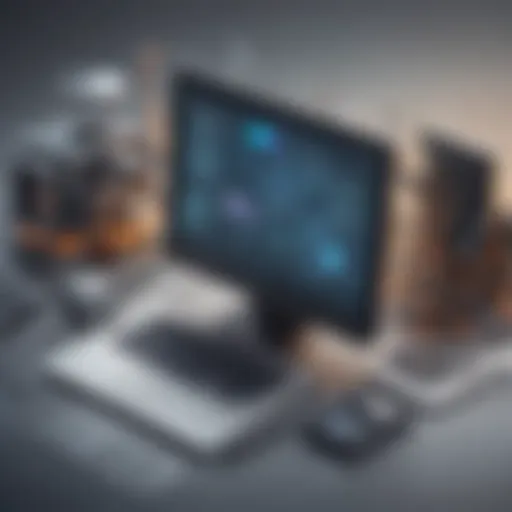