Understanding Data Analytics: Definition and Importance
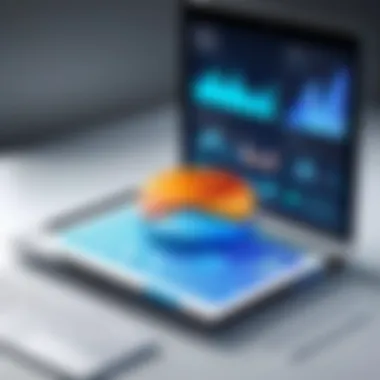
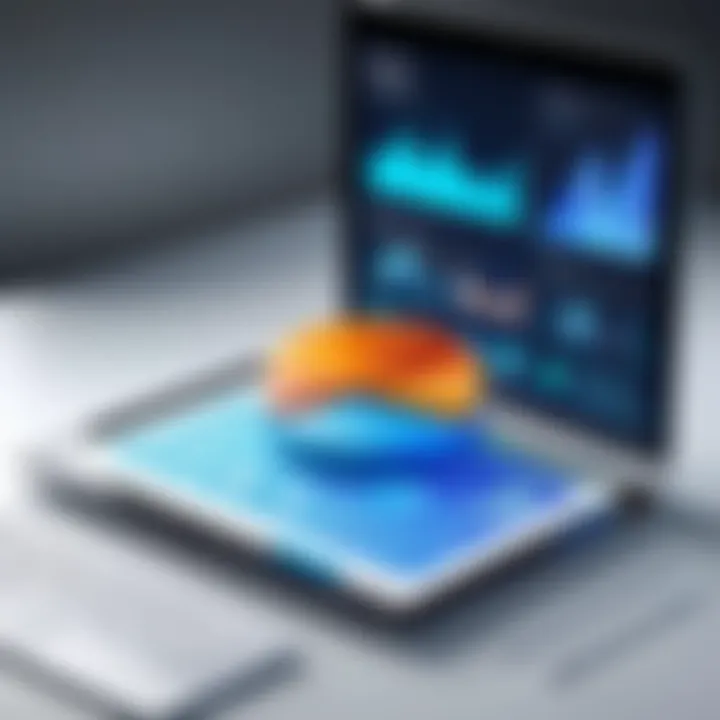
Intro
In the modern landscape, data analytics has emerged as a cornerstone of informed decision-making. The ability to collect, process, and analyze data allows organizations to uncover valuable insights that drive strategies and foster innovation. Understanding the key concepts of data analytics is crucial for individuals and businesses alike as we navigate an increasingly data-driven world.
Data analytics encompasses various methodologies and tools that help in interpreting complex data sets. The importance of data analytics transcends industries, influencing sectors such as healthcare, finance, and marketing. With the right analytical techniques, businesses can enhance productivity, improve customer experiences, and stay competitive in their respective markets.
This article will explore the definition of data analytics and its significance. We will delve into different processes involved in analytics, the tools commonly used, and the challenges faced during implementation. Moreover, we will study the applications of data analytics across various sectors, providing insights that highlight its growing relevance in today's context.
Tech Trend Analysis
Overview of the current trend
The rise of big data has stimulated the evolution of data analytics. Organizations are increasingly investing in advanced analytics solutions to leverage data for strategic advantages. Technologies like artificial intelligence and machine learning are becoming prevalent in analytics frameworks, enabling faster insights from larger datasets.
Implications for consumers
Consumers today experience the direct impacts of data analytics through personalized services. Retailers use analytics to recommend products based on past purchasing behavior. Similarly, companies like Netflix analyze viewer habits to tailor suggestions, enhancing user engagement and satisfaction. Thus, consumers can expect more customized experiences across various platforms.
Future predictions and possibilities
The future of data analytics indicates promising advancements. As the volume of data generated continues to grow, the methods for analyzing this data will become more sophisticated. Predictive analytics, for instance, is set to provide deeper insights that help in forecasting market trends. Furthermore, the integration of data analytics with IoT devices is likely to revolutionize how industries operate. Companies will gain real-time insights to optimize processes and reduce waste.
Industry Updates
Recent developments in the tech industry
The tech industry has witnessed significant enhancements in data analytics tools. Companies like Tableau and Microsoft Power BI now offer more user-friendly interfaces, allowing users to visualize data effortlessly. Open-source platforms like Apache Spark and R are also empowering analysts to conduct complex data analyses without substantial investment in software.
Analysis of market trends
Current market trends reveal that organizations are also focusing on data privacy. With regulations like GDPR and CCPA, companies need to be meticulous in handling data. This shift means that analytics must not only be effective but also ethical. As a result, there’s an increasing emphasis on developing transparent algorithms that protect consumer data while still providing insights.
Impact on businesses and consumers
For businesses, leveraging data analytics leads to enhanced efficiency and productivity. The ability to make data-driven decisions can significantly reduce operational costs. Consumers, on the other hand, benefit from improved products and services that meet their specific needs. As analytics continues to mature, the symbiotic relationship between businesses and consumers will play a pivotal role in shaping market dynamics.
What is Data Analytics?
Data analytics is an essential field that focuses on examining raw data to derive meaningful insights. It combines statistical analysis, data mining, and predictive modeling to facilitate decision-making in various industries. In today's technology-driven landscape, understanding data analytics has become crucial for organizations seeking to optimize performance and maintain competitiveness. The ability to analyze and interpret vast amounts of data allows businesses to make informed choices, predict trends, and enhance customer experiences.
Defining Data Analytics
Data analytics can be defined as the systematic computational analysis of data sets. This field uses various statistical and computational methods to uncover patterns and correlations within data. The main goal is to transform raw data into actionable insights that can enhance decision-making.
Data analytics encompasses several types of analysis, including:
- Descriptive analysis: Explores past data to summarize what has happened.
- Diagnostic analysis: Investigates data to identify reasons behind past outcomes.
- Predictive analysis: Uses historical data to forecast future outcomes.
- Prescriptive analysis: Suggests actions based on the predicted outcomes.
These facets of data analytics play a significant role in helping companies understand their operations, customer behavior, and market dynamics.
Components of Data Analytics
The components of data analytics integrate various processes and tools to achieve effective analysis. Key components include:
- Data Collection: Gathering data from diverse sources such as databases, surveys, and websites.
- Data Cleaning: Ensuring data accuracy and consistency by removing errors and duplicates.
- Data Storage: Utilizing databases and data warehouses to store data securely.
- Data Analysis Techniques: Applying various techniques to extract insights effectively.
- Data Visualization: Representing data graphically to facilitate understanding and communication of information.
Each of these components plays a pivotal role in the analytics process, creating a framework that enables organizations to leverage their data effectively. Understanding these components is vital for any organization that wishes to harness the power of data analytics to drive growth and innovation.
The Importance of Data Analytics
The realm of data analytics stands at the core of contemporary business strategies and operational frameworks. Its significance is underscored by the rapid evolution of technology and the increasing volume of data generated every day. Organizations, across various sectors, leverage data analytics to extract meaningful insights, which directly influence decision-making processes, enhance efficiency, and act as a catalyst for innovation. The interplay between these elements reveals why data analytics has become indispensable in modern-day operations.
Decision Making Process
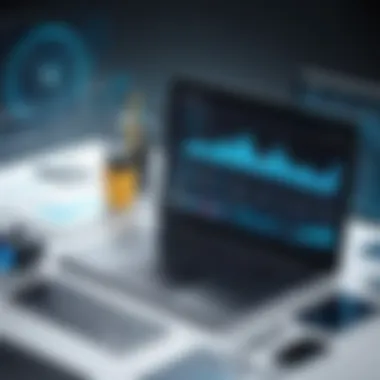
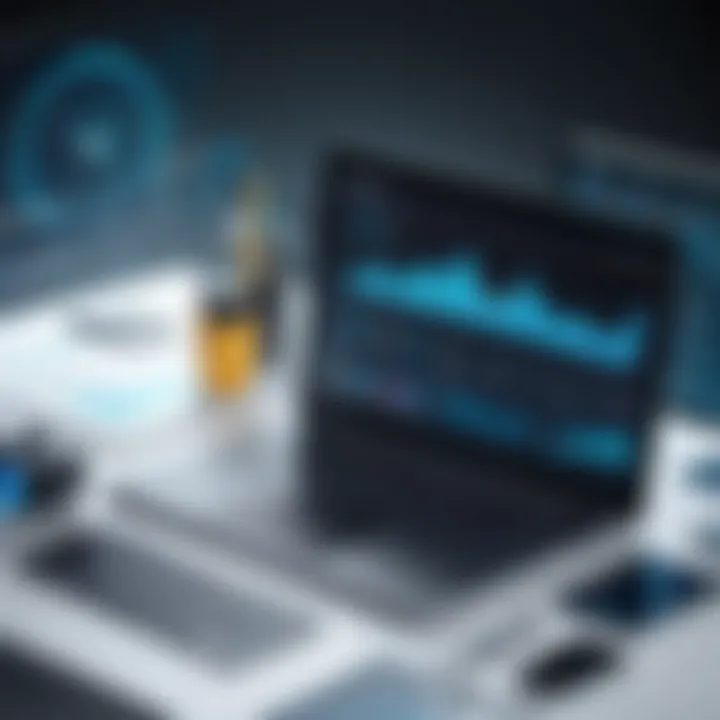
Data analytics plays a pivotal role in shaping informed decision-making. Businesses utilize analytical models to interpret data patterns and trends, enabling leaders to foresee potential market shifts and consumer behaviors. By accessing greater clarity through analytics, organizations can minimize risks and leverage opportunities more effectively. This data-driven approach facilitates strategic planning by providing actionable insights.
"Informed decisions are based on insights extracted from thorough analysis rather than intuition alone."
When decision-makers rely on verifiable data, they are more likely to achieve favorable outcomes. Companies develop scenarios and forecast results, which help weigh different choices systematically. The precision afforded by data analytics significantly reduces the margin of error in decisions, leading to better results.
Enhancing Operational Efficiency
Efficiency is essential in maintaining a competitive edge in today's fast-paced environment. Data analytics provides organizations the ability to streamline operations and optimize resource utilization. By analyzing workflow processes and identifying bottlenecks, companies can implement targeted improvements.
For example, tools like Tableau or Microsoft Power BI can aid in monitoring performance metrics, revealing areas of waste or inefficiency. Efficient operations lead to cost savings and improved service delivery, directly impacting customer satisfaction. The focus on enhancing operational efficiency through analytics creates a cycle of continuous improvement and innovation.
Driving Innovation
Innovation is key for growth. In an era where technology shapes consumer expectations, companies must adapt quickly. Data analytics not only identifies trends but also uncovers gaps in the market. By analyzing customer feedback and purchasing behaviors, companies can develop new products and services that meet evolving demands.
Data analytics fosters a culture of experimentation. Organizations can test hypotheses through A/B testing and assess results with sophisticated analytics. This iterative process allows for rapid prototype development, ensuring products are better aligned with consumer needs before a full rollout. Innovation driven by data is often more aligned with market realities, facilitating sustainable growth over time.
Processes Involved in Data Analytics
The processes in data analytics are fundamental to the understanding and application of this concept. These processes are crucial as they guide organizations in extracting value from vast amounts of data. By using systematic techniques, businesses can turn raw data into actionable insights. Effective data analytics involves several steps, each with its own importance, which contributes to the overall objectives of enhanced decision-making and strategic planning.
Data Collection
Data collection is the first step in the data analytics process. Organizations gather data from various sources, including surveys, customer transactions, and online interactions. This data might be quantitative or qualitative. The importance of data collection cannot be understated. Accurate and comprehensive data forms the foundation for subsequent analysis. Without quality data, the entire analytical process can lead to misleading results. Organizations need to employ robust methods to collect relevant data that aligns with their goals.
Data Cleaning and Preparation
After data collection, the next step is cleaning and preparation. This step ensures that the data is accurate, consistent, and usable. Often, data from different sources has missing values, duplicates, or errors. The process of cleaning involves identifying these issues and rectifying them. Data preparation further includes transforming the data into a suitable format for analysis. The significance of this step lies in improving the quality of the data, as cleaner data leads to more reliable analysis results. It requires attention to detail and an understanding of what constitutes clean data.
Data Analysis Techniques
Data analysis techniques encompass the various methodologies employed to process and interpret data. These techniques provide different perspectives and insights into the data. An analyst will typically select a technique based on the nature of the data and the questions they seek to answer. The three main analytical techniques are descriptive, predictive, and prescriptive analysis.
Descriptive Analysis
Descriptive analysis focuses on summarizing past data to identify patterns or trends. It answers the "what happened?" questions. One key characteristic of descriptive analysis is its simplicity, making it widely used in various sectors. This technique helps organizations comprehend historical performance, which is crucial for informed decision-making. A unique feature is its capacity to provide visual summaries, such as charts and dashboards. Its main advantage lies in its ability to offer quick insights, though it does not predict future events.
Predictive Analysis
Predictive analysis, as the name implies, looks ahead. It uses historical data to forecast future outcomes. The key characteristic that sets predictive analysis apart is its reliance on statistical techniques and algorithms to identify trends. In the context of this article, predictive analysis is beneficial because it helps organizations anticipate changes and make proactive decisions. The unique feature of predictive analysis is the use of machine learning models, which can continuously improve with new data. However, it can be complex and requires a robust data infrastructure to implement effectively.
Prescriptive Analysis
Prescriptive analysis goes a step further by suggesting actions based on data. It answers the question, "What should we do?" The key characteristic is its focus on making recommendations. This technique is beneficial in optimizing processes and decisions in business settings. A unique feature of prescriptive analysis is its integration with advanced algorithms and simulations which allow organizations to visualize the potential outcomes of different actions. The main advantage is its ability to guide strategic planning. However, implementing prescriptive analysis may demand extensive resources and expertise.
Data Visualization
Data visualization plays a critical role in the data analytics process. It refers to the representation of data in graphical formats. The purpose is to make complex data more understandable and accessible. Effective visualization can enhance comprehension and highlight important trends. Without it, the results of analysis can remain abstract and hard to interpret. Using tools like Tableau or Microsoft Power BI, organizations can create visual stories that communicate insights clearly, thus fostering better decision-making.
Applications of Data Analytics
Data analytics transforms raw data into meaningful insights. Its applications span a wide range of industries, revealing its pivotal role in making informed decisions and optimizing processes. The importance of data analytics in this context cannot be overstated. It touches on aspects such as competitive advantage, enhanced customer experience, and more efficient operations.
In Business
Customer Insights
Customer insights are one area where data analytics shines prominently. Understanding customers' behaviors, preferences, and needs allows businesses to tailor their offerings more effectively. This aspect contributes significantly to overall revenue enhancement and customer satisfaction. A key characteristic of customer insights is their ability to provide detailed profiles and segmentation of consumers. Businesses can identify trends and patterns that often go unnoticed. This can lead to more personal marketing strategies, which are increasingly in demand.
An unique feature of customer insights is how they can predict future behaviors. For example, predictive analytics models can suggest which customers are likely to churn or which products may interest certain segments. This decision-making tool provides a significant edge, yet it also has drawbacks. Over-reliance on data may cause businesses to overlook human factors that cannot be quantified.
Market Trends Analysis
Market trends analysis captures the heartbeat of an industry. By utilizing data analytics, businesses can detect shifts in market dynamics. This understanding aids in strategic positioning and competitive actions. The main aspect of market trends analysis is its focus on large datasets to reveal patterns that inform marketing strategies and product development.
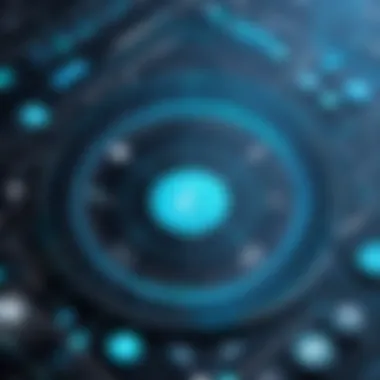
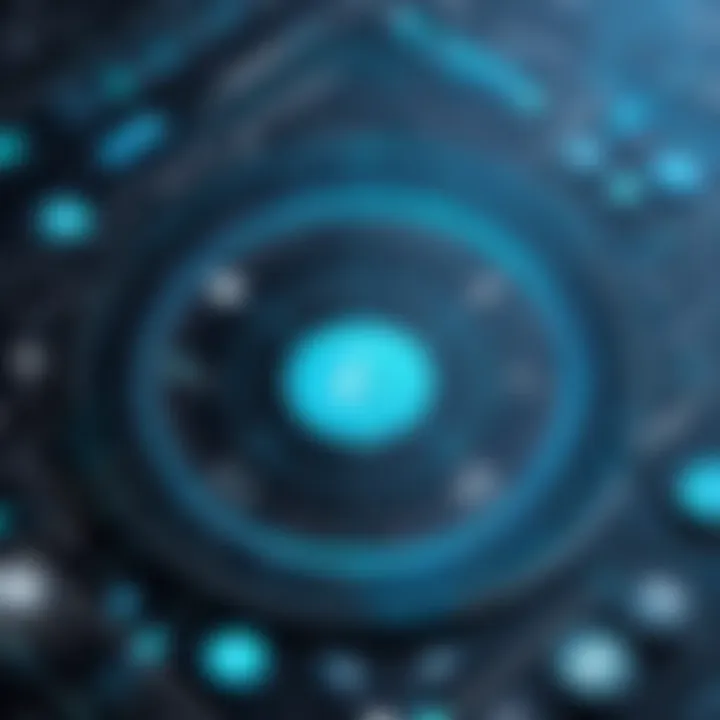
One key feature of this analysis is the ability to perform real-time assessments of market conditions. Companies can quickly adapt to changes, potentially increasing their market share. However, one potential pitfall is the risk of misinterpreting data. Without proper context, decisions based solely on data trends could misfire and result in losses.
In Healthcare
Patient Data Management
Patient data management is crucial for maintaining the quality of care. Efficiently organizing and analyzing patient records lead to better clinical outcomes. One fundamental aspect here is the integration of various data points, from demographics to medical history. This integration allows healthcare practitioners to provide personalized care.
The unique feature of patient data management systems often lies in their usability. They enable healthcare providers to access critical information swiftly. Yet, challenges arise with data security and privacy concerns, which must be managed carefully to ensure patient trust and compliance.
Predictive Healthcare Analytics
Predictive healthcare analytics enhances patient care by using historical data to forecast future health trends. This is particularly significant for managing chronic diseases. A key characteristic of predictive analytics in healthcare is its proactive approach. Instead of just reacting to health issues, providers can anticipate them.
This feature allows for targeted interventions, potentially reducing the need for emergency care. However, the reliance on predictions can lead to potential ethical considerations. Patients may feel uncomfortable with their data being used to make assumptions about their health without their explicit consent.
In Finance
Risk Assessment
Risk assessment utilizes data analytics to evaluate the potential risks associated with investments and financial decisions. It is vital for making informed choices in a volatile market. One key characteristic is the use of sophisticated models that analyze various factors affecting risk.
These models can highlight which investments might be prone to loss under certain conditions. The unique feature of risk assessment is its ability to quantify risks, making them more understandable. However, models are only as good as the data fed into them. Flawed data can lead to poor risk evaluations.
Fraud Detection
Fraud detection is another critical application of data analytics in finance. Institutions use analytics to identify suspicious transactions in real time. The specificity of fraud detection tools lies in pattern recognition capabilities that flag unusual transactions.
This enables prompt action against potential fraud. Yet, there are challenges; too many false positives can drain resources. Financial institutions must fine-tune their algorithms to strike a balance, ensuring efficiency without losing sight of genuine customer interactions.
In Education
Learning Analytics
Learning analytics focuses on analyzing student data to enhance learning outcomes. It helps educators understand how students engage with course materials. A key characteristic of learning analytics is its feedback loop. Educators can modify their teaching strategies based on data trends collected about student performance.
The unique feature of learning analytics is its adaptability. It offers personalized learning paths that cater to individual student needs. However, concerns about data privacy can limit its effective implementation in educational settings, often creating hesitancy in data sharing.
Student Performance Evaluation
Student performance evaluation utilizes data to assess how well students are doing academically. This aspect is essential in identifying students who may need additional support. One main characteristic is the comprehensive approach it takes in analyzing various metrics, from test scores to participation.
Uniquely, it can provide insights not only into individual performance but also on broader educational trends. However, relying solely on data might overlook qualitative aspects of education, such as creativity, which cannot be easily assessed through traditional metrics.
"Data analytics is not just about technology; it encompasses the ability to interpret and use data for better decision-making across various fields."
In summary, the applications of data analytics breathe life into many industries. Each sector, whether it be business, healthcare, finance, or education, finds unique ways to utilize analytics, demonstrating its expansive relevance and importance.
Technologies Used in Data Analytics
The landscape of data analytics is increasingly shaped by various technologies. These technologies serve as the backbone for processing, analyzing, and visualizing vast amounts of data. Leveraging the right tools enhances decision-making and operational efficiency across various sectors. Understanding these technologies is crucial for professionals seeking to harness the power of data in today's competitive environment.
Data Analysis Software
Data analysis software is essential for effectively managing data analytics. Such software tools offer functionalities for data manipulation, exploratory analysis, and visualization. Popular examples include Microsoft Excel, Tableau, and R. These platforms allow users to:
- Import and clean data from various sources.
- Apply statistical methods to derive insights.
- Create visual representations to simplify communication of findings.
Excel is often the starting point due to its accessibility and familiarity. However, as data complexity grows, tools like Tableau enable more advanced visualization capabilities. They allow for interactive dashboards that help stakeholders grasp key metrics and trends quickly.
Big Data Technologies
Big data technologies address the challenges associated with processing large and complex data sets. The term "big data" refers to data that is too vast or complex for traditional data-processing applications. Technologies like Apache Hadoop and Apache Spark play a significant role in this domain. They provide the infrastructure needed for distributed storage and parallel processing, which optimizes the way data is handled.
Key benefits of big data technologies include:

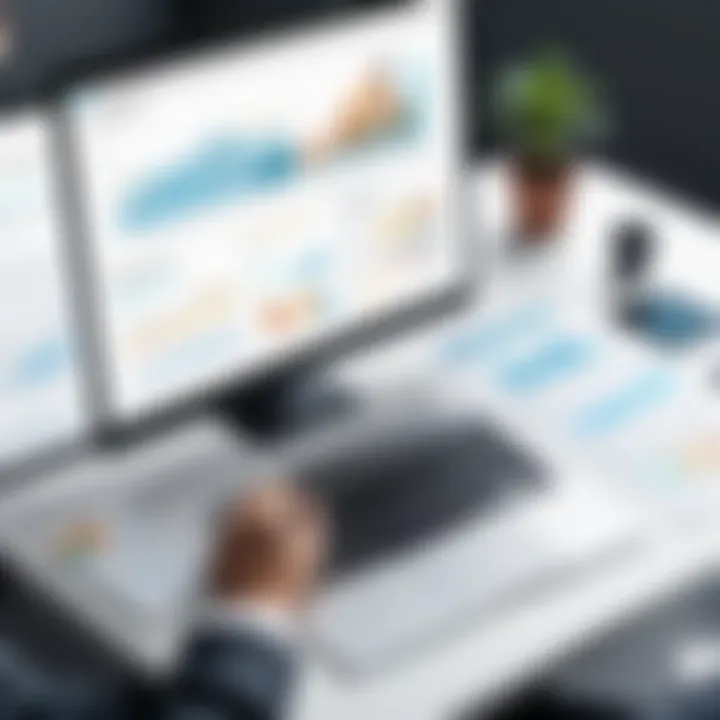
- Scalability: They can handle increasing volumes of data.
- Cost Efficiency: Distributed storage options reduce the overall storage costs.
- Real-Time Processing: Technologies like Apache Kafka enable real-time data streaming and processing.
By using these technologies, organizations can derive actionable insights from real-time data, assisting in timely decision-making.
Machine Learning Algorithms
Machine learning algorithms are at the forefront of advanced data analytics. They enable predictive analytics, which is essential in various applications from finance to healthcare. These algorithms learn from existing data to recognize patterns and predict future trends. Common machine learning algorithms include regression analysis, decision trees, and neural networks.
Utilizing machine learning has several benefits:
- Automation of Predictions: Algorithms can automatically refine their predictions as more data becomes available.
- Improved Accuracy: As these systems learn from past data, they can enhance the accuracy of predictions over time.
- Personalization: Businesses can tailor their offerings based on predictive insights, leading to improved customer satisfaction.
Incorporating machine learning into data analytics processes transforms data from mere numbers into actionable insights. These insights can guide strategy, enhance user experience, and ultimately drive organizational growth.
Understanding the technologies used in data analytics is vital. It equips professionals with the necessary tools to transform raw data into meaningful insights, facilitating strategic decision-making that aligns with organizational goals.
Challenges of Data Analytics
Data analytics is a powerful tool in various sectors, unlocking insights and enhancing decision-making. However, it is not without its challenges. Addressing these obstacles is crucial to fully harnessing the potential of data analytics. In this section, we will explore three primary challenges: data privacy and security concerns, data quality issues, and the skill gap in professionals.
Data Privacy and Security Concerns
With the surge of data collection, data privacy and security have emerged as significant concerns. Organizations often gather personal information from customers, which can expose them to breaches if not handled correctly. Regulations, such as the General Data Protection Regulation (GDPR), have set stringent guidelines on data handling and storage.
Ensuring data privacy involves implementing measures like encryption and access controls. In addition, organizations must establish clear policies regarding how data is used and shared. Data leaks can damage a company's reputation, lead to legal ramifications, and ultimately erode customer trust. Therefore, prioritizing data security is not just a legal responsibility; it is key to maintaining a competitive edge in the market.
Data Quality Issues
The effectiveness of data analytics largely depends on data quality. Poor-quality data can lead to inaccurate insights and flawed decision-making. This problem arises from various factors, such as incorrect data entry, lack of standardization, or outdated information. For example, if a business relies on outdated sales data, its strategies may not align with current market demands.
Maintaining high-quality data necessitates robust data cleaning processes. Organizations should adopt automated tools that can identify and correct erroneous entries and standardize data formats. Moreover, establishing a culture of data governance within the organization is essential. By doing so, businesses can streamline their analytics efforts and ensure that the insights derived are reliable.
Skill Gap in Professionals
As data analytics continues to evolve, there is an increasing demand for skilled professionals in the field. However, a significant skill gap exists. Many organizations face challenges in finding individuals who possess the necessary technical expertise and analytical skills. This shortage can hinder the successful implementation of data analytics initiatives.
To address this gap, organizations should invest in training programs for their existing workforce. Encouraging employees to pursue certifications in data analytics can also help bridge the skills divide. As data analytics becomes more integrated into business processes, equipping professionals with the right knowledge will be critical. This investment will not only enhance internal capabilities but also strengthen overall data-driven strategies.
"The journey of harnessing data analytics begins by recognizing and addressing its challenges, paving the way for more informed and effective decision-making."
Future Trends in Data Analytics
Data analytics is a rapidly evolving field that profoundly impacts various sectors. Understanding the future trends in data analytics is essential, as these trends shape the direction of technology and the choices that companies make. They influence how organizations collect, process, and leverage data to drive results. Keeping an eye on these trends can position businesses to adapt and optimize their operations effectively.
Integration with AI and Machine Learning
The integration of data analytics with artificial intelligence (AI) and machine learning is a significant trend shaping the landscape. This collaboration allows organizations to analyze larger datasets more efficiently and derive meaningful insights. AI algorithms can identify patterns within data that might go unnoticed using traditional analysis methods.
Businesses can leverage these insights for various applications like customer personalization, targeted marketing, and operational improvements. For example, retail companies like Amazon utilize AI-driven analytics to forecast customer preferences, optimizing their product recommendations and inventory management.
The continuous improvement of machine learning models indicates that this aspect will grow stronger. Enhanced predictive capabilities can lead to smarter decision-making processes. Companies investing in this integration can gain a competitive advantage by staying ahead of market trends.
Real-time Data Processing
Real-time data processing is another compelling trend that ensures businesses stay agile and responsive. As the volume of data increases, the necessity for timely insights becomes paramount. Real-time processing enables organizations to analyze data as it is collected, rather than after the fact.
For instance, social media platforms like Facebook rely heavily on real-time data to monitor user engagement and interactions. The ability to respond promptly to trends or issues is vital in today’s fast-paced environment. Companies can adjust marketing strategies, respond to customer feedback, and even detect fraud or security breaches in real-time, minimizing risks and maximizing opportunities.
Implementing real-time data analytics can be challenging but rewarding. Businesses must invest in robust infrastructure and data management practices to harness the full potential of this trend.
Enhancing Predictive Analytics
Enhancing predictive analytics continues to be a focal point for organizations aiming to forecast future trends and behaviors. Predictive analytics involves using historical data and statistical algorithms to predict future outcomes. With advancements in technology and more sophisticated analytical tools, the accuracy of these predictions has significantly improved.
Industries such as finance and healthcare particularly benefit from enhanced predictive analytics. Financial institutions analyze market trends, customer behavior, and risk factors to make informed decisions. Similarly, healthcare providers apply predictive models to anticipate patient needs, improving outcomes and resource allocation.
Organizations are now also combining predictive analytics with prescriptive analytics, which suggests optimal actions based on data insights. This dual approach can help companies devise strategies that are both data-driven and actionable.
"Investing in enhanced predictive analytics is not just about understanding the past and present but about preparing for the future."
As trends continue to evolve, companies in various sectors should remain vigilant. Staying informed about future trends in data analytics can enhance their ability to harness data meaningfully. Such foresight is paramount in a world increasingly defined by data-driven decisions.