Understanding Data Analytics: Concepts and Applications
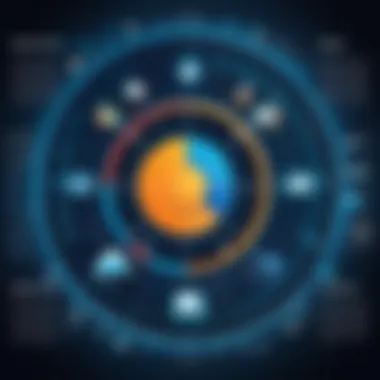
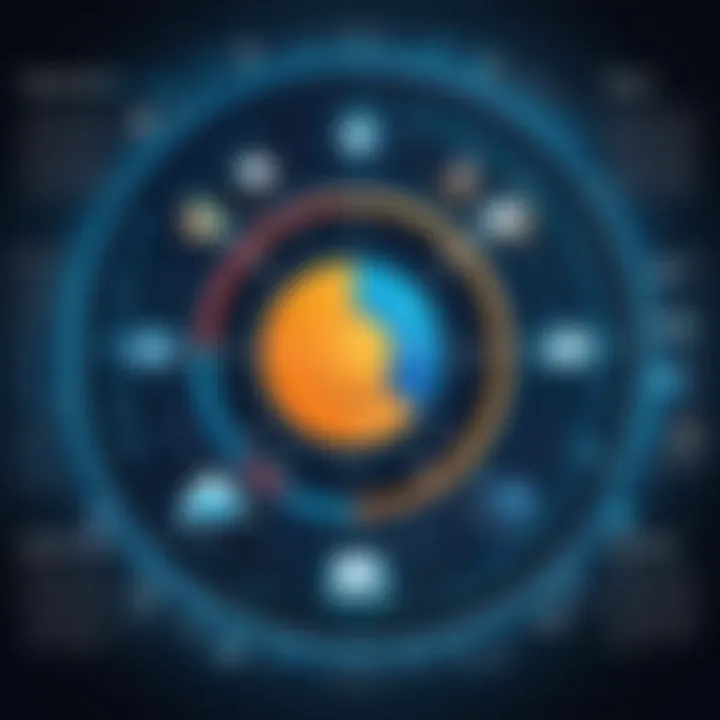
Intro
Data analytics has become a cornerstone in the evolution of contemporary technology. Organizations are increasingly relying on data to steer decision-making processes. This article aims to elucidate the key concepts, methodologies, and tools surrounding data analytics. Furthermore, it inspects how various industries harness these elements for optimal results.
The scope of data analytics stretches across many sectors. Companies from healthcare to finance utilize analytics to interpret vast amounts of information. In doing so, they can make informed decisions that influence their operations and strategies.
Through an exploration of real-world applications, this article seeks to highlight the significance of data analytics in shaping modern technology. It will also provide insights regarding the various types of data analytics, the challenges professionals face, and the trends that are shaping the field.
Tech Trend Analysis
Overview of the current trend
Data analytics is witnessing rapid transformation, driven largely by advancements in technology. Machine learning and artificial intelligence are playing crucial roles in processing and analyzing data. This integration facilitates the extraction of valuable insights from large datasets, which were once considered cumbersome to manage.
Implications for consumers
For consumers, these trends mean more personalized services and products. Companies can leverage analytics to understand customer preferences deeply. As a result, consumers experience tailored marketing strategies that enhance engagement.
Future predictions and possibilities
Looking ahead, we can expect an expansion of data analytics methodologies. The rise of real-time analytics will support faster decision-making. In addition, the integration of predictive analytics will empower businesses to forecast future trends accurately. These possibilities present a compelling landscape for organizations that wish to maintain a competitive edge.
"The future of data analytics lies in its ability to transform raw data into actionable insights at unprecedented speeds."
Key Trends to Watch
- Increased Automation: Firms are automating data collection and analysis processes, reducing human error and improving efficiency.
- Enhanced Data Security: As data privacy becomes paramount, sectors are investing more in data protection technologies.
- Cloud-Based Solutions: The movement towards cloud technology allows for scalable storage and processing capabilities, making data analytics more accessible to all industries.
By understanding these trends, businesses can better position themselves to exploit the potential of data analytics effectively.
Preamble to Data Analytics
Data analytics plays a crucial role in the modern world, where the sheer volume of data generated daily has reached unprecedented levels. Understanding the core principles of data analytics allows professionals to extract relevant information from this data deluge, thus enabling informed decision-making. In this section, we provide insight into what data analytics entails and highlight its significance in various sectors.
What is Data Analytics?
Data analytics refers to the systematic computational analysis of data. It involves methods and technologies that allow organizations to collect, process, and analyze data to make informed decisions. The process often includes various steps, starting from data collection to data visualization. Each aspect of data analytics contributes to revealing patterns, trends, and relationships hidden within the data.
To understand it further, consider the following components involved in data analytics:
- Data Collection: Gathering data from multiple sources, such as surveys, databases, and sensors.
- Data Processing: Cleaning and organizing data to ensure accuracy and consistency.
- Data Analysis: Employing statistical techniques and models to interpret data.
- Data Visualization: Presenting data in graphical formats like charts and graphs for easier comprehension.
This comprehensive approach to data enables organizations to derive insights and drive actionable strategies based on solid evidence.
Importance of Data Analytics in Today’s World
The impact of data analytics cannot be overstated. Organizations across various sectors leverage data analytics to gain a competitive edge. Here are key points illustrating its importance:
- Informed Decision-Making: By analyzing data, businesses can make better decisions, reduce risks, and optimize operations.
- Efficiency: Data analytics identifies inefficiencies in processes, allowing for quick adjustments that lead to better resource management.
- Customer Insights: Analyzing customer data helps companies understand behaviors and preferences, enhancing customer relationship strategies.
- Innovation: Data can reveal gaps in the market or new trends, driving innovation in products and services.
- Predictive Analysis: Companies use data analytics to forecast future trends, which is vital for strategic planning.
"Data analytics drives significant value from large amounts of data, turning raw information into a strategic asset for organizations."
The ability to interpret data accurately has transformed industries. Thus, data analytics is not merely a technical process; it is an essential driver of progress and innovation in today’s data-centric world.
Core Concepts of Data Analytics
Data analytics is a multifaceted field, and grasping its core concepts is essential for effective application in various industries. Understanding these concepts is fundamental for anyone interested in leveraging data for informed decision-making. Core concepts include data collection, processing, and analysis techniques. Each plays a unique role in the analytics workflow, ensuring that valuable insights can be derived from raw data.
Data Collection
Data collection serves as the foundation of data analytics. It involves gathering information from various sources, which might include surveys, digital transactions, sensor outputs, or social media interactions. The quality and relevance of collected data significantly impact the final analysis.
Companies must use proper methodologies to obtain accurate data, minimizing bias and errors. Techniques like web scraping, APIs, and manual entry come into play here. Each method has its benefits and drawbacks. It's crucial that organizations prioritize systems that ensure data integrity. Without well-collected data, the rest of the analytics process is compromised.
Data Processing
Once data is collected, it must be processed. This involves cleaning and organizing the data to make it suitable for analysis. Processing may include removing duplicates, addressing missing values, and standardizing formats. Tools like Microsoft Excel and Python libraries such as Pandas are frequently used for these tasks.
Effective data processing ensures that the analysis is based on sound data. Poorly processed data can lead to erroneous conclusions, which might result in misguided business decisions. Thus, investing time and resources in data processing is critical.
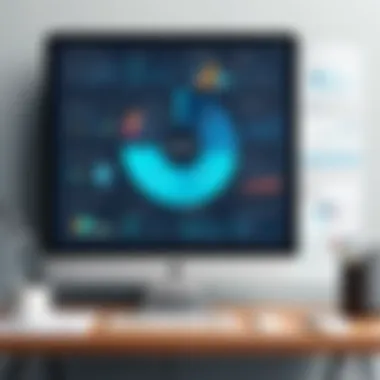
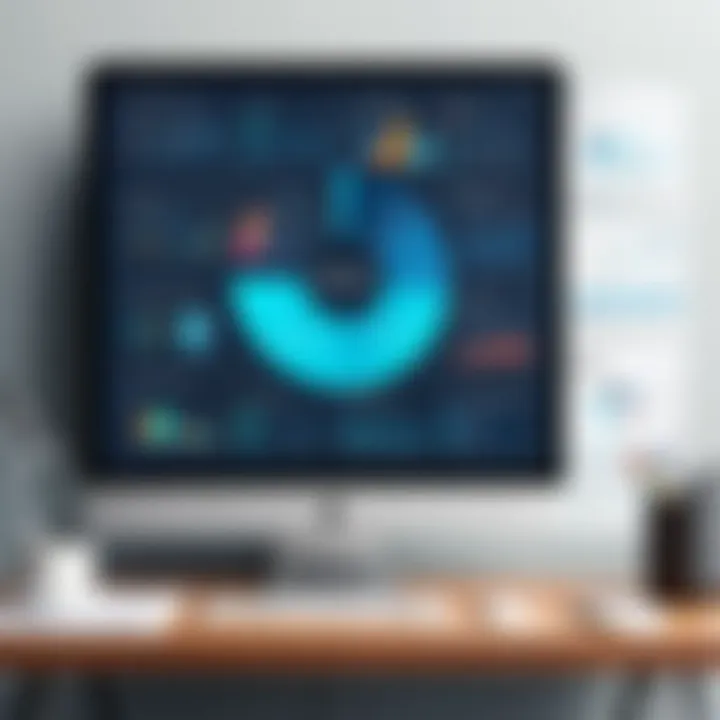
Data Analysis Techniques
Data analysis techniques form the method through which insights are gained from data. There are various types, each serving distinct purposes, which enhance data-driven decision-making.
Descriptive Analysis
Descriptive analysis focuses on summarizing historical data. It provides insights into what has happened over a specific period. Key characteristics of this technique include its straightforward approach and ability to provide a clear snapshot of past performance. This makes it a beneficial choice for organizations trying to understand trends or behaviors in their data.
A unique feature of descriptive analysis is its use of visualization tools like bar charts or line graphs, which simplify complex datasets for better comprehension. However, it does not explain why something happened, limiting its depth.
Diagnostic Analysis
Diagnostic analysis digs deeper than descriptive analysis. It seeks to identify causes behind observed outcomes. By employing techniques such as correlation and regression analysis, it helps organizations determine the underlying factors that led to specific results.
This technique is popular because it enables insights into causation rather than merely correlation. However, its unique feature lies in its complexity, as it requires a higher level of statistical knowledge which could be a barrier for some users.
Predictive Analysis
Predictive analysis is forward-looking. It employs historical data and statistical algorithms to forecast future outcomes. This type is increasingly valuable in sectors like finance and retail, where anticipating trends can drive competitive advantage. One of its key characteristics is the utilization of machine learning models.
Predictive analysis stands out for its ability to inform strategic planning. However, reliance on past data carries inherent risks; if patterns do not repeat, predictions may fail. Thus, it demands continuous validation.
Prescriptive Analysis
Prescriptive analysis provides recommendations based on predictive outcomes. It not only suggests possible actions but also evaluates their potential impact. This method combines data analytic techniques to present options. Its key characteristic is helping decision-makers navigate complex scenarios by considering various variables and potential outcomes.
While this type achieves significant depth in insights, it is notably intricate due to the required computational resources and expertise. Therefore, organizations may face challenges around implementation and interpretation, making thorough training essential.
"Data analytics transforms raw data into actionable insights, materially influencing strategic directions and operational efficiencies across industries."
In summary, understanding these core concepts equips individuals and companies to harness the full potential of data analytics. Each analytical technique has distinct roles, but all are essential for drawing meaningful conclusions from data.
Methodologies in Data Analytics
Methodologies in data analytics provide a structured framework for analyzing data effectively. Understanding these methodologies is essential for deriving valuable insights from data. They encompass a variety of techniques and practices that guide organizations in making informed decisions. This section delves into three key methodologies: quantitative and qualitative analysis, statistical methods, and the role of machine learning.
Quantitative vs. Qualitative Analysis
Quantitative and qualitative analyses serve distinct purposes in data analytics. Quantitative analysis focuses on numerical data. It involves statistical techniques to analyze trends, patterns, and relationships. This method is beneficial for organizations seeking measurable results. For example, a retail company may use quantitative data to track sales performance over time.
On the other hand, qualitative analysis explores non-numerical data. It seeks to understand underlying motivations and behaviors. Interviews and surveys are common tools used in qualitative analysis. These insights can help organizations tailor strategies to meet consumer needs.
In summary, both approaches are valuable. Quantitative analysis provides a solid foundation for decision-making, while qualitative analysis adds context and depth to the findings.
Statistical Methods in Data Analytics
Statistical methods are at the core of data analytics. These techniques enable analysts to make sense of complex datasets. They help in making predictions and identifying trends. Some common statistical methods include regression analysis, hypothesis testing, and correlation analysis.
Regression analysis assesses the relationship between variables. For instance, a business could use regression to understand how advertising spend impacts sales. Hypothesis testing helps researchers validate assumptions about datasets. For example, an analyst might test if a new product increases sales compared to an existing one.
Correlation analysis explores the relationship between two variables. Understanding this can aid in detecting patterns. For instance, an e-commerce company might study how weather conditions affect online purchasing behaviors.
Machine Learning in Data Analytics
Machine learning represents a significant advancement in data analytics. It involves algorithms that improve automatically through experience. Machine learning enables systems to learn from data and make predictions without explicit programming.
In the context of data analytics, machine learning can be applied in various ways. For instance, in the finance sector, machine learning algorithms are used for credit scoring. They analyze vast datasets to evaluate risks efficiently.
Another application is in marketing, where predictive analytics forecasts customer behaviors. By analyzing buying patterns, businesses can create targeted marketing campaigns.
The integration of machine learning enhances data analysis capabilities. It enables businesses to process large volumes of data swiftly. This capability improves accuracy and supports better decision-making.
Tools and Technologies for Data Analytics
In the expanding realm of data analytics, the selection of appropriate tools and technologies is crucial. These tools not only enhance the efficiency of data processing but also empower users to derive valuable insights from complex datasets. As data becomes increasingly central to decision-making processes, leveraging the right technologies can significantly amplify the effectiveness of analytics efforts.
Data Visualization Tools
Data visualization tools play a vital role in transforming raw information into comprehensible visual formats. Tools such as Tableau, Power BI, and Google Data Studio are commonly used for creating interactive dashboards and detailed reports. These platforms facilitate a better understanding of data trends and patterns.
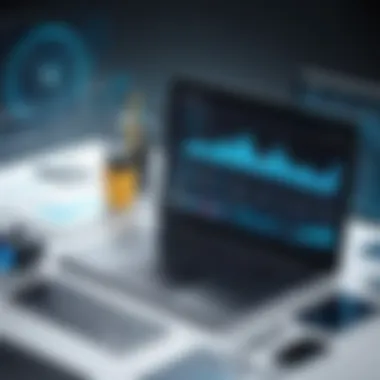
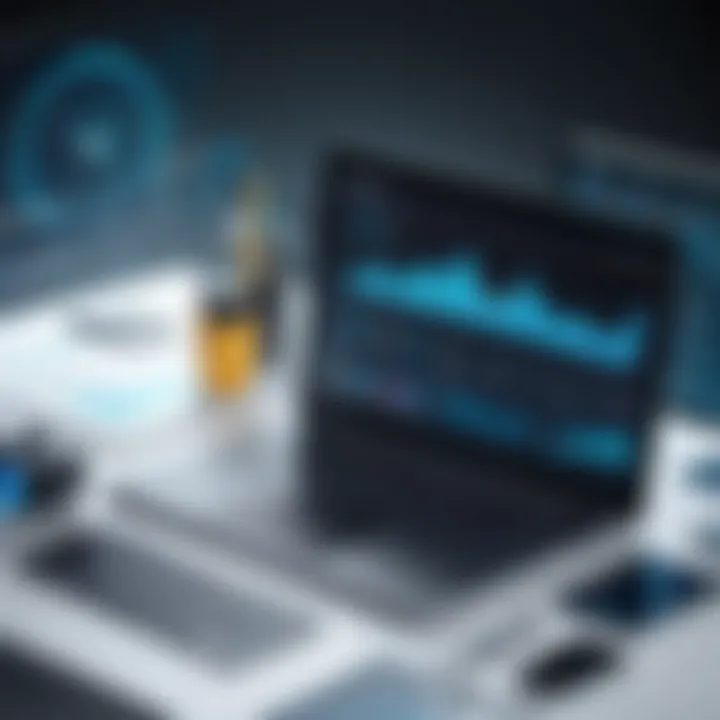
Effective data visualization can help stakeholders grasp complex data relationships without delving into extensive statistical analysis. Furthermore, visuals simplify the communication of findings across diverse audiences, making the information accessible even to those without a technical background. Greater accessibility results in more informed decision-making, as stakeholders can quickly interpret visual data and apply it to their business strategies.
Considerations when selecting data visualization tools include:
- User-friendliness: The tool should be intuitive, allowing users to easily create visualizations without needing extensive training.
- Integration capability: Analysis can be hampered if tools lack compatibility with existing data management systems, so seamless integration with other software is essential.
- Real-time data updates: For businesses that rely on up-to-date information, tools that offer real-time updating features should be prioritized.
Data Management Software
Data management software is foundational for the effective handling of data throughout its lifecycle. Tools like Microsoft SQL Server, Oracle Data Management, and MongoDB provide robust solutions for storing, retrieving, and manipulating large quantities of data efficiently.
The need for quality data management practices cannot be overstated. Businesses generate massive amounts of data, and without proper management, this data can become unwieldy and obscure valuable insights. Good data management software offers:
- Structured storage: Structured data ensures that information is organized and easily accessible, promoting speed in data retrieval.
- Data integrity: It's pivotal to maintain accurate data through validation processes, which management software typically provides.
- Scalability: As businesses grow, so does their data. Scalable solutions accommodate increased data loads without performance degradation.
In summary, the significance of tools and technologies in data analytics cannot be overlooked. Effective data visualization enhances comprehension and communication, while robust data management software ensures quality and accessibility. By focusing on these two critical areas, organizations can better navigate the complexities of data analytics and unearth actionable insights.
Applications of Data Analytics Across Industries
The impact of data analytics spans multiple industries, reflecting its versatility and essential role in modern decision-making processes. By comprehending its applications, organizations can harness data as a powerful resource. Data analytics contributes not only to enhance efficiency but also to foster innovation and competitive advantage. Each sector utilizes data analytics uniquely to derive meaningful insights and drive outcomes based on facts rather than intuition.
Healthcare Sector
In the healthcare sector, data analytics is revolutionizing patient care and operational efficiency. By analyzing vast amounts of medical data, healthcare providers can identify trends, manage resources effectively, and improve patient outcomes. Hospitals leverage predictive analytics to anticipate patient admissions, which helps optimize staffing and resource allocation.
Moreover, by integrating data from electronic health records, wearables, and other sources, healthcare professionals can monitor patient health more proactively. For instance, data analytics enables physicians to detect early signs of diseases through pattern recognition, improving early intervention strategies.
Any changes to patient care plans can be continually monitored using feedback loops generated through analytics, ensuring personalized treatment plans based on real-time data. This approach enhances patient satisfaction and leads to cost reduction in long-term care.
Finance Industry
In the finance industry, data analytics serves as a cornerstone for risk management and fraud detection. The ability to analyze transaction data allows financial institutions to identify unusual patterns in real-time, which is crucial for minimizing potential losses.
Risk assessment models depend heavily on historical and predictive analysis to forecast potential defaults on loans or investments. This model aids in tailoring financial products that meet customer needs while managing risks effectively. For example, algorithms can evaluate a customer's creditworthiness by analyzing their financial history, enabling quicker and more accurate loan decisions.
Furthermore, trading firms harness data analytics for high-frequency trading through algorithmic strategies. By processing market data rapidly, they can exploit minute price discrepancies, enhancing profitability.
Retail and E-commerce
The retail and e-commerce sectors benefit deeply from data analytics, particularly through understanding customer behaviors and preferences. Retailers collect data on purchasing patterns, inventory levels, and customer feedback. By analyzing this data, they can tailor marketing strategies and optimize inventory management.
For instance, personalized promotions can be developed based on shopping habits, leading to increased customer engagement and retention. E-commerce platforms like Amazon extensively use data analytics to recommend products based on previous searches and purchases. This not only enhances the customer experience but also drives sales.
Another critical application lies in supply chain management, where data analytics helps in forecasting demand, which minimizes overproduction and stockouts.
Manufacturing and Supply Chain
In manufacturing and supply chain management, data analytics drives efficiency and innovation. Using IoT devices, manufacturers can gather real-time data from machinery, helping to predict maintenance needs and reduce downtime.
Predictive analytics allows those in manufacturing to monitor production processes and quality control, effectively ensuring that products meet the desired standards. This decreases waste and increases productivity. Manufacturers also utilize analytics to streamline supply chain operations, identifying bottlenecks, optimizing routes, and reducing costs.
As companies aim for agile methodologies, incorporating data analytics enables improved decision-making throughout the supply chain, from procurement to delivery. This holistic approach forms the basis for future-ready manufacturing practices.
Key Takeaway: The integration of data analytics across diverse sectors demonstrates its vital role in driving efficiency, enhancing decision-making, and fostering innovation.
Challenges in Data Analytics
In the field of data analytics, professionals face various challenges that can hinder the effectiveness of their work. Understanding these challenges is crucial for anyone involved in data-driven decision-making. Each challenge offers unique obstacles, but they also unveil opportunities for improvement and innovation. By being aware of these issues, organizations can better prepare for the demands of modern analytics and maximize the value derived from their data.
Data Quality Issues
Data quality stands as a primary concern in data analytics. Poor quality data can lead to misleading conclusions and poor decision-making. Factors such as missing values, inaccuracies, and inconsistencies can significantly affect the outcomes of analyses.
- Sources of Data Quality Issues:
- Inconsistent data entry processes.
- Faulty data collection methods.
- Lack of standardization across databases.
Organizations must implement strict data governance practices to ensure accurate and reliable data. Techniques for improving data quality include regular audits, cleansing processes, and employing validation techniques at the point of entry. Without addressing data quality issues, the credibility of analyses and reports becomes questionable.
Privacy and Ethical Considerations
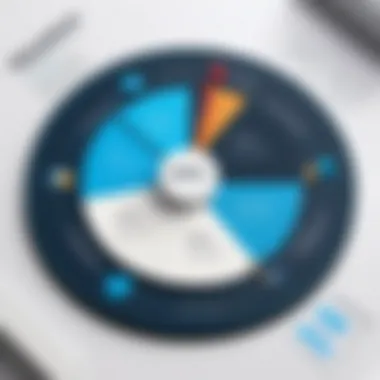
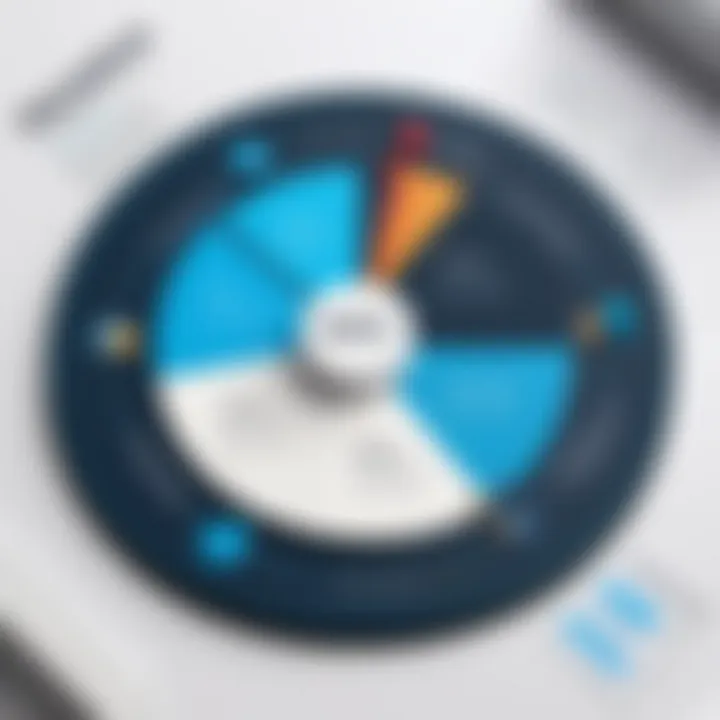
Privacy and ethical considerations are paramount in data analytics. As organizations gather immense amounts of data, protecting user information has become a pressing concern. This attention stems from legal requirements and ethical obligations to uphold user privacy.
Key considerations include:
- Compliance with Regulations:
- User Awareness:
- Familiarity with GDPR and other privacy laws is essential.
- Consumers are increasingly aware of how their data is used. Organizations must be transparent about data usage.
Failure to address privacy concerns can result in legal repercussions and damage to an organization's reputation. It is vital to balance the benefits of data analytics with ethical standards, ensuring user trust and compliance with regulations.
Integration with Existing Systems
Integration of data analytics tools with existing systems poses another significant challenge. Many organizations have legacy systems that may not be compatible with newer analytics platforms. This incompatibility can create data silos, complicating the analysis process.
- Challenges to Integration:
- Disparate data formats.
- Resistance from employees accustomed to old systems.
- Resource constraints preventing updates or overhauls.
To mitigate these integration issues, organizations can adopt cloud-based solutions that offer greater flexibility and scalability. Additionally, investing in proper training for employees can ease the transition to new systems. Addressing integration challenges can lead to a more cohesive data analytics strategy and improved access to insights.
In summary, recognizing and addressing these challenges in data analytics is crucial for optimizing data usage in today’s competitive landscape. Ensuring data quality, respecting privacy, and facilitating system integration can significantly enhance decision-making processes.
The Future of Data Analytics
Data analytics continues to evolve, making it an important area of study and practice for many industries. As the collection and storage of data grow exponentially, so does the need to analyze it effectively. The future of data analytics will play a crucial role in shaping business strategies, technological innovations, and consumer experiences.
The dynamics of data analytics are changing as new techniques, tools, and philosophies emerge. Organizations must adapt to remain competitive and meet the needs of their clients. In this rapidly changing landscape, understanding the future of data analytics involves recognizing the trends that are driving innovation and efficiency.
Emerging Trends
The future holds several significant trends in data analytics that are gaining traction. These trends include:
- Automation and AI Integration: More businesses are utilizing automation to streamline data collection and analysis processes. This shift allows for faster insights and reduced human error.
- Real-time Analytics: The demand for real-time data insights is on the rise, enabling organizations to make timely decisions based on current data.
- Data Democratization: Companies are breaking down barriers by making data accessible to more employees. This trend fosters a data-driven culture where more team members can contribute to decision-making based on analytics.
- Edge Analytics: Edge computing allows data processing closer to data sources. This method improves response times and reduces data transmission costs.
These emerging trends reflect a gradual shift towards more sophisticated and accessible data analytics. Organizations that stay ahead of these trends can leverage unique insights to drive innovation and efficiency.
Impact of Artificial Intelligence
Artificial Intelligence is profoundly transforming data analytics. The integration of AI technologies allows for advanced analytic capabilities that were previously unattainable. AI models can sift through vast amounts of data, identify patterns, and generate insights much faster than a human analyst could.
Furthermore, machine learning algorithms improve over time, enhancing prediction accuracy. Businesses can make better forecasts about customer behavior, market movements, and operational efficiencies, all contributing to informed decision-making.
However, reliance on AI also raises questions about transparency and accountability. It is crucial for organizations to maintain a balance between automated insights and human oversight to ensure reliability and ethical use of AI in decision-making.
The Role of Big Data
Big Data serves as both a catalyst and a challenge in the field of data analytics. The sheer volume of data generated today allows organizations to uncover insights that were not possible before. Companies can use big data analytics to understand complex trends and patterns in their operations and market environments.
Moreover, tools for processing big data continue to improve. Technologies like Apache Hadoop and Spark facilitate efficient data management. Companies can harness big data to enhance customer experiences and operational efficiencies.
However, managing large datasets presents challenges related to data quality, security, and privacy. Organizations must prioritize robust strategies to address these issues, as they can significantly affect analytical outcomes.
"The future of data analytics is bright, but organizations must navigate the complexities of technology and ethics to fully benefit from its potential."
In summary, the future of data analytics promises many opportunities driven by emerging trends, AI advancements, and the power of big data. By recognizing and adapting to these changes, organizations can ensure they remain competitive in a data-driven world.
Closure
The conclusion serves a pivotal role in this article, emphasizing the multifaceted aspects of data analytics. It not only ties together essential concepts outlined throughout the text but also reinforces how these concepts impact various sectors today. Understanding these elements is vital for anyone looking to leverage data-driven decision-making, as analytics continues to evolve.
In summarizing the main ideas, the reader can reflect on the significance of data collection, processing, and analysis techniques. Each of these components builds on one another, creating a structured framework that drives effective decision-making and innovative solutions across industries.
Summary of Key Points
- Definition of Data Analytics: Data analytics involves examining datasets to draw conclusions and inform decisions.
- Importance: It is invaluable across sectors including healthcare, finance, retail, and more, aiding in predictive modeling and operational efficiency.
- Core Methodologies: Techniques such as quantitative and qualitative analyses, along with statistical methods and machine learning, highlight diverse approaches to data analytics.
- Tools and Technologies: Tools for visualization and management play critical roles in making sense of complex data, facilitating better insights.
- Challenges: Issues like data quality, integration, and ethical considerations pose challenges that professionals must navigate.
- Future Trends: The ongoing emergence of AI and big data analytics suggests an ever-evolving landscape, which professionals must stay abreast of.
The Continuous Evolution of Data Analytics
The field of data analytics is dynamic, marked by rapid advancements and technological innovations. Practitioners must acknowledge this continuous evolution and be adaptable in their methodologies. As industries increasingly rely on data, understanding emerging trends becomes essential.
Data analytics is moving toward greater automation through AI. Technologies like predictive analytics and machine learning are reshaping how businesses approach problems. As tools become more sophisticated, the ability to analyze vast datasets in real-time is enhancing decision-making further.
Moreover, the integration of big data has revolutionized the landscape, allowing for deeper insights than ever before. Organizations leveraging these trends not only enhance operational efficiency but also cultivate a culture of informed decision-making.
In summary, the continuous evolution of data analytics demands that industry professionals remain up to date with emerging technologies and methodologies. This commitment to learning ensures that organizations can harness the full potential of their data to navigate complex business environments.