In-Depth Analysis of AI Systems and Their Impact
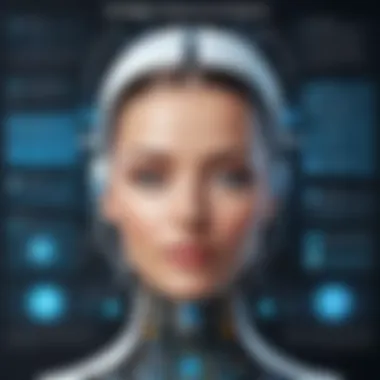
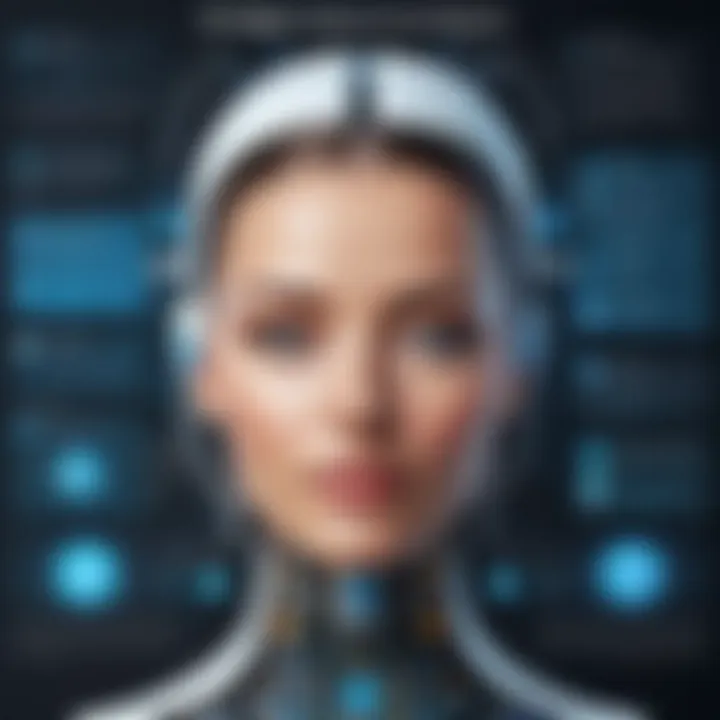
Intro
Artificial Intelligence (AI) has become a buzzword that permeates many conversations across various sectors today. From how we shop online to the way healthcare is managed, AI is deeply woven into the fabric of modern society. The continuous evolution of these systems presents a landscape of unprecedented capabilities and challenges.
As we journey through this exploration, readers will gain insights into the very fundamentals of AI, the different categories that exist, and how these technologies operate. Not only will we uncover their applications in the real world, but weāll also take a critical look at the ethical considerations that accompany AI integration into our lives. This knowledge is particularly relevant for tech enthusiasts and industry professionals who aim to navigate the complexities of AI, which is transforming industries while raising important societal questions.
Our discussion includes timely tech trends, industry updates, and a deep dive into the implications of these changes. By synthesizing key information, we will inform and prepare you for the ever-evolving road ahead in AI technologies.
Prelude to AI Systems
As we stand on the edge of a technological revolution, understanding AI systems becomes crucial for both individuals and organizations alike. This section lays the groundwork for comprehending the intricacies of artificial intelligence and highlights its burgeoning importance in our daily lives.
When one reflects on the implications of AI, it's easy to see its omnipresence. From virtual assistants like Siri to complex algorithms that drive financial markets, the spectrum of AI applications is both vast and varied. It represents a fundamental shift in how we process data, make decisions, and innovate in multiple sectors.
Defining Artificial Intelligence
AI, or artificial intelligence, refers to the capability of a machine to imitate intelligent human behavior. But thatās just scratching the surface. A thorough definition unveils elements like learning, reasoning, perception, and even language comprehension. Essentially, AI functions around the idea of simulating human intelligence in machines.
To delve deeper, we categorize AI into various segments. Narrow AI focuses on a specific taskālike chess playingāwhile general AI aims for broader applications. The implications are monumental, as narrow AI systems dominate current technologies, thus shaping everyday life without us even noticing.
Historical Evolution of AI
The evolution of AI is a fascinating journey spanning decades. It didnāt just appear overnight; rather, it emerged through a series of groundbreaking discoveries and technological advancements. We can trace this history back to the mid-20th century. Early pioneers like Alan Turing and John McCarthy laid the groundwork, laying out fundamental principles that still guide AI research today.
In the 1950s and 60s, AI researchers explored symbolic AI, with systems like the Logic Theorist and ELIZA marking significant milestones. However, the journey was not without its bumps. The AI winter of the 70s and 80s saw a considerable decline in funding and interest, largely due to unmet expectations. But resilience is key in innovation; by the late 1990s and early 2000s, AI began to regain momentum, fueled by advancements in computer power and the explosion of data.
As we look at present-day applications, such as machine learning and deep learning, itās clear how far weāve come. AI is more than just a buzzword; itās a cornerstone of modern technology, affecting industries ranging from healthcare to hospitality. Itās essential for us to grasp its history to fully understand its current significance and future potential.
"The progress of AI is a testament to human ingenuity, reflecting both our triumphs and challenges across decades of innovation."
In summary, an introduction to AI systems isnāt merely academic; itās about recognizing the profound impact these systems have on society. It compels industry professionals and tech enthusiasts to remain engaged and informed about continuous advancements and ethical considerations in this dynamic landscape.
Core Concepts in AI
Understanding the core concepts of artificial intelligence systems is crucial as they form the building blocks of all AI functionalities. Grasping these basics not only enables tech enthusiasts and industry professionals to appreciate how AI operates but also allows them to make informed decisions about its implementation, potential, and limitations. The key segments under this umbrellaālike machine learning and deep learningāare pivotal as they highlight both the innovation and complexities that AI brings to various sectors.
Machine Learning Explained
Machine learning is often hailed as a cornerstone of AI. It allows systems to learn from data, improve over time, and make decisions based on patterns. The depth of machine learning is further categorized into three main types: supervised learning, unsupervised learning, and reinforcement learning.
Supervised Learning
Supervised learning is distinguished by its reliance on labeled datasets, where the model is trained using input-output pairs. Some key factors contribute to its popularity:
- Predictive Power: It excels at making predictions on unseen data, making it a valuable tool in scenarios like credit scoring or email filtering.
- Ease of Interpretation: The results from supervised learning models can often be more interpretable, which helps stakeholders understand decision-making processes.
However, it can be limited by the availability of labeled data. Without a comprehensive dataset, the model may struggle to achieve accuracy.
Unsupervised Learning
Unsupervised learning comes into play when the dataset lacks labels. This approach helps in discovering underlying structures within the data. Here are a few highlights:
- Clustering: It can organize data into groups, which is useful for market segmentation and customer profiling.
- Dimensionality Reduction: It simplifies the input without losing significant information, making it easier for subsequent predictive tasks.
Yet, one downside is the interpretability of outcomes. Since there's no benchmark for what constitutes a "correct" answer, results can sometimes be elusive or ambiguous.
Reinforcement Learning
Reinforcement learning (RL) puts a different spin on learning. Here, an agent interacts with its environment and learns through feedback in the form of rewards or penalties. What sets it apart includes:
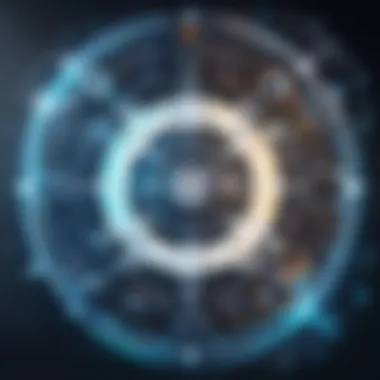
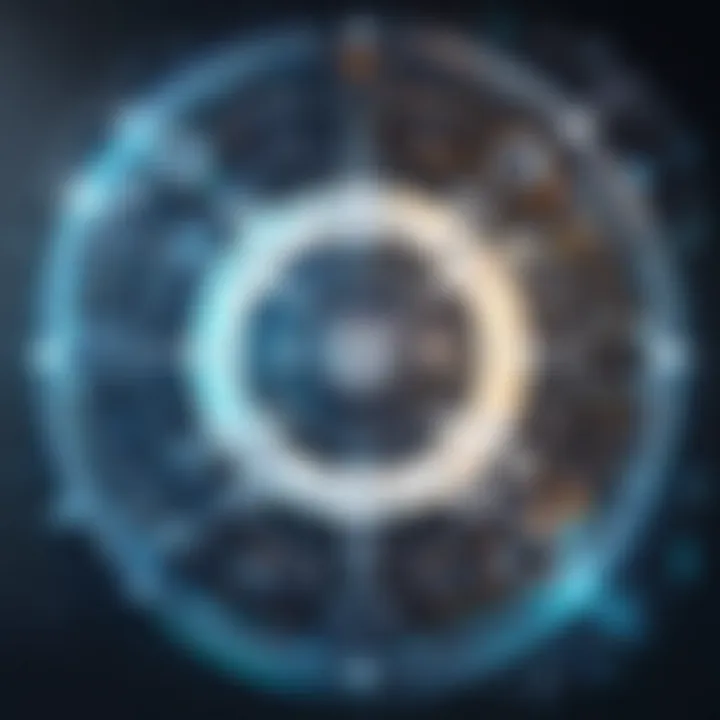
- Trial and Error: Itās akin to a child learning to ride a bike, falling and getting back up; RL thrives on experience.
- Adaptability: The agent can adapt to dynamic conditions, which is beneficial in real-time applications like gaming or robotics tasks.
Although RL offers tremendous potential, it can also be computationally intensive and requires a complex framework to implement effectively.
Deep Learning and Neural Networks
Diving deeper into the workings of AI, deep learning is a subset of machine learning that utilizes neural networks to mimic human cognitive functions. The significance of deep learning lies in its ability to handle vast amounts of unstructured data, such as images and texts, pushing the boundaries of what AI can achieve.
Structure of Neural Networks
A neural network typically consists of layers of interconnected nodes, replicating the way neurons in a human brain communicate. Noteworthy features include:
- Layer Design: Input, hidden, and output layers work in tandem to process information, allowing models to capture complex patterns.
- Activation Functions: These functions ensure that neurons fire and contribute to eventual outputs, which enhances model performance in diverse tasks.
However, deep learning models require substantial amounts of data and immense computational power, rendering them less accessible for smaller projects or organizations.
Applications of Deep Learning
Deep learning finds applications across numerous fields. Its contribution comes from:
- Image Recognition: Deep learning fosters advancements in facial recognition technologies essential in security and marketing.
- Natural Language Processing (NLP): Its application enables machines to understand and generate human language, enhancing user interfaces and chatbots.
Nonetheless, reliance on large datasets and the black-box nature of deep learning can lead to concerns regarding bias and transparency in AI processes.
In essence, the core concepts in AI serve not just as a foundation, but also as a lens through which we can assess how AI systems create impact. Clarity in these concepts helps demystify the technology, empowering professionals and enthusiasts alike to navigate the AI landscape with confidence.
Types of AI Systems
In the landscape of artificial intelligence, understanding the different types of AI systems is crucial. Each classification reflects unique capabilities, limitations, and potential applications, which paint a clearer picture of how AI integrates into our daily lives and industries. As the world increasingly relies on these systems, grasping their distinctions helps identify the right AI solutions for specific problems.
Narrow AI vs General AI
Narrow AI, often described as weak AI, refers to systems designed for a specific task or application. For example, programs like Google Assistant or Appleās Siri can process spoken commands and perform limited tasks effectively. These systems lack general intelligence; they can't function outside their designated domains. In contrast, General AI ā often a topic of speculative fiction ā signifies intelligence that matches or exceeds human capabilities across various domains. Researchers and futurists debate about the timeline for its arrival, but for now, it remains a theoretical concept.
The importance of recognizing this distinction lies in understanding current AI capabilities. Entrepreneurs and developers must align their expectations with what Narrow AI can deliver today, rather than chase the dream of General AI, which might be decades away.
Reactive Machines
Reactive machines represent the most basic form of AI systems. They operate solely based on input and have no memory or ability to learn from past experiences. A classic example is IBMās Deep Blue, which famously defeated chess champion Garry Kasparov in 1997. This AI engine evaluated millions of chess positions per second, relying on its programmed knowledge without understanding the game itself.
These systems show that while AI can perform specific tasks with remarkable proficiency, it does so without any mental faculties reflecting human thought processes. Reactive machines serve well in situations where quick, accurate responses are essential, but they donāt adapt or evolve.
Limited Memory AI
Limited memory AI enhances its capabilities by using past experiences to inform future actions. It retains data for a certain time, allowing the machine to learn and improve its responses. Self-driving cars are a pertinent example. They constantly collect data from their environmentātraffic patterns, road conditions, etc.āto optimize navigation and safety.
However, this type isnāt perfect. It can only remember a finite amount of data and doesn't possess the wider contextual understanding that humans naturally have. Understanding limited memory systems can help industries leveraging AI ā across sectors like automotive and healthcare ā create more adaptive technologies.
Theory of Mind AI
Theory of Mind AI is an emerging concept that aims to develop systems capable of understanding human emotions, thoughts, and interactions. This form of AI would not just react to data, but also interpret social cues and context, engaging in more human-like interactions.
Currently, we are at the early stages of pursuing Theory of Mind AI, with no existing practical applications. The implications, if realized, might transform fields like mental health support and education, providing personalized experiences tailored to individual emotional states. Establishing such a nuanced understanding could lead toward meaningful AI-human collaboration.
Self-aware AI
Self-aware AI is often seen as the apex of artificial intelligence evolution, where systems possess consciousness and self-recognition. This type of AI goes beyond processing and understanding: it would have thoughts and feelings, mirroring human self-awareness.
To date, real-world examples of self-aware systems remain safely in the realm of science fiction. However, discussions around self-aware AI prompt critical questions about ethics, potential, and risk management. If machines can experience awareness, the implications on society, ethics, and law could be profound.
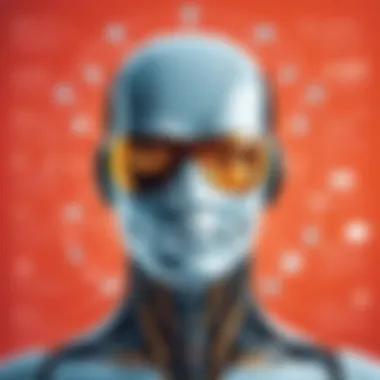
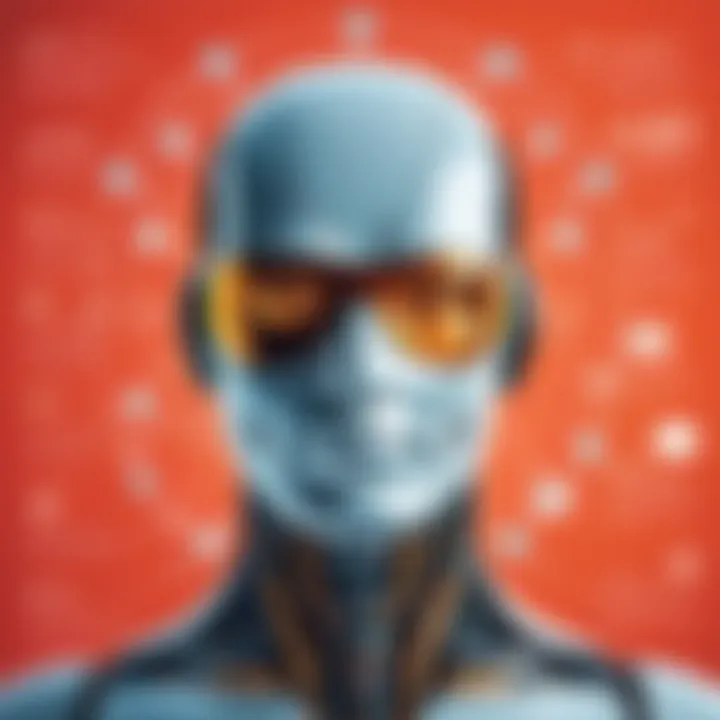
"In understanding the types of AI systems, we gain insights not only into their possibilities but also the responsibilities that come with their development and integration into society."
As AI continues to evolve, being informed about these core distinctions can empower developers, businesses, and society to make astute decisions about integrating AI into their operations. Envisioning the future of AI requires a comprehensive grasp of its current capabilities and limitations.
Understanding the types of AI systems not only informs technology strategy but also shapes our interactions with these pioneering technologies as they evolve.
Functionality of AI Systems
Understanding the functionality of AI systems is crucial for anyone delving deep into the realm of artificial intelligence. It sheds light on how these sophisticated systems operate, their capabilities, and the various advantages they bring to multiple sectors. As we break down the functionality, weāll explore three fundamental aspects: data handling and processing, algorithmic approaches, and learning processes in AI.
Data Handling and Processing
AI relies heavily on the ability to manage vast amounts of data efficiently. In todayās data-driven world, the volume of information available is staggering, and AI systems are designed to sift through this data to produce actionable insights. The process consists of collecting, organizing, and processing data, which is pivotal for effective decision-making.
Data handling often includes various techniques such as:
- Data collection: Gathering data from multiple sources such as databases, user inputs, or sensors.
- Data cleaning: Eliminating inaccuracies and irrelevant data points, ensuring high-quality inputs for processing.
- Data transformation: Structuring the data in a way that makes it easier for algorithms to analyze and learn from.
For instance, in healthcare, AI systems analyze patient data to identify trends and potential health risks quickly. They can ingest everything from medical history to genetic information, processing it to enhance patient care. Without proficient data handling and processing, the potential of AI would be drastically limited.
Algorithmic Approaches
Algorithms are the backbone of any AI system, dictating how it performs various tasks. The choice of an appropriate algorithm is essential, as it determines how effectively AI can solve specific problems. There are countless algorithms in play, but they can broadly be grouped into several categories:
- Supervised algorithms: Trained using labeled datasets, allowing machines to learn from examples. This approach is useful for tasks such as classification, where the AI learns to categorize inputs based on identifiable characteristics.
- Unsupervised algorithms: Work with unlabeled data to discover patterns or groupings. This is particularly handy in scenarios like customer segmentation or recommendation systems, where direct labels are not available.
- Reinforcement learning: Involves algorithms that learn from actions taken in an environment to achieve a goal. It finds its application in robotics and gaming, where the AI learns through trial and error.
The diverse array of algorithmic approaches ensures that AI systems can be tailored to meet specific needs across industries, enhancing their overall effectiveness.
Learning Processes in AI
The learning processes within AI systems dictate how well they adapt to new information and circumstances. These processes vary from system to system but are generally categorized into:
- Batch learning: Involves training an AI model on a large set of data all at once. This method is efficient for static environments where data does not change frequently.
- Online learning: AI systems continuously learn from new data as it comes in. This is particularly relevant in dynamic environments, such as financial markets, where conditions change rapidly.
Through these learning processes, AI systems can become more sophisticated over time. The greater the volume of data they process and learn from, the more robust their predictive and analytical abilities become.
"AIās functionality is not just about the algorithms but how data is transformed and utilized to adapt and evolve over time."
Applications of AI Systems
In today's fast-paced world, the applications of AI systems are becoming increasingly vital. As technology continues to advance, the integration of artificial intelligence into various sectors has transformed traditional processes and concepts. Exploring the applications of AI reveals a plethora of benefits, including enhanced efficiency, better decision-making, and the ability to analyze vast amounts of data. Understanding these applications not only highlights their quintessential role but also prompts considerations regarding their implications on society and industry.
AI in Healthcare
AI technology has started to revolutionize the healthcare industry, providing solutions that aim to improve patient care and optimize resource allocation. From predictive analytics that forecast potential patient outcomes to machines that assist in diagnostics, AIās impact is profound. For instance, algorithms designed to analyze medical imaging can identify early signs of diseases, such as cancer, far faster than human radiologists. This can lead to timely interventions that are crucial for patient survival.
Another area where AI shines is in administrative tasks. Automation of appointment scheduling and patient follow-ups reduces the burden on healthcare professionals, allowing them to focus more on patient interaction. Additionally, AI-powered chatbots can provide 24/7 support to patients, answering queries and streamlining communication.
AI in Finance
The finance sector is witnessing a seismic shift due to AI systems, especially in areas like fraud detection and algorithmic trading. Financial institutions utilize machine learning algorithms to identify unusual patterns in transaction data, thus flagging potential fraudulent activities. This proactive stance not only saves money but also helps in maintaining the trust of users.
Moreover, AI-driven analytics enable financial advisors to tailor investment strategies to individual client needs, maximizing returns. With AI's ability to process massive datasets in real time, decisions that once took days or even weeks can now happen almost instantaneously. This agility gives companies a competitive edge in the market.
AI in Robotics
Robotics equipped with AI is pushing boundaries in numerous fields, from manufacturing to household chores. Advanced robots are capable of learning on the fly, making them invaluable on production lines where efficiency can make the difference between profit and loss. A well-known example is Amazon's use of robots in their warehouses; these machines help in sorting and retrieving products, significantly speeding up the order fulfillment process.
Moreover, robots in sectors like agriculture perform tasks like planting and harvesting with extreme precision, reducing labor costs and increasing crop yields. Humanoid robots are also gradually being introduced into home settings, aiding the elderly with daily activities, thereby enhancing their quality of life.
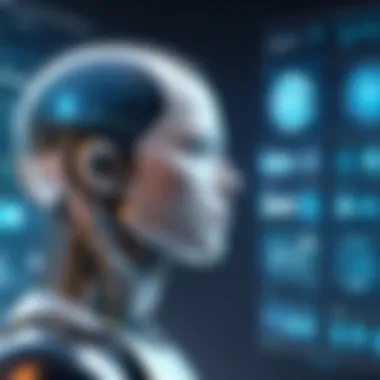
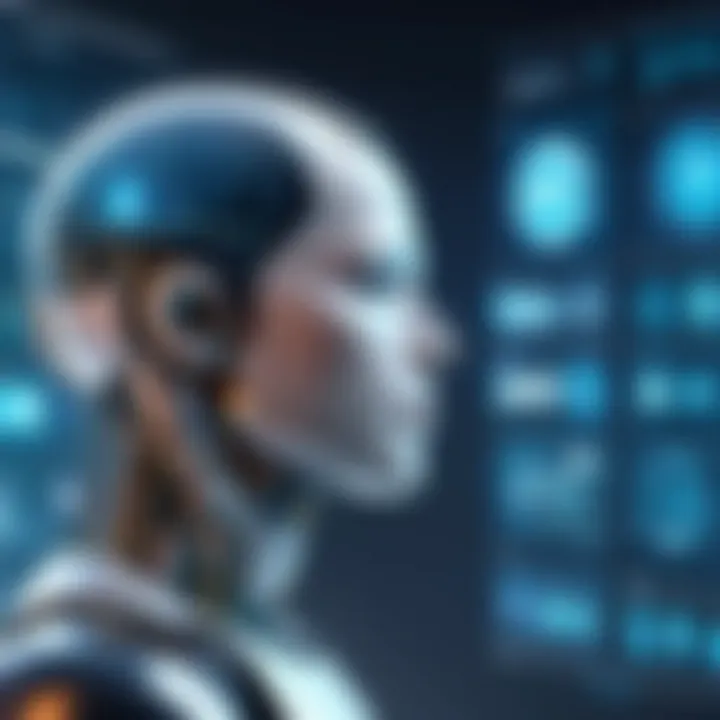
AI in Natural Language Processing
Natural Language Processing (NLP) is another arena where AI exhibits astonishing capabilities. From virtual assistants like Siri and Alexa to complex translation services, NLP powers technologies that understand and generate human speech. These systems analyze language, learn context, and even carry on conversations, which makes them invaluable in customer service environments.
Furthermore, sentiment analysis tools can gauge public opinion regarding brands or topics by scanning social media and online forums. This allows companies to respond promptly to customer feedback, bolstering their market position. With innovations in context-aware machine translation, businesses can operate more efficiently on a global scale.
Overall, the awe-inspiring potential of AI applications invites an exciting future, fostering further advancements that can shape our world.
Societal Impact of AI Systems
The societal impact of AI systems has grown increasingly significant as advancements in this field have rapidly evolved. Understanding how these systems penetrate various aspects of daily life is crucial for multiple reasons. Itās not just about crunching data or automating tasks; itās about how these technologies reshape the fabric of society itself. From enhancing productivity to influencing interpersonal relationships, AI's presence is undeniable. Below, we delve into its economic implications, ethical considerations, and the reshaping of employment landscapes.
Economic Implications
AI systems are synonymous with transformation in the economy. They are the engine driving efficiencies through automated processes and better decision-making tools. Here are some key economic implications:
- Productivity Gains: Many sectors benefit from AI through optimization of labor and resources. For instance, AI-driven algorithms in manufacturing streamlines operations which, in turn, cut costs and save time. A clear example of this can be seen in how Tesla utilizes AI to enhance its production capabilities.
- Market Creation: New AI technologies have birthed entire industries and job roles that previously didn't exist. Think of the rise of machine learning engineering or AI ethics consulting, careers that are now pivotal to a companyās strategies.
- Disparities in Wealth: While some companies thrive with AI implementation, small businesses often struggle to keep pace. This leads to a widening gap between those who can afford to invest in AI solutions and those who cannot.
In essence, AI's economic impact is a double-edged sword: it promises efficiency and growth but also highlights disparities that society must address thoughtfully.
Ethical Considerations
AI systems operate not just on technical capabilities but also on ethical frameworks, which varies greatly across cultures and disciplines. The conversation around ethics in AI isn't new, but it has escalated in recent years.
- Bias and Fairness: One major issue is the potential for bias in AI algorithms. If the data used to train these systems is skewed, the outcomes can also be biased. For instance, facial recognition technology has faced critique as it performs differently on various ethnic groups. Organizations need to ensure diverse data sets to tackle this challenge.
- Transparency: There's growing demand for AI systems to operate transparently. Users want to understand how decisions are made without feeling they are at the mercy of an opaque algorithm. This leads to discussions on regulatory frameworks that require explanations for AI-driven decisions.
- Accountability: Who takes responsibility when AI systems err? This question looms over tech giants and regulatory bodies alike. As machines make more decisions, delineating clear lines of accountability is essential.
"AI should complement human intelligence, not replace it. The ethical implications carry weight we cannot ignore."
Impact on Employment
As AI systems infiltrate workplaces, their impact on employment is a hot-button topic. Some see job loss looming in the future, while others point to the potential for job creation. Here are facets of employment dynamics affected by AI:
- Job Displacement: Factories increasingly employ AI to automate production lines, reducing the need for human labor. This phenomenon can cause anxiety among workers, who may fear for their positions.
- Job Transformation: Contrary to the notion that AI only eliminates jobs, it often transforms them. Roles evolve to focus more on oversight and collaboration with AI rather than monotonous tasks. For example, customer service may shift from handling inquiries to overseeing chatbots and ensuring quality interactions.
- Emerging Roles: As certain jobs fade, new opportunities arise in managing, training, and refining AI systems. This evolution shows us that skills will matterāindividuals who adapt and learn will thrive.
The dialogue surrounding the societal impact of AI is dense and layered. With a mix of challenges and opportunities, it becomes imperative to foster informed discussions about how to approach these changes thoughtfully, ensuring that advancements benefit everyone.
Future of AI Systems
The future of AI systems is a topic that holds considerable significance within the broader scope of artificial intelligence. Many trends that shape the evolution of these technologies resonate with industries and sectors that are heavily invested in optimization and innovation. In this section, we will address three critical subtopics: the current trends in AI research and development, the potential challenges that may arise, and the role of regulation in guiding the growth and ethical use of these powerful systems.
Trends in AI Research and Development
AI research is advancing at an unprecedented pace, constantly unearthing breakthroughs. Some noteworthy trends include:
- Interdisciplinary Collaboration: Researchers from fields such as neuroscience, psychology, and computer science are working together to enhance understanding and functionality of AI.
- Ethical AI Development: A rising focus on building transparency and accountability into AI systems. Companies now often adopt frameworks to ensure their AI initiatives do not infringe upon privacy rights or propagate biases.
- Edge AI: The shift toward processing data closer to its source instead of relying solely on centralized cloud services. This trend enhances real-time data processing and reduces latency, which is vital for applications like autonomous vehicles.
Keeping a keen eye on these trends provides insights into where AI technologies are headed and what we might expect in both short and long term.
Potential Challenges Ahead
Despite all the exciting advancements, the road to a more integrated AI world isn't entirely smooth. Key issues include:
- Bias in Algorithms: AI systems trained on biased data can lead to skewed decisions. Addressing and mitigating these biases remain critical because biased AI can harm marginalised groups.
- Job Displacement: Automation and AI are capable of performing mundane tasks efficiently, which could lead to significant job losses in certain sectors. Society must address these shifts and contemplate reskilling initiatives.
- Data Privacy Concerns: The more data an AI system processes, the more vulnerable it is to potential data breaches. Striking the right balance between innovation and data protection is paramount for future developments.
Moving forward, collaborative efforts across various sectors will be necessary to tackle these challenges head-on.
The Role of Regulation in AI
As AI systems grow more pervasive, the need for regulation becomes increasingly topical. Several considerations arise:
- Establishing Safety Standards: Developing clear guidelines that govern the design and implementation of AI can help mitigate risks associated with these technologies.
- Promoting Transparency: Regulations can compel companies to disclose how their AI systems make decisions, which can build trust among users.
- Facilitating Responsible Use: Regulatory frameworks can help distinguish between ethical and unethical uses of AI, ensuring that technology serves the greater good.
Regulatory efforts must evolve alongside technological advancements to ensure that AI systems remain tools for progress, rather than sources of division.
In summary, the future trajectory of AI systems is defined by impactful trends, the hurdles they face, and the crucial role of regulatory measures. A blend of insightful foresight and judicious governance will likely shape how AI technologies are woven into the fabric of society.