Understanding AI: Definitions and Real-World Applications
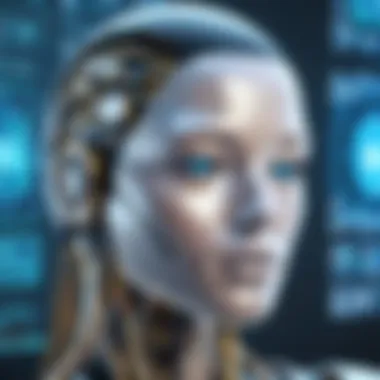
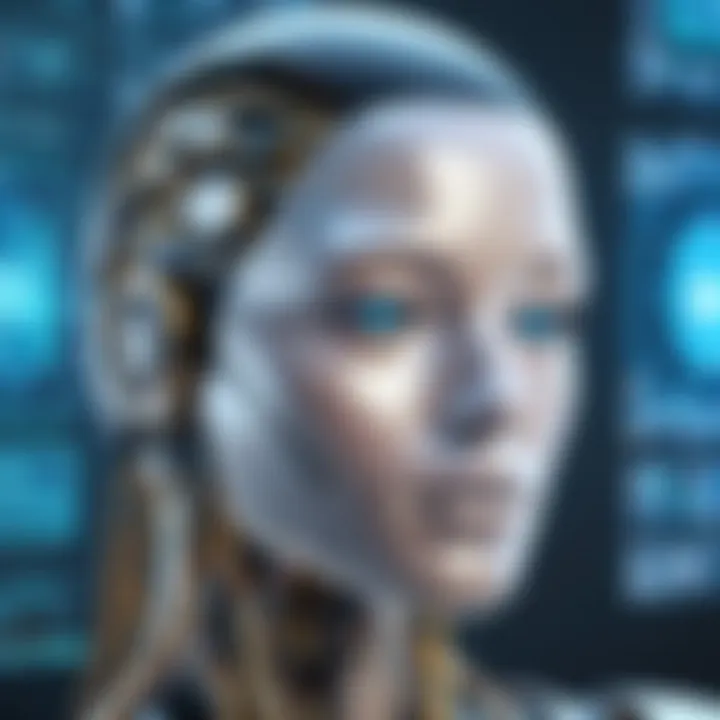
Intro
Artificial intelligence has become a central topic in technology discussions. It is crucial to understand what AI encompasses. Many definitions exist, often leading to confusion about its capabilities and scope. This article aims to provide clarity on AI and illustrate its real-world applications. We will explore different facets of AI, from its basic definitions to its implementation in various sectors. This comprehensive guide is designed for a diverse readership, including tech enthusiasts and industry professionals.
Tech Trend Analysis
Overview of the Current Trend
Artificial intelligence is rapidly evolving. It plays an integral role in sectors like healthcare, finance, and transportation. Recent advancements in machine learning and natural language processing are transforming industries and user experiences. New AI tools are emerging, often featuring capabilities that were previously deemed futuristic.
Implications for Consumers
The rise of AI significantly affects consumers. Personalized services are becoming standard, with AI-driven recommendations enhancing user satisfaction. In finance, AI algorithms offer real-time analysis for better investment decisions. However, this trend also raises concerns about privacy and data security. Users must become aware of how their data is utilized and processed.
Future Predictions and Possibilities
Looking ahead, the potential of AI continues to grow. Innovations in robotics and autonomous systems may reshape labor markets and daily life. AI could enhance efficiency and precision, yet ethical considerations will play an essential role in guiding these developments. Policymakers and industry leaders must collaborate to harness AI's benefits responsibly, setting a framework for its appropriate use.
"The future of AI depends not only on technological advancements but also on our ethical and societal frameworks."
Real-World Examples
AI in Healthcare
Many healthcare organizations are applying AI technologies for diagnostics and patient care. Algorithms analyze imaging data to detect diseases more accurately. Tools like IBM Watson assist doctors by recommending treatment options based on vast databases of medical literature.
AI in Financial Services
In finance, AI algorithms review extensive data sets to identify trends and predict market movements. Companies like ZestFinance leverage AI to assess credit risk, thus improving lending outcomes.
AI in Transportation
AI is transforming transportation with developments in autonomous vehicles. Companies like Tesla are utilizing AI for self-driving capabilities, significantly impacting how people perceive travel.
Closure
Through this exploration, it is evident that artificial intelligence is reshaping the landscape across multiple industries. Its implications deserve careful consideration. Understanding both its advantages and challenges will better prepare individuals and organizations for the future. A balanced approach in leveraging AI will be essential for maximizing its potential while addressing ethical concerns.
Intro to Artificial Intelligence
Artificial intelligence (AI) has permeated various dimensions of modern life, influencing how we work, communicate, and interact with technology. Understanding this subject is essential in today’s world as AI reshapes industries and personal experiences alike. It offers unprecedented possibilities along with several challenges, making it a focal point of discussion in technological advancement.
The exploration of AI in this article aims to clarify its definition, historical context, core components, and applications. By dissecting these elements, the reader can gain a thorough understanding of AI's significance. This understanding not only helps in recognizing the current landscape of AI technology but also prepares individuals for future developments and ethical considerations.
What is Artificial Intelligence?
Artificial intelligence refers to the capability of a machine to imitate intelligent human behavior. In essence, AI systems are designed to perform tasks that typically require human intelligence. This can range from simple functions such as recognizing patterns or solving basic problems, to more complex processes like understanding natural language or making decisions.
AI encompasses a broad field including machine learning, where systems learn from data; deep learning, which uses neural networks to model complex patterns; and natural language processing, focused on facilitating communication between humans and machines. The definitions may vary slightly depending on the specific application, but the core idea remains rooted in automating tasks that usually need human cognitive capabilities.
Historical Context of AI Development
The development of artificial intelligence can be traced back to the mid-20th century, emerging from ideas in mathematics, psychology, and cognitive science. Initial theories posited by pioneers like Alan Turing laid the groundwork for computing machines capable of intelligent behavior. Turing’s test remains a pivotal benchmark for determining machine intelligence.
By the 1950s and 1960s, interest in AI grew, leading to the establishment of universities and research institutions dedicated to the field. Early successes included symbolic methods for problem solving and simple game-playing algorithms. However, progress slowed in the 1970s due to high expectations that were not met, leading to a period often referred to as the “AI winter.”
Advancements resumed in the 1980s with increased computational power and availability of massive datasets. The rise of machine learning in the 2000s, propelled by big data, marked a transformative phase. Today, AI technology is integral to commercial applications such as Google Search and Facebook’s recommendation systems, firmly establishing its relevance in everyday life.
"AI is changing the way we live and work, creating new opportunities and challenges in equal measure."
Core Components of AI
In the realm of artificial intelligence, understanding its core components is essential for grasping how these systems operate and the benefits they offer. The main elements of AI involve the processes of Machine Learning, Deep Learning, and Natural Language Processing. Each component plays a critical role in creating intelligent systems capable of learning, reasoning, and understanding human language.
Machine Learning Explained
Machine Learning (ML) is a pivotal aspect of AI. It is the method by which machines learn from data and improve their performance over time without being explicitly programmed. Through ML, systems can analyze vast quantities of information, uncover patterns, and make decisions based on that data. The benefit here lies in its ability to automate tasks that would require significant human effort otherwise.
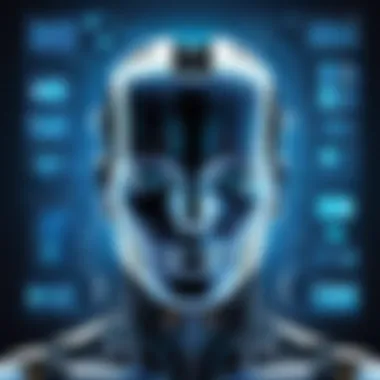
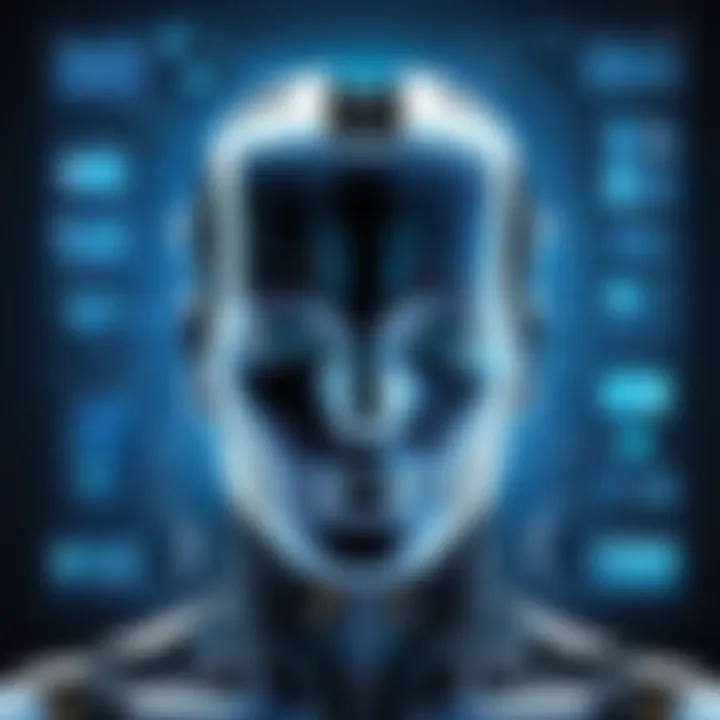
The basic types of machine learning are supervised, unsupervised, and reinforcement learning. In supervised learning, models are trained on labeled datasets, making predictions based on input-output pairs. Unsupervised learning involves discovering hidden patterns in data when no labels are provided. Reinforcement learning, on the other hand, focuses on making sequences of decisions by learning from the consequences of actions taken in an environment.
Deep Learning: A Subset of AI
Deep Learning is a specific subset of machine learning that utilizes neural networks with many layers to analyze various factors of data. This structure allows models to learn at multiple levels of abstraction, making it particularly effective for complex tasks, such as image and speech recognition. Deep Learning is behind many breakthrough applications, powering systems like Google Photos, where it can categorize images automatically.
The capability of deep learning is highlighted when it comes to handling unstructured data. Traditional machines do not perform well with complex data types that do not fit into predefined categories. Deep learning effectively manages this with great success. The advancements made in this field have been a catalyst for numerous innovations in AI functionalities, enabling machines to learn features directly from data.
Natural Language Processing
Natural Language Processing (NLP) allows machines to understand and interact with human language. NLP combines various skills, including speech recognition, text analysis, and machine translation. This area of AI is particularly relevant as it impacts how we use technology daily. Voice assistants like Apple's Siri or Amazon's Alexa rely heavily on NLP techniques.
The principal challenge of NLP is the inherent ambiguity and complexity of human languages. Variations in sentiment, context, and culture can alter meaning. Addressing these challenges is critical for enhancing user interactions with AI systems.
Natural Language Processing can serve as a bridge between humans and machines, enabling meaningful communication.
The components of AI—Machine Learning, Deep Learning, and Natural Language Processing—are fundamental in shaping the future of technology. These elements are interconnected, working together to equip machines with the ability to learn, make decisions, and interact in ways that are becoming increasingly sophisticated.
Types of Artificial Intelligence
Understanding the different types of artificial intelligence is crucial for comprehending its capabilities and limitations. The classification separates AI into categories based on their functionalities and applications, which further influences how they are developed and employed. By grasping these distinctions, stakeholders can make informed decisions about integrating AI into various sectors.
Narrow AI versus General AI
Narrow AI, often referred to as weak AI, is designed to perform a specific task. Examples include voice recognition systems like Apple’s Siri or recommendation algorithms seen in Netflix. These systems excel in a limited domain but lack the ability to perform tasks beyond their programmed capacities.
In contrast, General AI, known as strong AI, possesses cognitive functions comparable to those of human beings. This includes reason, problem solving, and adaptation across multiple domains. While theoretical constructs of General AI are discussed in academia, practical implementations remain elusive. The pursuit of General AI raises numerous debates concerning ethics and safety in technology.
Reactive Machines and Limited Memory
Reactive machines are a category of Narrow AI that operate strictly on the rules and inputs they receive without storing past experiences. An example is IBM’s Deep Blue, the chess-playing computer that defeated a world champion in 1997. It analyzes the current state of the game and responds accordingly, but it does not learn or improve from previous matches.
Limited memory AI, however, has the ability to learn from past data. This type actively uses historical data to inform its actions and decisions. Self-driving cars, equipped with radar and cameras, fall under this category. They learn to navigate and drive based on previous interactions and the data they continuously collect while on the road. This evolution in AI allows for more sophisticated functionalities.
Theory of Mind and Self-aware AI
Theories surrounding Theory of Mind AI focus on the idea that machines could eventually simulate human-like understanding of emotions, intentions, and beliefs. This concept emphasizes empathy and responsiveness to human experiences, seen in more advanced robots that aim to interact on a social level. Current technology, however, is far from achieving this capability, as AI lacks a comprehensive understanding of human emotions.
On the other hand, Self-aware AI takes the notion even further, where machines would develop self-consciousness and autonomy. This level of AI would not only perceive its surroundings but also understand its existence and its impact on the environment. Discussion surrounding Self-aware AI often delves into ethical concerns, as it raises questions about rights and responsibilities of sentient entities.
In summary, categorizing AI into types such as Narrow AI, General AI, Reactive Machines, Limited Memory, Theory of Mind, and Self-aware AI enables a clearer understanding of the technology's landscape. Each segment has unique characteristics and implications, shaping how society interacts and coexists with increasingly intelligent systems.
Applications of Artificial Intelligence
The applications of artificial intelligence (AI) are integral to understanding its role in modern society. AI solutions have transitioned from theoretical concepts to practical tools that significantly improve various sectors. The importance of this topic lies in the variety of AI applications that not only enhance efficiency but also transform the way we interact with technology. Exploring AI applications reveals the benefits, challenges, and the future landscape of different industries.
AI in Healthcare
AI is making profound impacts in healthcare, a sector where precision and efficiency are critical. The technology enhances processes such as diagnostics, treatment personalization, and complex surgical procedures.
Diagnostics
Diagnostics is a crucial area where AI shows substantial promise. AI algorithms can analyze medical images faster, often with greater accuracy than human experts. This enhances early detection of diseases, which is vital for effective treatment. The key characteristic of AI diagnostics is its ability to process vast datasets, identifying patterns that might be overlooked by human specialists. This capability makes it a popular choice within the healthcare systems. However, reliance on AI also raises concerns about misdiagnosis and the need for human oversight.
Personalized Medicine
Personalized medicine tailors treatment plans based on individual patient data. AI systems analyze genetic data alongside other health information to offer customized therapies. The key characteristic is the focus on individualization, making it a beneficial aspect of modern medical treatment. Personalized medicine optimizes outcomes and minimizes side effects. Nonetheless, the complexity of data analysis can be a disadvantage, as variations in data quality may lead to inconsistent results.
Robotic Surgery
Robotic surgery utilizes AI to assist surgeons in complex procedures. This technology improves precision and reduces recovery time for patients. The key characteristic of robotic surgery is its ability to perform minimally invasive operations through small incisions. This makes it a popular choice among surgeons and hospitals aiming to enhance patient outcomes. However, training and maintenance costs for robotic systems can be prohibitive.
AI in Finance
The finance industry has embraced AI to enhance decision-making and streamline operations. From trading to fraud detection, AI is reshaping financial services and making them more efficient.
Algorithmic Trading
Algorithmic trading uses AI to analyze market data and execute trades at speeds unattainable by humans. This is crucial for maintaining competitive advantage in financial markets. The key characteristic of this method is its ability to remove emotional decision-making, yielding data-driven trades. The benefit of algorithmic trading is its capacity to manage vast numbers of transactions simultaneously. However, reliance on algorithms can lead to significant risks, particularly if the underlying models are flawed.
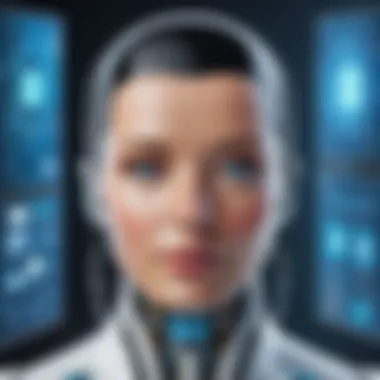
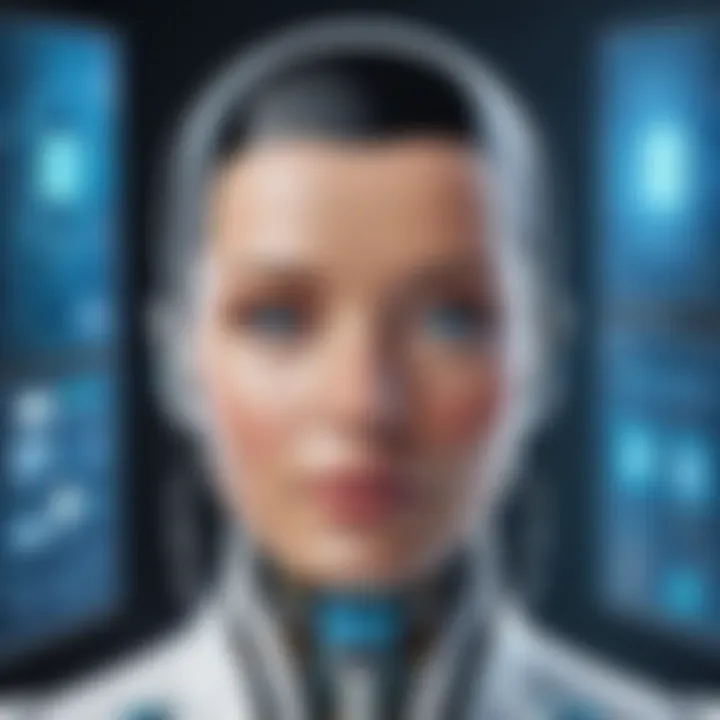
Fraud Detection
Fraud detection systems analyze transaction patterns to identify unusual activity. This application is essential as the financial landscape becomes more complex and susceptible to fraud. The key characteristic lies in its proactive capabilities, allowing organizations to act quickly. This is a preferred choice for institutions seeking to safeguard assets and data. Nevertheless, false positives can disrupt legitimate transactions and affect customer relations.
Risk Management
AI plays a significant role in risk management by predicting potential risks based on historical data. The key characteristic is its analytical strength in forecasting and assessing risks. This makes it a beneficial approach for financial institutions. AI-enhanced risk management systems can analyze various scenarios more effectively than traditional methods. However, the challenge remains in ensuring the accuracy of the data used in these models.
AI in Transportation
AI is at the forefront of transportation innovation, improving efficiency and safety while addressing logistical challenges in real-time.
Autonomous Vehicles
Autonomous vehicles are among the most talked-about applications of AI, capable of operating without human intervention. Their key characteristic is integration of machine learning and sensors for navigation. This makes them a popular choice for future urban planning. While they promise to reduce accidents and traffic congestion, legal and ethical concerns arise regarding liability during incidents.
Traffic Management
AI enhances traffic management systems by analyzing data from various sources in real-time. Traffic patterns can be predicted, optimizing flow and reducing congestion. The key characteristic is its ability to adapt to changing conditions automatically. This is beneficial for city planners aiming to improve urban mobility and reduce emissions. However, implementation costs and the need for robust infrastructure remain challenges.
Public Transportation Systems
AI also supports public transportation systems by optimizing routes and schedules based on user demand. The key characteristic of this application is its ability to improve service efficiency. This makes it an attractive choice for municipalities aiming to enhance public transport. Nonetheless, integration with existing systems can be complex and require significant investment.
AI applications across sectors like healthcare, finance, and transportation highlight the transformative power of this technology. Each application presents unique benefits and challenges, together shaping a landscape where AI increasingly plays a central role.
AI Technologies in Daily Life
AI technologies have become woven into the fabric of our daily existence. Their importance lies not only in the convenience they provide but also in their ability to enhance functionality across various platforms. From managing our schedules to providing personalized content recommendations, AI has transformed the way we interact with technology and each other. Understanding the benefits and considerations of these technologies can help individuals leverage their capabilities effectively.
Smart Assistants and Chatbots
Smart assistants like Amazon Alexa and Google Assistant exemplify AI’s role in simplifying our daily tasks. These systems utilize natural language processing to understand and respond to user queries. Smart assistants offer a range of functionalities, from setting reminders to controlling home devices. Their integration with multiple applications provides seamless access to information, which increases productivity.
Chatbots have also gained traction in customer service and engagement. They are programmed to handle inquiries, provide support, and even facilitate transactions. Companies like Shopify and Zendesk deploy these AI tools to enhance user experience by offering instant feedback and support, reducing wait times for human agents. This integration not only saves time but also assists businesses in managing large volumes of interactions.
Recommendation Systems
Recommendation systems are crucial in helping users navigate vast amounts of content. Platforms like Netflix and Spotify harness AI to analyze user behavior and preferences. Through collaborative filtering and content-based algorithms, these services deliver tailored recommendations that enhance user engagement. This approach not only simplifies content discovery but also encourages users to explore new options, thereby enriching their experiences.
The effectiveness of recommendation systems highlights the significance of user data. However, there is a delicate balance between personalization and privacy. Users must be aware of how their data is collected and used. Transparent practices in data management can build trust and improve overall satisfaction with these technologies.
AI in Smart Homes
Smart home technologies have revolutionized how we interact with our living spaces. Devices such as smart thermostats, security cameras, and lighting systems utilize AI to enhance convenience and efficiency. For instance, the Nest Learning Thermostat adapts to user preferences and optimizes energy consumption. This leads to cost savings and a reduced environmental footprint.
Moreover, smart home ecosystems promote interconnectivity among devices. Through platforms like Apple HomeKit and Google Home, various gadgets can be centralized and controlled easily. This interconnectedness allows for automation of daily tasks, providing a streamlined lifestyle. It raises considerations regarding security, as interconnected devices can be vulnerable to hacking.
"AI technologies are not just innovative tools; they shape our experiences in tangible ways."
In summary, AI technologies in daily life serve as force multipliers. By automating tasks and enabling personalization, they enhance the overall quality of life. However, understanding the implications these technologies bring is vital. Security, privacy, and the balance of convenience should be top of mind as we navigate this ever-evolving landscape.
Challenges in AI Development
The realm of artificial intelligence brings remarkable benefits and innovations. However, it is essential to understand the challenges that accompany this powerful technology. This section highlights key issues, including data privacy, bias in algorithms, and the potential economic impact of AI on jobs. Addressing these challenges is critical to ensure that AI benefits society as a whole, not just certain segments.
Data Privacy Concerns
Data privacy is a significant issue in AI development. AI systems rely heavily on data, often personal, for training and functioning. When companies collect and process large amounts of data, the potential for misuse increases. Individuals may not even know how their information is being used. This lack of transparency raises concerns over consent and the security of personal data.
Innovations like the General Data Protection Regulation (GDPR) aim to protect users' privacy. Businesses must navigate these regulations while still leveraging data for AI applications. Failure to respect privacy can lead to regulatory penalties and reputational damage. Therefore, achieving a balance between data utilization and privacy protection is vital for sustainable AI development.
"Protecting personal data is not just a legal obligation; it is a moral one."
Bias and Discrimination in AI Algorithms
Bias in AI systems can manifest in various ways. Algorithms are trained on historical data, which may contain inherent biases. If not recognized, these biases can lead to discriminatory practices. For example, in hiring processes, an AI system might favor candidates of a certain background, reflecting past inequalities. Such biases can have far-reaching implications, affecting opportunities for underrepresented groups.
Mitigating bias requires thoughtful consideration during the development process. Developers need to ensure diverse data sets and apply fairness metrics. Continuous monitoring is also crucial to identify and rectify biases as they emerge. Addressing these concerns fosters trust in AI technologies and ensures equitable outcomes across different societal sectors.
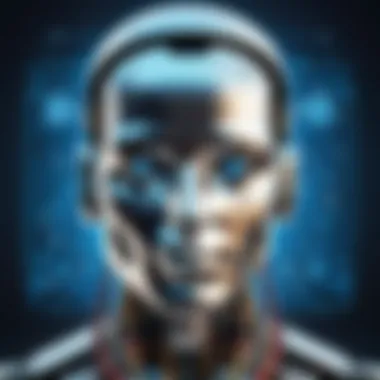
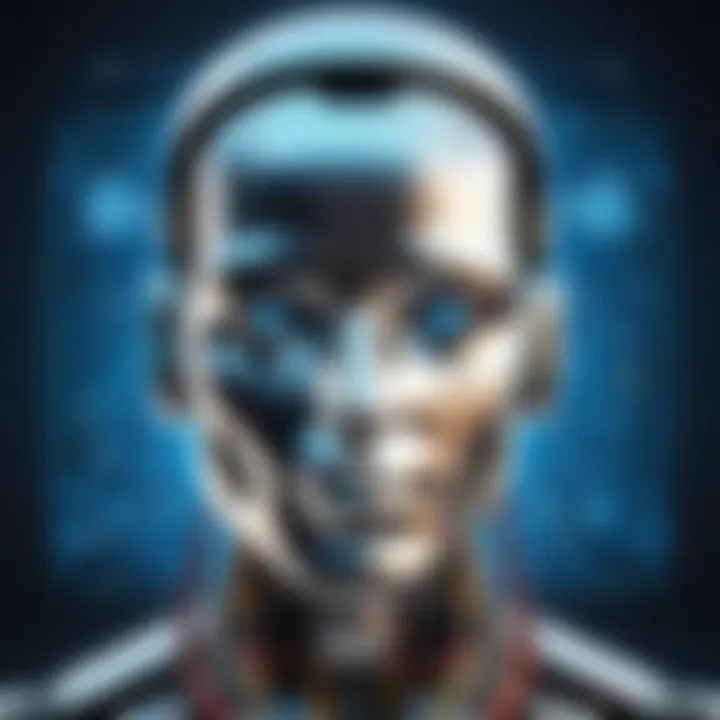
Job Displacement and Economic Impact
As AI technologies advance, concerns about job displacement grow. Automation and AI can perform tasks traditionally done by humans. This shift can lead to significant job losses, especially in industries like manufacturing, transportation, and customer service. The economic impact is twofold: while some jobs may vanish, new opportunities will arise in AI management and oversight.
Preparation is critical in this evolving landscape. Upskilling and reskilling programs can help workers transition into new roles that demand human ingenuity. Policymakers also need to consider the economic implications of AI, supporting innovation while providing safety nets for those affected by job displacement. Ultimately, a proactive approach can help mitigate negative consequences and build a competitive workforce.
Understanding these challenges in AI development is essential for responsible technological advancement. Addressing data privacy, bias, and job impact can lead to more inclusive and trustworthy AI systems that benefit all members of society.
Ethical Considerations in AI
Artificial intelligence brings transformative abilities and efficiencies to various aspects of life. However, along with this power comes the responsibility to address ethical considerations. In this section, we will explore why ethics in AI is critical. It encompasses several key factors such as transparency, accountability, and the impact of technology on society. Furthermore, ethical practices can lead to innovation and create trust among users and authorities. Understanding these components is vital to mitigate risks associated with AI deployment.
Transparency in AI Systems
Transparency is foundational in understanding how AI systems operate. Being clear about how these systems make decisions is crucial. It ensures that end-users understand the reasoning behind outputs. There is an increasing push for explainability in AI algorithms, especially in high-stakes areas like healthcare and criminal justice. When an AI system suggests a diagnosis or a legal outcome, individuals deserve to know how such conclusions were reached.
Some critical points regarding transparency include:
- Clear communication: Providing users with accessible information about AI processes.
- Auditability: Establishing methods to audit AI systems post-deployment.
- Documentation: Maintaining detailed records of AI training datasets and decision-making processes.
With transparency, biases in the system can be identified and addressed more effectively. This can lead to higher accuracy and better public confidence in AI applications. Failure to prioritize transparency could lead to misuse of AI technologies, damaging trust among stakeholders.
Accountability and AI Governance
The role of accountability in AI governance cannot be overstated. As AI systems take on decision-making roles traditionally held by humans, there arises the question of who is responsible for their actions. This is especially pertinent when systems make errors or cause harm. Accountability frameworks must be established to clarify responsibility and recourse.
Considerations for accountability include:
- Liability standards: Defining who is liable when AI fails – the developer, the user, or the organization.
- Regulation: Implementing policies that govern the usage of AI and ensure ethical compliance.
- Stakeholder involvement: Engaging various stakeholders, including ethicists, engineers, and affected communities, in the governance of AI technologies.
Effective governance can prevent exploitation and enhance the societal benefits of AI. The integration of ethics in AI development fosters responsibility and ensures systems serve all communities fairly and justly. Collaborations between tech companies, governments, and academia will be essential in shaping a future where AI can operate ethically and responsibly.
"AI technology holds immense potential, but its ethical implications must be considered seriously to maximize benefits while minimizing harm."
By addressing ethical considerations such as transparency and accountability, we not only improve AI algorithms but also contribute to a greater good where technology uplifts rather than undermines human values.
The Future of Artificial Intelligence
The future of artificial intelligence holds significant importance in the overall technology landscape. As industries increasingly embrace AI, understanding its trajectory is critical. AI can enhance problem-solving capacities, drive innovation, and improve efficiency in various sectors. Society should consider the implications of these advancements carefully.
Emerging Trends in AI Research
AI research is advancing rapidly, influenced by new trends and breakthroughs. Here are some critical areas of focus:
- Explainable AI: As AI systems become more complex, the need for transparency grows. Explainable AI seeks to clarify how algorithms make decisions. This not only boosts trust in AI but also aids in compliance with regulatory frameworks.
- Federated Learning: This approach enables multiple devices to collaboratively learn from datasets without sharing the data itself. This method addresses privacy concerns while increasing AI's collaborative potential.
- AI and Edge Computing: Moving AI processing closer to the data source reduces latency and improves response times. This trend supports applications requiring real-time insights, such as in autonomous vehicles or smart homes.
Research continues to evolve in these areas, enhancing AI's capabilities and applications.
Potential Impact on Society
The societal impact of AI is profound and multifaceted. Several perspectives warrant attention:
- Workforce Transformation: AI has the potential to reshape job markets. While some roles may become obsolete, others will emerge, necessitating reskilling and education.
- Ethical Considerations: As AI systems become integrated into more facets of daily life, ethical dilemmas surface. This includes biases in algorithms and privacy concerns. Society must navigate these challenges thoughtfully.
- Economic Growth: AI promises efficiency gains that can drive economic growth. By streamlining processes, businesses can allocate resources more effectively, potentially leading to new markets and opportunities.
The relationship between AI and society is not linear; it demands an ongoing dialogue to ensure beneficial outcomes.
Ending
In this article, we explored the complex landscape of artificial intelligence through various perspectives, emphasizing its significance and the role it plays across multiple industries. The concluding section aims to recap the foundational elements of AI and highlight the importance of ongoing research and ethical considerations within this domain.
Recap of AI's Significance
Artificial intelligence fundamentally alters how we interact with technology and processes. AI systems enhance efficiency, accuracy, and innovation across sectors such as healthcare, finance, and transportation. The applications discussed throughout demonstrate AI's versatility, from aiding in diagnostic procedures to optimizing resource management in public transport.
Moreover, AI contributes to decision-making processes that affect daily lives, driving advancements while also raising questions about transparency and accountability. Without a firm understanding of AI's significance, stakeholders risk overlooking the implications of these transformative technologies. Therefore, it is essential for tech enthusiasts and industry professionals to consider the broader effects of AI on society.
Call for Continued Research and Ethics in AI
As AI continues to evolve, the demand for comprehensive research and ethical frameworks becomes increasingly pressing. Understanding how AI can impact privacy, bias, and employment is crucial. Here are important considerations:
- Data Privacy Concerns: AI systems often require vast amounts of data. Ensuring that this data is collected and used responsibly is paramount.
- Addressing Bias: Various studies have shown that AI algorithms can exhibit bias. Research is needed to develop methods that mitigate these unfair biases.
- Job Displacement: The rise of AI might lead to job losses in certain sectors. Therefore, proactive strategies should be implemented to help workers transition to new opportunities.
In essence, the call for ongoing research and ethical practices in the AI domain is not just beneficial but necessary. It promotes an understanding of AI's capabilities and its risks, ensuring a responsible path forward in technology development.
“The future of AI lies not only in its technological advancements but also in its ethical deployment.” - Anonymous