Exploring the Diverse Landscape of Artificial Intelligence Models
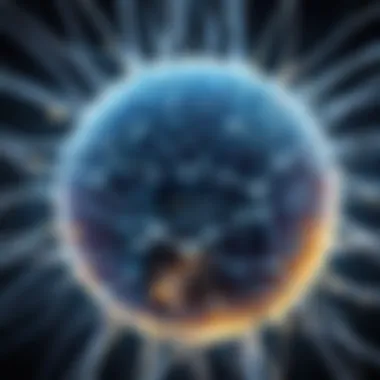
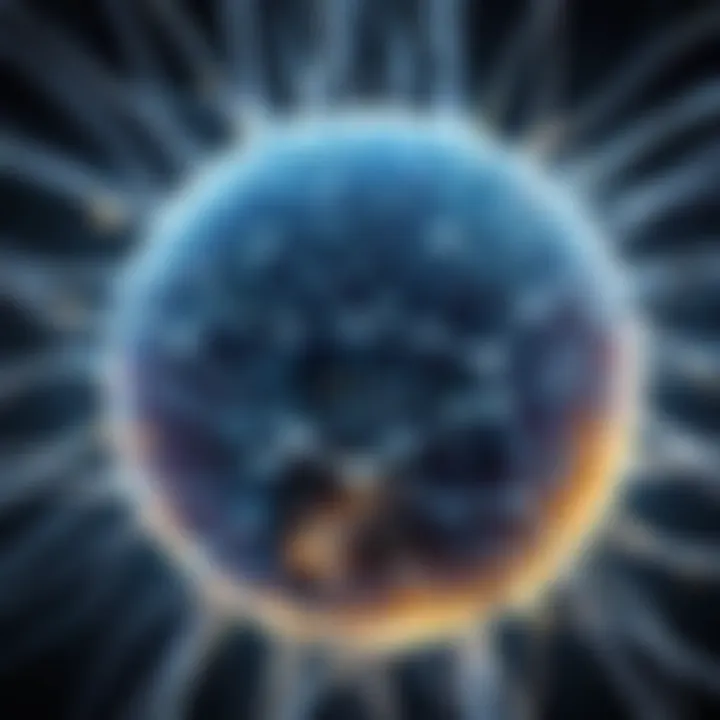
Tech Trend Analysis
Artificial intelligence has paved its path to becoming a prominent player shaping the current tech trends. Its influence is palpable in various sectors, from personalized recommendations on e-commerce platforms to autonomous vehicles navigating our roads. With the burgeoning advancements in AI technology, consumers find themselves amidst a wave of innovation that promises efficiency and convenience. The implications for consumers are substantial, offering tailor-made experiences and streamlined processes. Looking to the future, the possibilities seem boundless, with AI expected to revolutionize industries even further, presenting new opportunities and challenges alike.
Product Reviews
In the realm of artificial intelligence models, each offers a distinct set of features and capabilities that cater to specific needs. From the intricacies of supervised learning to the complex algorithms of reinforcement learning, AI models vary in their applications and effectiveness. Delving into the features and specifications of each model provides a deeper understanding of their functionalities. Performance analysis sheds light on their efficiency and accuracy, while weighing the pros and cons aids in making informed decisions regarding their deployment. Ultimately, offering recommendations based on a comprehensive evaluation is crucial for guiding individuals and businesses in leveraging these AI models effectively.
How-To Guides
Introducing novices to the intricacies of AI models requires a systematic approach that simplifies complex concepts without compromising on depth. Providing step-by-step instructions ensures a logical progression in learning about the nuances of each AI model. Sharing tips and tricks enhances the understanding of practical applications, fostering a hands-on approach to implementation. Troubleshooting common challenges equips enthusiasts with the necessary skills to navigate and adjust AI models according to specific requirements, promoting a robust comprehension of their functionalities.
Industry Updates
Amidst the rapidly evolving landscape of the tech industry, staying abreast of recent developments is paramount. Analyzing market trends provides insights into the dynamic nature of AI models, highlighting shifts in consumer preferences and technological advancements. The impact on businesses and consumers is profound, influencing decision-making processes and strategic planning. As AI continues to redefine industry standards, being informed about these updates equips professionals with the foresight to adapt and innovate in an ever-changing environment.
Foreword
In the fast-paced realm of technology, the advent of artificial intelligence (AI) models has revolutionized how systems interpret and respond to information. This article embarks on a journey to dissect and illuminate the intricacies of various AI models that drive innovation across industries. By unraveling the core concepts and practical applications of these models, readers will gain profound insights into the dynamic landscape of AI.
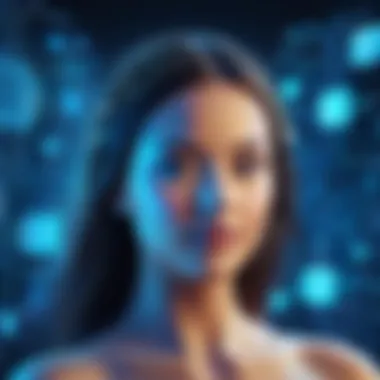
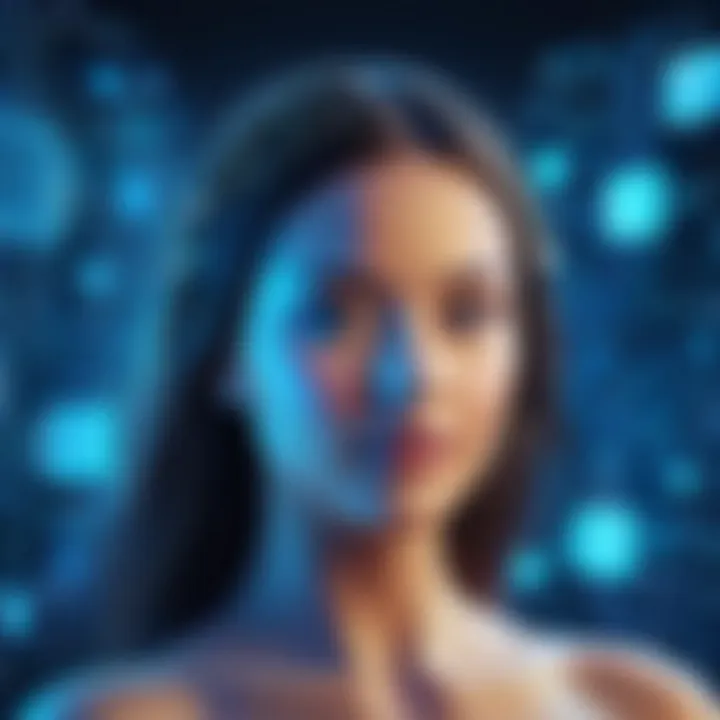
Defining Artificial Intelligence Models
Artificial intelligence models serve as the backbone of modern-day technological advancements, encompassing a wide range of algorithms and frameworks designed to replicate human decision-making processes. These models rely on algorithms to process vast datasets, identify patterns, and make decisions autonomously. By defining these models, we lay the groundwork for a comprehensive exploration of their functionalities and implications in diverse domains.
Significance of Understanding AI Models
Understanding AI models goes beyond surface-level comprehension; it is a gateway to unlocking the immense potential of AI in transforming industries and enhancing efficiency. Delving into the significance of comprehending AI models sheds light on how businesses can leverage these technologies to drive innovation, improve decision-making processes, and optimize operations. By grasping the intricacies of AI models, professionals can harness the power of AI to revolutionize their approaches and gain a competitive edge in the rapidly evolving tech landscape.
Types of AI Models
Exploring the types of AI models is crucial in understanding the intricate world of artificial intelligence. These models serve as the backbone of AI systems, encompassing a wide range of functionalities and applications that drive technological advancements. By delving into supervised learning, unsupervised learning, reinforcement learning, neural networks, genetic algorithms, and other models, one can grasp the diverse landscape of AI and its significance in shaping the future of technology.
Supervised Learning
Definition and Basics
Supervised learning stands out as a fundamental aspect of AI, where algorithms are trained on labeled data to make predictions or decisions. This foundational principle enables machines to learn patterns and relationships, paving the way for accurate forecasting and classification tasks. The key characteristic of supervised learning lies in the guidance provided by labeled datasets, allowing models to map input to output effectively. Despite its effectiveness, supervised learning faces challenges such as overfitting and the need for large amounts of labeled data.
Applications in Real World
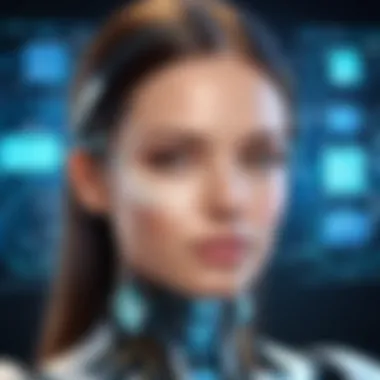
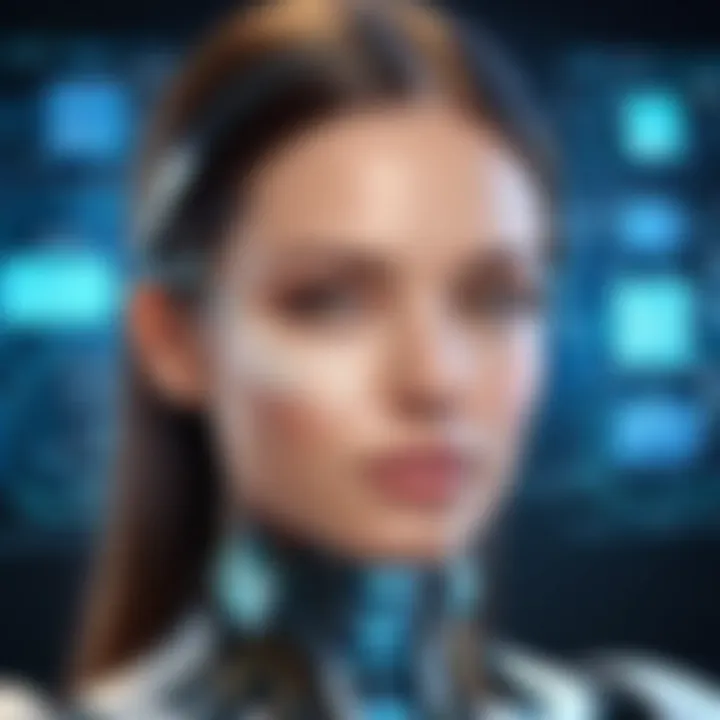
The real-world applications of supervised learning are vast and impactful. Industries leverage this model for tasks like image and speech recognition, spam detection, and predictive analytics. Its accuracy and efficiency make it a popular choice in healthcare for diagnosing diseases from medical images and in finance for predicting market trends. However, interpreting complex data and ensuring the quality of labels pose as challenges.
Challenges and Limitations
Challenges and limitations in supervised learning revolve around the dependence on labeled data, which can be expensive and time-consuming to acquire. Additionally, over-reliance on labeled datasets may hinder adaptability to new scenarios or outliers. Despite its effectiveness in certain domains, supervised learning may struggle with unstructured or ambiguous data, requiring further enhancements to handle diverse datasets.
Unsupervised Learning
Conceptual Overview
Unsupervised learning operates differently from supervised learning by extracting patterns and information without labeled data. This model identifies hidden structures within datasets, enabling clustering and dimensionality reduction. Its key characteristic lies in discovering inherent relationships, making it ideal for exploratory data analysis and segmentation tasks. The challenge with unsupervised learning lies in interpretability and evaluating model performance without explicit labels.
Use Cases
Unsupervised learning finds applications in anomaly detection, customer segmentation, and recommendation systems. Its ability to unveil previously unknown patterns enhances customer experiences and optimizes operational processes. However, the lack of clear objectives and feedback in unsupervised learning can pose challenges in determining the accuracy and relevance of outcomes.
Advancements and Future Prospects
The continuous advancements in unsupervised learning hold promise for revolutionizing data analysis and pattern recognition. Machine learning models are evolving to handle unsupervised tasks more effectively, opening doors for innovative solutions in various fields. However, the complexity of unsupervised algorithms and the need for substantial computing power present obstacles to widespread adoption.
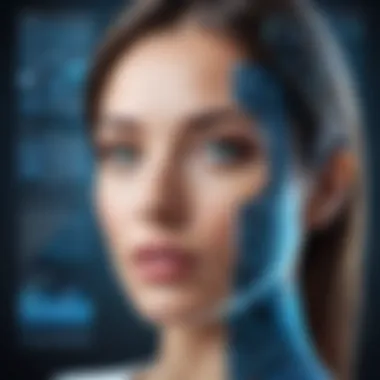
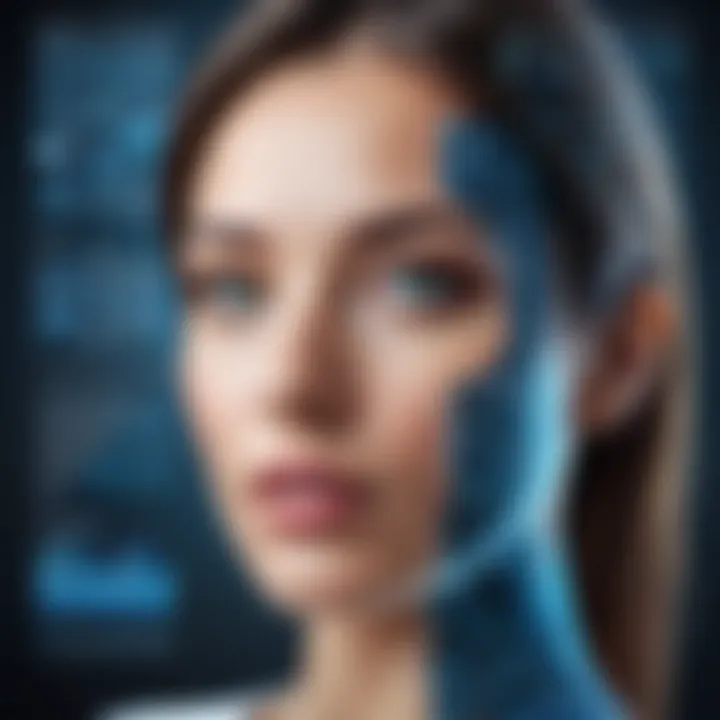
Reinforcement Learning
Fundamentals and Mechanisms
Reinforcement learning operates on a reward-punishment mechanism, where agents learn through interaction with an environment. This learning paradigm focuses on maximizing cumulative rewards by taking optimal actions based on feedback. The key characteristic of reinforcement learning lies in balancing exploration and exploitation to achieve long-term objectives. Despite its potential, reinforcement learning faces challenges such as large state spaces and defining suitable reward structures.
Industrial Implementations
Industries harness reinforcement learning for robotics, game playing, and autonomous systems to enhance decision-making processes. This model's ability to learn from trial and error accelerates automation and efficiency in various domains. However, the complexity of training reinforcement models and ensuring robust performance in dynamic environments pose as challenges.
Ethical Considerations
Ethical considerations in reinforcement learning are paramount to ensure fair and unbiased outcomes. As models make decisions affecting individuals and society, addressing issues of accountability and transparency becomes crucial. The balance between optimizing rewards and ethical considerations poses challenges in maintaining ethical standards while advancing the capabilities of reinforcement learning models.
Finale
In the realm of artificial intelligence models, the conclusion serves as a pivotal section that encapsulates the diverse landscape explored in this article. Understanding the implications and potential of different AI models is crucial for tech enthusiasts and industry professionals alike. The discussion surrounding the various types of AI models, from supervised to unsupervised learning, reinforcement learning, neural networks, and more, offers a deeper insight into the evolution of AI systems. By delving into the nuances of each model, readers can grasp the intricacies of their applications, limitations, and future prospects. This comprehensive overview instills a sense of appreciation for the complexity and capability of AI technologies, shedding light on the importance of continuous exploration and innovation in this dynamic field.
Implications of Diverse AI Models
The implications of diverse AI models are profound, shaping the way we interact with technology and the world around us. Each type of AI model brings unique capabilities and challenges, influencing how systems learn, adapt, and make decisions. From supervised learning's structured approach to training data to unsupervised learning's ability to uncover hidden patterns, AI models offer a spectrum of tools for solving complex problems. Reinforcement learning, with its focus on rewarding positive behavior, opens up possibilities for autonomous systems in various industries. Neural networks, inspired by the human brain, enable deep learning applications that revolutionize fields like image and speech recognition. Expanding our understanding of AI models allows us to harness their potential for innovation while navigating ethical considerations and societal impact.
Future Trends and Developments
Looking ahead, the future of AI models holds promising advancements and challenges. With rapid progress in machine learning algorithms and computing power, AI systems are becoming more sophisticated and pervasive. Future trends suggest a fusion of different AI models through hybrid approaches, leveraging their strengths synergistically. Enhanced natural language processing and computer vision capabilities are poised to transform how we communicate and perceive the world. Evolving towards explainable AI and ethical frameworks, the future of AI models emphasizes accountability, transparency, and fairness. As these technologies continue to evolve, it is imperative for researchers, developers, and policymakers to collaborate in guiding AI towards a human-centric and socially responsible future.