Exploring the Fusion of Trend Analysis and Data Science for Tech Advancements
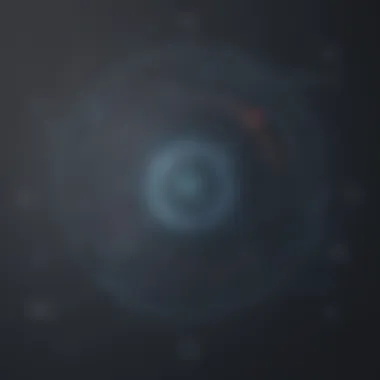
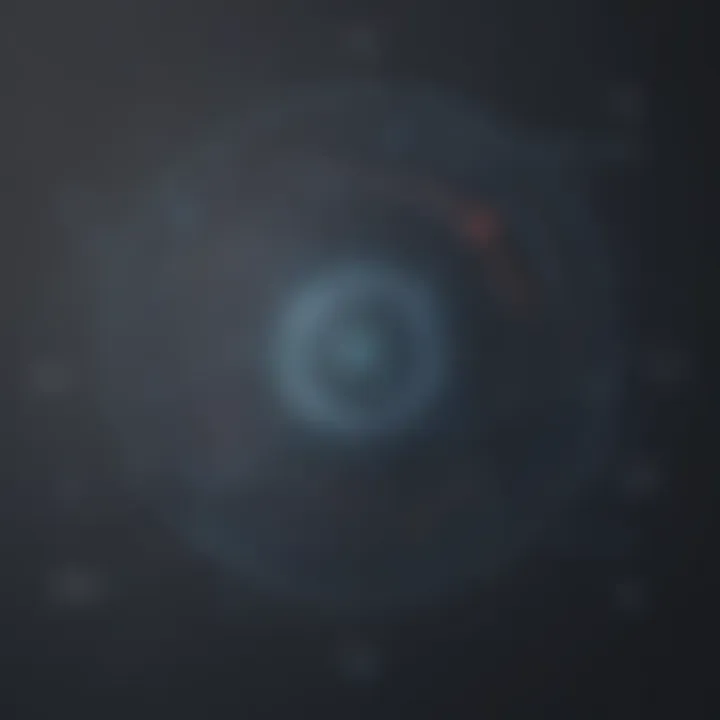
Tech Trend Analysis
In the dynamic realm of technology, keeping abreast of trends is paramount. Trend analysis, a pivotal facet in navigating the tech landscape, involves dissecting prevailing trends and forecasting future trajectories. The convergence of trend analysis and data science amplifies the insights gleaned, offering a comprehensive understanding of market dynamics. Consumers stand to benefit significantly from this synergy, as it enables companies to tailor products and services to evolving demands. Looking ahead, the amalgamation of these disciplines holds the promise of revolutionizing how tech trends are identified, harnessed, and applied.
Product Reviews
Delving into in-depth product reviews is integral to gauging the efficacy and appeal of technological offerings. By providing an overview of the product, outlining its features and specifications, conducting performance analyses, and assessing pros and cons, consumers gain invaluable insights to make informed purchasing decisions. Recommendations based on thorough evaluations add a layer of expert guidance to navigate the myriad choices available in the tech market.
How-To Guides
For tech enthusiasts seeking to enhance their proficiency in leveraging cutting-edge technologies, comprehensive how-to guides are invaluable resources. Introduction to complex topics, step-by-step instructions, incorporation of tips and tricks, and troubleshooting insights equip readers with practical knowledge and skills. With a concisely curated guide, individuals can explore new territories within tech with confidence and competence.
Industry Updates
The tech industry is a landscape characterized by rapid advancements and disruptions. Staying informed about recent developments, analyzing market trends, and assessing their impacts on businesses and consumers are crucial for adapting to the evolving tech landscape. By delving into industry updates, professionals gain strategic insights to position themselves advantageously amidst the ever-evolving tides of technology.
Introduction
In the vast landscape of tech and data analytics, the convergence of trend analysis and data science stands out as a beacon of innovation and opportunity. This article embarks on a riveting journey into the intricate interplay between these two domains, shedding light on their symbiotic relationship and transformative potential within the tech industry. By unraveling the crux of trend analysis and its fusion with data science, we are poised to unlock a realm brimming with insights, predictions, and strategic applications.
As we navigate through the realms of trend analysis and data science, a key objective is to demystify the underlying frameworks and methodologies that underpin these disciplines. Understanding the theoretical foundations of trend analysis equips us with a robust knowledge base to interpret patterns, forecast trends, and make informed decisions. Moreover, delving into the practical tools and techniques employed in trend analysis offers a nuanced perspective on how data-driven insights can drive strategic advancements in various sectors.
The essence of this article lies in dissecting how data science intertwines with trend analysis, amplifying the capabilities of both disciplines synergistically. By delving into the fundamentals of data science - encompassing data collection, statistical analysis, and machine learning algorithms - we pave the way for a deeper comprehension of its role in augmenting trend analysis. Furthermore, exploring the seamless integration of data science in trend analysis unravels a panorama of functionalities such as pattern recognition, forecasting trends, and detecting anomalies, which catalyze informed decision-making and strategic planning.
Through a comprehensive exploration of the applications of trend analysis and data science in the tech and business realms, we gain invaluable insights into consumer behavior analysis, product development strategies, competitive intelligence, market trend forecasting, risk assessment, and strategic decision support. These practical applications underscore the pivotal role that trend analysis and data science play in shaping the contemporary tech landscape and driving business optimization strategies.
The narrative of this article also delves deep into the challenges and future perspectives of trend analysis and data science, shedding light on overcoming limitations such as data quality issues, interpretation complexity, and ethical considerations. Additionally, by exploring emerging trends like real-time analytics, explainable AI, and privacy-preserving techniques, we gain foresight into the evolving paradigms that will define the future of these disciplines. Join us on this enlightening discourse on the intersection of trend analysis and data science, where possibilities abound and insights flourish.
Understanding Trend Analysis
Exploring the Foundations of Trend Analysis
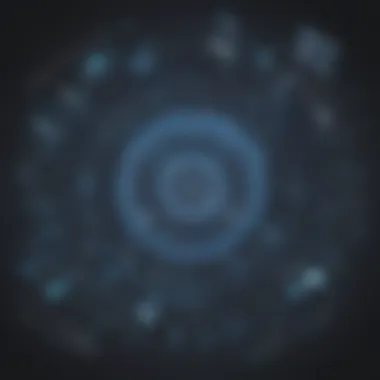
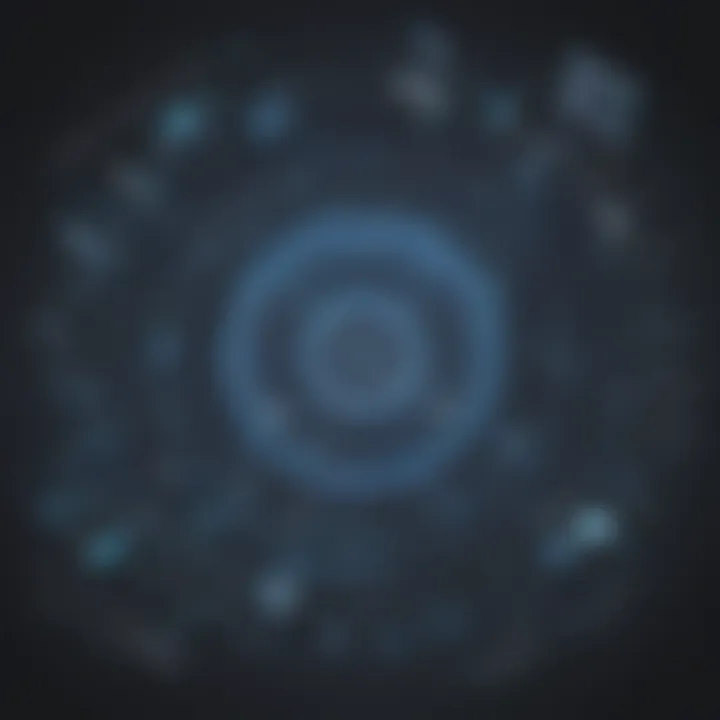
Theoretical Underpinnings
Exploring the Theoretical Underpinnings offers a profound understanding of the foundational concepts that drive trend analysis. These theoretical frameworks form the basis for interpreting data trends and making informed decisions. By delving into the fundamental principles of trend analysis, analysts can gain valuable insights into market dynamics and consumer preferences. While Theoretical Underpinnings provide a robust foundation for trend analysis, it is essential to acknowledge their limitations in capturing real-time market fluctuations.
Methodologies in Trend Identification
Examining the Methodologies in Trend Identification highlights the diverse approaches used to identify and analyze trends effectively. These methodologies range from statistical models to machine learning algorithms, each offering unique advantages in trend analysis. By utilizing a combination of methodologies, analysts can enhance the accuracy of trend predictions and derive actionable insights from complex data sets. However, the choice of methodology must align with the specific goals of trend analysis to optimize results.
Significance in Decision-Making
The Significance in Decision-Making underscores the pivotal role of trend analysis in shaping strategic decisions across industries. By leveraging trend analysis insights, organizations can mitigate risks, identify growth opportunities, and tailor their business strategies to meet evolving market demands. While trend analysis enhances decision-making processes, it is essential to interpret trends accurately and consider external factors that may influence outcomes.
Trend Analysis Techniques and Tools
Statistical Approaches
Statistical Approaches play a crucial role in trend analysis by quantifying trends and identifying significant patterns in data. These approaches utilize mathematical algorithms to analyze historical data trends and extrapolate future projections. While statistical approaches offer valuable insights into trend behavior, they may overlook non-linear trends or anomalies that deviate from conventional patterns.
Data Visualization Methods
Data Visualization Methods enable analysts to present complex trend data in a visually intuitive format, enhancing comprehension and decision-making. By using graphs, charts, and infographics, analysts can communicate trend insights effectively to stakeholders and identify patterns that may be overlooked in raw data. However, the effectiveness of data visualization methods relies on the selection of appropriate visualizations that align with the nature of the trend data.
Predictive Modeling
Predictive Modeling empowers analysts to forecast future trends based on historical data patterns and statistical models. By employing machine learning algorithms, analysts can build predictive models that anticipate market trends and support strategic decision-making. While predictive modeling offers valuable predictive capabilities, it is crucial to validate models regularly and adapt to changing market dynamics for accurate trend forecasting.
The Role of Data Science in Trend Analysis
Data science plays a pivotal role in the realm of trend analysis, intersecting two distinct yet closely related fields. In this article, the significance of data science in trend analysis is emphasized through its ability to harness and interpret vast amounts of data effectively. By delving into data science fundamentals, the article unravels the intricate processes involved in data collection, preparation, statistical analysis, and the application of machine learning algorithms specifically tailored for trend analysis. This comprehensive exploration sheds light on how data science acts as the cornerstone in unveiling valuable insights from trends in various domains.
Data Science Fundamentals
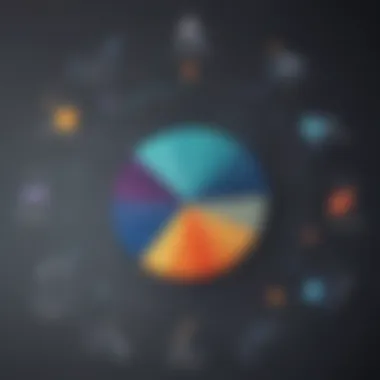
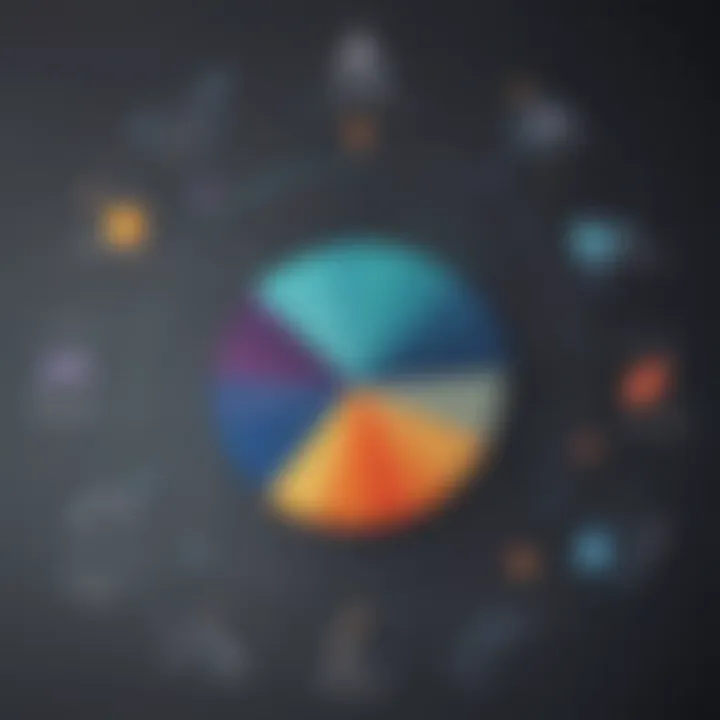
Data Collection and Preparation
Data collection and preparation are fundamental aspects of data science that underpin the efficacy of trend analysis. The meticulous process of collecting and organizing relevant data sets is imperative for yielding accurate and meaningful analytical results. The key characteristic of data collection lies in its ability to compile diverse sources of information into a structured format suitable for analysis. Despite the time and resource-intensive nature, data collection is indispensable in ensuring the reliability and comprehensiveness of trend insights. While this method enhances the depth of analysis, its limitations may include challenges in managing large datasets efficiently.
Statistical Analysis
Statistical analysis serves as the analytical backbone in deriving insights from trends identified through data science methodologies. By employing statistical techniques, trends are quantitatively evaluated, allowing for informed decision-making and predictive modeling. The key characteristic of statistical analysis lies in its ability to derive correlations, patterns, and probabilities from large datasets, aiding in trend identification and interpretation. While statistical analysis enhances the robustness of trend insights, its complexity may require a certain level of expertise to derive accurate conclusions.
Machine Learning Algorithms
Machine learning algorithms are instrumental in automating trend analysis processes, enhancing efficiency, and predicting future trends with a higher degree of accuracy. The key characteristic of machine learning algorithms lies in their capacity to learn from data patterns, adapt to new information, and optimize trend detection algorithms over time. By leveraging advanced algorithms, trend analysts can extract hidden patterns and insights, enabling proactive decision-making and trend forecasting. Despite their sophistication and performance benefits, machine learning algorithms may face challenges in interpretability and the potential for algorithmic bias that could impact trend analysis outcomes.
Integration of Data Science in Trend Analysis
Pattern Recognition
Pattern recognition is a critical aspect of integrating data science into trend analysis, enabling the identification of recurring trends and anomalies within datasets. The key characteristic of pattern recognition lies in its capacity to classify data patterns based on similarities and deviations, facilitating trend visualization and interpretation. By incorporating pattern recognition techniques, trend analysts can streamline data processing, enhance trend forecasting accuracy, and gain actionable insights for strategic decision-making. However, the reliance on patterns may overlook nuanced and outlier trends that require a more in-depth analysis.
Forecasting
Forecasting plays a pivotal role in trend analysis by providing invaluable insights into future trends and potential market shifts. The key characteristic of forecasting lies in its ability to extrapolate existing trend data, model future scenarios, and assess potential outcomes with a certain level of confidence. By utilizing forecasting methodologies, trend analysts can anticipate market trends, consumer behaviors, and competitive landscapes, contributing to informed decision-making and proactive strategy development. Despite its predictive power, forecasting techniques may encounter challenges in evolving market dynamics and unforeseen external factors that could impact trend accuracy.
Anomaly Detection
Anomaly detection serves as a crucial tool in identifying irregular patterns and outliers within datasets, enabling trend analysts to mitigate risks and address potential issues proactively. The key characteristic of anomaly detection lies in its capacity to flag unexpected deviations from normal data patterns, signaling anomalies that require further investigation. By integrating anomaly detection mechanisms, trend analysts can enhance data integrity, reduce false positives, and improve the overall reliability of trend analysis outcomes. However, anomaly detection methods may generate false alarms and require constant fine-tuning to differentiate genuine anomalies from noise in data streams.
Applications in Tech and Business
The section on Applications in Tech and Business delves into the crucial role that this topic plays within the broader context of trend analysis and data science. In the fast-paced and competitive tech landscape, understanding consumer behavior and product development strategies is paramount for success. By analyzing consumer behavior patterns, companies can tailor their products and services to meet specific needs and preferences, ultimately driving customer satisfaction and loyalty. Product development strategies, on the other hand, guide organizations in creating innovative solutions that address market demands effectively. Furthermore, competitive intelligence equips businesses with valuable insights into their competitors' strategies and market positioning, enabling them to make informed decisions and stay ahead of the curve.
Tech Industry Insights
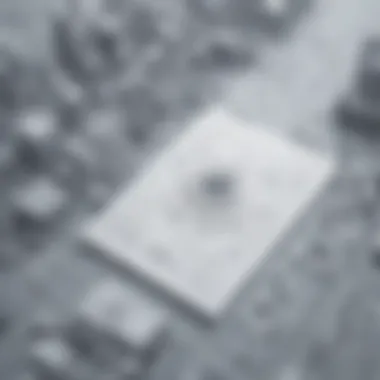
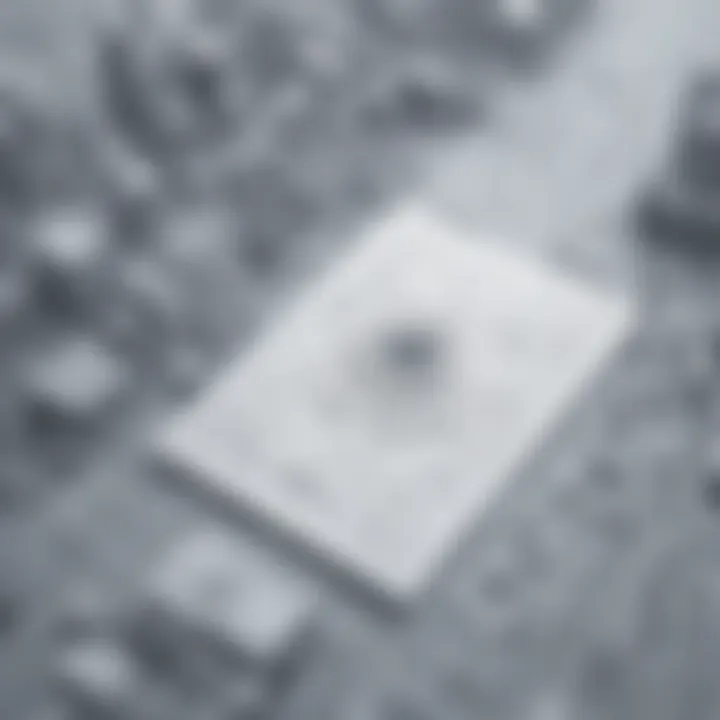
Consumer Behavior Analysis
Consumer Behavior Analysis is a fundamental aspect of understanding customers' purchasing habits, preferences, and decision-making processes. By analyzing consumer behavior data, businesses can identify trends, predict future buying patterns, and personalize marketing strategies. This analytical approach provides companies with a competitive edge by improving targeting, product positioning, and overall customer engagement. While Consumer Behavior Analysis offers valuable insights into consumer preferences and behaviors, its complexity lies in interpreting vast amounts of data accurately and ethically.
Product Development Strategies
Product Development Strategies are essential for creating successful and marketable products that meet consumer needs. By aligning product development with market trends and customer feedback, businesses can enhance product quality and differentiate themselves from competitors. These strategies involve research, innovation, and agile methodologies to adapt quickly to changing market dynamics. However, the challenge lies in balancing market demands with innovation to ensure products remain relevant and competitive.
Competitive Intelligence
Competitive Intelligence focuses on gathering and analyzing information about competitors to gain a strategic advantage in the market. By studying competitors' strengths, weaknesses, and market positioning, businesses can refine their own strategies and offerings. This intelligence helps organizations anticipate market trends, identify potential threats, and capitalize on opportunities effectively. Despite its benefits, maintaining accurate and up-to-date competitive intelligence can be resource-intensive and requires careful analysis and interpretation.
Business Optimization
The Business Optimization section explores how businesses can leverage trend analysis and data science to streamline operations, mitigate risks, and enhance decision-making processes. Market Trend Forecasting enables organizations to anticipate market changes, demand fluctuations, and consumer preferences, aiding in strategic planning and resource allocation. In contrast, Risk Assessment plays a crucial role in identifying and mitigating potential risks that could impact business performance. By evaluating internal and external risk factors, organizations can proactively address threats and optimize their risk management strategies. Strategic Decision Support provides decision-makers with data-driven insights and recommendations to make informed and strategic choices that align with business objectives and market dynamics.
Market Trend Forecasting
Market Trend Forecasting involves analyzing historical data and market trends to predict future events and developments accurately. By identifying patterns and extrapolating future trends, businesses can adapt their strategies and offerings to capitalize on emerging opportunities. This forecasting enables organizations to stay agile and responsive in dynamic markets, ensuring they remain competitive and relevant. However, the accuracy and reliability of forecasts heavily depend on the quality of data sources and the efficacy of analytical tools and methodologies.
Risk Assessment
Risk Assessment is a systematic process of identifying, evaluating, and managing potential risks that could affect business operations and objectives. By conducting comprehensive risk assessments, organizations can anticipate threats, assess their impact, and implement risk mitigation measures effectively. This proactive approach allows businesses to safeguard their assets, reputation, and sustainability against unforeseen events or challenges. Nonetheless, the challenge lies in forecasting unknown risks and addressing potential blind spots that could impact decision-making and operational resilience.
Strategic Decision Support
Strategic Decision Support systems provide decision-makers with data-driven insights, analysis, and recommendations to facilitate strategic planning and decision-making. By integrating data science capabilities, organizations can enhance the quality and accuracy of decision-making processes, leading to improved outcomes and performance. These systems enable executives to evaluate various scenarios, assess risks, and identify opportunities to inform strategic choices aligned with business goals. However, the effectiveness of strategic decision support depends on the availability of relevant data, robust analytics tools, and insightful interpretation to guide informed decision-making and optimize business strategies efficiently.
Challenges and Future Perspectives
In the realm of Trend Analysis and Data Science, exploring Challenges and Future Perspectives is crucial. This section delves into the obstacles that practitioners may encounter and the possibilities that lie ahead. Understanding these challenges is essential for making informed decisions and advancing the field. By addressing limitations and envisioning future trends, professionals can adapt strategies and enhance outcomes.
Overcoming Limitations
- Data Quality Issues: Data Quality is a fundamental aspect that impacts the reliability and effectiveness of analyses. Ensuring data accuracy, consistency, and completeness is imperative in generating meaningful insights. Addressing Data Quality Issues involves implementing robust validation processes, data cleansing techniques, and efficient storage solutions. By enhancing data quality, organizations can make informed decisions and derive precise predictions.
- Interpretation Complexity: The complexity of interpreting data poses significant challenges in Trend Analysis and Data Science. As datasets grow in size and complexity, extracting relevant trends and patterns becomes increasingly nuanced. Handling Interpretation Complexity requires employing advanced algorithms, visualization tools, and domain expertise. By simplifying complex data structures and modeling techniques, practitioners can uncover actionable insights and make informed decisions.
- Ethical Considerations: Ethical considerations play a pivotal role in Trend Analysis and Data Science. Ensuring ethical practices in data collection, processing, and usage is essential for maintaining trust and compliance. Addressing Ethical Considerations involves implementing transparent data policies, ensuring data privacy, and promoting responsible data usage. By upholding ethical standards, organizations can build credibility, foster customer loyalty, and mitigate potential risks.
Emerging Trends in Trend Analysis and Data Science
- Real-time Analytics: Real-time Analytics empowers organizations to extract insights and make decisions instantaneously. By analyzing data streams in real-time, businesses can respond to changing market conditions swiftly and effectively. Real-time Analytics offers the advantage of immediate feedback, enabling proactive decision-making and enhancing operational efficiency. However, managing real-time data streams and processing volumes pose scalability and latency challenges.
- Explainable AI: Explainable AI enhances transparency and interpretability in AI-driven decision-making processes. By providing explanations for AI outcomes and predictions, organizations can build trust and confidence in AI systems. Explainable AI enables stakeholders to understand the rationale behind AI decisions, facilitating informed choices and accountable AI usage. However, balancing between model complexity and interpretability remains a key focus in Explainable AI implementation.
- Privacy-Preserving Techniques: Privacy-Preserving Techniques safeguard sensitive data while enabling valuable analysis and insights. By using encryption, anonymization, and secure computation methods, organizations can protect individual privacy rights and comply with data protection regulations. Privacy-Preserving Techniques ensure data security and confidentiality, fostering trust among users and stakeholders. However, reconciling data utility and privacy protections presents ongoing challenges in the adoption of such techniques.