Choosing the Right Data Analysis Techniques: A Comprehensive Guide
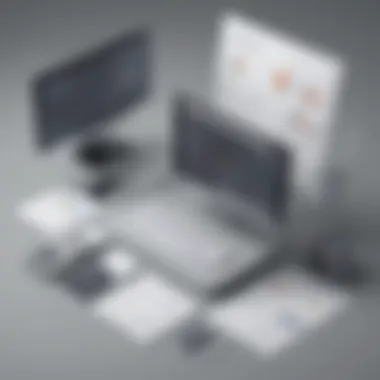
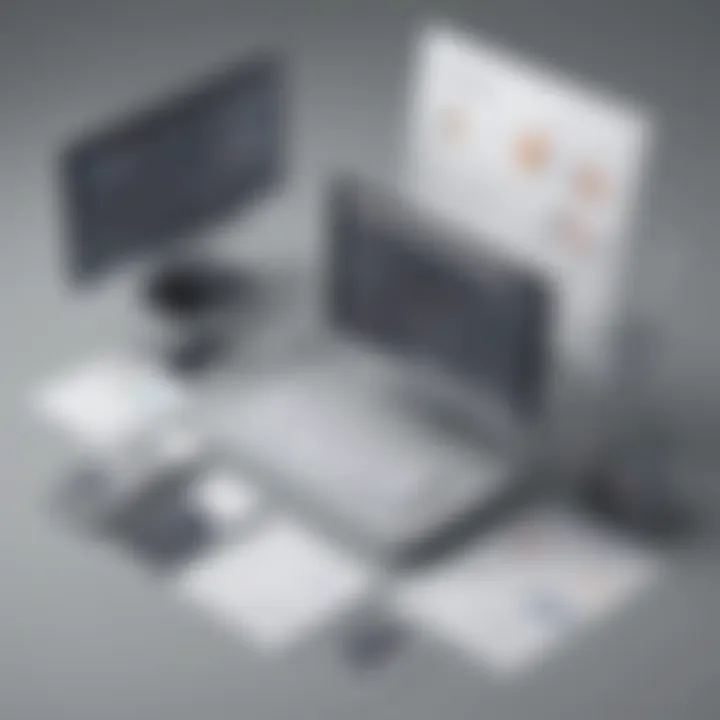
Tech Trend Analysis
In the realm of data analysis, understanding the current tech trends is paramount. By delving into the latest methodologies, professionals can gain crucial insights into consumer behavior. Observing these trends allows analysts to anticipate future directions and possibilities in the rapidly evolving landscape of data analytics.
Product Reviews
Navigating through the myriad of data analysis tools and methodologies can be overwhelming. Detailed product reviews provide an insightful overview of various analysis tools, highlighting their unique features, specifications, and performance indicators. By exploring the pros and cons of each tool, professionals can make informed decisions when selecting the most suitable techniques for their specific research needs. Recommendations based on comprehensive product analyses offer valuable guidance in selecting the optimal data analysis methods.
How-To Guides
For individuals new to data analysis, grasping the fundamental concepts is the initial step towards mastering the craft. How-to guides serve as an entry point by introducing beginners to the intricacies of data analysis. Offering step-by-step instructions, practical tips, and tricks, these guides simplify complex analytical processes for easy comprehension. Troubleshooting suggestions further aid beginners in navigating potential challenges that may arise during data analysis tasks.
Industry Updates
Staying abreast of the latest developments in the tech industry is essential for professionals seeking to optimize their data analysis strategies. Industry updates provide valuable insights into recent technological advancements, market trends, and their implications for businesses and consumers alike. By analyzing the evolving industry landscape, professionals can adapt their analytical approaches to align with emerging market dynamics, thus ensuring the relevance and efficacy of their data analysis techniques.
Introduction
Data analysis is a crucial aspect of any research endeavor, influencing decision-making and ultimately impacting business outcomes. In this comprehensive guide, we delve into the intricacies of selecting the right data analysis techniques. By exploring various methodologies and tools, this article aims to empower professionals and enthusiasts to make well-informed choices when it comes to data analysis.
Understanding the Importance of Data Analysis
The role of data analysis in decision-making
Data analysis plays a pivotal role in shaping decision-making processes. By deciphering patterns and trends within datasets, data analysis equips individuals and organizations with invaluable insights to guide strategic choices. Its ability to transform raw data into actionable information is a cornerstone of effective decision-making strategies. In this article, we shed light on the significance of data analysis in empowering decision-makers with the necessary analytics to drive impactful outcomes.
Impact of data-driven insights on business outcomes
Data-driven insights have revolutionized the business landscape, offering unprecedented opportunities for growth and optimization. By leveraging data to forecast trends and identify opportunities, businesses can streamline operations, enhance profits, and gain a competitive edge. The impact of data-driven insights on business outcomes cannot be understated. This article delves into how organizations can harness the power of data analysis to drive their bottom line and achieve sustained success.
Key Factors to Consider
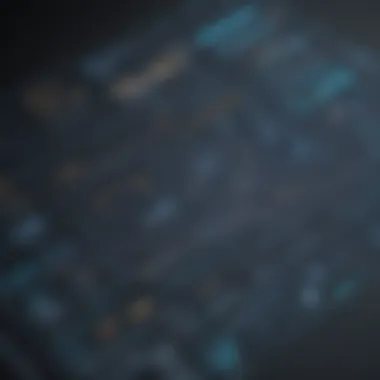
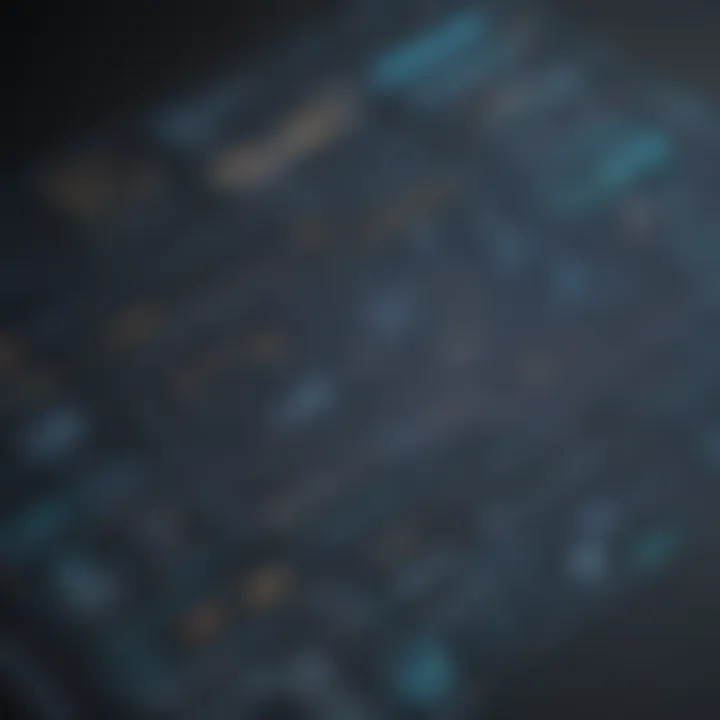
Nature of the data
The nature of the data under analysis significantly influences the choice of analytical techniques. Whether dealing with structured or unstructured data, understanding its intricacies is crucial for accurate analysis and interpretation. Factors such as volume, velocity, and variety of data play a vital role in determining the most suitable analytical approach. This section delves into the nuances of data characteristics and their implications on the data analysis process.
Research objectives
Aligning data analysis techniques with research objectives is imperative for achieving meaningful insights. The research objectives act as guiding beacons, directing the analytical focus towards answering specific questions or addressing predefined hypotheses. By aligning data analysis methodologies with research goals, researchers can extract relevant and actionable findings from their datasets. This article explores the pivotal role that research objectives play in shaping the data analysis journey and driving impactful outcomes.
Types of Data Analysis Techniques
Understanding the critical role data analysis techniques play in decision-making processes is fundamental for any professional or enthusiast delving into the realm of data analysis. In this comprehensive guide, we will explore the various types of data analysis techniques that cater to different research objectives, data characteristics, and analysis requirements. By diving into descriptive analysis, inferential analysis, diagnostic analysis, and prescriptive analysis, individuals can gain a holistic view of how data can be leveraged to extract valuable insights and drive strategic decision-making processes.
Descriptive Analysis
Descriptive analysis serves as the foundational pillar in data analysis, focusing on summarizing key features of the data set and establishing meaningful patterns within the information. By utilizing techniques such as creating frequency distributions, professionals can comprehend the central tendencies, dispersion, and shape of the data, providing crucial insights into the data's nature and structure. Summarizing key features of the data enables analysts to present information effectively and make informed decisions based on a comprehensive understanding of the dataset dynamics.
Summarizing key features of the data
Summarizing key features of the data involves condensing complex information into manageable summaries that highlight essential aspects of the dataset. This process simplifies data interpretation, allowing stakeholders to grasp critical insights swiftly. This method proves beneficial in identifying trends, outliers, and general patterns that guide further analysis and decision-making. Despite its advantages in simplifying complex data, there is a necessity to consider potential limitations, such as oversimplification of information or overlooking subtle nuances inherent in the dataset.
Frequency distributions
Frequency distributions provide a structured representation of the occurrence of values within a dataset, offering clarity on data distribution and probability density. By visualizing frequency distributions through histograms, professionals can discern the concentration of values around specific intervals and identify potential outliers. This method facilitates the identification of trends, anomalies, and distribution characteristics crucial for making data-driven decisions. While advantageous in providing a clear visual representation of data patterns, frequency distributions may occasionally oversimplify complex data relationships and obscure nuanced insights within the dataset.
Inferential Analysis
Inferential analysis extends beyond descriptive statistics to make inferences and predictions about a population based on sample data. By employing techniques like hypothesis testing and predictive modeling, analysts can draw meaningful conclusions and foresee future trends from existing observations. This mode of analysis empowers professionals to extrapolate insights beyond the dataset at hand, enabling strategic decision-making and proactive business planning.
Hypothesis testing
Hypothesis testing allows analysts to evaluate assumptions about a population parameter using sample data, guiding inferences and decision-making processes. By establishing hypotheses, conducting statistical tests, and interpreting results, professionals can validate or refute proposed claims with data-driven evidence. This strategic approach aids in drawing substantial conclusions based on empirical evidence, fostering a culture of informed decision-making and evidence-based practices.
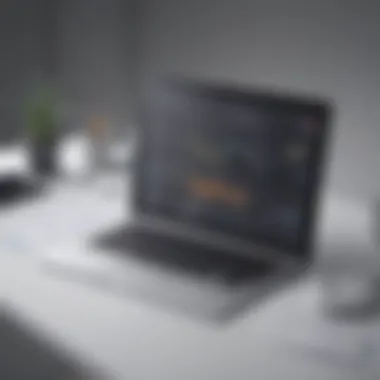
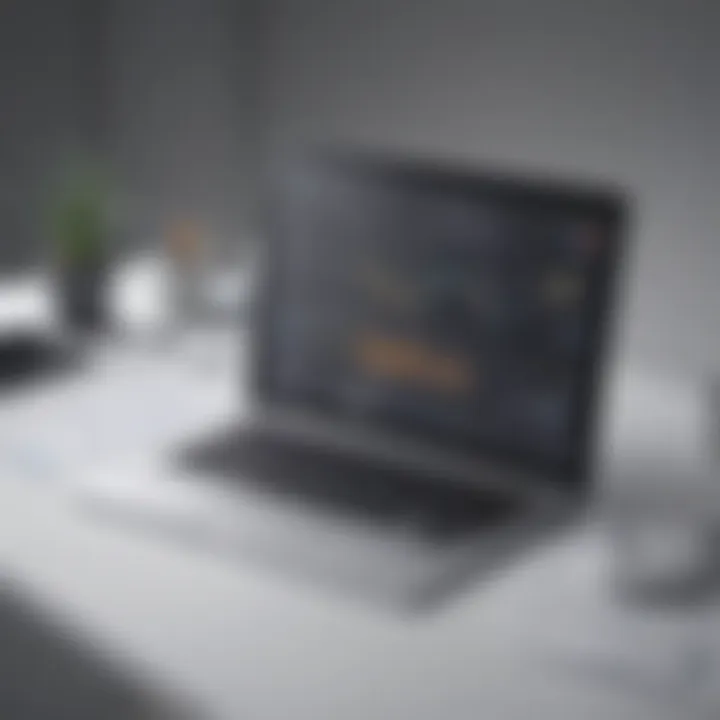
Predictive modeling
Predictive modeling leverages historical data to forecast future trends, behaviors, or outcomes with a significant degree of accuracy. By training models on existing datasets, professionals can develop robust predictive algorithms that anticipate changes and opportunities within the market or operational landscape. This predictive capacity equips decision-makers with foresight, enabling proactive strategies and preemptive measures to address potential challenges or capitalize on emerging opportunities.
Diagnostic Analysis
Diagnostic analysis delves into identifying patterns, anomalies, and cause-and-effect relationships within the dataset to uncover underlying trends or irregularities. Through techniques like anomaly detection and trend analysis, professionals can isolate critical factors influencing business operations and performance. By understanding these relationships, stakeholders can implement targeted interventions and optimizations to enhance operational efficiency and mitigate risks.
Identifying patterns and anomalies
Identifying patterns and anomalies involves scrutinizing data to detect recurring trends, irregularities, or deviations from expected behavior. By conducting thorough analyses and visual inspections, professionals can identify outliers, cyclical patterns, or atypical behavior that may impact decision-making processes. This diagnostic capability is instrumental in flagging potential issues, opportunities, or inconsistencies within the dataset, guiding actionable insights and remedial actions.
Cause-and-effect relationships
Cause-and-effect relationships examine the intricate connections between variables to grasp how one factor influences another within a system or process. By establishing causal links through statistical analyses or regression models, professionals can uncover the drivers behind specific outcomes or behaviors. This depth of insight aids in strategic planning, risk assessment, and performance optimization by addressing root causes rather than surface symptoms.
Prescriptive Analysis
Prescriptive analysis focuses on optimizing decision-making processes and enhancing operational efficiencies through data-driven recommendations and decision support systems. By emphasizing algorithmic optimization and recommendation engines, professionals can streamline complex decision-making processes and ensure informed, strategic choices that align with organizational objectives.
Optimizing decision-making processes
Optimizing decision-making processes involves developing algorithms and frameworks that optimize operational efficiencies and strategic outcomes. By leveraging prescriptive analysis, professionals can automate decision-making routines, improve resource utilization, and minimize human error. This analytical approach ensures that decisions are data-informed, efficiency-driven, and aligned with overarching business goals, enhancing organizational performance and competitiveness.
Recommendation systems
Recommendation systems utilize sophisticated algorithms to infer user preferences and deliver personalized recommendations tailored to individual needs and behaviors. By analyzing historical data and user interactions, recommendation systems can anticipate user interests, facilitate decision-making, and enhance user experience. Despite their efficacy in providing tailored suggestions and streamlining choices, recommendation systems may encounter challenges related to privacy concerns, data biases, and algorithm transparency, necessitating ongoing refinement and optimization.
Choosing the Right Technique
When delving into the world of data analysis, the significance of selecting the appropriate technique cannot be overstated. The process of choosing the right technique plays a pivotal role in the accuracy and relevance of the results obtained. By aligning analysis methods with specific research questions and objectives, professionals can ensure that their data analysis endeavors are purposeful and yield actionable insights. This section will explore how matching techniques to research questions is an essential step in the data analysis process, emphasizing the importance of strategic alignment for effective decision-making.
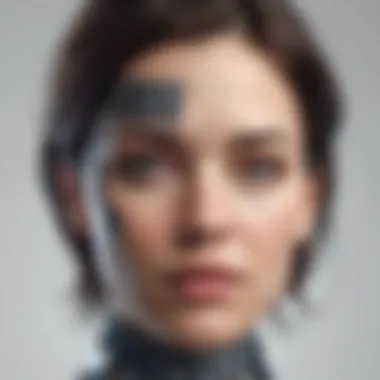
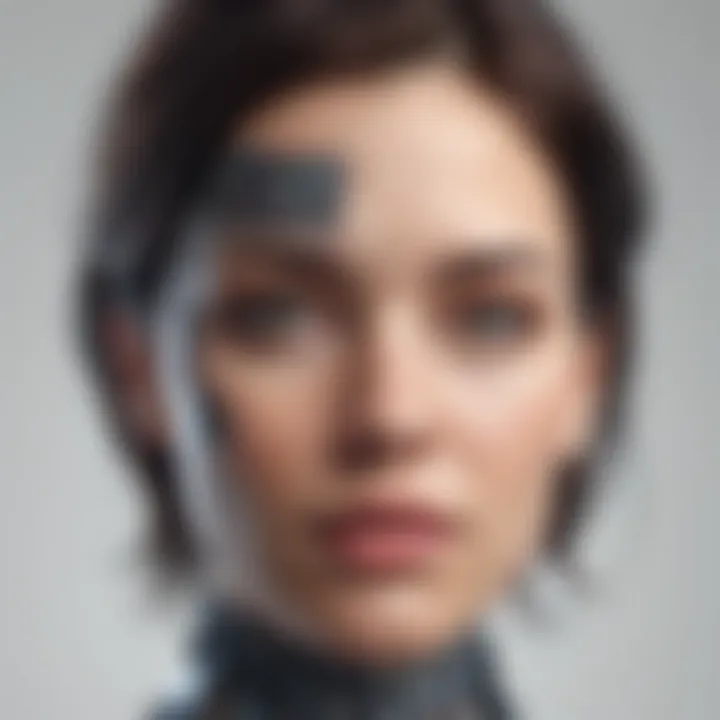
Matching Techniques to Research Questions
Aligning analysis methods with objectives
In the realm of data analysis, aligning analysis methods with research objectives is crucial for deriving meaningful conclusions. This approach involves customizing analytical techniques based on the specific goals of a research project, ensuring that the chosen methods are suitable for the desired outcomes. By aligning analysis methods with objectives, researchers can enhance the accuracy and relevance of their data analysis, leading to more insightful findings. The adaptability and precision offered by this approach make it a preferred choice for professionals seeking to optimize their analytical processes.
Considering Data Characteristics
In the context of data analysis, the characteristics of the data being analyzed play a fundamental role in shaping the analytical approach. Two key aspects that demand attention are continuous versus categorical data and data distribution. Understanding the distinctions between continuous and categorical data is essential as it influences the choice of analytical techniques and tools. Similarly, comprehending the data distribution provides valuable insights into patterns and trends within the dataset. Exploring these nuances equips analysts with the knowledge needed to make informed decisions and draw accurate conclusions from the data.
Continuous vs. categorical data
When evaluating continuous versus categorical data, analysts must consider the nature of the variables being examined. Continuous data consists of numerical values that can take any real number within a specific range, enabling precise measurement and analysis. On the other hand, categorical data comprises distinct categories or labels that represent qualitative characteristics. While continuous data offers granularity and precision, categorical data facilitates the classification and comparison of different groups within the dataset. Understanding the distinctions between these data types is essential for selecting the most appropriate analysis techniques and interpreting the results effectively.
Data distribution
The distribution of data refers to the way values are spread or dispersed within a dataset. Analyzing data distribution provides insights into the central tendencies, variability, and shape of the data, offering valuable information for decision-making. Different types of distributions, such as normal, skewed, or uniform distributions, can impact the choice of analytical methods and influence the conclusions drawn from the data. By examining the data distribution, analysts can gain a deeper understanding of the underlying patterns and structures within the dataset, enabling them to extract meaningful insights and derive informed conclusions.
Common Mistakes to Avoid
Overlooking Assumptions
When delving into the realm of data analysis, it is essential to be vigilant and steer clear of common mistakes that could potentially skew your findings. One crucial aspect to take into account is the significance of not overlooking assumptions. By disregarding the underlying assumptions of statistical techniques, the integrity and reliability of your analysis may be compromised, leading to erroneous conclusions and flawed decision-making processes. Emphasizing the meticulous scrutiny of assumptions can greatly enhance the accuracy and credibility of your research outcomes, ensuring that your insights are robust and trustworthy.
Impact of Violating Statistical Assumptions
In the context of data analysis, the impact of violating statistical assumptions plays a pivotal role in determining the validity of your results. By breaching these assumptions, you risk introducing bias, inaccuracies, and misleading interpretations into your analysis. This can have far-reaching consequences, influencing the overall quality and utility of your findings. Understanding the key characteristic of this phenomenon is paramount - it serves as a warning signal, highlighting potential pitfalls and prompting a more comprehensive evaluation of your analytical approach. Recognizing the implications of violating statistical assumptions can aid in refining your methodologies, fortifying the rigour of your analysis, and elevating the dependability of your research output.
Conclusion
Data analysis is a crucial aspect of any research or decision-making process. The ability to accurately interpret and draw insights from data can determine the success or failure of a project. In this article, we have delved deep into the various data analysis techniques available, highlighting their importance in different scenarios. By understanding the significance of selecting the right technique, professionals and enthusiasts can enhance the quality and accuracy of their research findings. This comprehensive guide aims to equip readers with the knowledge and tools necessary to navigate the complex landscape of data analysis effectively.
Key Takeaways
Importance of Informed Decision-Making in Data Analysis
Informed decision-making lies at the heart of effective data analysis. By incorporating informed decisions into the analytical process, researchers and analysts can ensure that their findings are robust and reliable. The emphasis on informed decision-making underscores the need for thorough research planning, clear objectives, and critical thinking skills. This approach not only enhances the quality of analysis but also boosts the credibility of results. In this article, the importance of informed decision-making is highlighted as a foundational principle for successful data analysis. By prioritizing informed decisions, professionals can mitigate risks, identify key trends, and make strategic choices that drive positive outcomes. The focus on informed decision-making serves as a guiding principle throughout this article, offering readers a solid framework for conducting effective data analysis.