In-Depth Analysis of Scientific Data Methodologies

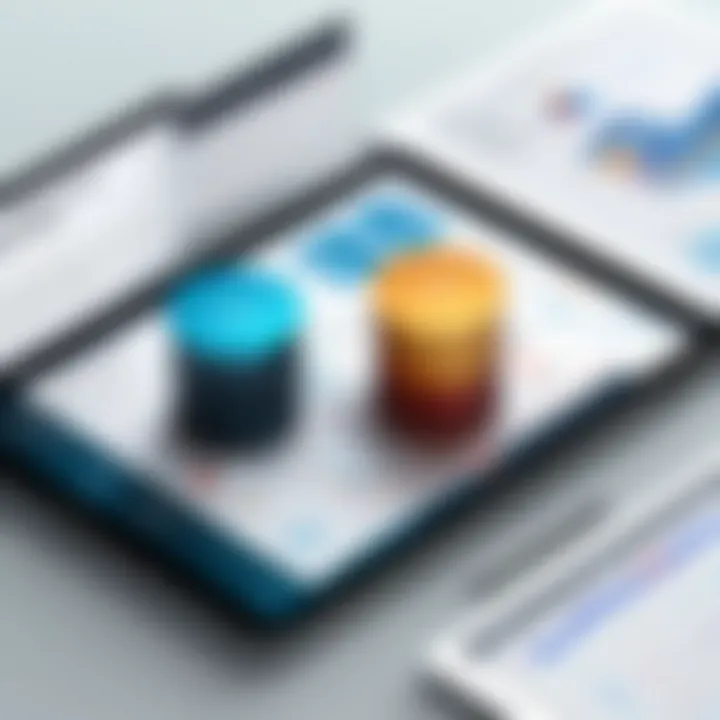
Intro
In the realm of scientific inquiry, understanding and analyzing data is akin to navigating a labyrinth without a map. Every collected datum is a potential treasure, holding insights waiting to be uncovered. The right methodologies enable researchers and analysts to sift through raw information, distilling it down into meaningful perspectives that can drive innovation, inform policies, and enhance our collective knowledge.
Scientific data analysis is not just a technical skill; it is a critical inquiry that can influence outcomes across various fields, from healthcare to environmental studies. Historically, the focus has leaned heavily toward overarching strategies, yet the nuances of qualitative versus quantitative methods have come to the forefront. This article explores these elements in depth. Whether it’s thriving in a bustling tech environment or investigating environmental shifts, the ability to analyze scientific data effectively inclines towards success.
"The goal is to turn data into information, and information into insight."
- Carly Fiorina
As we further dissect this topic, we'll delve into lively case studies, revealing how specific analytical techniques manifest in real-world applications. Not only will we highlight the importance of selecting appropriate frameworks and tools specific to unique research objectives, but we’ll also reflect on the challenges that frequently complicate this critical process. In doing so, we aim to illuminate various strategies that can bolster the validity and reliability of conclusions drawn from data.
Throughout this exploration, you will gain a clearer understanding of the driving forces behind scientific data analysis and how those forces ripple through industries and disciplines alike. Buckle up, as we journey into the heart of methodologies and applications of scientific data analysis, expanding our vision of what's possible.
Understanding Scientific Data
In the realm of scientific inquiry, understanding data is akin to possessing a compass for navigating uncharted waters. Scientific data is the lifeblood of research, providing evidence to support hypotheses and drive forward knowledge across disciplines. Grasping the nature and complexities of this data is essential for anyone involved in serious investigation, from seasoned researchers to budding scientists.
The Nature of Scientific Data
Scientific data, at its core, can be seen as a collection of facts and figures compiled to facilitate analysis. But it extends beyond mere numbers or comments; it encapsulates the contextual richness of the information being studied. Different forms of data exist: they vary in scale, the methods used in their collection, and their interpretation. This variety can improve the reliability of results and enhance the depth of insights gleaned from studies.
The data’s reliability also hinges upon its context. For instance, a statistic reflecting increased patient outcomes in a hospital may not simply reflect effective treatments; it may also indicate changes in operational procedures or patient demographics. Thus, situational awareness becomes paramount when interpreting data.
Categorizing Types of Data
Being able to categorize data effectively is a stepping stone to meaningful analysis. The distinction between qualitative and quantitative data shapes the direction of research. Each type serves its unique purpose, contributing differently to the body of scientific knowledge.
Qualitative Data
Qualitative data focuses on the essence of human experience. It captures perceptions, opinions, and emotions in ways numbers cannot. This type of data is often seen in case studies or interviews, where the aim is to gather rich, descriptive information.
A key characteristic of qualitative data is that it offers depth over breadth. Instead of providing a broad overview, it seeks to understand the nuances of a particular situation. This makes it a beneficial choice when the goal is to explore a phenomenon in detail or capture the subtleties of human behavior.
However, one must consider its subjectivity. While offering rich insights, qualitative data can be challenging to interpret consistently and can introduce bias into conclusions drawn. Researchers must remain vigilant to these potential pitfalls to ensure robust analysis.
Quantitative Data
Quantitative data, on the other hand, shines the spotlight on measurement and numbers. It provides a foundation for statistical analysis and is paramount for studies that require hard evidence of trends and patterns. This type of data is often collected through surveys, experiments, and observational studies that yield numerical results.
The attractiveness of quantitative data lies in its objectivity. By relying on numbers, researchers can apply statistical methods to validate their findings rigorously. This reproducibility is a cornerstone of scientific research. Yet, its rigidity can sometimes be a hindrance. Numbers might overlook the context behind the data, leading to conclusions that miss the broader narrative.
Discrete and Continuous Data
Beyond qualitative and quantitative categories lies the division between discrete and continuous data. Discrete data is characterized by specific, countable values – think of the number of patients in a study. In contrast, continuous data represents measurable quantities that can take on an infinite number of values within a given range, such as the temperature in a lab.
Understanding this distinction is vital. Discrete data can offer snapshots, while continuous data provides trends over time. This versatility enables researchers to select appropriate methods for data collection and analysis effectively. Each type carries its own unique advantages and challenges, dictating the methodology employed in studies.
In summary, understanding scientific data is crucial for harnessing its potential to drive inquiry. The categories of qualitative and quantitative data, along with their respective sub-types, enrich the landscape of analysis by providing varied insights. Informed choices regarding these forms facilitate the extraction of deeper meanings from data, paving a path to enhanced scientific understanding.
The Importance of Data Analysis in Science
Data analysis stands at the heart of scientific inquiry, transforming the vast amounts of raw data generated in various fields into actionable insights. Without diligent analysis, data remains a collection of numbers and words, lacking context and meaning. In today’s fast-paced world, discerning information from chaos is crucial, driving decisions that can influence a wide spectrum of outcomes, from healthcare to environmental policy.
Transforming Data into Insights
To truly appreciate the significance of data analysis, one must consider how it converts data into knowledge. This transformation process often begins with raw data being meticulously cleaned and organized. Imagine being handed a treasure chest, but finding it filled with broken shards instead of gleaming gold. Just like a savvy treasure hunter would arrange and examine each piece, scientists sift through data, removing inconsistencies before heading into deeper analysis.
- Identifying Patterns: Data analysis helps in spotting trends or patterns that might not be apparent at first glance. For instance, in healthcare, tracking patient recovery rates can uncover essential correlations between treatment protocols and outcomes. This places a spotlight on which methods may yield the best results.
- Drawing Conclusions: Through various statistical methods, scientists can draw conclusions that influence future research. Certain correlations or causations become evident through analysis, allowing informed decisions that push the disciplines forward.
- Communicating Findings: Well-analyzed data tells a story. By converting complex findings into visual representations such as graphs or infographics, presentations can not only engage the audience but also help in disseminating crucial knowledge effectively.
Driving Decision-Making Processes
While insights derived from data can be enlightening, the ability to influence decision-making cannot be overstated. Every industry today, from finance to healthcare, relies upon data-driven strategies to steer their paths. In essence, data analysis serves as a compass, guiding decisions that affect many lives.
- Informed Choices: Utilizing data analytics allows leaders to base their decisions on evidence rather than instinct. Consider a corporation deciding to launch a new product. If their analyses indicate that consumer preference is strongly leaning towards sustainability, they might adjust their strategy accordingly, ensuring product offerings resonate with customers.
- Risk Assessment: Through careful data evaluation, organizations can identify potential risks before they escalate into crises. In environmental science, analyzing historical climate data can enable researchers to forecast and mitigate natural disasters. Having a clear vision of potential challenges allows for better preparation.
- Strategic Direction: Data analysis provides a forward-looking perspective. It helps identify not just where we stand but where we need to go in the future. Organizations that leverage predictive analytics can anticipate market changes, adjust marketing strategies, or innovate new solutions preemptively.
"Data-driven decisions are the cornerstone of successful strategy in today’s interconnected world."
In summary, data analysis is not merely an auxiliary task in scientific research; it is a fundamental component that fosters insight, innovation, and growth across a variety of fields. It transforms raw figures into substantial knowledge, shaping decisions that can lead to far-reaching impacts across society.
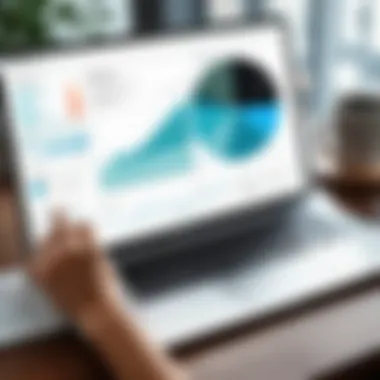
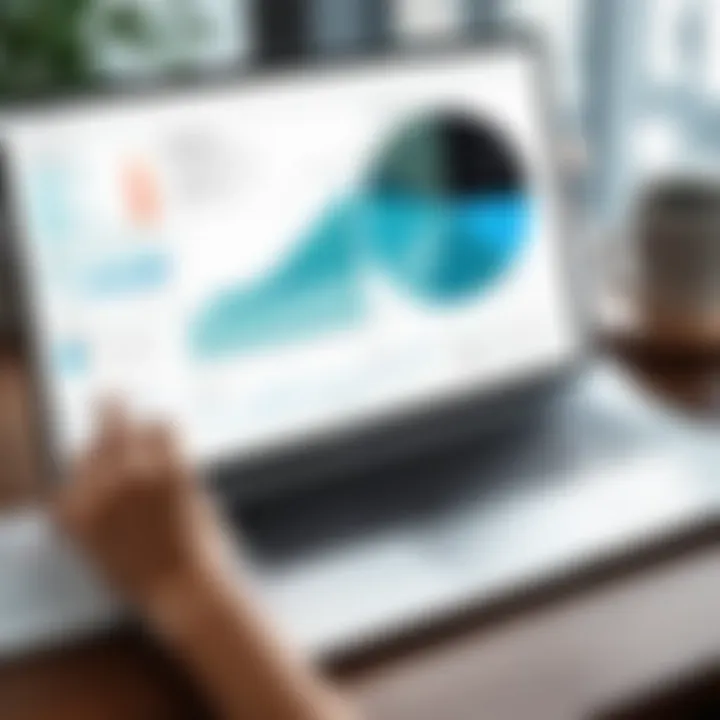
Common Methodologies in Data Analysis
In today's scientific landscape, the robust analysis of data is crucial for deriving meaningful insights, informing research decisions, and shaping future studies. Common methodologies in data analysis serve as the backbone for unlocking the potential hidden in raw data. Employing these methodologies effectively can significantly enhance the quality and relevance of scientific findings.
These methodologies introduce systematic approaches to handling large datasets, whether quantitative or qualitative. By applying the right technologies and techniques, researchers can glean deeper insights that inform a wide range of fields, from healthcare to environmental science. The incorporation of statistical analysis, machine learning techniques, and data mining approaches allows scientists to tailor their methodologies according to their specific objectives, ensuring more reliable and actionable outcomes.
Statistical Analysis
Statistical analysis plays a pivotal role in scientific data analysis, providing tools to summarize, explore, and infer relationships within data.
Descriptive Statistics
Descriptive statistics involves summarizing and presenting dataset features in a digestible format. This method simplifies complex data sets by showcasing essential characteristics such as mean, median, and mode. One significant aspect of descriptive statistics is its ability to provide a clear snapshot of the dataset, making it exceptionally useful in exploratory data analysis.
Its strong suit lies in its straightforwardness, which is why many researchers favor it as a starting point in their analysis. Statistics such as standard deviation and variance help in assessing the variability within the data, allowing researchers to comprehend their data's spread. However, descriptive statistics alone cannot lead to predictions or insights beyond the collected data, which may be seen as a drawback in some analytical contexts.
Inferential Statistics
On the other hand, inferential statistics delves deeper. This methodology allows scientists to draw conclusions beyond the initial dataset by applying techniques like hypothesis testing and confidence intervals. Inferential statistics thrives on samples to make generalizations about a larger population. Its popularity is fueled by its power to suggest trends and patterns, enabling researchers to make predictive claims based on their data.
With its unique feature of utilizing sample data to infer population parameters, inferential statistics comes with its challenges. Potential biases in sampling or misinterpretation of results can lead to misleading conclusions, which necessitates careful application and understanding.
Machine Learning Techniques
Machine learning techniques have emerged as game changers in the domain of data analysis, enhancing the ability to identify patterns and make predictions from large datasets.
Supervised Learning
Supervised learning is a core component of machine learning, allowing models to be trained on labeled datasets. This technique builds a function based on input-output pairs, honing its predictive capabilities. An important factor contributing to its usefulness is the fact that it can handle various types of data, be it images, text, or numerical information. Its application ranges broadly across industries—from predicting disease outbreaks in healthcare to custom-tailoring marketing strategies in business sectors.
The standout characteristic of supervised learning is the precision it can achieve in prediction tasks. However, it requires ample amounts of well-labeled data for training, which can sometimes be a limitation in specific fields where data labeling is labor-intensive or costly.
Unsupervised Learning
In contrast, unsupervised learning does not rely on labeled datasets. Instead, it identifies patterns and structures within input data by exploring inherent characteristics, such as clustering or association. This methodology is particularly beneficial when the insights are not predefined, allowing researchers to uncover hidden relationships or categories in the data.
The beauty of unsupervised learning lies in its flexibility. It can automatically identify segments within data without prior information, which can lead to innovative discoveries. Nonetheless, the challenge arises from difficulty in interpreting results, as it does not offer a clear path to validation like supervised methods do, potentially complicating the application of findings.
Data Mining Approaches
Lastly, data mining approaches draw from techniques across statistics, machine learning, and database systems. They are essential in discovering patterns and knowledge from large amounts of data.
Employing a mix of methodologies allows researchers to leverage data mining for tasks like predictive modeling and pattern recognition. As the field continues to evolve, staying abreast of innovative trends can propel scientists into new realms of understanding.
By understanding these common methodologies in data analysis, researchers and industry professionals can greatly improve their ability to derive insights from scientific data, paving the way for more informed decisions and richer scientific contributions.
"The ability to extract meaningful insights from data is pivotal for scientific advancement in any domain."
Choosing the right methodology, whether it's through traditional statistical means or cutting-edge machine learning, enables a comprehensive approach to tackling the challenges presented in scientific research.
Case Studies of Scientific Data Analysis
When it comes to scientific data analysis, the application of case studies stands out as a pivotal element. These case studies not only highlight real-world scenarios where data analysis techniques have been implemented, but they also serve as educational tools, illuminating the methodologies used in various fields. The benefits of examining case studies lie in their ability to provide concrete examples of how data analysis tangibly impacts decision-making and results across industries.
By showcasing specific instances of data analysis, researchers and practitioners can see the methodologies in action. This kind of insight can motivate others to adopt similar practices, enhancing the overall quality of scientific inquiry. Indeed, these case studies emphasize the interplay between data-driven insights and practical applications, fostering a culture of informed decision-making across the board.
Healthcare Analytics
Patient Outcome Studies
The aspect of Patient Outcome Studies is crucial as it centers on measuring the efficacy of medical interventions. By investigating the results of treatments, healthcare professionals can ascertain which methods lead to better patient health outcomes. What makes these studies particularly appealing is their ability to synthesize vast arrays of data, revealing patterns that may not be immediately obvious.
A key characteristic of Patient Outcome Studies is their reliance on longitudinal data. This time-based approach allows researchers to track a patient’s health journey over extended periods, providing a clear view of how treatment impacts overall well-being. This method has gained popularity due to its strong focus on real-life applications; healthcare systems greatly value insights that can enhance patient care.
One unique feature of these studies is their use of multi-faceted data sources, such as electronic health records, patient surveys, and clinical trial data. This richness of information offers advantages in identifying trends, although there are disadvantages in terms of data privacy and the need for robust analytical skills to interpret complex datasets.
Treatment Effectiveness
The specific aspect of Treatment Effectiveness delves into the comparison of different therapeutic approaches to determine their success rates. This evaluation contributes significantly to informed healthcare practices and policy decisions, fostering evidence-based medicine. The pivotal characteristic of this analysis is its rigorous methodology, which often employs control groups to isolate variables. Notably, it’s a beneficial choice for practitioners committed to improving patient care based on solid evidence.
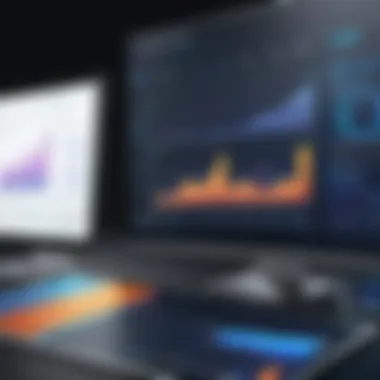
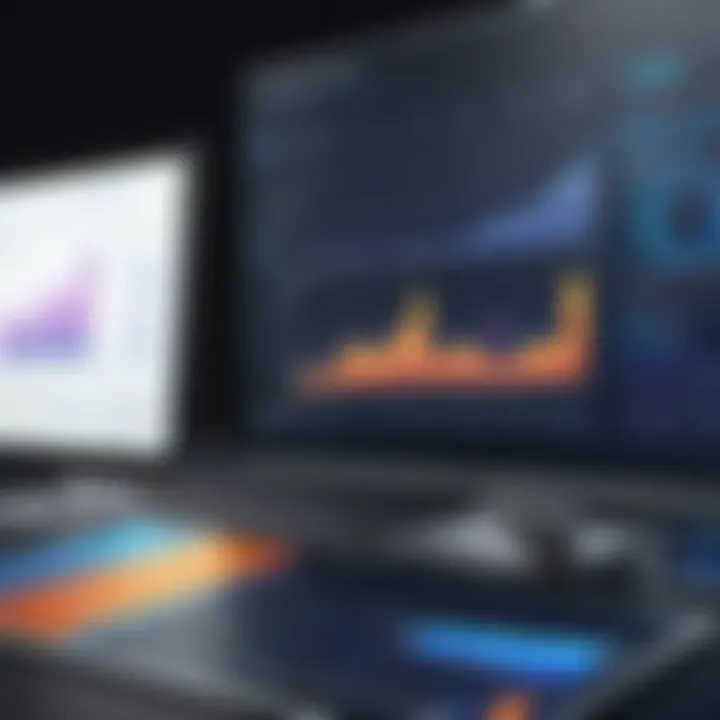
The uniqueness of Treatment Effectiveness studies lies in their highly structured framework, which strengthens the reliability of the outcomes. Such studies can rapidly inform stakeholders about best practices in treatment protocols, although there can be disadvantages like the high costs associated with conducting extensive trials and the ethical considerations involved in randomizing treatment groups.
Environmental Monitoring
Climate Change Data Analysis
Climate Change Data Analysis plays a vital role in understanding global shifts. This approach relies heavily on large datasets derived from satellite observations and ground measurements. Its contribution to scientific discourse is significant, as it helps decode trends that directly affect ecosystems and human life. A key characteristic is the focus on long-term data, providing insights that drive policy conversations around sustainability.
One beneficial aspect of this type of analysis is that it can leverage predictive modeling to forecast future climate scenarios, aiding in proactive measures. However, there's a unique challenge in that data can be subject to interpretation biases, which necessitates careful scrutiny to ensure that conclusions drawn are based on solid evidence.
Pollution Studies
The examination of Pollution Studies contributes to our understanding of environmental health. By analyzing data from various sources, stakeholders can identify pollution levels and their impacts on human and ecological systems. The defining characteristic of these studies is their ability to aggregate data from multiple pollutants over time and geography. This comprehensive approach is beneficial since it highlights cumulative effects that smaller studies might overlook.
Uniquely, Pollution Studies often integrate real-time monitoring technology, which enhances the credibility of findings. This method does, however, come with disadvantages regarding data collection, as limitations in sensor technology or coverage can affect overall accuracy.
Market Research
Consumer Behavior Analysis
Consumer Behavior Analysis focuses on understanding how individuals make purchasing decisions. This specific aspect is pertinent as it guides businesses in tailoring their products and marketing strategies effectively. A critical characteristic here is the application of both quantitative and qualitative research methods that facilitate a nuanced understanding of consumer motivations.
This analysis is uniquely beneficial as it blends psychological insights with market dynamics, giving businesses actionable strategies to engage their target audiences better. However, one must be wary of the complexities and variances in consumer behavior across different demographics, which can sometimes skew results.
Sales Trends Identification
Sales Trends Identification centers on analyzing historical sales data to forecast future performance. This aspect contributes immensely to strategic decision-making in businesses, helping them fine-tune inventory and marketing efforts. One key characteristic of this analysis is its predictive capacity, enabled by sophisticated statistical techniques.
The unique feature here is the ability to segment sales data by various criteria—such as regions, demographics, or time periods—enabling businesses to identify specific trends. The advantages of these methodologies are evident in enhanced operational efficiencies; however, disadvantages may include over-reliance on past data for forecasting, which can lead businesses astray if market conditions change abruptly.
"Embracing insights from case studies not only fosters a better understanding but also equips decision-makers with the necessary tools to navigate complexities in scientific data analysis."
In summary, these diverse case studies illustrate the broad applications of scientific data analysis. They underscore the methodologies that have been effective in various fields, revealing both their strengths and limitations. Through this exploration, one can appreciate how the analytical techniques employed have transformative potential—shaping not just theories but also tangible outcomes in healthcare, environmental sustainability, and market dynamics.
Tools and Software for Data Analysis
In the realm of scientific data analysis, having the right tools at your disposal can make or break your findings. These tools help researchers wade through the vast ocean of data, uncovering trends and insights that may otherwise remain hidden. Thus, understanding the significance of tools and software for data analysis is paramount. They not only enhance the efficiency of the analysis process but also improve accuracy, allowing for more reliable outcomes that can stand the test of scrutiny.
Statistical Software Packages
Statistical software has become the backbone of data analysis in various fields, offering robust frameworks to tackle different analytical challenges. Here, we delve into two prominent examples: R Language and SPSS.
R Language
R Language stands out due to its open-source nature. It’s often favored for its flexibility and extensive package ecosystem, which means users can customize functionality tailored to their analysis needs. This adaptability is crucial, particularly in a scientific setting where unique data types and research questions abound.
The key characteristic of R is undoubtedly its programming capabilities; it allows for intricate statistical analyses and creative data visualizations. Researchers can explore complex datasets that traditional software might struggle with. For instance, the ggplot2 package within R provides powerful tools for data visualization, turning raw numbers into insightful graphical representations.
However, R does come with a notable steep learning curve for those not accustomed to coding. Some users may find it challenging to navigate at first. Despite this, its rich functionality and supportive community often outweigh the initial hurdles, contributing significantly to its popularity in the scientific community.
SPSS
SPSS, on the other hand, is renowned for its user-friendly interface, making it accessible for non-programmers. It's often employed in social science research, healthcare analytics, and market research due to its strengths in descriptive statistics and regression analyses.
What makes SPSS particularly appealing is its straightforward point-and-click interface, which allows users to conduct analyses without deep technical knowledge. This feature expands its accessibility to a broader audience, from students to seasoned professionals.
Nonetheless, SPSS can be more restrictive compared to open-source alternatives like R. Its high licensing costs may deter independent researchers or smaller institutions from utilizing its full capabilities. Furthermore, while it has substantial predictive analytic options, users might find themselves limited if they wish to customize analyses beyond provided templates.
Programming Languages for Data Analysis
As data complexity grows, utilizing programming languages can offer even greater control and customization. Python and MATLAB are among the key players in this domain.
Python
Python shines in its versatility. It is a go-to language in many fields because of its readability and the vast array of libraries dedicated to data analysis, like Pandas and NumPy. These libraries simplify data manipulation and allow for efficient numerical calculations, which are essential in scientific inquiries.
A significant advantage of Python lies in its extensive community support, making troubleshooting and learning much easier. Moreover, the integration of visualization tools like Matplotlib and Seaborn further enhances its capabilities. Researchers can perform complex analysis and visualize results in one fluid workflow.
Yet, Python may require some preliminary coding knowledge. New users might feel overwhelmed by the numerous libraries and tools available, which can be daunting at first.
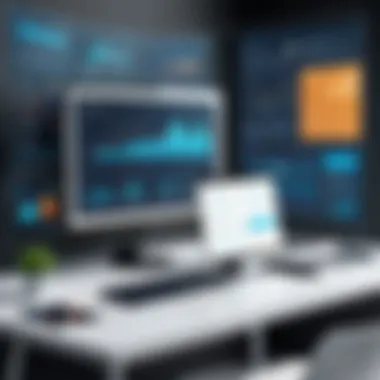
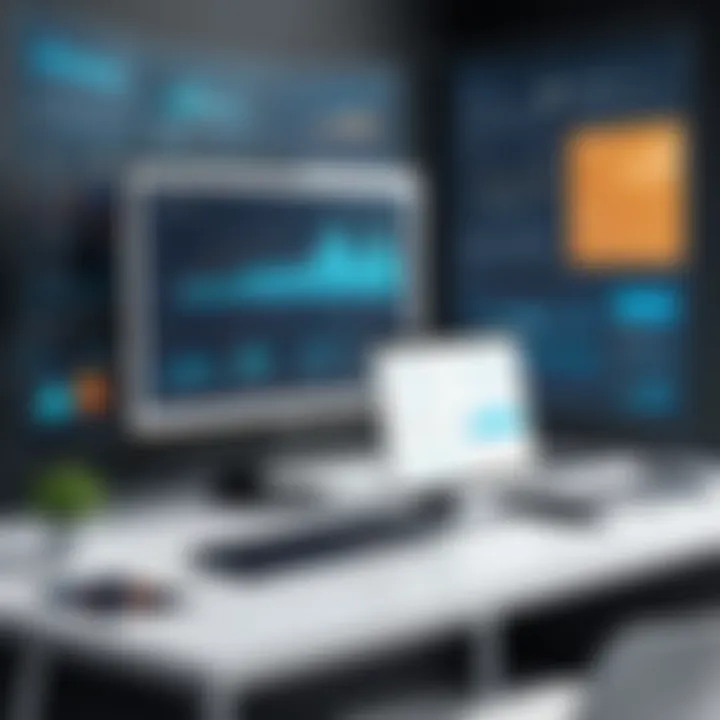
MATLAB
MATLAB is distinguished by its powerful computational abilities, primarily used in engineering and scientific research. Its integrated environment promotes matrix computations, making it ideal for numerical analysis and advanced data manipulation.
What sets MATLAB apart is its built-in functions for mathematical modeling and simulation, which are particularly valuable in fields like physics and engineering where simulations are essential. In essence, it combines simplicity and robust analytical power, fostering a conducive environment for data analysis.
However, MATLAB is not free. Its licensing fees can be prohibitive for many researchers, especially in academia. Learning MATLAB also requires navigating its specific commands and functions, which can be a steep climb for those who are not familiar with programming.
Visualization Tools
Data visualization is crucial in making sense of complex datasets, and tools like Tableau and Power BI play pivotal roles in translating intricate data into visual insights.
Tableau
Tableau excels in data visualization and is widely adopted for its intuitive drag-and-drop interface, allowing users to create interactive dashboards with ease. It's particularly beneficial for those looking to present findings to stakeholders. Through its real-time data analytics capabilities, it helps users quickly grasp key trends and patterns.
A unique feature of Tableau is its ability to connect to multiple data sources effortlessly, making it an excellent choice for businesses needing to analyze data from diverse origins. On the flip side, while Tableau’s ease of use is a plus, the depth of analytical capability can feel limited compared to complex statistical software.
Power BI
Power BI, developed by Microsoft, is another powerful tool for data visualization. It integrates seamlessly with other Microsoft applications, making data consolidation and analysis straightforward for users who are already embedded in the Microsoft ecosystem. Its features are geared toward business intelligence, providing insightful dashboards that can inform strategic decisions.
Nevertheless, Power BI can be restrictive when it comes to advanced analytics. Users searching for in-depth statistical functions may find it lacking in comparison with R or Python. Additionally, while it is user-friendly, those entirely new to data visualization software may require some time to become familiar with its features and functionality.
Ethics and Challenges in Data Analysis
In an age where data reigns supreme, the ethics and challenges in data analysis are becoming not just relevant but crucial to conversations surrounding scientific integrity. As researchers, analysts, and industry professionals delve into the world of data, they must tread carefully, balancing innovation and responsibility. This section seeks to illuminate the significant ethical concerns and challenges that come into play when analyzing scientific data.
Data Privacy Concerns
One of the foremost ethical considerations in data analysis is data privacy. The collection and use of data can involve sensitive information about individuals, particularly in sectors like healthcare and finance. Analysts must navigate a complex landscape of regulations and best practices to ensure they do not violate privacy rights.
Imagine an analyst working on patient data from a healthcare provider. They aim to identify trends related to treatment effectiveness. In doing so, they must ensure that personal identifiers are stripped away from the data sets or anonymized properly to prevent any potential breaches of patient confidentiality. The arrangement and structuring of data become imperative—compliance with laws like GDPR (General Data Protection Regulation) in Europe or HIPAA (Health Insurance Portability and Accountability Act) in the U.S. is no laughing matter. Analysts should consistently ask whether the data handling methods could expose personal details to unwanted prying eyes. Any misstep here isn't just a breach of trust; it can lead to legal ramifications for the institution as a whole.
"Once compromised, the trust vested in data can shatter beyond repair, impacting both individuals and entire organizations."
Furthermore, with the rise of big data, the volume and variety of data being processed have exponentially increased. This challenges analysts to keep their ethical guard up. There's often a temptation to squeeze every ounce of information from a data set, raising the risk of overlooking privacy issues. Thus, implementing strong ethical frameworks during the data analysis process isn't just recommended—it’s essential.
Bias in Data Interpretation
Another critical challenge lies in bias in data interpretation. Bias can seep into the data collection process, the analysis methods, or the cognitive biases of the analysts themselves. For instance, consider a scenario where a research team is studying the effects of a particular drug across various demographics. If the sample population is predominantly made up of individuals from a single demographic group, the results may not represent the wider population. Consequently, this could lead to skewed interpretations that affect treatment recommendations and outcomes in real-world settings.
The issue of bias is more than just about recognizing discrepancies in data; it's about validating the very processes that lead to interpretation. Analysts need to be acutely aware of their preconceptions and the way they might shape outcomes. Implementing diverse teams that bring varied perspectives can play a crucial role in mitigating this type of bias. Every member can act as a sounding board, enriching discussions and challenging assumptions.
Moreover, there lies a broader responsibility on analysts to assess the impact of their interpretations. A biased interpretation can lead to faulty conclusions, affecting public perception, policy decisions, and ultimately the direction of future research. This calls for a rigorous commitment to transparency in both methods and conclusions drawn from data analysis.
Overall, as data continues to shape our world and drive decisions, the demand for ethical considerations and awareness of bias will only grow. Ensuring responsible practices can contribute not only to the integrity of scientific research but also to the trust of the public in its outcomes.
The Future of Scientific Data Analysis
The landscape of scientific data analysis is evolving rapidly, driven by advancements in technology and the growing volume of data generated across all fields. As researchers and industries alike grapple with an explosion of information, understanding why the future of data analysis is crucial cannot be overstated. Embracing new methodologies and tools will not only enhance our ability to make informed decisions but also push the boundaries of what we can discover.
Emerging Trends in Data Analytics
Artificial Intelligence Integration
Incorporating artificial intelligence into data analysis is no longer just a novelty; it's becoming a standard in effective analysis strategies. This integration lets us sift through vast datasets with speed and precision. AI can identify patterns that might be invisible to the naked eye, providing a distinct advantage in areas such as predictive analytics.
A key characteristic of AI integration is its adaptability. As more data becomes available, AI systems can learn and improve their algorithms, increasingly refining their analyses. This self-improvement aspect makes AI a popular choice, particularly in environments where data is both abundant and complex.
However, it’s essential to acknowledge the unique feature of bias in AI algorithms. If the training data is skewed, the AI outputs can also become biased, leading to potential misinterpretations. While AI holds promise, users must remain vigilant to ensure the integrity of insights generated through these systems.
Big Data Applications
The concept of big data centers around the handling of massive datasets that traditional data-processing software cannot manage efficiently. As industries lean more towards data-driven strategies, big data analytics becomes an invaluable tool for determining trends, preferences, and outliers.
A noteworthy characteristic of big data applications is their ability to analyze diverse types of data—from structured to unstructured. This versatility enables researchers to gain insights across various domains, making it an attractive option in the contemporary analysis landscape.
Nevertheless, like any other tool, big data has its downsides. The sheer volume and complexity can pose significant challenges related to data management and integrity. Ensuring accuracy and reliability in big data applications is crucial, as misinterpretations can lead to erroneous conclusions that impact decision-making.
The Role of Interdisciplinary Collaboration
Science is no longer the sole purview of individual disciplines. The increasingly interconnected world necessitates interdisciplinary collaboration. Professionals from diverse fields bring unique perspectives to the table, enriching data analysis. Combining expertise—from statisticians to domain specialists—fosters a more holistic understanding of data, which can unveil richer insights than what isolated approaches might reveal.
This collaboration is particularly vital as the nature of scientific inquiries grows more complex. While traditional approaches have their merit, blending methodologies can drive innovation and open new avenues for research. The future of scientific data analysis hinges on our ability to work across boundaries, integrating knowledge and skills to navigate an ever-changing data landscape.