Exploring the Capabilities of Question-Answering Robots
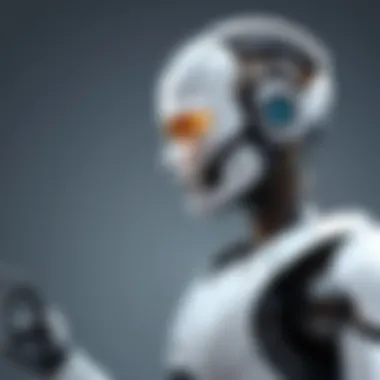
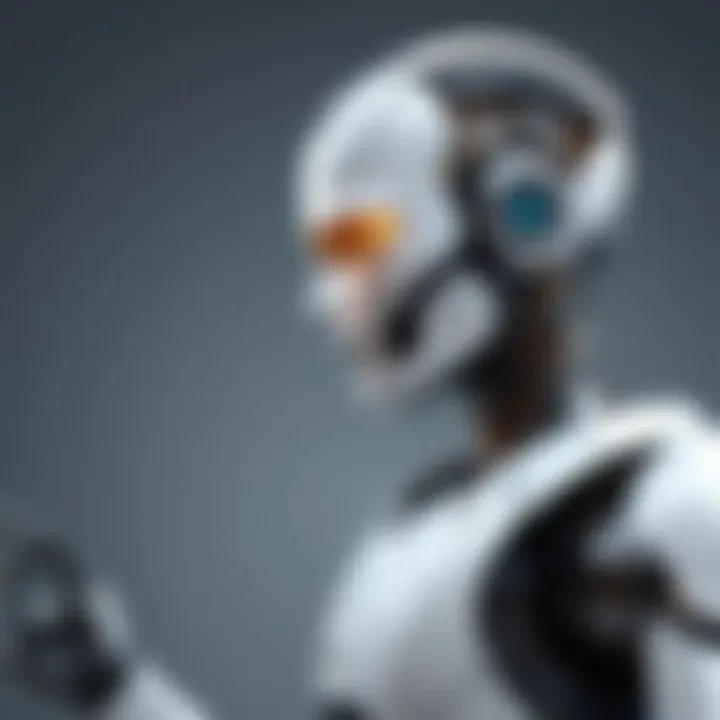
Intro
The rise of question-answering robots represents a significant shift in how we interact with technology. Gone are the days when sifting through endless manuals or troubleshooting guides was required for even the simplest of tasks. Instead, we are entering an era where intelligent systems provide immediate answers, breaking down barriers of time and accessibility. This article explores the capabilities of these robots, shedding light on their technology, applications, and the potential challenges they face.
By leveraging artificial intelligence and natural language processing, these robots aren't just solving trivia questions. They engage with users, analyze patterns, and even adapt to varied contexts. Imagine asking a robot about how to fix a leaky faucet, and rather than receiving a dry, technical response, it responds as if you're having a conversation with a knowledgeable friend.
The journey ahead requires us to understand both the technology underpinning these robots and their implications across different sectors. From customer service enhancement to assisting in medical diagnostics, the applications appear endless. Let's dive into the tech trend analysis to understand where we are now and how far we can go.
Prelims to Question-Answering Robots
The realm of technology is rapidly shifting towards automation and enhanced interactivity. Among the most intriguing advancements are question-answering robots. These systems are not just machines; they symbolize a bridge between human communication and computational efficiency. Their ability to provide information and assistance on a wide range of topics has significant implications across various industries, from healthcare to customer service.
Why Dive Into This Topic?
Understanding the capabilities of these robots equips tech enthusiasts, industry professionals, and the curious minds with the knowledge needed to navigate an increasingly automated world. As we explore their functionalities, the historical context, and their real-world applications, we unveil how these systems are reshaping our interactions with technology and each other.
Definition and Purpose
A question-answering robot is a specialized program designed to respond to inquiries posed by users, often using natural language processing. These systems analyze the questions raised and leverage vast data repositories to generate responses. They serve multiple purposes:
- Efficiency: By providing rapid answers, they reduce the wait time associated with traditional information-gathering methods.
- Accessibility: They can operate around the clock, offering users immediate assistance regardless of time zones.
- Personalization: With proper training, these robots can learn user preferences, making interactions smoother and more relevant.
In practice, whether it's a customer seeking support on a product or a student searching for educational resources, question-answering robots aim to deliver accurate, relevant information swiftly.
History and Evolution
The roots of question-answering robots extend back to early computational research that sought to mimic human intelligence. The journey began in the mid-20th century with foundational work in artificial intelligence and linguistics. For example:
- 1950s: The first attempts at computer-based language processing appeared, with systems like ELIZA simulating conversation by recognizing keywords in user input.
- 1970s-80s: Significant advancements in knowledge representation allowed for more sophisticated queries and responses. Projects like SHRDLU showcased the potential of understanding natural language in specific contexts.
- 2000s: The rise of vast databases and powerful AI models, most notably with developments such as Google Search and IBM’s Watson, ushered in a new era. These systems employed complex algorithms to sift through massive amounts of data, providing answers to increasingly elaborate questions.
Today, we stand on the shoulders of these foundational technologies, leveraging deep learning and advanced machine learning algorithms. This evolution reflects both a growing understanding of human language nuances and a continuing push towards making technology more intuitive and user-friendly.
Key Technologies Behind the Robots
The realm of question-answering robots intertwines seamlessly with various key technologies. These technologies not only empower robots but also shape their capabilities, allowing for more accurate, accessible, and contextually relevant responses. Understanding these technologies is crucial, as they form the backbone of performance in solving real-world problems across different applications.
Artificial Intelligence Frameworks
Machine Learning Algorithms
Machine learning algorithms are a fundamental building block within AI frameworks, acting as the engine that drives the learning process. These algorithms enable systems to learn from data inputs and adapt over time without human intervention. One of the key characteristics is their ability to recognize patterns and predict outcomes effectively. This makes them a popular choice in the development of question-answering robots, as they can sift through large datasets to provide precise answers based on previous learning.
A unique aspect of machine learning algorithms is their capacity to improve accuracy as more data becomes available. However, this dependency on data can also pose challenges, particularly when data is sparse or biased. If the training data isn't diverse, the algorithm can misinterpret or misunderstand user queries, leading to erroneous responses.
Deep Learning Resources
Deep learning takes machine learning a step further. It employs neural networks with multiple layers, allowing for advanced processing of data. One notable characteristic of deep learning is its ability to handle unstructured data, such as images and text, which is critical when computing complex inquiries made by users.
The unique feature of deep learning resources is their capability to learn hierarchical representations of data, meaning they can derive insights at various levels. However, deep learning models often require significant computational resources and large datasets, making them less feasible for smaller-scale applications. Still, their high performance in understanding complex queries keeps them relevant in powering question-answering robots.
Natural Language Processing Techniques
Text Parsing
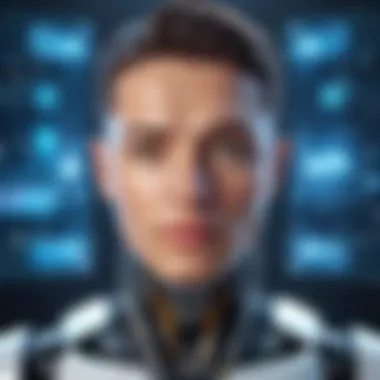
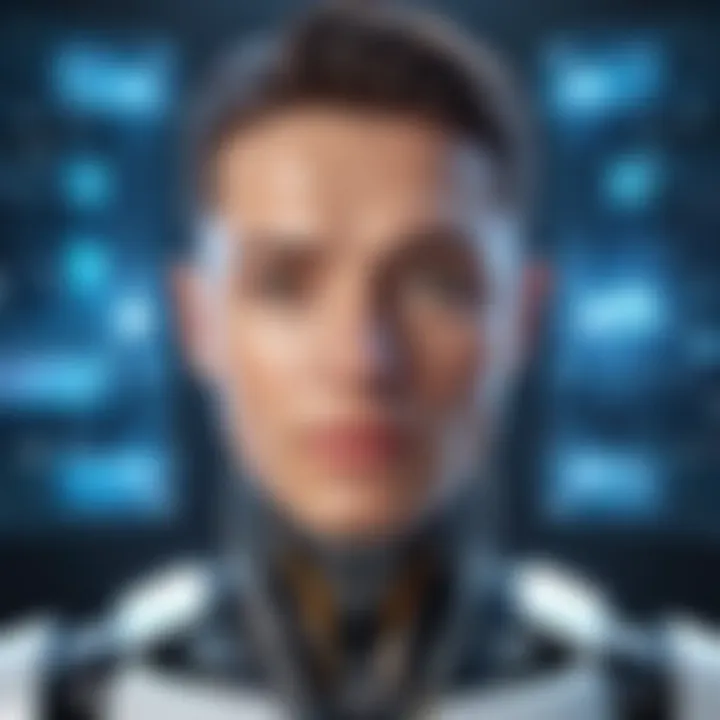
Text parsing is the method through which robots interpret the structure of a sentence, breaking it down into its components to understand meaning. This is especially important in answering questions since it allows robots to identify key parts of a query, such as the subject, verb, and object. One of its key characteristics is its efficiency in processing natural language inputs, enabling robust interaction between humans and machines.
The unique feature of text parsing lies in its ability to discern grammatical relationships within sentences. However, it can struggle with ambiguity or complex structures, leading to misinterpretation. Despite this drawback, it remains a valuable tool in the question-answering ecosystem as it lays the groundwork for a more thorough understanding of inquiries.
Sentiment Analysis
Sentiment analysis tool helps robots gauge the emotional tone behind a user's query. Understanding whether a user is frustrated, happy, or indifferent towards a topic can significantly enhance interaction quality. Its key characteristic is the ability to assess language nuance and context, which is necessary for crafting more empathetic responses.
The unique feature of sentiment analysis is how it combines linguistic rules and data-driven models to make predictions about user intent. However, it can encounter limitations with sarcasm or contextually rich phrases, potentially leading to misunderstandings. Yet, its importance in refining user experience is undeniable, guiding robots towards more human-like responses in discussions.
Popular Applications of Question-Answering Robots
The relevance of question-answering robots cannot be overstated in today’s rapidly advancing technological landscape. These systems are gaining traction across a spectrum of industries, fundamentally changing the way information is accessed and delivered. The implications stretch far beyond simple convenience; they serve as a bridge connecting users with the wealth of knowledge hidden in vast databases. As such, examining their applications is crucial to understanding their role in modern society.
Customer Support Systems
In customer service, the integration of question-answering robots has revolutionized traditional practices. Organizations like Amazon have implemented chatbots that handle a significant portion of customer inquiries. These systems operate around the clock, allowing companies to provide immediate assistance without the limitations of human resource availability. The efficiency of such robots means that users receive quick answers to their queries regarding product details, order tracking, and frequently asked questions.
The benefits are manifold:
- Cost Reduction: By minimizing the need for a large customer service team, companies can reallocate resources to other areas.
- Response Consistency: Robots deliver uniform answers, reducing the risks associated with human error or inconsistency in responses.
- Scalability: During high-demand periods, the ability to handle multiple inquiries simultaneously ensures customer needs are met without delays.
While there are notable advantages, the emotional nuance in customer interactions cannot be ignored. Human touch remains vital in situations involving complaints or complex issues, where empathy might be pivotal. Hence, a hybrid model combining human agents and question-answering robots is often observed to strike the right balance.
Educational Tools
In the realm of education, question-answering robots have unlocked new dimensions in learning methodologies. Tools like Google Assistant and Amazon's Alexa help students of all ages by answering queries on a myriad of topics, from historical facts to math problems. These robots serve not only as information providers but also as interactive aides that can facilitate learning in a more engaging way.
Specific advantages include:
- 24/7 Availability: Students can seek assistance at any time, which is especially helpful for those who study at unconventional hours.
- Personalization: These systems can learn user preferences, adapting the content and approach to better serve individual needs.
- Instant Feedback: Immediate responses enable a fluid learning process, allowing students to grasp concepts without delay.
However, challenges remain regarding the accuracy of the information provided and the potential to mislead. Students might inadvertently rely too heavily on the robot’s capabilities, leading to gaps in critical thinking or research skills. As such, educators are encouraged to integrate these tools into broader learning frameworks while emphasizing the importance of verification.
Healthcare Assistance
In healthcare, question-answering robots provide a plethora of opportunities to enhance patient care. Platforms such as IBM’s Watson are already being utilized in medical settings to assist physicians in diagnosing and treating patients. By analyzing vast datasets, these systems can recommend potential treatment plans based on the latest research and emerging practices.
The potential benefits of applying these technologies in healthcare environments are significant:
- Diagnostic Support: By harnessing historical patient data and clinical guidelines, robots can bolster decision-making processes for healthcare professionals.
- Patient Engagement: Chatbots can answer common patient queries, helping individuals better understand their symptoms or the next steps in their care journey.
- Efficiency Boost: Medical staff can focus on direct patient care rather than answering repetitive queries, which can lead to enhanced overall service efficiency.
Despite these advancements, ethical concerns surround the delivery of medical advice through robotic systems. Accuracy and the potential for misinterpretation must be thoroughly vetted. Regulatory frameworks are needed to ensure that such technologies are used responsibly, safeguarding patient welfare.
In summary, the applications of question-answering robots are highly diverse, spanning customer service, education, and healthcare. Each sector showcases the robots' capacity to enhance efficiency and accessibility. However, it is crucial to recognize the limitations and ethical considerations that accompany these technological advancements. As the capabilities of these robots continue to evolve, a cautious yet optimistic approach is necessary.
The Mechanics of Interaction
Understanding the mechanics of interaction is crucial in exploring how question-answering robots communicate and engage with users. This section delves into the user input and output generation processes which shape the user experience and ultimately define the effectiveness of these robots.
User Input and Processing
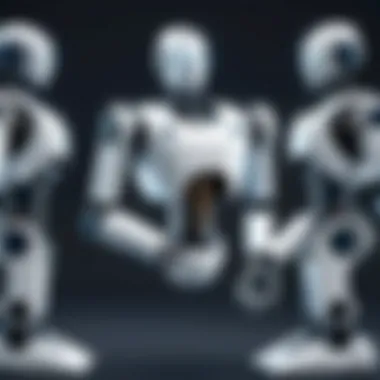
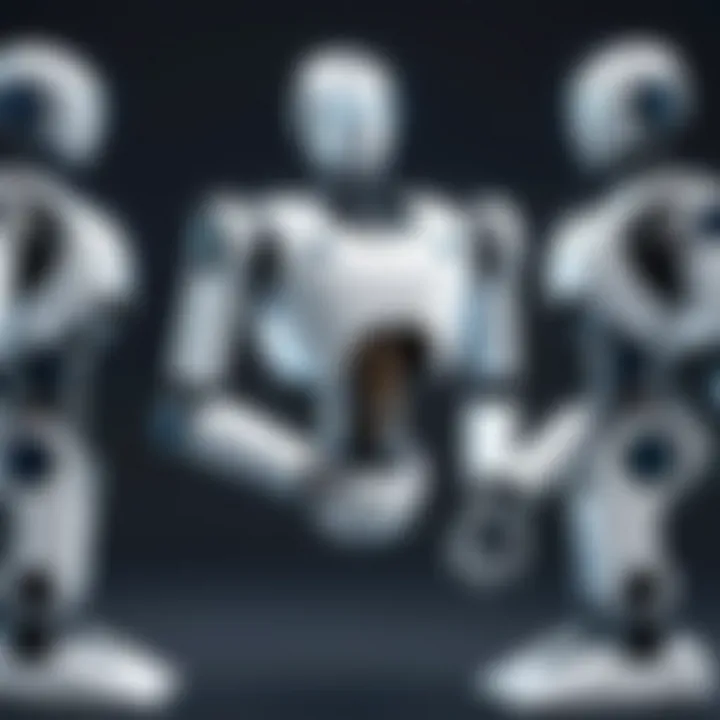
Speech Recognition
Speech recognition serves as a vital cog in the wheel of interaction within question-answering robots. This technology allows machines to convert spoken language into text, enabling users to engage in a more natural dialogue. One of the standout features of speech recognition is its ability to understand diverse accents and dialects, making it widely accessible and user-friendly. It caters to a broad audience, from tech-savvy individuals to those less familiar with technology.
Advantages of using speech recognition include quicker, hands-free interaction, and the ability to multitask while speaking to the robot. However, the limitations become apparent in noisy environments, where the clarity of speech can degrade, affecting accuracy. Also, it requires training to pinpoint specific user vocabulary or phrases which may be challenging in varied contexts.
Text Input Mechanisms
On the flip side, text input mechanisms facilitate a more traditional method of interaction. Users type their inquiries, which offers them better control over how they express their questions. The primary strength here lies in precision; it allows users to formulate phrases exactly as they intend. Text inputs are also less affected by environmental noise, providing consistent performance across different settings.
However, the major drawback is that typing can slow down the pace of conversation, contrast this with speech that often flows more freely. Also, not all users are agile typists, which may create a barrier to efficient interaction. Text input is more favorable in situations where recording or reference of exact queries is essential.
Output Generation
Textual Responses
Textual responses are integral to delivering information from a question-answering robot. They are straightforward and allow for detailed, nuanced answers, essential for maintaining user engagement and trust. One key characteristic that stands out is clarity; with written text, users have the opportunity to review replies at their own pace.
The unique feature of textual responses lies in their ability to integrate hyperlinks or references, making further reading a breeze. On the downside, cold text can sometimes lack the warmth and human-like interaction that many users appreciate in dialogue. As a result, textual responses risk being perceived as impersonal, particularly in customer service contexts where empathy is crucial.
Voice Synthesis
Voice synthesis is an exciting area as it provides a human-like touch to the interaction. This technology converts the textual responses back into spoken words, bridging the gap between text and speech. One significant advantage of voice synthesis is that it can convey tone and emotion, which enhances user engagement.
Nevertheless, the synthesis quality can vary widely. Some voices may sound robotic or artificial, creating a disconnect with the user. Additionally, certain accents or languages might not be supported adequately, limiting accessibility. Despite these challenges, voice synthesis remains a crucial part of creating a fluid conversational experience with question-answering robots.
"Effective communication hinges on how well robots can interact with humans. It’s not just about answering questions but building connections."
In summary, the mechanics of interaction in question-answering robots encompass a delicate balance between user input and output generation. Both speech recognition and text input mechanisms come with their strengths and weaknesses, as do textual responses and voice synthesis. By carefully observing these dynamics, we can better appreciate the intricate web of capabilities that define the interaction between humans and these advanced machines.
Challenges in Development
When it comes to the world of question-answering robots, one cannot skim over the challenges they face during development. These hurdles don't merely appear as speed bumps; they can resemble concrete barriers that disrupt the smooth flow of innovation. Addressing these challenges is not just beneficial but crucial for the effective functioning of these robots across various sectors.
Data Limitations
A significant bottleneck for question-answering systems is the limitations related to data availability and quality. Without a rich dataset, these systems resemble books lacking pages. The training data must be expansive and diverse to cover various topics and contexts. Often, the data used is either outdated or heavily biased.
To tackle this issue, developers often resort to techniques like data augmentation, where synthetic data is generated to fill gaps. By utilizing multimodal datasets, which include text, audio, and visual data, developers aim to bolster the robot's capabilities. However, ensuring that the augmented data accurately reflects real-world scenarios is an ongoing struggle. If a system's foundational data is flawed, the output will likely miss the mark.
Understanding Context and Nuance
The subtleties of human conversation are often the perplexing puzzle for question-answering robots. It's a realm where a simple word choice can shift the entire meaning of a sentence. For example, consider the difference between "Can you get that?" and "Could you get that for me?" Both phrases request assistance, yet the latter carries an implicit expectation of action, reflecting nuances that robots often fail to grasp.
True understanding requires contextual awareness, going beyond literal interpretations to grasp underlying messages. This entails integrating sophisticated techniques in Natural Language Processing (NLP), such as sentiment analysis and contextual embeddings. For instance, incorporating context around each interaction allows robots to tailor their responses, enhancing user experience. Yet, even with advanced algorithms, misunderstandings still occur, leading to frustration both for users and developers. An ongoing dialogue in the tech community pushes for better frameworks that capture these nuances.
Ethical Considerations
The ethical implications of question-answering robots can sometimes feel like a minefield. As these systems increasingly make decisions that can affect people's lives, the question of accountability looms large. Who is responsible if a robot provides erroneous or harmful information? Without clear guidelines, developers run the risk of creating systems that may perpetuate biases or lead to misinformation.
To address this, it is imperative to implement transparent algorithms and decision-making processes. Developers must consider ethical standards in AI, ensuring that the robots are designed with fairness and accountability in mind. Encouraging discussions among ethicists, developers, and users can help build a consensus on what responsible use looks like.
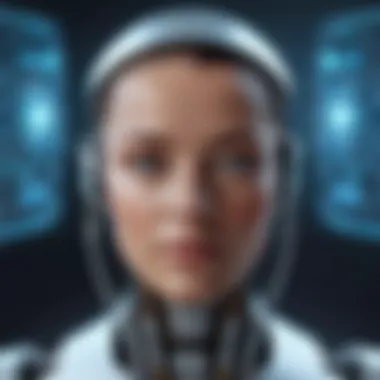
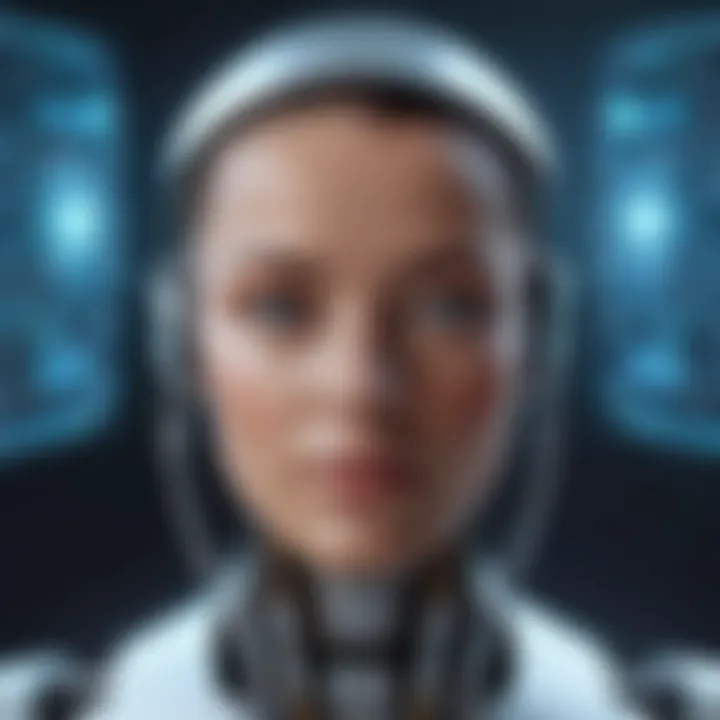
"The hope is that as we advance, ethical considerations become as fundamental to AI development as technological ones."
In essence, while these technological marvels hold promise, navigating the challenges of data limitations, context understanding, and ethical considerations is paramount to ensure they evolve into reliable and trustworthy assistants in daily life.
Future Prospects of Question-Answering Robots
The landscape of question-answering robots is constantly evolving, driven by ongoing advancements in technology and growing expectations from users. Understanding the future prospects of these robots is crucial, as it reflects not only the trajectory of artificial intelligence but also the potential shifts in various industries that can benefit from them. The implications are far-reaching, providing a glimpse into how we might interact with machines in our daily lives and how such technologies can enhance productivity and efficiency.
Advancements in AI Research
Artificial intelligence is not standing still; it's racing ahead at lightning speed. The investments in AI research are yielding unprecedented breakthroughs. One specific element to note is the development of more sophisticated neural networks that can learn from smaller data sets. By leveraging techniques like transfer learning, where knowledge gained in one area can enhance learning in another, question-answering systems are becoming more robust and flexible. These advancements allow robots to handle queries with greater context awareness and nuanced understanding.
Additionally, there are improvements in reinforcement learning, where AI learns optimal behaviors through trial and error. Imagine a robot evolving its responses based on feedback from users, leading to increasingly accurate and meaningful interactions over time. This shift signifies a move towards more personalized experiences, providing tailored answers rather than one-size-fits-all responses.
"Advancements in AI are not just changing machines; they are redefining how we communicate with technology."
Integration with IoT
The Internet of Things (IoT) is rapidly changing the way we perceive and interact with technology, and question-answering robots are set to become integral players in this ecosystem. With their ability to connect with various smart devices, these robots can harness data from multiple sources, enhancing decision-making processes. For example, a question-answering robot in a smart home doesn't just answer queries; it can control lights, adjust the thermostat, and manage security systems by integrating real-time data from everything around it.
The combination of IoT and question-answering robots opens doors to new possibilities in
- Smart homes, where robots help manage daily chores;
- Healthcare settings, providing real-time patient information;
- Manufacturing, optimizing workflows based on live data.
This interconnectedness not only improves efficiency but also elevates the users' overall experience, making technology feel more intuitive and accessible.
Potential for Personal Assistants
The rise of personal assistants powered by question-answering robots indicates a future where these systems might become our daily companions, assisting us in everything from professional tasks to personal management. The shift is already noticeable; AI systems are learning to interpret complex commands and context. This fine-tuning could transform how we handle routine responsibilities like scheduling appointments or making travel arrangements.
Benefits of integrating question-answering robots as personal assistants include:
- Time efficiency: Automating mundane tasks frees up valuable time for users;
- Customization: Advanced learning allows assistants to adapt to user preferences over time;
- Accessibility: Making technology more user-friendly and approachable for diverse demographics.
The potential is not just limited to a few sectors—imagine a world where every individual has a personal assistant that understands their unique needs. By evolving beyond simple question-and-answer routines, these robots could lead to significant shifts in how we interact with technology, redefining productivity and personal engagement.
As we move into the future, the synergy between AI advancements, IoT integration, and personal assistants will be pivotal in shaping the capabilities of question-answering robots, pushing boundaries, and opening up new frontiers in technology.
Epilogue
The significance of this conclusion in the context of question-answering robots cannot be overstated. As we have seen throughout the sections of this article, these systems are at the intersection of advanced technology and practical application, carving out an important niche in modern society. Their role extends beyond mere functionality; they offer conveniences that align with the fast-paced world we inhabit. The understanding we gain from the various aspects discussed here, such as key technologies and challenges, equips us with vital insights into the evolution of these robots.
Summary of Findings
Our exploration has illuminated several key findings regarding question-answering robots:
- Technological Backbone: AI frameworks and natural language processing techniques propel these systems, making them capable of understanding and responding to human queries.
- Diverse Applications: From customer service to healthcare and education, the scope of their application is broadening, impacting countless lives positively.
- Challenges Ahead: Data limitations and the nuances of human speech present ongoing challenges that developers must navigate in their quest to enhance these robots.
- Future Integration: With the rise of IoT, their future seems integrated into smarter environments, highlighting the potential for improved personal assistants and automation.
This synthesis of findings paints a picture of a field poised for significant advancements as technology continues to evolve.
Call for Future Research
Looking forward, the call for future research in the domain of question-answering robots is clear. Enhanced exploration is necessary in several areas:
- Improving Contextual Understanding: Future work must focus on better capturing the context and subtleties of language to improve accuracy.
- Ethical Guidelines: As these robots become more prevalent, establishing robust ethical frameworks is essential to address privacy and misuse concerns.
- User Experience Design: Research on how humans interact with these robots can lead to more intuitive interfaces that cater to a wider audience.
As these robots permeate various facets of life, a thoughtful approach to research will ensure their continued success and relevance in society. The blending of technology with everyday human activity reveals not just the potential benefits but also necessitates careful consideration of future challenges.