Essential Qualifications for Aspiring Data Analysts
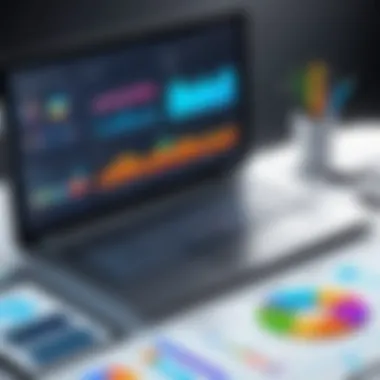
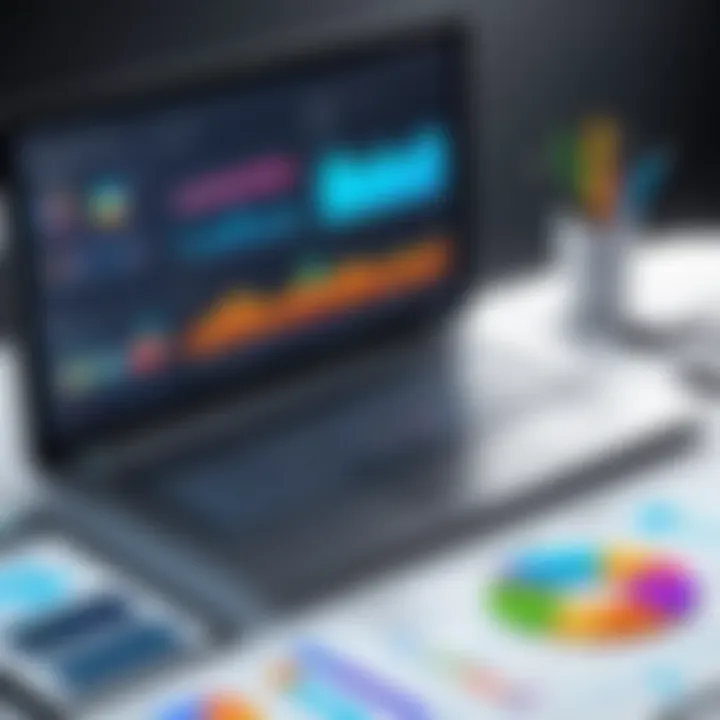
Intro
In today's world, data is invaluable. Organizations generate, collect, and analyze vast amounts of data. Because of this, the demand for data analysts is on the rise. A career in data analytics is appealing for those who enjoy working with information and deriving insights. To embark on this professional journey, understanding the qualifications needed is essential. This includes education, technical skills, and soft skills.
Data analysts play a critical role in helping companies make informed decisions. They merge statistical knowledge with analytical techniques to interpret complex datasets. Also, relevant certifications can significantly enhance a candidate's appeal.
This article examines the qualifications required to excel as a data analyst, highlighting crucial components that equip individuals for success.
Tech Trend Analysis
Overview of the current trend
Data analyst jobs are increasingly important in a fast-evolving environment. With more companies adopting data-driven strategies, the demand for skilled analysts rises. Organizations focus on leveraging data analytics to improve efficiency, optimize processes, and enhance decision-making.
Implications for consumers
As businesses adapt to technological changes, consumers benefit from improved services. A long-term trend is increased personalization in marketing, often due to data analysis. Organizations understand customer preferences better, enhancing user experience and satisfaction.
Future predictions and possibilities
Looking ahead, the role of data analysts will only expand. Advanced technologies, like artificial intelligence and machine learning, are becoming integral in data analytics. Given the growth trend, analysts may need to adapt and broaden their skill sets continually to stay competitive.
Core Qualifications
Understanding what qualifications are required to become a data analyst is crucial. This section explains educational requirements, key technical skills, and the essential soft skills.
Educational Background
A bachelorâs degree is often the minimum requirement. Degrees in fields such as mathematics, statistics, computer science, or economics are beneficial. However, some professionals may pursue advanced degrees to deepen their analytical capabilities.
Technical Skills
The following skills are critical for a data analyst:
- Statistical analysis: Familiarity with statistical concepts is essential to interpret data accurately.
- Data visualization: Tools like Tableau or Power BI help present data effectively.
- Programming languages: Knowledge of SQL, Python, or R allows analysts to manipulate and analyze data.
- Data cleaning: The ability to clean and organize raw data is fundamental to ensure accurate results.
Soft Skills
In addition to technical skills, soft skills are equally significant. Skills like critical thinking, attention to detail, and effective communication enable analysts to convey complex findings clearly. Collaboration is also vital, as analysts frequently work with stakeholders from various departments.
Certifications
Earning certifications can enhance credibility and demonstrate expertise. Acquiring credentials such as the Certified Analytics Professional (CAP) or Google Data Analytics Certificate helps confirm a candidate's qualifications. These specialized programs often cover essential topics and software used in the field.
End
A career in data analytics requires a blend of education, technical skills, and soft skills. As organizations continue to rely on data for strategic decisions, the demand for well-trained data analysts is likely to grow. Transitioning to this field can provide numerous career opportunities for those who meet the necessary qualifications.
Prelims to Data Analytics
Data analytics has grown into an essential domain that helps organizations in various industries make informed decisions. Understanding the core principles of this field lays a strong foundation for anyone aspiring to become a data analyst. This section serves as an introductory guide that uncovers what data analytics incorporates and how critical it is in shaping business direction.
Understanding the Role of a Data Analyst
The role of a data analyst is unique and multifaceted. A data analyst sifts through large sets of data to find trends, patterns, or insights that can drive decision-making across an organization. Their responsibilities can vary based on the industry and the specific business needs, but typically involve collecting data, processing and cleaning it, performing statistical analysis, and presenting findings in a comprehensible format.
Key aspects of a data analyst's role include:
- Collecting Data: Gathering raw data from various sources, such as internal databases, market research, or open data repositories, ensures that the analysis rests on a solid ground.
- Data Processing: Handling vast amounts of data necessitates strong organizational skills, as analysts must ensure that the data is accurate and well-structured, commonly using tools like Microsoft Excel or programming languages such as Python and R.
- Statistical Analysis: Proficiency in statistical methods allows analysts to interpret data correctly, revealing patterns that are not immediately obvious.
- Data Presentation: Analysts must convey their findings effectively to non-technical stakeholders. This includes utilizing visualization tools like Tableau or Power BI, as well as crafting detailed reports.
The role plays a critical part in business strategy. From marketing to finance, having a clear understanding of this function enhances clarity around what organizations can achieve with their data resources, making it indispensable.
Importance of Data in Decision-Making
In today's data-centric world, organizations find themselves swimming in an ocean of information. Every decision, whether strategic or operational, can benefit from meticulous data analysis. The decisions guided by accurate data not only increase efficacy but often minimize risks as well.
Listed below are some methodical advantages offered by data-informed decision-making:
- Informed Choices: Uncovering what lies within the numbers helps companies avoid guesses, providing sound bases for strategic marketing direction, operations optimizations, and product development.
- Competitive Advantage: Employing analytics can highlight trends ahead of the competition, giving organizations a unique position in their respective market sectors.
- Efficiency and Resource Allocation: Analytics helps stakeholders streamline processes and prioritize resource allocation based on insight drawn from the data.
In essence, proper utilization of data allows organizations to pivot and adapt to changing markets and trends, solidifying this skillâs key role in ongoing business success. As data continues to grow in importance, so does the need for qualified professionals capable of embracing its potential.
Educational Background
Educational background forms the bedrock of qualifications necessary for effective performance as a data analyst. This sector thrives on analytical techniques and reasoning supported by rigorous academic training. The educational achievements accumulated help professionals navigate complex datasets, extract achievable insights, and propose informed solutions tailored to organizational needs.
A well-rounded educational background encompasses not only opportunities to acquire essential technical skills but also skills that enable analysts to contextualize their findings in the bigger picture. This combination amplifies the ability to innovate and respond to rapidly evolving technology trends.
Data analysts often find themselves faced with an array of situations requiring understanding not only of data but also of the intricacies of business strategies and market dynamics. Proper education thus furnishes them with critical frameworks to approach problem-solving astutely.
Relevant Degree Programs
Various academic fields cultivate the vital knowledge and skills required in data analytics. Each of these fields contributes uniquely to an analystâs competence.
Computer Science
Computer Science emerges as a fundamental pillar within the educational background of many data analysts. This institute's systematic approach trains analysts in programming concepts and data structures, facilitating efficient processing methodologies. A key characteristic is not only proficiency in programming languages but also an understanding of algorithms, machine learning, and database management.
One beneficial aspect is the emphasis on technical foundations that position future analysts for proficiency in handling languages such as Python or SQL. However, while it equips analysts well technically, it may lack emphasis on practical business application compared to some business-focused degrees.
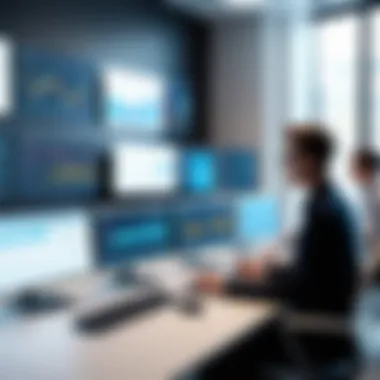
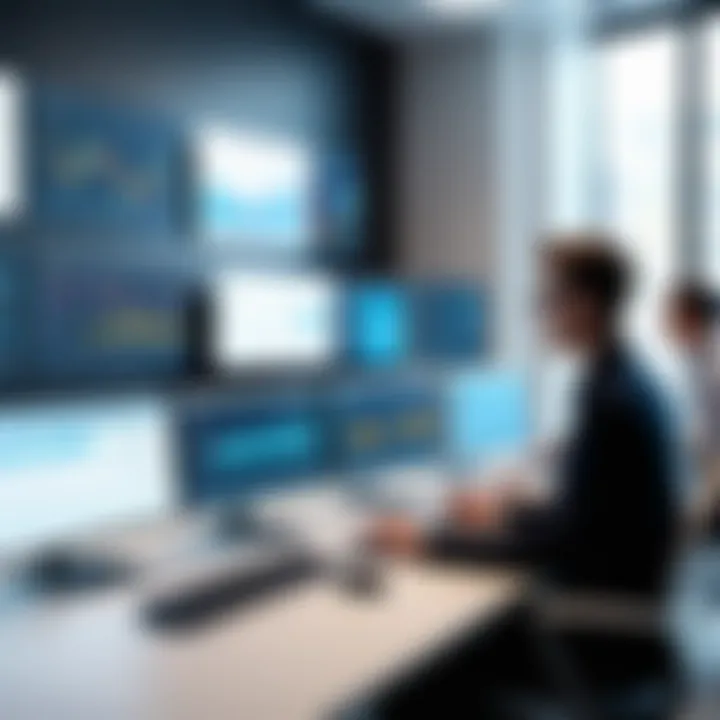
Statistics
Statistics plays a pivotal role in establishing qualitative and quantitative analytical skills among data analysts. It teaches how to interpret data effectively and comprehend variability amidst data sets. An important feature is the focus on hypothesis testing, analysis, and meaningful interpretation of data trends which are essential for decision-making.
Statistics is widely regarded as an advantageous choice since it transforms raw data into actionable insights. Data analysts with a statistics background typically excel at identifying significance in data; nonetheless, they might occasionally struggle with implementation in business frameworks directly due to a limited conceptual understanding of overall business objectives.
Mathematics
Mathematics reinforces the capability to work alongside analytical formulas and methodologies extensively utilized in data analytics. This program emphasizes logical reasoning and complex problem-solving. A key characteristic of such a program is its ability to enhance critical thinking skills and logical deduction, vital for data analysis processes.
Mathematics stands out as a beneficial choice due to its rigorous training in modeling, which translates well to predictive analytics. On the flip side, this foundation lacks direct application of analysis to real-world problems without supplementary business or context knowledge.
Business Administration
When looking at degrees in Business Administration, there exists an immediate grounding in the principles of economics and organizational behavior. This understanding culminates in a comprehensive overview of market dynamics, complementing the data analyst's work. An important facet of this degree is the blend of qualitative and quantitative learning, making analysts versatile.
Having familiarity with business functions enables analysts to present their findings in relatable terminology for stakeholders. While more access-focused towards practical applications, it may not cover the technicalities and intricacies of programming to the full extent.
Importance of a Strong Foundation
Having a robust educational background is essential for developing proficiency in data analysis. It sets the stage for lifelong learning and adaptation. Organizations often seek analysts who demonstrate both educational depth and practical knowledge.
In a field constantly evolving due to technological advancements, strong foundational knowledge is critical. It allows individuals to pivot and acquire new skills efficiently. Providing context through proper training helps professionals remain relevant and valuable in an increasingly competitive job market. Overall, investment in education lays the groundwork for a successful career as a data analyst.
Technical Skills Required
In the realm of data analytics, technical skills are vital for success. These skills enable data analysts to transform raw data into meaningful insights that guide business strategies. With the exponential growth of data, being skilled in the right tools and languages allows analysts to communicate effectively and discover patterns that are not readily apparent.
Proficiency in Programming Languages
Programming languages are the foundation for any data analyst. A strong command in recognized languages allows for efficient data manipulation and analysis. Among these, Python, R, and SQL stand out for their unique features and applicability in data analytics.
Python
Python is often heralded for its versatility and readability. Its expansive library ecosystem, such as Pandas and NumPy, strongly supports data analysis tasks. Python's simple syntax makes it accessible, encouraging a wide range of users from various backgrounds to adopt it. Moreover, Pythonâs suitability for both analysis and web applications adds to its appeal.
Python allows data analysts to:
- Perform complex calculations efficiently
- Automate repetitive data tasks
- Carry out machine learning applications
However, Python can sometimes have higher memory consumption, which might be a constraint for large data sets.
R
R is another prominent programming language specializing in statistical analysis. It shines in extensive data testing and visualization tasks. Râs rich set of packages makes it extremely effective for advanced statistical techniques, providing a broad array of tools directly relevant to data analysis.
Some reasons R is recommended include:
- Advanced statistical functions that are prebuilt
- High-quality data visualization libraries like ggplot2
- A strong community support
Despite its advantages, R might present a steeper learning curve for those unfamiliar with programming.
SQL
SQL, or Structured Query Language, is the backbone of database management. It plays a key role in data extraction, organization, and manipulation within relational databases. SQL is essential for querying data, allowing data analysts to access required information effectively.
Key characteristics of SQL include:
- Powerful querying capabilities
- Ability to handle large databases with ease
- Standardized language, recognized across multiple database systems
While SQL is highly advantageous, it mainly focuses on data retrieval, which limits its functionalities compared to larger programming languages like Python.
Data Visualization Tools
In addition to programming skills, visual representation of data is critical. Data visualization tools allow analysts to present findings in a manner understandable to all stakeholders, fostering informed decision-making. Recommended visualization tools like Tableau, Power BI, and Matplotlib are essential to a data analyst's toolkit.
Tableau
Tableau is renowned for its data visualization capabilities. Its user-friendly interface allows for swift creation of situations where visuals represent data in interactive dashboards. Moreover, Tableau's ability to connect with sensory inputs adds to its power.
Advantages of using Tableau include:
- Ease of integration with various data sources
- Robust analytic features
- Effective storytelling through visuals
However, users may observe a license fee that can deter smaller businesses.
Power BI
Power BI offers strong data visualization combined with excellent organizational features. It supports seamless connection with Microsoft products, which is advantageous for companies integrated into the MS ecosystem. The flexibility of Power BI allows for easy report sharing among team members.
Distinctive features include:
- Cost-effectiveness for organizations of all sizes
- Comprehensive environmental integrations
- Dashboard sharing capability within organizations
On the downside, beginners may find the interface challenging to navigate initially.
Matplotlib
Matplotlib needs no introduction among Python users. It is a well-known plotting library that facilitates visibility of data trends. Analysts often rely on it for ease of use and customization.
Strengths of Matplotlib comprise:
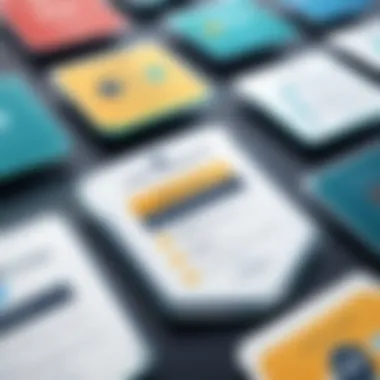
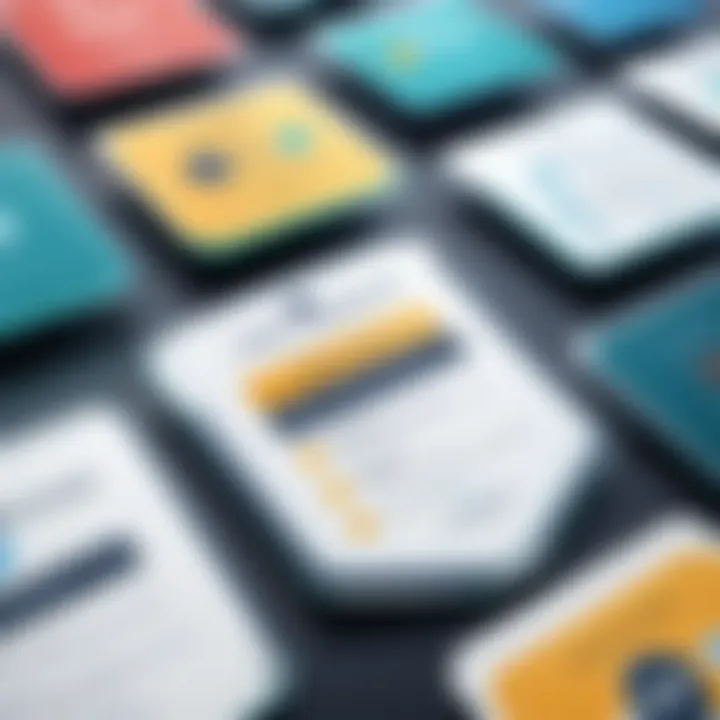
- Highly customizable visuals
- Work seamlessly together with other plotting libraries
- Suitable for academic use
Yet, when managing complex visualization, it can become cumbersome due to its low-level functionalities, sometimes demanding more input than other tools.
Advanced Statistical Analysis
Statistical analysis will enable better decision-making for any analyst. Knowing methodologies, including probability distributions and inferential statistics, enhances analytical insights. A firm grasp on choosing appropriate statistical tests is crucial for accurate results. For data analysts, proficiency here allows not just understanding of data, but the power to present logically sound conclusions that benefit business operations.
Data analysts must be skilled in both practical application of statistical tests and theoretical principles guiding them. This mix assists in yielding predictions and tailored solutions enhancing quality methodologies within organizations.
Soft Skills Essential for Data Analysts
Soft skills play a crucial role in a data analyst's success. While technical skills are undoubtedly important, soft skills complement these abilities by enhancing how analysts interact with data and collaborate with colleagues. The fusion of these skills can lead to significant benefits for the analyst and their organization. Without a keen understanding of soft skills, even the most technically proficient analyst might struggle in team settings or fail to convey their findings effectively.
Critical Thinking and Problem Solving
Critical thinking is the backbone of a data analystâs role. It involves the analysis of facts to form a judgment. In data analytics, various scenarios require not just processing of data, but the ability to make sense of it amid conflicting information. Analysts must evaluate data sets, recognize patterns, and formulate relevant questions. They should dissect the issues at hand, prioritize queries effectively, and present actionable insights. Problem solving requires a voyage through data. Fast analysis unquestionably becomes essential in identifying and mitigating potential issues.
Communication Skills
Communication skills serve a dual purpose: they ensure the data insights generated are understood and acted upon. Analysts need the ability to convert complex statistical findings into understandable concepts for non-technical stakeholders.
Presenting Data Insights
Presenting data insights effectively is a critical capability. It allows an analyst to share vital information concisely. Clear presentations help in demystifying data-driven concepts. The key characteristic here is clarity. In contrast to technical jargon, the focus should remain on simplicity for diverse audiences. Numerous data visualizations, like charts or graphs, can serve this purpose of simplifying complex analyses. However, an advantage might be its dependent nature on the analystsâ ability to create accessible narratives.
Collaborating with Teams
The skill of collaborating in teams enhances the quality of the analytic process. Analysts often work cross-functionally with various departments such as marketing, finance, or engineering. In this space, cooperation becomes indispensable. A strong characteristic is the development of rapport. Effective collaboration promotes shared understanding which, in turn, enriches project outcomes. Specifically, interdisciplinary relations allow data experts to gather diverse perspectives, producing more thorough analyses, which sometimes opens newer avenues.
Attention to Detail
Certifications That Enhance Qualifications
Certifications play a crucial role in establishing a data analyst's credibility. In an industry where skills and knowledge must continually evolve, obtaining relevant certifications demonstrates commitment and competency. Employers often view certifications as a significant asset when evaluating candidates. This section will explore relevant certifications, their features, and the benefits they bring to aspiring data analysts.
Overview of Relevant Certifications
Certified Analytics Professional (CAP)
The Certified Analytics Professional (CAP) is a recognized credential that underscores one's expertise in analytics. It validates a professional's ability to transform data into actionable insights. This certification is particularly appealing due to its broad acceptance in various industries and emphasizes practical analytical skills.
Another national characteristic of CAP is the focus on the analytics process from business understanding to data preparation to model development. This prepares candidates for real-world challenges. However, obtaining this certification requires a bachelorâs degree and several years of working experience, which may be a barrier for some.
Microsoft Certified: Data Analyst Associate
The Microsoft Certified: Data Analyst Associate certification demonstrates proficiency in using Microsoft Power BI for data analysis and visualization. This credential supports facilitating data-driven decision-making via interactive dashboards and reports. It suits individuals who often work with large datasets and need to present insights effectively in business environments.
A key characteristic of this certification is its focus on using Microsoft tools to maximize efficiency and skills in data modeling. However, its specificity to Power BI might limit its applicability if familiarization with other tools is needed for different jobs.
Google Data Analytics Professional Certificate
The Google Data Analytics Professional Certificate prepared individuals from diverse backgrounds to work in data analytics. Its content is user-friendly and designed for beginners. This certification is appreciated for its structured learning path, which includes hands-on projects and real-life case studies â essential proof to future employers.
One unique feature of this certification is its accessibility through Googleâs online learning platform, making it widely available. Yet, some might argue that the general nature may not match higher-level analytical roles.
Benefits of Obtaining Certifications
Obtaining certifications offers many benefits, including:
- Enhanced Career Opportunities: Certifications can significantly broaden job prospects, making candidates more attractive to employers.
- Skills Validation: They demonstrate specific capabilities, notably in technical and analytical fields.
- Networking Opportunities: Many organizations hosting certification programs also provide platforms for like-minded professionals to connect.
- Up-to-Date Knowledge: The pursuit of certifications promotes continuous learning, keeping professionals updated with the latest tools and techniques in data analytics.
Building a Portfolio
Creating a strong portfolio is a fundamental aspect of establishing oneself as a competent data analyst. A well-organized and insightful portfolio showcases one's ability to apply data analytics principles to real-world scenarios. This reflects not just technical skills but also practical understanding, which is paramount in this field.
The importance of having a portfolio in the data analytics domain cannot be overstated. Unlike traditional job applications where credentials might speak for oneself, a portfolio provides tangible proof of a candidate's capabilities. Employers often prefer to see examples of hands-on work, as it represents the applicant's experience in dealing with complex datasets and deriving actionable insights from them.
One of the specific benefits of building a portfolio is that it allows an analyst to illustrate unique problem-solving abilities. Moreover, candidates can select projects that align with their personal interests, thus making their applications more compelling.
Importance of Practical Experience
In data analysis, practical experience holds significant weight. Working on real projects helps to consolidate theoretical knowledge and translate it into executable skills. Often, formal education can impart critical concepts, but real-world experience reveals practical applications.
Having hands-on experience allows data analysts to navigate challenges effectively and strategize solutions. Employers seek professionals who do not just possess academic qualifications but also know how to implement those in practical scenarios. Furthermore, experience highlights an analyst's adaptability and creativity in leveraging data to inform decisions.
Through internships, freelance work, or personal projects, analysts can gain practical experience essential in building a competitive edge in the job market.
Showcasing Projects and Skills
A data analyst's portfolio must effectively showcase relevant projects and skills. It's advisable to select a diverse range of works to demonstrate versatility across different users cases. Each project should ideally present problems faced, and solutions provided, along with methodologies utilized.
Include details such as:
- Project Objective: A clear statement of what was aimed to achieve.
- Tools Used: Highlight any technical tools or software employed in the process, such as Python, R, or Tableau.
- Outcomes Realized: Discuss what insights were gained and how they contributed to business needs.
Such detailing demonstrates not only proficiency with analytic tools but also an analyst's capacity for critical thinking and presenting data conclusions compellingly. Building on all completed works, it illustrates an individual's personal brand and unique style.
Presentation matters. A neat and professional presentation of your work can enhance perception greatly.
Additionally, consider including a brief write-up with each project. This should convey the insights found, methods taken, and lessons learned during the process. Doing so adds depth and context, allowing potential employers to understand the value of your work. Highlighting various skills projectsâlike data cleaning, visualization, and advanced analyticsâbuilds a robust profile that can resonate strongly with recruiters and hiring managers.

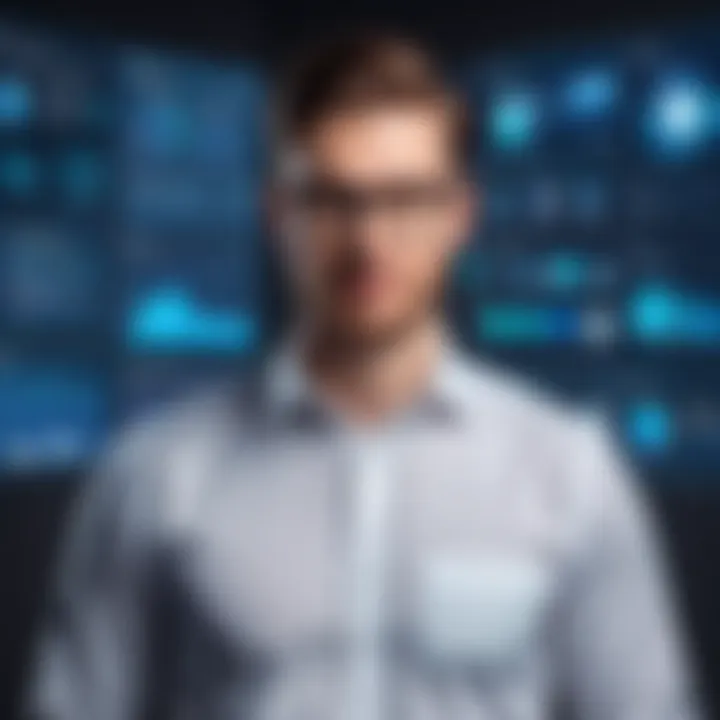
Networking and Professional Development
Networking and professional development play a crucial role in the career trajectory of a data analyst. The field is characterized by rapid advancement in technology and information. Mo'st job opportunities come as a result of understanding the collaboractive ecosystem in which data analytics operates. Establishing connections with industry leaders, peers, and mentors helps bolter professional knowledge and skills.
Effective networking can unlock employement opportunities that are not always publicly advertised. When connections are avaiable, analysts can learn about these roles before they beocome broadly known.
Additionally, networking allows for the exchange of ideas and best practices. Attending industry events, joinin online forums, and engaging with communities such as those on platforms like Reddit can enhance your understanding of the latest tools, techniques, and trends in data analysis. Participating in conversations where similar-in-interest professionals share experience can solidfi relationships that may later prove advantageous in oneâs career journey.
Connecting with Industry Professionals
Reaching out to industry professionals can profoundly influence both personal growth and career progression. One way to do this is by participating in networking events and conferences. Such occasions bring together an array of specialists, creating a fertile ground for meaningful interactions. Not only can you gain valuable insights, but you can also introduce your proyecto or share your goals. A simple conversation could lead to mentorship or even collaboration.
Utilizing platforms like LinkedIn is essential. There you can connect with colleagues and other professionals. Sharing relevant articles or commenting on posts related to data science can bolster your visibility. As your network grows, so does your exposure to job leads and knowledge.
âNetworking is not just about what you can get, but also what you can give to those around you.â
You may also consider attending local meet-ups or joining specialized groups online. Each exposure can yield new perspectives relevant to specific problems or challenges you face. Connecting with industry experts ranges from meaningful impact to providing immense help for career beginners.
Staying Updated with Trends
In the data analytics landscape, staying current with the continuous evolution trends cannot be discounted. Data is formulated to be an esclusive domain that enters various industries, shaping how decisions are made. Accordingly, analysts should prioritize keeping informed about changes in methodologies, software tools, or industry standards that influence their work.
Feed through websites like Wikipedia can provide a general overview of new technologies that surface in the market. Furthermore, industry publications and journals provide peer-reviewed knowledge, which ensures the sustaintability of your skills and knowledge accrue over time.
Engaging with newsletters, podcasts, or enrolling in online courses ensures your skills remain sharp. Platforms such as Coursera provide vast opportunities to assimilate new information.
- Regularly motoring these resources optimizes your ability to harnass key skills relevant for your role.
- Participate in online webinars where you can engage directly with speakers and experts in the field to fortify your understanding.
Maintaining this tradition of lifelong learning ultimately caters to both your competence and your considerium as a valued employable candidate. As technology constantly metamorphosing, staying updated positions you distinctly among your peers.
Career Prospects and Opportunities
Understanding the career prospects and opportunities for data analysts is crucial for those entering the field. Data analytics has become increasingly vital across industries. Organizations harness data to inform decisions, substantiate strategies, and foster innovations. This trend underscores the importance of skilled data analysts. The demand for such professionals continues to rise, indicating solid job security and growth potential.
In this segment, we will explore the job market overview and various career paths available in data analytics. This knowledge will help aspiring data analysts identify suitable roles and decide on requisite qualifications.
Job Market Overview for Data Analysts
The job market for data analysts is expansive. Companies are realizing the significance of data-driven decisions, which has led to an increased demand for analysts. Reports indicate that the number of positions is expected to grow steadily over the next several years. These numbers suggest favorable conditions for job seekers in this domain.
Key points about the job market include:
- Diverse Industry Needs: Analysts are needed in sectors such as technology, finance, healthcare, and retail.
- Increasing Entry-Level Positions: More entry-level roles are opening up, providing a vital gateway into the field.
- Evolution of Job Descriptions: The responsibilities of data analysts are continually advancing, with roles merging with data science and engineering aspects.
Potential Career Paths
Choosing the right career path is essential for a successful future. Data analysts have various options available. Each option has distinct characteristics and merits. The most notable career paths include:
Business Intelligence Analyst
A Business Intelligence (BI) Analyst focuses on gathering and leveraging data to help organizations improve operations and make better strategic choices. The core task involves utilizing tools and technologies to turn data into actionable insights. Key aspects of becoming a BI Analyst include:
- Data Warehousing Techniques: Understanding tools for collecting and storing data is vital.
- Familiarity with BI Software: Proficiency in software like Tableau or Microsoft Power BI is beneficial.
Because of demand across various businesses, pursuing a career as a BI Analyst is a popular choice. However, its efficacy largely depends on an analyst's ability to communicate clearly with other departments.
Data Scientist
Data Scientists delve deeper than traditional data analysts, employing complex algorithms and machine learning techniques to predict trends and develop models. They often discover patterns hidden within large data pools.
Noteworthy points related to data science include:
- Strong Statistical Knowledge: A solid foundation in statistical methodologies is indispensable.
- Predictive Analysis Skills: This role requires advanced analytical skills to build predictive models effectively.
While a career in data science can lead to higher remuneration and job recognition, it often entails more extensive academic training. Thus, educators usually recommend such a path for individuals with a strong quantitative background.
Data Engineer
Data Engineers are responsible for designing and maintaining data infrastructures. Unlike data analysts, their workload often includes preparing data for analysis rather than interpreting it. Their work is foundational to the success of analytics, as it stabilizes the data requirements.
Essential features of a Data Engineer entail:
- Expertise in Data Pipeline Technologies: Knowledge of technologies like Hadoop and Spark is crucial.
- Database Management Proficiency: Handling databases such as SQL or NoSQL databases is fundamental.
Choosing a role as a Data Engineer is a beneficial choice for tech-minded individuals who enjoy programming and technical challenges, although it may limit direct engagement with business processes.
Data analytics offers a landscape rich with opportunity. Understanding various career paths allows aspiring professionals to align their skills and interests effectively.
Culmination
In this article, we have explored the essential qualifications necessary for becoming a data analyst. The field of data analytics is quickly evolving, with insights becoming pivotal to strategic decisions across various sectors. Thus, it is critical for emerging data analysts to recognize and cultivate the engaging skills, education, and relevant certifications discussed.
Summarizing the Path to Becoming a Data Analyst
To summarize, the pathway to becoming a proficient data analyst begins with a strong educational foundation, preferably in relevant degree programs such as Computer Science or Mathematics. This education should be paired with technical skills in programming and analytical tools like Python, SQL, and Tableau. Beyond technical know-how, possessing soft skillsâlike critical thinking and the ability to effectively communicate insightsâwill significantly enhance oneâs professional efficacy. Acquiring relevant certifications can further cement expertise and present a competitive edge in the job market.
Engagement in project work to build a solid portfolio can also benefit those preparing for a career in this discipline. Networking with established professionals in the industry allows for better insights into trends and increases learning opportunities while impossible trends raise the importance of continuous self-development.
Overall, becoming a data analyst involves a blend of education, certifications, ongoing learning, and demonstrated technical and soft skills.
Encouragement for Aspiring Data Analysts
For those aspiring to join this dynamic field, it's important to stay motivated. Understand that the journey might be challenging. Yet, the skills and knowledge you acquire will be important assets. With the right passion and dedication, anyone can grow into a refined data analyst.
Consider seeking internships or mentorships, as these experiences provide practical understanding. Remember that failure can come along the way, but it often serves as an opportunity for growth and is part of any professional journey.
Embrace this path, allow your passion to drive you forward. The world of data awaits those willing to explore its depths.