Unraveling the Profound Capabilities of a Data Scientist in the Digital Age
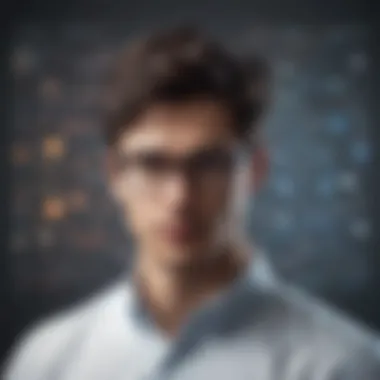
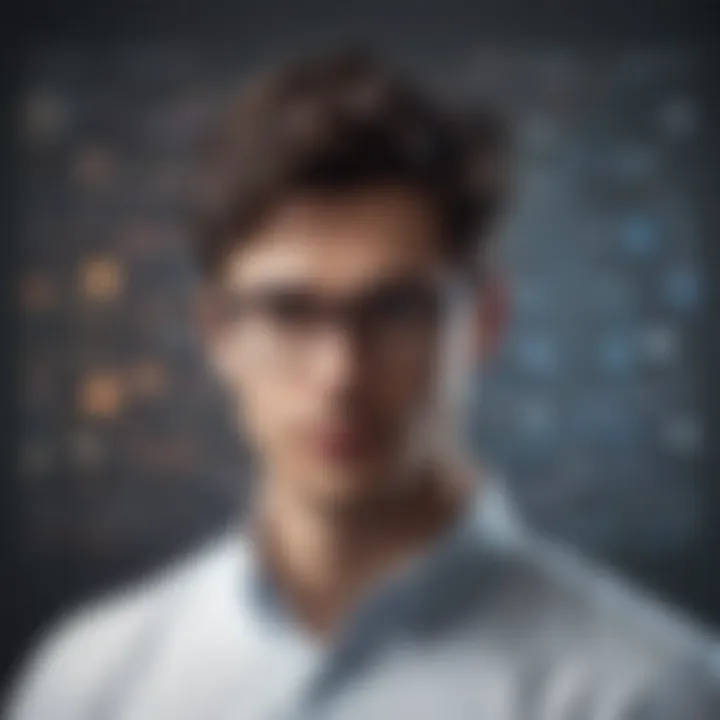
Tech Trend Analysis
Product Reviews
Exploring the Essence of a Data Scientist involves analyzing the tools and technologies that empower these modern-day wizards. Reviewing key products in the data science realm can shed light on their features, specifications, and performance metrics. By conducting a thorough analysis of the pros and cons of popular data science products, we can offer informed recommendations to professionals seeking to enhance their analytical capabilities. Recommendations based on in-depth product reviews can aid data scientists in optimizing their workflow and decision-making processes.
How-To Guides
Embarking on the journey of unraveling the Essence of a Data Scientist requires a comprehensive guide that illuminates the core aspects of data science expertise. Introducing the fundamentals of data science, we lay the groundwork for understanding complex analytical techniques and strategic approaches. Step-by-step instructions on leveraging data science tools and platforms provide a practical framework for aspiring data scientists to hone their skills. In addition to practical guidance, tips and tricks offer valuable insights into streamlining analytical processes and troubleshooting common challenges encountered in data science projects.
Industry Updates
Staying abreast of the latest Industry Updates in the realm of data science is essential for professionals seeking to excel in this field. Analyzing recent developments in technology and data analytics, we gain insights into the shifting landscape of the tech industry. By examining market trends and their implications for businesses and consumers, we can anticipate the potential impact on data science practices. Understanding industry updates equips data scientists with the knowledge and foresight to adapt to emerging technologies and leverage them for competitive advantage.
Understanding the Data Scientist Profile
In the intricate realm of technology and data-driven decision-making, understanding the essence of a data scientist holds paramount significance. The role of a data scientist serves as a linchpin, orchestrating the symphony of insights and analytics to drive business outcomes. Within this article, we unravel the multifaceted qualities that delineate a proficient data scientist, drawing attention to the intricate amalgamation of technical acumen, analytical prowess, and strategic thinking that distinguishes these modern-day analytics magicians. By magnifying the importance of comprehending the Data Scientist Profile, we embark on a journey to illuminate the core competencies and attributes requisite for thriving in this domain.
Technical Proficiency
Mastery of Programming Languages
Delving into the intricacies of Mastery of Programming Languages unveils a cornerstone in a data scientist's skill set. The proficiency in languages like Python, R, or SQL is akin to wielding a potent tool for navigating the labyrinth of data analysis and modeling. The adept handling of coding languages empowers data scientists to manipulate, clean, and extract insights from vast datasets efficiently. Embracing Mastery of Programming Languages bestows data scientists with a competitive edge, enabling them to craft robust algorithms and data pipelines with finesse and accuracy.
Expertise in Data Wrangling
The realm of data science echoes with the resonance of Data Wrangling expertise as a pivotal component of a data scientist's arsenal. Data Wrangling entails the art of cleansing, transforming, and preparing raw data for analysis, constituting the bedrock of insightful decision-making. A data scientist's prowess in Data Wrangling ensures the harmonization of disparate data sources into a coherent format, paving the way for seamless data exploration and interpretation. Embracing Expertise in Data Wrangling catalyzes efficient data processing, reinforcing the foundation for deriving meaningful conclusions from complex datasets.
Advanced Statistical Knowledge
Embarking on the terrain of Advanced Statistical Knowledge unfurls a tapestry of indispensable skills for any data scientist. Acquaintance with statistical concepts, hypothesis testing, and predictive modeling imparts data scientists with the acumen to unravel intricate patterns and trends within datasets. Advanced Statistical Knowledge underpins the construction of robust machine learning models and aids in discerning correlations amidst voluminous data points. The adroit utilization of statistical methodologies accentuates a data scientist's ability to glean valuable insights, propelling data-driven decision-making to new heights.
Analytical Skills
Critical Thinking Abilities
Critical Thinking Abilities constitute the bedrock of a data scientist's analytical acuity, encapsulating the proficiency to dissect complex problems and devise innovative solutions. The adept application of logic and reasoning enables data scientists to navigate ambiguity, unearth hidden patterns, and formulate data-driven strategies. Pioneering Critical Thinking Abilities empowers data scientists to approach challenges with a structured mindset, fostering a culture of astute problem-solving and robust decision-making.
Pattern Recognition
The prowess of Pattern Recognition serves as a beacon guiding data scientists through the labyrinth of data complexity, facilitating the identification of recurring trends and anomalies within datasets. Proficiency in recognizing patterns empowers data scientists to distill meaningful insights from noise, extracting valuable nuggets of information essential for informed decision-making. Harnessing the art of Pattern Recognition amplifies a data scientist's capacity to unravel intricate relationships within data sets and forecast future trends with precision and accuracy.
Problem-Solving Aptitude
The Problem-Solving Aptitude of a data scientist epitomizes the ability to navigate challenges with ingenuity and finesse, charting pragmatic pathways towards solutions. Armed with a blend of creativity and systematic reasoning, data scientists adept in problem-solving transcend hurdles, transform complexity into simplicity, and drive data-centric innovations. Cultivating Problem-Solving Aptitude not only sharpens analytical prowess but also augments a data scientist's resilience in the face of intricate data puzzles, sculpting them into agile problem solvers capable of conquering data conundrums.
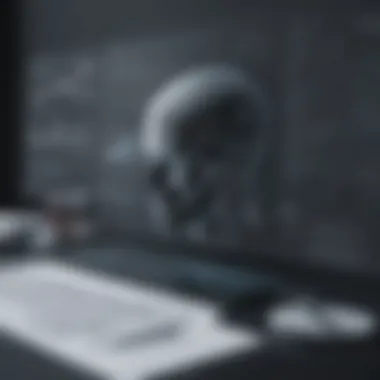
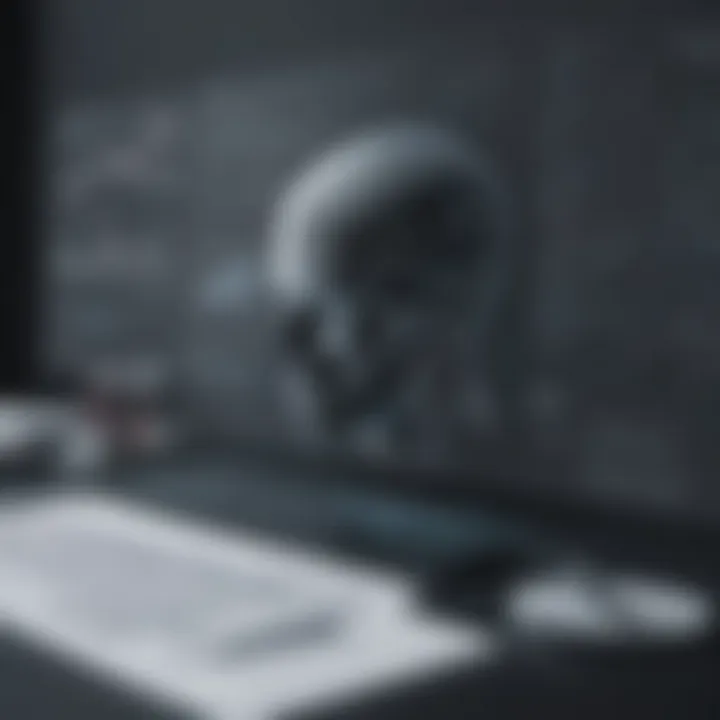
Business Acumen
Understanding of Industry Dynamics
The realm of data science not only demands technical prowess but also necessitates an acute Understanding of Industry Dynamics. Data scientists immersed in industry nuances possess the foresight to align analytics initiatives with organizational objectives, steering data-driven strategies towards impactful outcomes. A profound comprehension of industry dynamics equips data scientists to contextualize insights within broader business landscapes, catapulting them into the realm of strategic advisors poised to shape organizational trajectories.
Alignment with Organizational Goals
Alignment with Organizational Goals serves as the lodestar guiding data scientists towards actionable insights that resonate with business imperatives. Embedding organizational objectives at the core of data science endeavors fuels a synergy between data analytics and business outcomes, ensuring that data-driven interventions resonate with strategic directives. By fostering Alignment with Organizational Goals, data scientists transcend mere data crunching to emerge as strategic partners, sculpting data narratives that fuel business growth.
Communication Skills
Communication Skills emerge as the cornerstone of effective data storytelling, encapsulating the art of translating complex insights into compelling narratives for diverse stakeholders. Proficient communication bridges the gap between data science findings and actionable outcomes, fostering a shared understanding of analytical insights across organizational echelons. Nurturing Communication Skills empowers data scientists to elevate their impact, articulating data-driven recommendations with clarity and persuasiveness to drive informed business decisions.
Ethical Considerations
Data Privacy Awareness
Data Privacy Awareness stands as a foundational pillar underpinning the ethical practice of data science, heralding the safeguarding of sensitive information and user confidentiality. Data scientists well-versed in data privacy protocols navigate the ethical tightrope of data usage with prudence and integrity, ensuring the ethical handling of personal information within analytics frameworks. Upholding Data Privacy Awareness not only cultivates trust with stakeholders but also reinforces data-driven initiatives with a robust ethical foundation, positioning data scientists as custodians of data integrity and privacy.
Fairness and Bias Mitigation
The pursuit of Fairness and Bias Mitigation underscores a data scientist's commitment to eradicating algorithmic biases and ensuring equitable data practices. Mitigating biases in data algorithms fosters fair decision-making processes, curbing the perpetuation of discriminatory outcomes in data-driven initiatives. Prioritizing Fairness and Bias Mitigation in data science endeavors fortifies the ethical fabric of analytics, engendering a culture of inclusivity and equitable access to data-driven insights.
Transparency in Decision-Making
Transparency in Decision-Making embodies the ethos of openness and accountability in data science practices, exemplifying the traceability and comprehensibility of analytical processes and outcomes. Infusing transparency into decision-making cultivates trust among stakeholders, elucidating the rationale behind data-driven conclusions and fostering a culture of data accountability. The practice of Transparency in Decision-Making bolsters the credibility of data science initiatives, engendering a culture of transparency and ethical stewardship within the data science ecosystem.
Educational Background and Qualifications
In the realm of data science, one cannot overlook the significance of a robust educational foundation. Educational background and qualifications play a crucial role in shaping the expertise and capabilities of a data scientist. Individuals with degrees in Computer Science, Mathematics, or Statistics possess a strong analytical mindset and a solid foundation in quantitative reasoning. These disciplines provide a comprehensive understanding of algorithms, probability theory, and data structures, which are fundamental in data science. Moreover, pursuing certifications in data science specializations further enhances one's credibility and knowledge in the field, demonstrating a commitment to continuous learning and professional development.
Degrees in Computer Science, Mathematics, or Statistics
Degrees in Computer Science, Mathematics, or Statistics offer a diverse skill set that aligns perfectly with the requirements of a data scientist role. The emphasis on logical reasoning, mathematical modeling, and problem-solving skills distinguishes these degrees. Computer Science programs equip individuals with programming proficiency, essential for handling and analyzing large datasets. Mathematics coursework enhances statistical reasoning and quantitative analysis capabilities, which are vital for extracting actionable insights from data. Degrees in Statistics provide a deep understanding of data variability and distributions, crucial for making reliable predictions and decisions in data science initiatives.
Certifications in Data Science Specializations
On the other hand, certifications in data science specializations serve as validation of one's expertise in specific areas of data science. These certifications cover advanced topics such as machine learning, data visualization, and big data analytics, enabling professionals to deepen their knowledge and stay current with industry trends. By earning these certifications, individuals showcase their dedication to mastering new technologies and methodologies, which is highly valued in the competitive data science landscape.
Continuous Learning Initiatives
Continuous learning is a cornerstone of success in the dynamic field of data science. Engaging in online courses, attending data science workshops, and participating in industry conferences are instrumental in staying abreast of emerging trends and expanding one's professional network. These initiatives not only provide exposure to the latest tools and technologies but also foster collaboration and idea exchange among industry peers.
Engagement in Online Courses
Online courses offer flexibility and accessibility, allowing data scientists to enhance their skills at their own pace. They cover a wide range of topics, from foundational concepts to advanced techniques, catering to professionals at different stages of their careers. The interactive nature of online courses facilitates hands-on learning, enabling individuals to apply theoretical knowledge to real-world projects and scenarios.
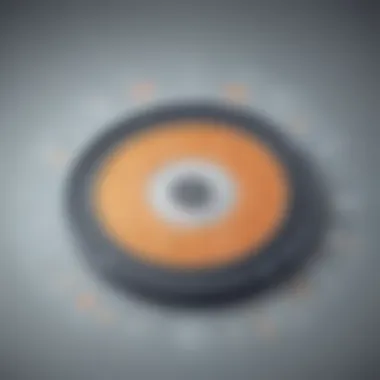
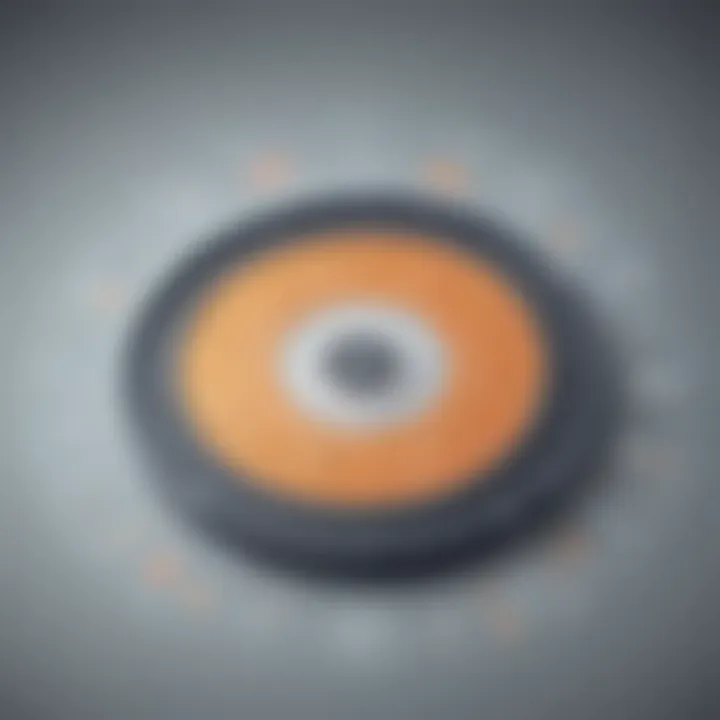
Attendance of Data Science Workshops
Data science workshops provide a condensed learning experience focused on specific topics or tools. These workshops often feature industry experts and thought leaders who share practical insights and best practices. By attending workshops, data scientists can gain in-depth knowledge about cutting-edge technologies and methodologies, accelerating their learning curve and equipping them with relevant skills for solving complex data challenges.
Participation in Industry Conferences
Industry conferences serve as knowledge hubs where data scientists converge to exchange ideas, insights, and innovations. These events feature keynote presentations, panel discussions, and networking opportunities that foster collaboration and professional growth. By participating in industry conferences, data scientists can gain exposure to diverse viewpoints, stay informed about industry trends, and form valuable connections with experts and peers.
Work Experience and Industry Exposure
Work experience and industry exposure play a pivotal role in shaping a data scientist's expertise. Through hands-on experience in diverse industries, data scientists refine their skills and adapt to the dynamic demands of the field. Exposure to real-world data scenarios enhances problem-solving capabilities and fosters a practical understanding of data science applications. Moreover, working in different industry verticals exposes data scientists to various data challenges and allows them to develop industry-specific insights and expertise.
Relevant Job Roles
Data Analyst Positions
Data analyst positions are key entry points for professionals aspiring to venture into the realm of data science. These roles focus on gathering, analyzing, and interpreting data to extract valuable insights. Data analysts play a crucial role in data preprocessing, descriptive analytics, and deriving actionable recommendations from data sets. The emphasis on data visualization and reporting makes data analyst positions ideal for individuals keen on honing their analytical and communication skills within the data science domain.
Machine Learning Engineer Roles
Machine learning engineer roles revolve around designing and implementing machine learning algorithms to develop AI-based solutions. These roles require a strong foundation in statistics, programming, and data modeling. Machine learning engineers are tasked with optimizing algorithms, training models, and deploying them in real-world applications. The complexity and innovation inherent in machine learning engineer roles appeal to individuals inclined towards exploring cutting-edge technologies and driving AI advancements.
Data Science Consultant Engagements
Data science consultant engagements involve collaborating with organizations to provide data-driven solutions and strategic recommendations. Consultants leverage their expertise in data analysis, statistical modeling, and problem-solving to address unique business challenges. Data science consultants navigate complex data landscapes, identify trends, and deliver actionable insights to drive informed decision-making. The consultancy aspect of these engagements appeals to individuals interested in applying data science principles in a consultancy setting.
Industry Verticals
Finance and Banking
The finance and banking sector presents data scientists with a multitude of opportunities to leverage data for enhancing operational efficiency and risk management. Analyzing vast financial datasets, detecting fraud patterns, and optimizing trading strategies are key focal points within this industry vertical. Data scientists working in finance and banking gain exposure to high-frequency trading systems, regulatory compliance analytics, and predictive modeling for investment decisions.
Healthcare and Pharmaceuticals
In the healthcare and pharmaceuticals industry, data scientists contribute to revolutionizing patient care, drug discovery, and clinical research. The analysis of medical records, genomic data, and drug responses enables data scientists to drive precision medicine initiatives and optimize healthcare delivery. Data scientists working in healthcare and pharmaceuticals engage in predictive analytics for disease diagnosis, personalized treatment recommendations, and epidemiological trend analysis.
E-commerce and Retail
E-commerce and retail industries rely heavily on data-driven insights to enhance customer experiences, optimize inventory management, and personalize marketing strategies. Data scientists involved in this sector focus on customer segmentation, demand forecasting, and recommendation systems to improve sales and customer loyalty. By analyzing customer behavior data, transaction histories, and market trends, data scientists in e-commerce and retail drive targeted marketing campaigns and operational efficiencies.
In the ever-evolving landscape of technology and data-centric decision-making, the role of a data scientist emerges as a pivotal force. This discourse aims to intricately dissect the multifaceted qualities that delineate a proficient data scientist, shedding light on the intricate amalgamation of technical acumen, analytical prowess, and strategic thinking that typify these contemporary analytics maestros.
Tools and Technologies Proficiency:
In this article, emphasis is placed on delving into the crux of 'Tools and Technologies Proficiency' - a cornerstone in the arsenal of a data scientist. Understanding and mastery of various tools and technologies hold paramount significance in the realm of data science, playing a vital role in deciphering complex datasets and deriving actionable insights. This section delves deep into the significance and nuances of tools and technologies proficiency, highlighting its crucial role in the realm of data science.
Data Wrangling Tools:
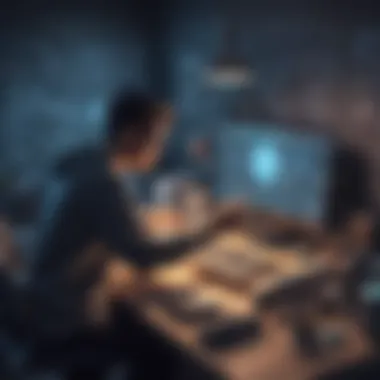
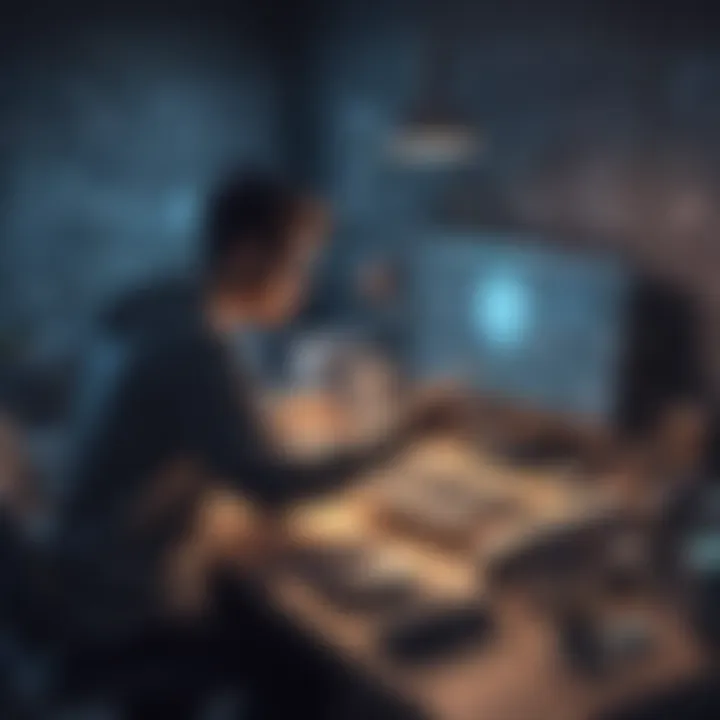
Python pandas:
Python pandas, with its robust data manipulation and analysis capabilities, plays a pivotal role in the realm of data science. Its user-friendly syntax and extensive functionality make it a go-to choice for data professionals. The versatility and efficiency of Python pandas streamline data preprocessing tasks, enhancing efficiency and reducing turnaround time in data wrangling processes.
R Programming:
R Programming's statistical computing and graphics capabilities are instrumental in data analysis and visualization. Its rich ecosystem of packages and libraries caters to various data science requirements, making it a prevalent choice among data scientists for statistical functions and exploratory data analysis.
SQL Databases:
SQL Databases form the backbone of data storage and retrieval in most organizations. The structured query language facilitates seamless interaction with databases, enabling efficient data retrieval and manipulation. Its relational database management system enhances data integrity and scalability in handling vast datasets.
Machine Learning Frameworks:
Machine learning frameworks such as Scikit-learn, Tensor Flow, and PyTorch play a pivotal role in developing robust machine learning models. Scikit-learn's simplicity and efficiency in implementing machine learning algorithms, alongside TensorFlow and PyTorch's deep learning capabilities, offer diverse options for data scientists to build and deploy advanced models for predictive analytics and pattern recognition.
Data Visualization Platforms:
Data visualization platforms like Tableau, Power BI, and Matplotlib enable data scientists to present insights in a visually compelling manner. Tableau's interactive dashboards, Power BI's integration capabilities with other tools, and Matplotlib's customization options empower data scientists to create intuitive visualizations that facilitate better understanding and decision-making based on data-driven insights.
This comprehensive guide sheds light on the integral role of 'Tools and Technologies Proficiency' in the realm of data science, underpinning the essence of a data scientist's toolkit in navigating the complexities of data analysis and interpretation.
Soft Skills and Personal Traits
In the realm of data science, soft skills and personal traits play a vital role in shaping the effectiveness and success of a data scientist. These qualities go beyond technical proficiency, serving as the backbone that supports seamless integration within teams and effective communication across diverse stakeholders. Emphasizing the significance of soft skills and personal traits in this article highlights the nuanced blend of human-centric abilities that complement the data-driven nature of the field, ultimately contributing to well-rounded and impactful data scientists.
Team Collaboration
Effective Communication
Effective communication is a cornerstone of team collaboration in data science. Its role in facilitating clear information dissemination, fostering understanding, and promoting collaborative problem-solving cannot be overstated. The ability to articulate complex concepts in a concise and accessible manner is a key characteristic of effective communication that proves invaluable in driving successful project outcomes. In this article, effective communication stands out as a foundational aspect that bridges the gap between technical expertise and practical application, ensuring that insights are communicated effectively to stakeholders for informed decision-making.
Conflict Resolution
Conflict resolution is an essential skill that contributes to cohesive teamwork and project harmony in the data science domain. By addressing conflicts constructively and seeking mutually beneficial solutions, data scientists can maintain team dynamics and minimize disruptions to workflow. The key characteristic of conflict resolution lies in its ability to navigate disagreements and differing perspectives with tact and diplomacy, fostering a collaborative environment where diverse viewpoints contribute to innovative problem-solving approaches. In this article, conflict resolution emerges as a crucial factor in maintaining team synergy and productivity amidst challenges.
Leadership Potential
Leadership potential holds substantial value in driving project success and team performance in data science endeavors. The ability to inspire, guide, and motivate team members towards shared goals elevates the collective output and fosters a culture of continuous improvement. The key characteristic of leadership potential lies in its capacity to influence and empower others, leveraging individual strengths to achieve common objectives. Recognizing and cultivating leadership potential in data scientists featured in this article underscores the importance of nurturing future industry leaders who can steer projects towards innovation and sustainable growth.
Adaptability and Learning Agility
Embracing Change
Embracing change is a pivotal aspect of adaptability and learning agility in data science practice. The ability to seamlessly transition and adapt to evolving technologies, methodologies, and project requirements is essential in staying relevant and effective in a dynamic landscape. The key characteristic of embracing change lies in cultivating a growth mindset that embraces challenges as opportunities for growth and development. In this article, embracing change emerges as a driving force that propels data scientists towards continuous learning and adaptation, fostering resilience in the face of ever-changing industry demands.
Quick Grasping of New Concepts
Quickly grasping new concepts is a fundamental trait that enhances learning agility and cognitive flexibility in data science roles. The capacity to assimilate and apply new information rapidly enables data scientists to stay ahead of industry trends, innovate solutions, and adapt to fast-paced project requirements. The key characteristic of quick grasping of new concepts lies in the efficiency and efficacy of knowledge acquisition, empowering data scientists to make informed decisions and drive impactful outcomes. Highlighting quick grasping of new concepts in this article underscores its role as a catalyst for professional growth and expertise expansion in the dynamic field of data science.
Curiosity and Inquisitiveness
Curiosity and inquisitiveness fuel a culture of exploration, experimentation, and continuous learning in data science practice. The innate drive to question, investigate, and seek deeper understanding drives innovation, problem-solving, and knowledge expansion. The key characteristic of curiosity and inquisitiveness lies in their role as catalysts for discovery and creativity, inspiring data scientists to push boundaries and explore new possibilities. Addressing the significance of curiosity and inquisitiveness in this article underscores their transformative impact on driving curiosity-driven research, novel discoveries, and trailblazing advancements in the realm of data science.