Revolutionizing Factory Automation: The Impact of Machine Learning on Manufacturing Processes
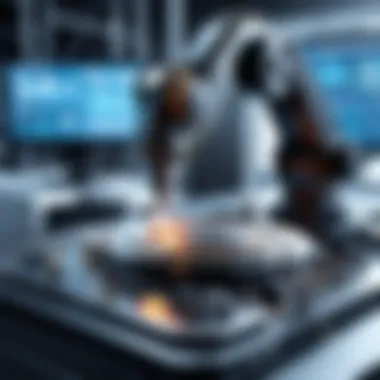
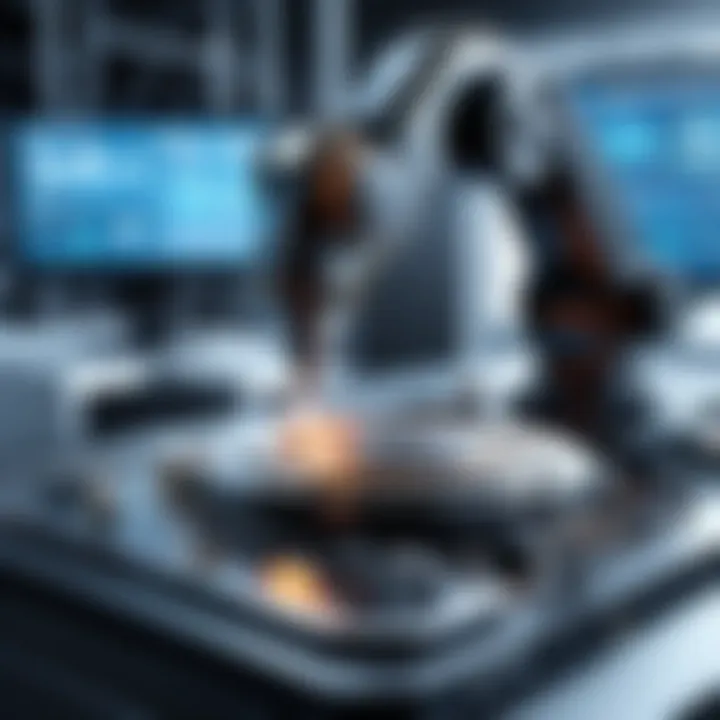
Tech Trend Analysis
In an era of rapid technological advancements, the impact of machine learning on factory automation is a pivotal trend reshaping the industry. From streamlining production processes to optimizing resource allocation, the integration of advanced technologies like machine learning is revolutionizing traditional manufacturing approaches. Consumers stand to benefit significantly from enhanced efficiency and precision in manufacturing, leading to improved product quality and reduced lead times. Looking ahead, the possibilities are vast, with forecasts indicating a continued evolution towards autonomous systems and data-driven decision-making in manufacturing.
Industry Updates
Recent developments in the tech industry are centering around the convergence of artificial intelligence and automation, with machine learning playing a crucial role in driving efficiency and innovation in factory settings. Analyzing market trends reveals a growing adoption of AI-driven solutions across various sectors, including manufacturing. Businesses and consumers alike are experiencing the transformative impact of these technologies, with improved productivity and cost-effectiveness becoming defining factors in the competitive landscape. As automation continues to redefine industry standards, staying informed about the latest advancements is essential for industry professionals navigating this dynamic landscape.
Introduction
In the realm of manufacturing, the integration of machine learning stands as a pivotal advancement, heralding a new era of efficiency and innovation. The evolution of factory automation through the lens of machine learning is a topic of pertinence, resonating deeply within the industry's core. This section serves as a gateway into understanding the profound implications of harnessing machine learning in the manufacturing landscape. By delving into the intricacies of this transformative technology, we unravel the layers of impact it brings to the way factories operate and streamline their processes. Exploring the benefits, challenges, and considerations surrounding the implementation of machine learning is paramount in comprehending its significance within the context of factory automation.
Understanding Machine Learning
-#### Definition and Basics : Machine learning, in essence, revolves around the utilization of algorithms to enable computer systems to learn from and make predictions or decisions based on data sets. This fundamental aspect of machine learning serves as the backbone of predictive analytics and automated model building. The capacity to iteratively learn from data without being explicitly programmed distinguishes machine learning from traditional computational approaches. While the autonomous learning ability of machine learning systems streamlines processes and enhances efficiency, the potential reliance on vast amounts of data poses challenges in data quality and processing speed.
-#### Types of Machine Learning : The taxonomy of machine learning bifurcates into supervised, unsupervised, and reinforcement learning paradigms, each tailored to distinct learning objectives. Supervised learning involves training models on labeled data to make predictions or classifications, ideal for tasks with a clear outcome. Unsupervised learning, conversely, deals with unlabeled data to reveal underlying patterns or structures, facilitating tasks where predictions are not predetermined. Reinforcement learning fosters agent-based learning through trial and error methods to maximize rewards by exploring various actions. Selecting the most suitable machine learning type hinges on the specific needs and goals of the manufacturing environment.
-#### Applications in Various Industries : The versatility of machine learning finds extensive applications across diverse industries, ranging from healthcare to finance, and prominently within manufacturing. In the manufacturing sector, machine learning orchestrates predictive maintenance models, anomaly detection systems, and real-time quality control mechanisms. The integration of machine learning algorithms optimizes production processes, enhances product quality, and augments operational efficiency. Despite the manifold advantages conferred by machine learning applications, considerations regarding data privacy, model interpretability, and ethical implications underscore the need for robust frameworks that address these challenges in industrial settings.
Evolution of Factory Automation
-#### Traditional Automation Methods : The historical trajectory of factory automation traces back to the deployment of mechanized systems to execute repetitive tasks with precision and consistency. Traditional automation methods relied on fixed programming and mechanical components to drive production lines and assembly processes. While instrumental in enhancing operational efficiency, these methodologies lacked adaptability and intelligence, constraining the flexibility of manufacturing systems. The shift towards machine learning heralds a paradigmatic evolution from deterministic automation towards cognitive automation, infused with learning capabilities that enable dynamic responses to changing production demands.
-#### Challenges Faced : Overcoming challenges associated with legacy automation systems constitutes a substantial hurdle in the transition towards machine learning-driven automation. Legacy systems exhibit siloed data sources, compatibility issues with newer technologies, and limited scalability in accommodating evolving manufacturing requirements. The complexity of integrating machine learning algorithms within existing frameworks necessitates substantial re-engineering efforts and workforce upskilling initiatives. Addressing these challenges mandates a holistic approach towards technology adoption and organizational preparedness to navigate the intricacies of automation transformation.
-#### Need for Advanced Solutions : The imperative for advanced solutions in factory automation emanates from the exigency to amplify operational agility, precision, and adaptability amidst evolving market dynamics. The infusion of machine learning augments the responsiveness of manufacturing systems to real-time data insights, predictive analytics, and autonomous decision-making capabilities. Unlocking the potential of advanced solutions in factory automation entails embracing integrated AI-assisted technologies, user-centric interfaces, and scalable architectures that streamline production workflows and harmonize human-machine interactions. The symbiosis between advanced solutions and machine learning fosters an ecosystem of innovative practices that catalyze sustainable growth and competitive edge in the manufacturing landscape.
Integration of Machine Learning in Manufacturing
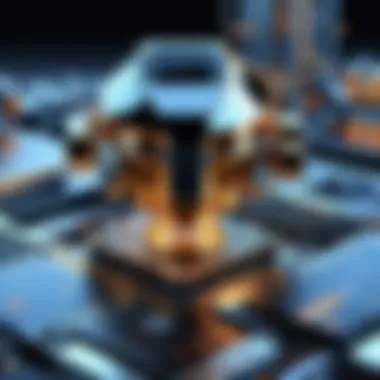
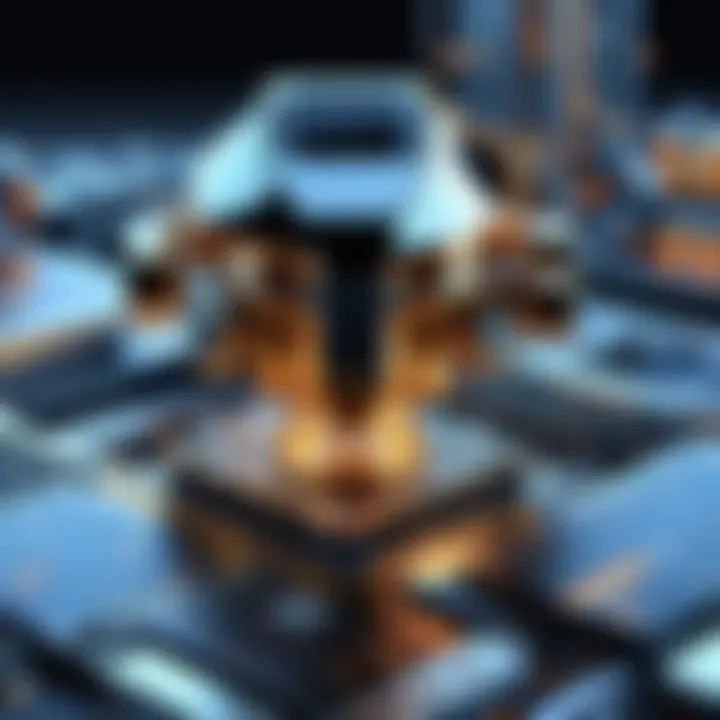
In delving into the realm of revolutionizing factory automation through the lens of machine learning, the integration of this cutting-edge technology into the manufacturing sector emerges as a critical focal point. As Industry 4.0 continues to reshape traditional manufacturing processes, the role of machine learning in driving efficiency and productivity becomes increasingly pronounced. The symbiosis between meticulous data analysis and real-time decision-making not only streamlines operations but also paves the way for predictive insights that empower businesses to preemptively address issues before they escalate.
Benefits of Implementing Machine Learning
Enhanced Precision and Accuracy
Within the landscape of factory automation, enhanced precision and accuracy stand as pillars of efficiency and quality assurance. By harnessing machine learning algorithms to fine-tune production processes, manufacturers can achieve unparalleled levels of precision in product development and streamlined operations. The ability to reduce variations, minimize errors, and optimize workflows through data-driven insights elevates the overall quality of output, thereby bolstering competitiveness in the market.
Predictive Maintenance
Gone are the days of reactive maintenance as predictive maintenance takes center stage in the manufacturing domain. Machine learning's predictive capabilities empower industrial players to anticipate equipment failures, schedule maintenance activities efficiently, and mitigate downtime risks. By leveraging historical data and real-time monitoring, predictive maintenance optimizes asset performance, prolongs machinery lifespan, and ultimately, reduces operational costs.
Optimized Production Processes
Optimizing production processes through machine learning not only accelerates time-to-market but also enhances resource utilization and operational efficiency. The agility afforded by dynamic production adjustments based on real-time insights ensures that manufacturers remain adaptable to market fluctuations and consumer demands. From predictive inventory management to just-in-time production scheduling, machine learning fosters a responsive manufacturing ecosystem that epitomizes efficiency and sustainability.
Case Studies of Successful Integration
Automotive Industry
In the automotive sector, the integration of machine learning has revolutionized vehicle manufacturing, enabling agile production lines and customizations at scale. By embedding machine learning algorithms in quality control processes, automakers can identify defects early, optimize supply chain logistics, and streamline production workflows for seamless operations. The result is a paradigm shift towards smarter, more efficient manufacturing practices that redefine industry benchmarks.
Electronics Manufacturing
Within electronics manufacturing, machine learning drives innovation by enhancing product design, optimizing supply chain management, and automating quality assurance processes. From automated PCB inspection to real-time demand forecasting, machine learning algorithms empower electronics manufacturers to achieve operational excellence while delivering cutting-edge products that meet consumer demands for efficiency and reliability.
Food Processing
The food processing industry witnesses a transformational impact through the integration of machine learning, where optimization of production processes and quality control protocols ensures food safety standards and operational efficiency. By implementing machine learning for predictive maintenance of processing equipment, real-time monitoring of perishable goods, and supply chain optimization, food processors can mitigate risks, reduce waste, and ensure adherence to regulatory compliance, all within a rapidly evolving market landscape.
Challenges and Considerations
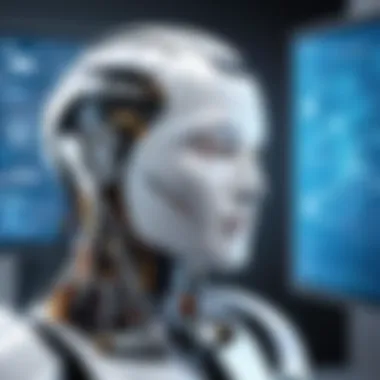
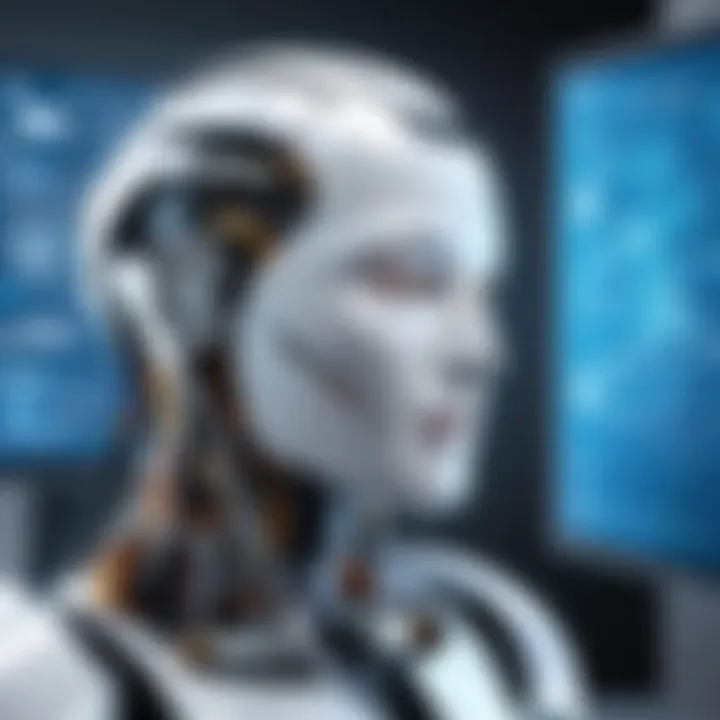
Machine learning's integration into manufacturing revolutionizes processes, yet poses unique challenges and considerations that demand attention. Within this realm, factors like data security, privacy, and ethical practices require meticulous scrutiny. Addressing these crucial elements ensures the seamless and ethical deployment of machine learning technologies within industrial setups.
Data Security and Privacy
Protecting Sensitive Information:
In the context of factory automation and machine learning, safeguarding sensitive data remains paramount. The step of Protecting Sensitive Information involves implementing robust encryption protocols, access controls, and data anonymization techniques. These measures aim to fortify the confidentiality of critical data points within manufacturing systems, reducing susceptibility to cyber threats or unauthorized access. The characteristic strength of Protecting Sensitive Information lies in its ability to ensure the integrity and confidentiality of proprietary data, fostering trust and reliability across automated processes.
Regulatory Compliance:
Furthermore, maintaining Regulatory Compliance stands as a crucial pillar in the seamless amalgamation of machine learning within manufacturing frameworks. Manufacturers must adhere to stringent industry regulations and guidelines to uphold transparency and accountability in data processing practices. Regulatory Compliance dictates the ethical handling and utilization of data, necessitating adherence to standards like GDPR, HIPAA, or industry-specific mandates. Embracing Regulatory Compliance not only mitigates legal risks but also cultivates a culture of responsible data management and governance within factory automation ecosystems.
Ethical Implications:
Beyond technicalities, Ethical Implications delve into the moral and philosophical considerations arising from the widespread adoption of machine learning in manufacturing. Ethical decision-making frameworks guide practitioners in navigating dilemmas related to data bias, algorithmic accountability, and societal impacts. Acknowledging Ethical Implications prompts introspection into the ethical ramifications of automated decision-making processes, urging a conscientious approach towards integrating machine learning seamlessly while upholding societal well-being and moral standards.
Skill Gap and Training Needs
Upskilling the Workforce:
Addressing the evolving landscape of factory automation demands a concerted effort towards Upskilling the Workforce. Equipping employees with advanced technical competencies, such as data analysis, machine learning algorithms, and automation maintenance, is imperative for optimizing workforce efficiency. Upskilling initiatives nurture a skilled workforce capable of harnessing the full potential of machine learning technologies, resulting in enhanced productivity and operational excellence within manufacturing facilities.
Adapting to Technological Changes:
Amidst rapid technological advancements, Adapting to Technological Changes emerges as a pivotal aspect for successful integration of machine learning in manufacturing. Industries must embrace agile methodologies, flexible frameworks, and adaptive practices to accommodate evolving technological landscapes. An organization's readiness to pivot and innovate in response to technological shifts ensures resilience and sustainability in the face of disruptive changes, fostering a culture of continuous improvement and adaptability.
Investing in Continuous Learning:
Striving for excellence in factory automation warrants continuous investment in Learning and Development initiatives. Establishing avenues for employees to engage in ongoing training programs, workshops, and certifications fosters a culture of continuous learning within manufacturing environments. Investing in Continuous Learning not only enhances employee retention and satisfaction but also bolsters organizational competitiveness by nurturing a workforce equipped with the latest industry insights and technical proficiencies.
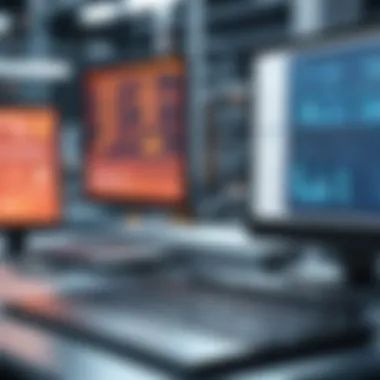
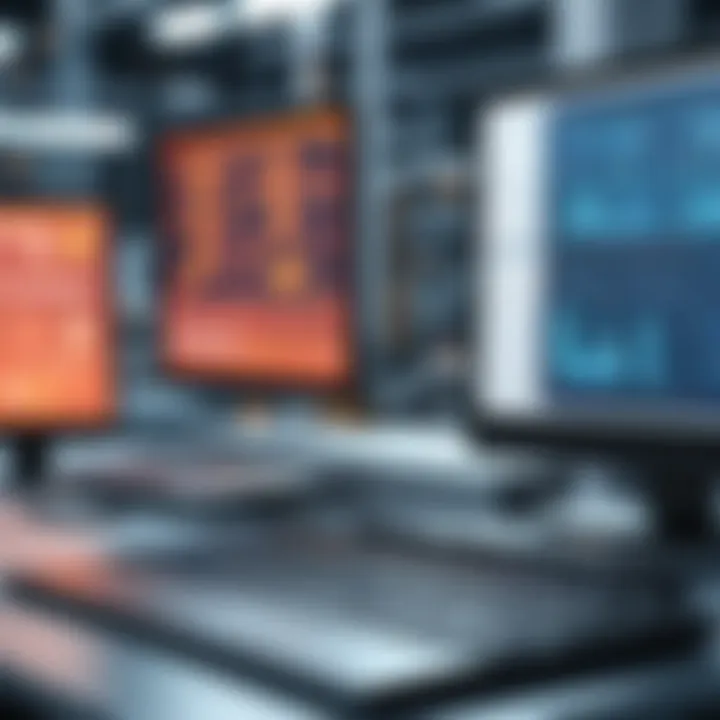
Future Trends and Implications
In the realm of revolutionizing factory automation, looking towards future trends and implications holds paramount significance. It provides a glimpse into the trajectory that this technological evolution will take, shedding light on upcoming advancements that will shape the manufacturing landscape. Understanding these future trends is essential for businesses to stay ahead of the curve and leverage emerging technologies effectively. By delving into the potential implications of these trends, companies can proactively adapt their strategies to embrace innovation and drive sustainable growth.
Emerging Technologies in Automation
Internet of Things (IoT)
The Internet of Things (Io T) stands out as a pivotal element in the evolution of factory automation. It revolutionizes connectivity by interlinking devices and systems, enabling seamless data exchange and real-time monitoring. IoT's key characteristic lies in its ability to enhance operational efficiency through automation and remote management. Its ubiquity and scalability make it a popular choice for integrating machine learning into manufacturing processes. However, challenges such as data security and interoperability should not be overlooked while leveraging IoT in factory automation.
Robotics Integration
Robotics integration plays a crucial role in redefining manufacturing operations by automating repetitive tasks and enhancing productivity. The key characteristic of robotics lies in its ability to perform tasks with precision and consistency, minimizing errors and reducing cycle times. Integrating robotics into factory automation proves beneficial, especially in high-speed production environments. Despite its advantages, considerations about initial setup costs and continuous maintenance should be factored into the decision-making process.
Cloud Computing
Cloud computing emerges as a game-changer in factory automation, offering scalable storage solutions and computing power on-demand. Its key characteristic lies in providing a virtualized environment for data processing and analysis, enabling seamless access to critical information from anywhere. The flexibility and cost-efficiency of cloud computing make it an attractive choice for manufacturing enterprises looking to streamline operations. However, ensuring data security and compliance with industry regulations remain critical aspects of adopting cloud-based solutions.
Impact on Employment Landscape
Exploring the impact of future trends in factory automation on the employment landscape unveils a spectrum of changes that organizations and workers will encounter. Understanding the implications of automation versus job displacement is crucial in navigating the transition towards a more automated environment. By delineating the evolving roles of humans in automated factories, businesses can optimize workforce utilization and promote skill development. Creating new opportunities not only signifies the expansion of job roles but also underscores the need for continuous learning and adaptability among workers. Balancing automation with workforce development is pivotal in shaping a sustainable employment landscape within the realm of advanced manufacturing.
Conclusion
Envisioning the Future of Factory Automation
Continuous Evolution of Technologies
Delving into the aspect of Continuous Evolution of Technologies within the context of factory automation, it is crucial to analyze its specific role and impact on the overall discourse. This particular facet emphasizes the ongoing advancements and innovations in technology that cater to streamlining manufacturing processes. The key characteristic of this continuous evolution is its adaptability to changing industry demands and its ability to integrate seamlessly with existing automation systems. By constantly updating and improving technologies, manufacturers can stay ahead of the curve in optimizing production efficiency and quality control.
Balancing Efficiency and Human Touch
Examining the interplay between efficiency and the human element within factory automation sheds light on the necessity of finding a harmonious equilibrium. Balancing efficiency and the human touch is essential in ensuring that while processes are optimized through automation, human oversight and intervention are still valued. The key characteristic here is the recognition of human expertise in areas that require creativity, problem-solving, and adaptability, which machines may not fully replicate. By striking a balance between efficiency-driven automation and human intervention, manufacturers can achieve streamlined operations without compromising on quality or the human element.
Adoption of Sustainable Practices
The adoption of sustainable practices in the realm of factory automation contributes significantly to the long-term viability and environmental impact of manufacturing processes. This aspect emphasizes integrating eco-friendly solutions and energy-efficient practices into automated systems to reduce carbon footprint and overall resource consumption. The key characteristic lies in the commitment to minimizing environmental harm while maximizing operational efficiency. By embracing sustainable practices, manufacturers can align with global sustainability goals and ensure the longevity of their operations in an environmentally conscious manner.