Unveiling the Revolutionary Impact of Machine Learning in Financial Services

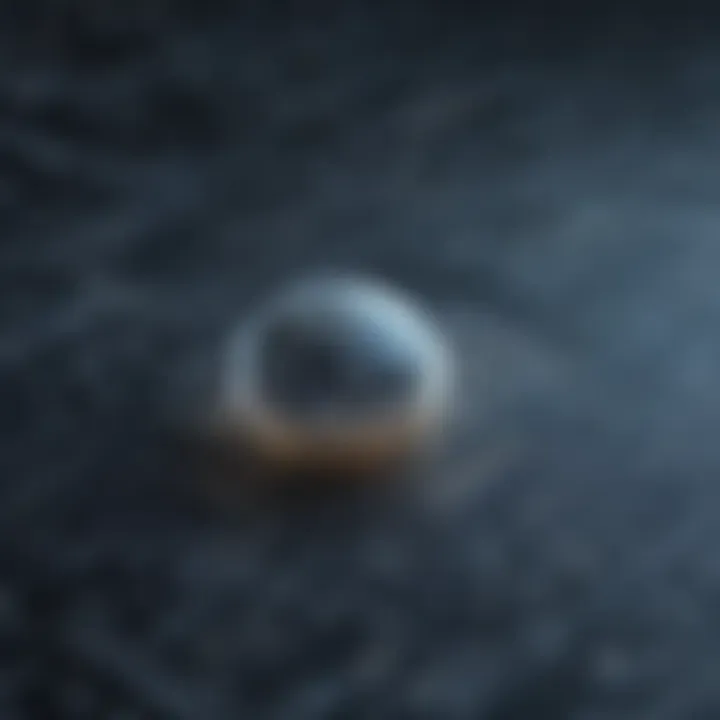
Tech Trend Analysis
In the realm of financial services, machine learning stands at the forefront of technological advancement, revolutionizing traditional practices and paving the way for enhanced customer experiences and optimized risk management strategies. This section will delve into the current trends surrounding the integration of machine learning in financial services, exploring its implications for consumers both now and in the future. As this innovative technology continues to evolve, we will also analyze future predictions and possibilities that could further shape the industry's landscape.
Product Reviews
Machine Learning Applications in Financial Services
Overview of the Product
The utilization of machine learning in financial services encompasses a broad spectrum of applications, ranging from fraud detection to personalized financial recommendations. These technologies are designed to sift through vast amounts of data to extract valuable insights, enabling financial institutions to streamline operations and provide more tailored services to their clients.
Features and Specifications
Key features of machine learning applications in financial services include data analysis, pattern recognition, and predictive modeling. By leveraging algorithms and computational tools, these applications can identify patterns, anomalies, and trends within financial data, ultimately enhancing decision-making processes and improving overall efficiency.
Performance Analysis
The performance of machine learning applications in financial services is marked by their ability to process data at scale, identify intricate patterns that may evade human detection, and adapt to evolving trends in real-time. These capabilities lead to improved accuracy in risk assessment, fraud prevention, and customer satisfaction, setting a new standard for operational excellence within the industry.
Pros and Cons
While the benefits of integrating machine learning into financial services are substantial, there are also inherent challenges to consider. Pros include enhanced data analysis, improved risk management, and personalized customer experiences. Conversely, cons may involve data privacy concerns, algorithm biases, and the need for continuous monitoring and updating of models to ensure accuracy and relevance.
Recommendation
For financial institutions looking to stay ahead in an increasingly competitive landscape, embracing machine learning technologies is essential. By leveraging the power of these innovative tools, organizations can unlock new opportunities for growth, efficiency, and customer satisfaction, ultimately establishing themselves as industry leaders in the era of digital transformation.
How-To Guides
Implementing Machine Learning in Financial Services
Introduction to the Topic
The integration of machine learning in financial services requires a strategic approach that considers the unique needs and goals of the organization. In this section, we will delve into the fundamental steps involved in implementing machine learning technologies effectively to drive value and innovation within the industry.
Step-by-Step Instructions
- Assess Organizational Needs: Begin by conducting a thorough assessment of your organization's requirements and objectives to identify key areas where machine learning can add value.
- Data Collection and Preparation: Gather relevant financial data and ensure it is structured and cleaned to facilitate accurate analysis and model building.
- Algorithm Selection and Training: Choose appropriate machine learning algorithms based on your specific use case and train them using historical data to develop predictive models.
- Testing and Validation: Validate the performance of the machine learning models using testing data sets to ensure accuracy and reliability in real-world scenarios.
- Integration and Monitoring: Integrate the machine learning models into your existing infrastructure and establish monitoring mechanisms to track performance and make iterative improvements.
Tips and Tricks
- Stay Updated: Keep abreast of the latest advancements in machine learning technology and apply relevant developments to enhance your financial services offerings.
- Collaborate with Experts: Seek collaboration with data scientists, AI specialists, and domain experts to augment your machine learning initiatives and drive innovation within your organization.
Troubleshooting
Identifying and addressing issues that may arise during the implementation of machine learning in financial services is crucial to the success of your initiatives. Common challenges include data quality issues, model inaccuracies, and interpretability concerns. By proactively troubleshooting these issues, organizations can ensure the seamless integration and optimal performance of machine learning technologies in their operations.
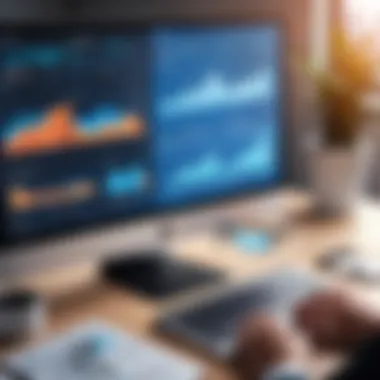
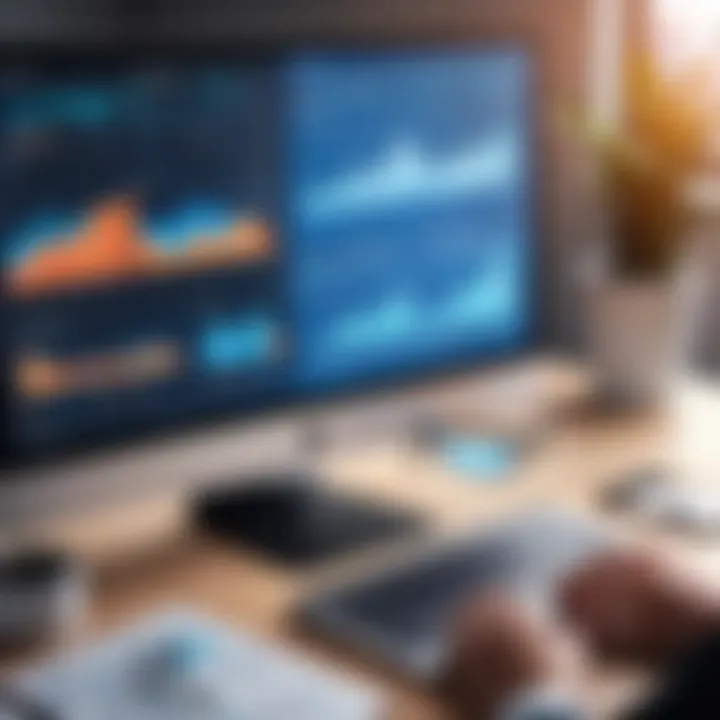
Industry Updates
The Future of Machine Learning in Financial Services
Recent Developments in the Tech Industry
The tech industry is witnessing rapid advancements in machine learning algorithms, computational tools, and data processing capabilities, presenting new opportunities for innovation in financial services. Recent developments such as the integration of AI-driven chatbots, predictive analytics, and automated trading systems are transforming the way financial institutions interact with customers and manage risk.
Analysis of Market Trends
Market trends in the financial services sector indicate a growing adoption of machine learning technologies to streamline operations, prevent fraud, and deliver hyper-personalized services to clients. As customer expectations evolve and regulatory requirements become more stringent, the role of machine learning in driving efficiency and compliance within the industry is set to expand significantly in the coming years.
Impact on Businesses and Consumers
The integration of machine learning in financial services not only benefits businesses by improving operational efficiency and reducing costs but also enhances the overall customer experience by delivering tailored services and personalized recommendations. Consumers can expect a more seamless and secure financial journey, with heightened levels of transparency, accessibility, and trust facilitated by innovative machine learning solutions.
Synthesis of Information Presented Throughout
As we navigate the complex landscape of financial services and machine learning integration, it becomes evident that the convergence of these technologies holds immense potential for reshaping industry dynamics and elevating customer experiences to new heights. By embracing the transformative power of machine learning, financial institutions can gain a competitive edge, drive innovation, and nurture lasting relationships with their clients in an ever-evolving digital ecosystem.
Introduction
In the realm of financial services, the impact of machine learning has transcended conventional paradigms, ushering in a new era of innovation and efficiency. This section of the article scrutinizes how machine learning has fundamentally altered the landscape of financial operations. It delves into the nuances of leveraging cutting-edge technology to streamline processes, enhance decision-making capabilities, and elevate customer experiences to unprecedented levels.
Understanding Machine Learning
Machine learning, a subfield of artificial intelligence, wields significant influence across various industries, including finance. Its application within financial services comprises a multitude of dimensions, each contributing uniquely to the overarching goal of operational optimization. From the fundamentals of machine learning algorithms to their diverse applications in real-world scenarios, the comprehension of these aspects is imperative for grasping the transformative essence they instill within financial frameworks.
The basics of machine learning
The essence of machine learning lies in its ability to enable systems to learn from data, identify patterns, and make decisions with minimal human intervention. This fundamental principle underpins the automation of tasks, prediction of future trends, and customization of services in the financial domain. The versatility and adaptability of machine learning algorithms render them indispensable tools for enhancing predictive analytics, risk modeling, and personalized customer interactions.
Types of machine learning algorithms
Delineating various types of machine learning algorithms illuminates the diversified approaches employed in distilling insights from data. Supervised, unsupervised, and reinforcement learning techniques each proffer distinct advantages in modeling and forecasting financial trends, detecting anomalies, and optimizing operational processes. Understanding the nuanced characteristics of these algorithms is imperative for orchestrating their integration within financial infrastructures effectively.
Applications of machine learning in various industries
The pervasive nature of machine learning applications across industries underscores its paramount significance in driving technological evolution. Within the financial sector, machine learning fuels advancements in fraud detection, credit scoring, portfolio management, and customer relationship management. These applications underscore the transformative impact of machine learning on optimizing business operations, mitigating risks, and bolstering decision-making frameworks.
Overview of the financial services sector
Nurturing a comprehensive understanding of the financial services sector sets the stage for evaluating the impact of machine learning within this domain. The intricate network of services, regulatory frameworks, and market dynamics characterizing the financial sector demands tailored machine learning solutions. Aligning machine learning initiatives with the specific requirements and challenges of financial operations fosters a synergistic amalgamation that catalyzes efficiency, innovation, and sustainability within financial institutions.
Machine Learning in Finance: A Paradigm Shift
Machine learning in finance represents a significant shift in the financial services sector, revolutionizing traditional practices and paving the way for enhanced efficiency and customer-centric services. This section explores how machine learning technologies are reshaping the industry landscape, focusing on key aspects such as personalized recommendations, fraud detection, and algorithmic trading strategies.
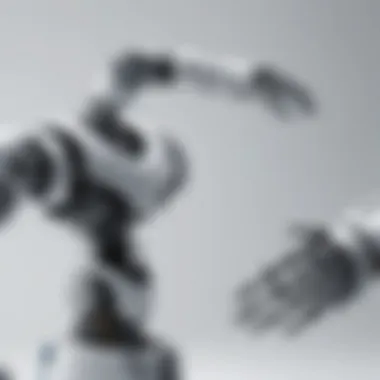
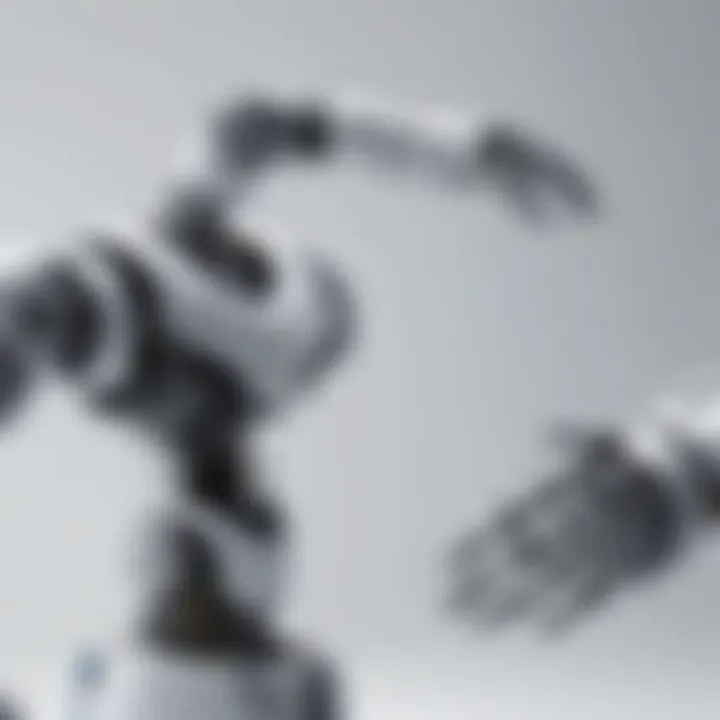
Enhancing Customer Experience
Personalized Recommendations and Services
Personalized recommendations and services play a pivotal role in redefining customer experiences within the financial services domain. By leveraging machine learning algorithms, institutions can offer tailored financial products and services based on individual preferences and behaviors. This customization enhances customer satisfaction and loyalty, ultimately driving business growth. Despite being a popular choice, the approach's advantages and disadvantages lie in its ability to predict customer needs accurately while also raising concerns about data privacy and ethical considerations.
Fraud Detection and Prevention
The adoption of machine learning for fraud detection and prevention has proven indispensable in safeguarding financial institutions and customers against fraudulent activities. Through sophisticated algorithms, anomalies and suspicious patterns can be identified in real-time, reducing financial losses and mitigating risks. The key characteristic of this approach is its proactive nature, allowing for swift responses to potential threats. However, the challenge lies in balancing accuracy with scalability, as complex models may sometimes yield false positives, impacting operational efficiency.
Chatbots and Virtual Assistants
Introducing chatbots and virtual assistants powered by machine learning algorithms has streamlined customer interactions and support services. These AI-driven tools enable quick responses to customer queries, facilitate seamless transactions, and enhance overall service accessibility. The key feature of chatbots and virtual assistants is their round-the-clock availability and consistent service quality. While their implementation results in improved user experiences, the reliance on AI poses challenges regarding communication nuances and personalized human touch.
Risk Management and Fraud Detection
Credit Scoring and Loan Approvals
Machine learning's application in credit scoring and loan approvals optimizes risk assessment processes, enabling financial institutions to make informed lending decisions swiftly. By analyzing vast amounts of data, ML algorithms can predict creditworthiness accurately, reducing the incidence of defaults and non-performing assets. The crux of this approach lies in its ability to merge efficiency with risk mitigation, ensuring sustainable loan portfolios. Nonetheless, concerns revolve around algorithmic biases and interpretability, necessitating constant monitoring and fine-tuning.
Anti-Money Laundering Efforts
Employing machine learning for anti-money laundering (AML) efforts fortifies regulatory compliance measures and deters illicit financial activities. By flagging suspicious transactions and identifying money laundering patterns, ML algorithms enhance surveillance capabilities and strengthen AML controls. The uniqueness of this approach lies in its dynamic adaptability to evolving money laundering techniques, bolstering financial sector integrity. Nevertheless, operational challenges such as model explainability and detecting complex laundering schemes persist, calling for continuous innovation and regulatory alignment.
Market Trend Analysis
The integration of machine learning for market trend analysis equips financial institutions with predictive insights into asset performance and investment opportunities. By analyzing historical data and real-time market fluctuations, ML algorithms aid in formulating strategic investment decisions and optimizing portfolio returns. The distinctive attribute of market trend analysis is its proactive nature, enabling investors to capitalize on emerging trends and mitigate risks effectively. However, the complexity of predictive models and reliance on historical data pose challenges in adapting to volatile market conditions, necessitating a balance between innovation and risk management.
Algorithmic Trading and Portfolio Management
Automated Trading Strategies
The utilization of automated trading strategies driven by machine learning algorithms revolutionizes the execution of trading activities in financial markets. By automating buysell decisions and portfolio rebalancing, these strategies optimize trading efficiencies and minimize emotional biases. The key characteristic of automated trading lies in its ability to react swiftly to market changes, ensuring timely responses and improved trade execution. While enhancing trading performance and liquidity, algorithmic trading faces scrutiny over algorithmic biases and market manipulation risks, demanding stringent regulatory oversight and robust risk management frameworks.
Predictive Analytics for Investment Decisions
Leveraging predictive analytics for investment decisions empowers financial professionals to forecast market trends and asset performances accurately. By analyzing large datasets and identifying patterns, ML algorithms offer informed insights for constructing robust investment portfolios. The central feature of predictive analytics is its data-driven approach to decision-making, enhancing investment strategies and minimizing uncertainties. Nevertheless, challenges exist in model interpretation and validation, urging investment firms to strike a balance between quantitative analysis and qualitative judgments in managing investment risks and returns.
Challenges and Opportunities
In the intricate landscape of financial services, the realm of challenges and opportunities holds paramount significance. The symbiotic relationship between identifying challenges and harnessing opportunities is imperative in driving innovation and progression. By delving deep into the challenges faced within the financial sector, organizations can strategically position themselves to unlock the untapped potential that lies within. Conversely, recognizing and seizing upon opportunities provides a competitive advantage that propels entities towards sustained growth and relevance within a dynamic ecosystem of continual change and evolution. It is through a meticulous evaluation and strategic response to challenges while simultaneously leveraging opportunities that firms can stay at the forefront of the ever-evolving financial services landscape.
Data Privacy and Security Concerns
Ensuring compliance with regulations
In the context of the financial sector, ensuring compliance with regulations stands as a linchpin in promoting trust and transparency. By adhering to regulatory frameworks meticulously crafted to uphold ethical standards and data privacy laws, financial institutions mitigate the risk of non-compliance penalties and reputational damage. The meticulous alignment with regulations such as GDPR or PCI DSS underscores the commitment towards safeguarding customer information and upholding industry best practices. Not only does this commitment enhance data security measures, but it also fosters a culture of accountability and integrity, establishing a robust foundation for sustainable growth and customer trust.
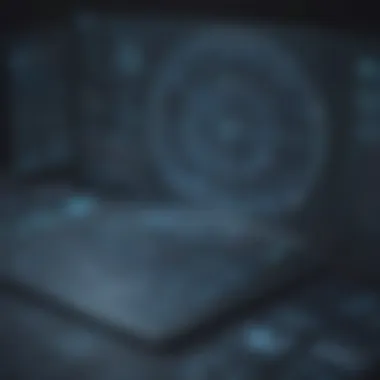
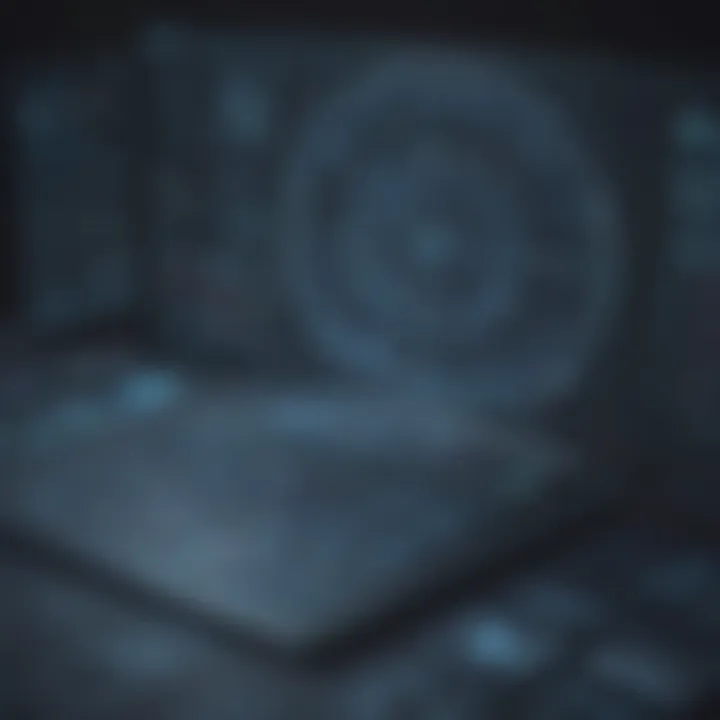
Protecting sensitive financial data
Protecting sensitive financial data acquires paramount significance in a digital era characterized by increasing cyber threats and data breaches. Efforts to safeguard critical financial information encompass a multi-faceted approach involving encryption, authentication protocols, access controls, and cybersecurity frameworks. The meticulous implementation of data encryption algorithms and secure data storage methodologies fortify the perimeter against potential cyber attacks, ensuring the confidentiality and integrity of sensitive financial data. However, the dichotomy lies in the balance between robust data protection measures and seamless user experience, where striking a harmonious equilibrium remains a perpetual challenge in the realm of financial data security.
Skills Gap and Talent Acquisition
Training employees in data science and machine learning
The paradigm shift towards data-driven decision-making necessitates a skilled workforce proficient in data science and machine learning. Equipping employees with the requisite knowledge and expertise empowers organizations to harness the transformative potential of data analytics, predictive modeling, and machine learning algorithms. By fostering a culture of continuous learning and upskilling, businesses not only fortify their internal capabilities but also cultivate a workforce adept at leveraging emerging technologies to drive innovation and strategic growth initiatives.
Attracting top talent in a competitive landscape
In a competitive talent market, the ability to attract and retain top-caliber professionals remains a strategic imperative for organizations seeking to navigate the complexities of the digital age effectively. Employing innovative recruitment strategies, emphasizing employer branding, and offering tailored professional development opportunities are instrumental in positioning a firm as an employer of choice within the competitive landscape. Moreover, fostering a work environment that values diversity, inclusivity, and innovation serves as a magnet for top talent seeking dynamic career progression and impactful contributions within the realm of financial services.
Integration with Legacy Systems
Overcoming compatibility issues
The integration of machine learning technologies with legacy systems necessitates a delicate balance between innovation and continuity. Overcoming compatibility issues requires a meticulous assessment of existing infrastructure, identification of integration points, and seamless migration strategies to ensure a synergistic convergence of old and new technologies. By harnessing interoperability standards, API integrations, and modular design principles, organizations can overcome compatibility hurdles and position themselves for enhanced operational efficiency and data-driven decision-making.
Upgrading infrastructure for seamless integration
Upgrading infrastructure to facilitate seamless integration of machine learning capabilities warrants a strategic investment in modernization and scalability. By leveraging cloud-based solutions, microservices architecture, and scalable infrastructure frameworks, businesses can future-proof their systems to accommodate the evolving demands of data analytics and machine learning applications. The meticulous planning and implementation of infrastructure upgrades enable organizations to unlock the full potential of machine learning technologies, driving innovation, agility, and competitive advantage in the realm of financial services.
Future Trends and Predictions
The section on Future Trends and Predictions in the article delves deeply into the upcoming shifts in the financial services industry driven by machine learning technologies. Analyzing the trajectory of these trends is crucial to understanding the direction in which the sector is headed. By focusing on potential advancements and innovations, we can better prepare for the evolving landscape of financial services. Predicting these trends allows stakeholders to anticipate challenges, seize opportunities, and adapt strategies for optimal outcomes.
Hyper-Personalization in Financial Services
Tailored services based on individual preferences
The importance of hyper-personalization, particularly tailored services based on individual preferences, cannot be understated in the financial sector. This level of customization allows financial institutions to cater to the unique needs and preferences of each customer, enhancing customer satisfaction and loyalty. By leveraging machine learning algorithms to analyze customer data, personalized services can be offered, leading to a more engaging and efficient customer experience. Tailored services based on individual preferences stand out for their ability to create a personalized touchpoint with clients, fostering long-term relationships and improving overall service quality.
Predictive financial advice
Amidst the dynamic financial markets, predictive financial advice plays a pivotal role in guiding investment decisions and financial planning. By utilizing predictive analytics powered by machine learning, financial institutions can forecast market movements, identify trends, and offer personalized advice to clients. This predictive approach enables proactive decision-making, reduces investment risks, and enhances financial outcomes for individuals. The unique feature of predictive financial advice lies in its ability to adapt to changing market conditions and provide real-time insights, offering clients a competitive edge in their financial endeavors.
AI-Powered Regulatory Compliance
Automated reporting and compliance checks
Within the realm of regulatory compliance, the integration of AI-powered solutions like automated reporting and compliance checks streamlines processes and ensures adherence to legal requirements. These tools facilitate rapid data analysis, identify potential compliance issues, and generate timely reports, enhancing operational efficiency and reducing the likelihood of regulatory violations. The key characteristic of automated reporting and compliance checks lies in their ability to automate monotonous tasks, increase accuracy, and mitigate compliance risks efficiently. While advantages include saving time and resources, disadvantages may involve initial setup costs and potential system complexities.
Enhanced risk assessment models
Enhanced risk assessment models, underpinned by AI technologies, empower financial institutions to assess risks with precision and agility. By leveraging complex algorithms and vast datasets, these models can identify potential risks, vulnerabilities, and opportunities proactively. The key characteristic of enhanced risk assessment models is their ability to provide in-depth risk analysis, enabling swift decision-making and tailored risk mitigation strategies. While their accuracy and speed offer significant advantages in risk management, potential disadvantages may include challenges in interpreting complex model outputs and ensuring the models' ongoing relevancy.
The Rise of Explainable AI
Ensuring transparency and accountability
The rise of explainable AI addresses the inherent opacity of traditional machine learning models, emphasizing the importance of transparency and accountability in decision-making processes. By elucidating how AI arrives at specific outcomes, stakeholders can better comprehend and trust the technology's recommendations. Ensuring transparency and accountability enhances stakeholder confidence, fosters ethical AI practices, and promotes responsible utilization of AI in financial settings. The unique feature of explainable AI lies in its ability to demystify complex algorithms, allowing for clearer interpretation and validation of model outputs. Although transparency and accountability offer considerable benefits in decision-making processes, potential disadvantages may arise in balancing model complexity with interpretability.