Harnessing AI in Marketing: Insights and Strategies
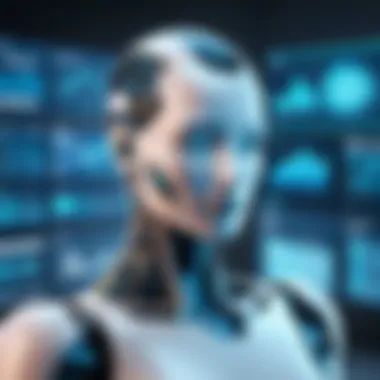
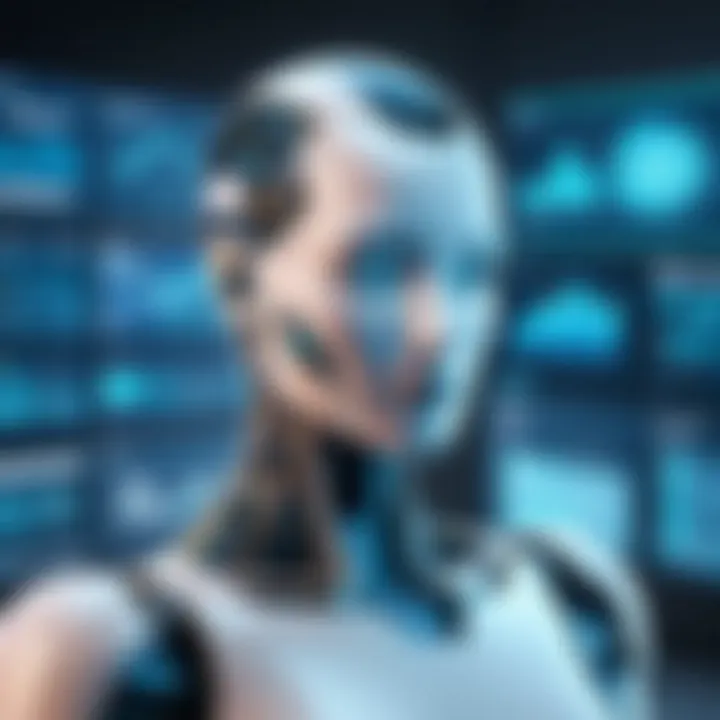
Intro
In today’s rapidly evolving digital landscape, machine learning plays a pivotal role in transforming marketing strategies. Businesses are often overwhelmed by the sheer volume of data generated through consumer interactions and marketplace dynamics. This is where artificial intelligence (AI) comes in as a game changer. By utilizing advanced machine learning algorithms, companies can better understand their customers' preferences, improve engagement, and optimize their marketing efforts.
With machine learning, marketers can analyze vast amounts of data in real time. This analysis helps businesses tailor their messaging, forecast trends, and personalize experiences. There are numerous applications, from automating customer service responses to predicting consumer behavior. Industry professionals must grasp these concepts, as they are crucial in staying competitive in a saturated market.
Furthermore, the benefits of integrating AI in marketing extend to operational efficiencies, ultimately leading to enhanced performance and growth. However, leveraging these technologies requires not only understanding their advantages but also recognizing the accompanying challenges, which are significant.
Next, we will explore the current trends in technology and their implications for the marketing field.
Understanding Machine Learning
Machine learning plays a crucial role in modern marketing frameworks. As businesses pivot towards data-driven strategies, understanding the nuances of machine learning becomes essential. It allows marketers to parse vast datasets and derive actionable insights. The ability to cater to individual preferences is now more pronounced and achievable through machine learning techniques. This section aims to clarify the foundational concepts to provide a springboard for deeper discussions throughout this article.
Definition of Machine Learning
Machine learning can be defined as a subset of artificial intelligence that enables systems to learn from data. Instead of explicitly programming machines to carry out tasks, machine learning gives them the ability to improve their performance based on experience. This method relies heavily on algorithms that analyze patterns in data. It becomes increasingly valuable in marketing, where insights drawn from consumer data can improve targeting and engagement strategies.
Types of Machine Learning Algorithms
Understanding the different algorithms is key to leveraging machine learning effectively. Each has its unique applications depending on the marketing goals.
Supervised Learning
Supervised learning refers to the approach of training algorithms on labeled datasets. A significant aspect of supervised learning is its efficiency in making predictions based on input data. For marketers, this means utilizing historical data to forecast customer behaviors or outcomes. This method's key characteristic is that it learns from examples, making it popular among those seeking accuracy. However, it requires a substantial amount of labeled data, which could be a limiting factor in some instances.
Unsupervised Learning
Unsupervised learning does not rely on labeled data. In this method, algorithms attempt to identify patterns and relationships within data without explicit labels. This can be particularly useful in marketing for segmenting customers or identifying trends. The key characteristic is its exploratory nature, allowing marketers to uncover insights that were previously unknown. However, the challenge lies in interpreting the results since there is no predefined output to compare against.
Reinforcement Learning
Reinforcement learning is a different approach where algorithms learn by interacting with their environment. It makes decisions based on a system of rewards and penalties. In marketing, this could mean optimizing ad placements or pricing strategies over time. One of its distinctive features is that it can adapt in real-time. However, the complexity of establishing a rewarding system could complicate implementations, making it less accessible for some marketers.
"Machine learning transforms how marketers engage with their audience, leading to more personalized and effective strategies."
Understanding these three types of machine learning algorithms allows businesses to select the most appropriate methods for their specific marketing needs. Each one carries distinct advantages and disadvantages, which will be explored further in subsequent sections.
The Role of Machine Learning in Marketing
Machine learning is redefining marketing strategies in profound ways. It empowers marketers to analyze vast amounts of data, glean insights about consumer behavior, and significantly increases the effectiveness of campaigns. Companies are using machine learning to adapt to rapidly changing market conditions. As a result, those who implement such technologies hold a competitive advantage.
Consumer Behavior Analysis
Understanding consumer behavior is essential for crafting effective marketing plans. Machine learning provides tools for analyzing data from various sources, such as social media, website interactions, and purchase history.
For instance, algorithms can identify patterns in consumer preferences and habits. This reveals what products are popular and when consumers are likely to buy. This insight informs marketing strategies and helps in shaping promotions tailored to specific customer needs.
Personalization and Recommendation Systems
Personalization is a critical aspect of modern marketing. Machine learning enhances personalization efforts through advanced recommendation systems. By leveraging historical customer data, these systems suggest products and services that align with individual preferences.
For example, Netflix utilizes machine learning to recommend shows based on viewing history. Likewise, Amazon analyzes previous purchases to suggest items. This tailored approach keeps customers engaged and often increases sales. It demonstrates the significant role of machine learning in facilitating a personalized shopping experience.
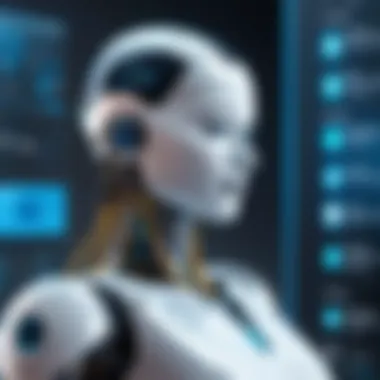
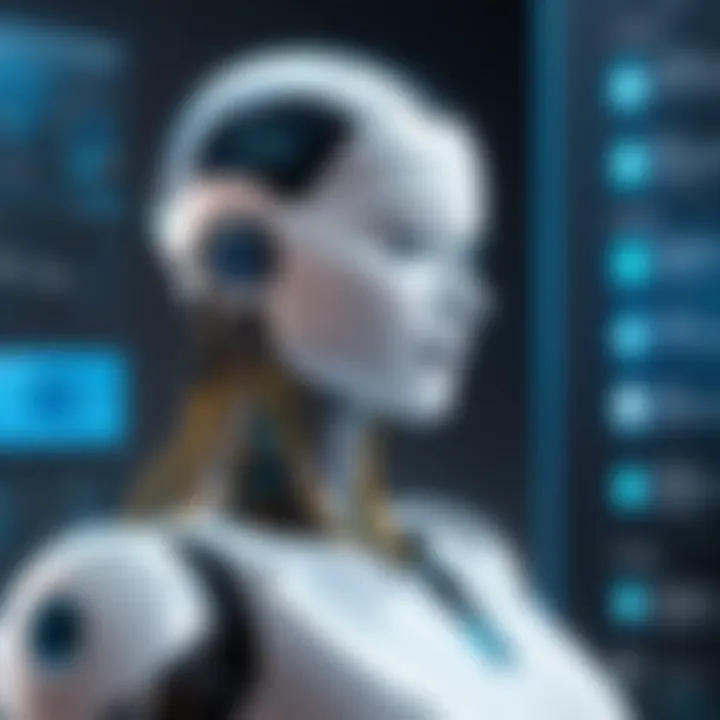
Predictive Analytics in Marketing Strategies
Predictive analytics employs machine learning algorithms to forecast future consumer behavior. By analyzing past trends, marketers can predict how consumers may respond to future campaigns.
For instance, companies can anticipate demand for products during specific seasons. This allows for better inventory management and targeted advertising. By using predictive insights, businesses can adjust their strategies proactively, thus enhancing overall effectiveness.
"Machine learning enables marketers to shift from reactive to proactive strategies, adapting to consumer needs in real-time."
In closing, the role of machine learning in marketing is both expansive and essential. It enables deeper insights into consumer behavior, fosters personalized experiences, and enhances predictive capabilities. These factors contribute to more effective marketing strategies and better customer interactions.
Key Applications of Machine Learning in Marketing
The significance of machine learning applications in marketing cannot be overstated. As businesses embrace digital transformation, understanding how to leverage data effectively is critical. Machine learning enables marketers to make data-driven decisions that enhance their strategies. The implementation of key applications like targeted advertising, customer segmentation, and sentiment analysis allows for refined strategies that not only meet consumer needs but also drive sales and engagement. These applications help organizations adapt to fast-changing market conditions, ultimately providing notable competitive advantages.
Targeted Advertising
Targeted advertising is a cornerstone of modern marketing, facilitated extensively by machine learning. The ability to analyze large datasets allows marketers to create ads tailored specifically to user preferences and behaviors. By utilizing algorithms, companies can predict which products or services may interest a specific consumer based on past interactions. This level of personalization leads to higher conversion rates as potential customers are presented with relevant content.
Moreover, machine learning can identify patterns in consumer data that human marketers might overlook. For instance, when companies like Google and Facebook utilize sophisticated ad systems, they rely on machine learning to optimize ad placements in real-time. This not only maximizes ad spend but also enhances the customer experience by reducing irrelevant advertisements.
Customer Segmentation
Effective customer segmentation is essential for addressing diverse consumer needs. Machine learning enables businesses to segment their audience more efficiently than traditional methods. By analyzing various attributes, such as demographics, purchase history, and online behavior, companies can identify distinct customer groups that require different marketing approaches.
The result is a more targeted strategy that resonates with individual segments. Companies like Amazon effectively use customer segmentation to personalize their shopping experiences. For instance, they analyze previous purchases and browsing history to recommend products, significantly boosting engagement and retention rates.
When executed correctly, segmentation can lead to dramatically improved marketing ROI. This success hinges on a deep understanding of data and the ability to interpret it accurately, a hallmark of machine learning capabilities.
Sentiment Analysis
Sentiment analysis is another powerful application of machine learning in marketing. This technology allows businesses to gauge consumer opinions, emotions, and perceptions regarding brands and products through various digital channels. Using natural language processing, machine learning algorithms can analyze customer reviews, social media posts, and feedback to determine the prevailing sentiments.
Understanding consumer sentiment enables companies to tailor their marketing strategies effectively. Brands can respond proactively to negative sentiments, addressing customer concerns or enhancing communication strategies. Furthermore, positive sentiments can be leveraged in marketing campaigns, showcasing customer testimonials or engaging influencers who reflect consumer desires.
Sentiment analysis not only aids in crafting targeted messaging but also helps in building stronger customer relationships by showing that brands itend to listen and adapt according to the feedback received.
By tapping into these key applications of machine learning, marketing professionals can not only enhance their operational efficiency but also create meaningful connections with their audience.
Benefits of Implementing Machine Learning in Marketing
The integration of machine learning within marketing strategies provides numerous advantages that can significantly enhance business operations. Understanding these benefits allows companies to harness the full potential of data-driven techniques. The focus here is on refined decision-making, increased operational efficiency, and enriched customer experiences. Each of these aspects plays a crucial role in shaping modern marketing practices.
Enhanced Decision-Making
Machine learning enables data-driven decision-making, which is fundamental for successful marketing strategies. By analyzing vast amounts of data, machine learning algorithms can identify patterns and trends that may not be evident through traditional analysis. Businesses can utilize these insights to make informed decisions on marketing expenditures, campaign effectiveness, and customer preferences.
- Predictive Models: Machine learning predicts future outcomes based on historical data. For instance, understanding which products are likely to sell well in upcoming seasons allows businesses to allocate resources more effectively.
- A/B Testing: Enhanced decision-making processes are supported by machine learning’s ability to analyze multiple variations of marketing assets, helping marketers determine which versions resonate best with their audience.
- Real-time Data Analysis: Businesses are now able to respond to customer behavior in real time by using machine learning tools. This immediacy facilitates agile strategies that can be adjusted quickly based on the latest data.
Increased Efficiency and Cost Reduction
One of the standout advantages of machine learning in marketing is its potential to streamline operations and reduce costs. Automated tasks can free up valuable human resources.
- Automation of Repetitive Tasks: Many tasks, such as data entry and segmentation, can be automated. This leaves marketing teams to focus on more strategic initiatives.
- Resource Optimization: Machine learning helps in allocating budget efficiently by targeting the right audiences with tailored messages. Businesses can reduce wastage and improve return on investment.
- Enhanced Performance Tracking: With machine learning, performance metrics can be monitored continuously. Companies can adjust their strategies based on real-time performance data to ensure maximum efficiency.
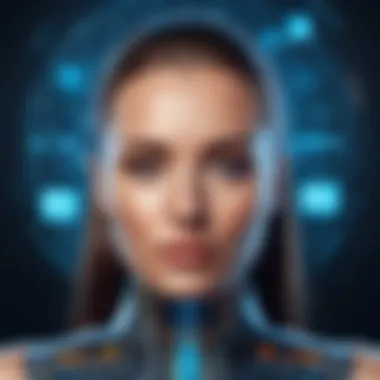
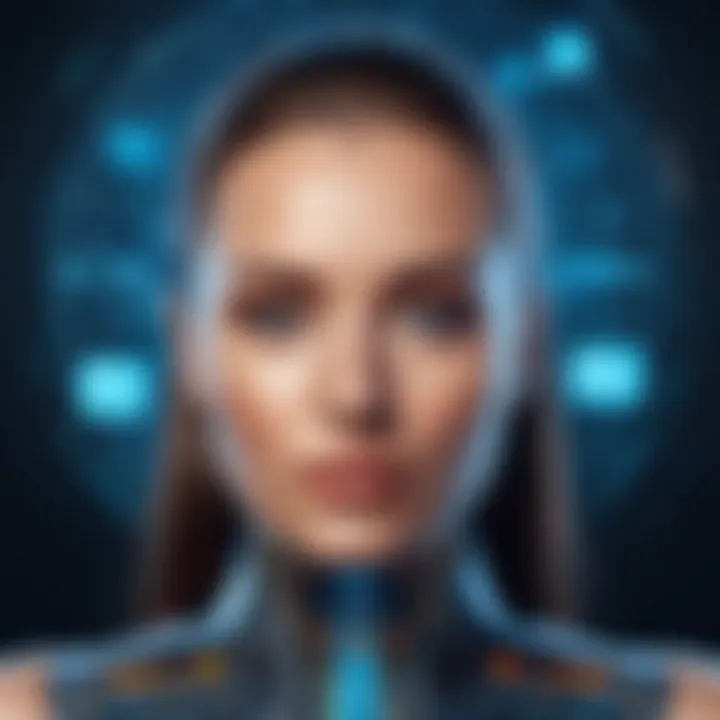
Improved Customer Experience
Machine learning enhances the customer journey by delivering personalized experiences that cater to individual preferences. Engaging with customers in a meaningful way is essential for retention and loyalty.
- Personalization: Machine learning algorithms can analyze customer behavior to deliver tailored content and product recommendations, creating a unique shopping experience for each user.
- Customer Support: Incorporating chatbots powered by machine learning into customer service can ensure fast responses to queries, leading to higher customer satisfaction.
- Understanding Customer Needs: By processing feedback and behavior patterns, machine learning helps brands anticipate customer needs, leading to improved product and service offerings.
"Machine learning is not just a tool, but an essential partner in creating a more strategic, responsive, and effective marketing landscape."
In summary, the implementation of machine learning in marketing provides profound benefits. Enhanced decision-making, increased efficiency, and improved customer experiences collectively contribute to a more effective marketing strategy. Companies embracing these technologies are likely to gain a significant competitive edge.
Challenges in Machine Learning Deployment for Marketing
Incorporating machine learning into marketing strategies is not without its challenges. Organizations must navigate a landscape filled with complexities, from data governance to integration issues. Recognizing these challenges is essential for businesses aiming to leverage machine learning effectively. Addressing these hurdles not only enhances deployment strategies but also safeguards investments and maximizes returns.
Data Privacy and Security Concerns
One of the foremost challenges in the deployment of machine learning in marketing revolves around data privacy and security. As organizations collect vast amounts of consumer data, ensuring that this information is handled responsibly becomes imperative. Regulations like the General Data Protection Regulation (GDPR) emphasize the need for compliance, making companies accountable for how they store and use personal data. Failure to adhere to these guidelines can lead to severe penalties and loss of consumer trust.
Additionally, brands must implement robust security measures to defend against data breaches. The sensitive nature of consumer information makes it a prime target for malicious attacks. Thus, businesses need to invest in advanced cybersecurity solutions and develop clear protocols to protect data assets effectively.
Integration with Existing Systems
The successful deployment of machine learning in marketing requires seamless integration with existing systems. Many organizations rely on various legacy systems that may not be compatible with newer technologies. The difficulty in merging old and new systems can lead to inefficiencies, increased costs, and, ultimately, a failure to realize the expected benefits of machine learning.
Companies must take a methodical approach to assess their current technological landscape and identify gaps. Engaging with IT professionals to create a streamlined integration plan can alleviate many of these challenges. The objective is to ensure that machine learning tools can coexist with existing software, facilitating consistent data flow and operational efficiency.
Skill Gap and Resource Allocation
Another significant challenge lies in the skill gap prevalent in many marketing departments. Successful machine learning implementation requires a blend of data science expertise and marketing acumen. Unfortunately, not all teams are equipped with professionals who possess the necessary skills to navigate complex machine learning frameworks.
Organizations often face resource allocation issues when allocating budget and time to upskill employees or hiring new talent. Prioritizing training and development in machine learning must be a top goal to ensure staff are capable and confident in utilizing AI and its applications in their daily tasks. Failure to invest in employee skill development can lead to underutilization of valuable technologies.
"Innovations like machine learning can provide a competitive edge, but tackling the challenges head-on is crucial for success."
Future Trends of Machine Learning in Marketing
As the dynamic landscape of marketing continues to evolve, the infusion of machine learning (ML) transforms strategies and reshapes interactions with consumers. The future trends of machine learning in marketing exhibit profound implications, driving efficiencies and enhancing customer experiences.
Evolution of AI Technologies
The rapid evolution of AI technologies is a significant factor in the marketing arena. Algorithms improve each year, becoming more sophisticated and capable of processing vast amounts of data. With advances like natural language processing and computer vision, marketers can engage more effectively with consumers.
Technologies such as Google's TensorFlow and Facebook’s PyTorch are widely adopted, leveraging neural networks to allow for deeper insights into customer preferences. Marketers can now utilize these tools for everything from automated content creation to improved ad targeting, thus optimizing campaigns in real time.
Increasing Importance of Real-Time Analytics
Real-time analytics emerges as a game-changer in marketing strategies. Businesses can now access and analyze data instantaneously, facilitating agile decision-making. This immediacy enables marketers to respond quickly to market trends and consumer behavior.
The capabilities of platforms like Tableau and Google Analytics offer valuable insights into customer interactions. This is critical in campaigns where timing is key. For instance, companies can adjust promotions or content dynamically based on the insights derived from customers’ online behaviors, enhancing engagement levels.
"Real-time analytics allows businesses to tailor customer experiences instantly, creating a more personalized interaction that can lead to higher retention and conversion rates."
Ethical Considerations in AI-Driven Marketing
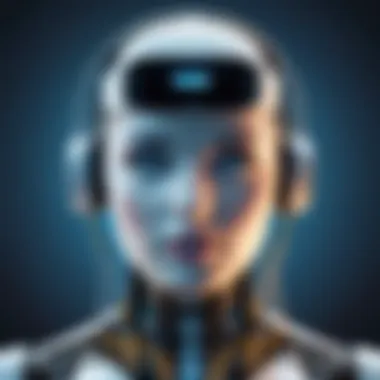
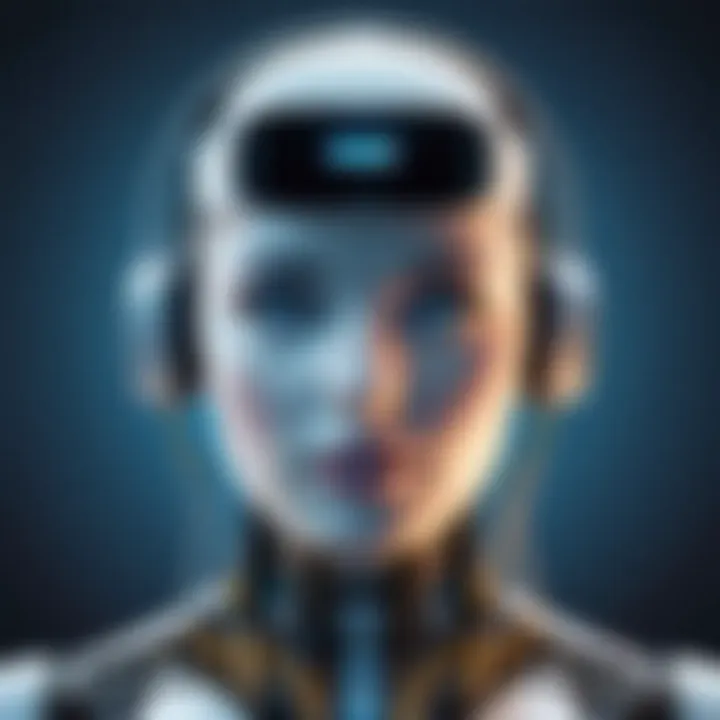
With the rise of machine learning in marketing, ethical considerations must be at the forefront of strategy development. Privacy concerns about how data is collected and used remain vital. Consumers are increasingly wary of how their personal information is leveraged, necessitating a transparency-first approach.
Marketers need to establish trust with consumers. Clear policies on data use, along with consent-based marketing practices, are essential. Companies utilizing AI must align with ethical standards and regulations, ensuring that consumer rights are respected. This not only enhances brand reputation but also fosters long-term customer loyalty.
Embracing these future trends in machine learning will not only elevate marketing efforts but also establish a framework that prioritizes ethical standards and consumer trust.
Case Studies of Successful Machine Learning Implementation
Machine learning has found numerous applications in marketing, showcasing its capabilities and providing valuable insights on effective strategies. By examining case studies of successful implementation, we can understand how different companies have leveraged this technology. Such analysis highlights the practical benefits and challenges associated with machine learning, while providing a blueprint for others in the industry.
E-commerce and Retail Applications
In the e-commerce sector, companies like Amazon and Zalando exemplify how machine learning can enhance user experience and drive conversions. These businesses utilize predictive analytics to tailor recommendations based on user behavior. Through analyzing data points such as previous purchases, browsing habits, and even customer reviews, these platforms present tailored suggestions that resonate with individual shoppers.
Benefits of these applications include:
- Increased sales through personalized recommendations.
- Enhanced customer satisfaction due to relevant suggestions.
- Improved inventory management based on predicted demand trends.
"The ability to predict what a customer is likely to purchase can significantly reduce cart abandonment rates."
Social Media Marketing Examples
Social media has seen transformative changes due to machine learning, particularly in platforms like Facebook and Instagram. These platforms employ machine learning algorithms to optimize ad targeting. By analyzing user interactions, preferences, and engagement history, they refine ad placements to reach the most relevant audience.
Key takeaways include:
- Higher engagement rates through targeted advertisements.
- Effective budget allocation with focused marketing efforts.
- Enhanced understanding of consumer preferences.
Travel and Hospitality Sector Innovations
In the travel and hospitality industry, companies such as Airbnb and Booking.com utilize machine learning to provide personalized experiences. These platforms analyze vast amounts of data to predict pricing trends and offer tailored travel suggestions based on user preferences. This results not only in increased bookings but also enhances overall customer experience.
Highlights of their strategies involve:
- Dynamic pricing models that reflect real-time market changes.
- Enhanced user interfaces that guide potential customers.
- Tailored travel recommendations that increase conversion rates.
How to Get Started with Machine Learning in Marketing
Understanding how to embark on the journey of integrating machine learning into marketing strategies is crucial. As businesses aim to remain competitive, leveraging machine learning can lead to improved insights and targeted campaigns. However, getting started requires meticulous planning and alignment with business objectives.
Identifying Business Objectives
Before implementing machine learning, it is essential to determine the specific goals of the marketing strategy. Ask what the business hopes to achieve. This clarity can drive a focused approach. Common objectives may include enhancing customer engagement, driving sales conversion, or optimizing campaign performance.
- Specific Goals: Set clear and measurable targets. For instance, a company could aim to improve customer retention by 15% within a year.
- Relevance to Marketing: Ensure the objectives relate directly to marketing efforts. For example, improving lead scoring systems helps prioritize potential customers effectively.
- Resource Allocation: Identify resources needed to achieve these goals, such as budget or personnel.
Choosing the Right Tools and Technologies
With objectives in place, the next step involves selecting appropriate tools and technologies. The market offers various platforms for machine learning applications. Picking the right tool is vital for achieving desired outcomes.
- Software Options: Tools like TensorFlow, IBM Watson, or Google Cloud AI can be good choices depending on the complexity and scale of the project.
- Suitability: Assess the compatibility of tools with existing systems. Ensure the selected tools integrate well with current marketing technology stacks.
- Ease of Use: Consider user-friendliness. Platforms that require lesser coding knowledge may be beneficial for teams without deep technical expertise.
Monitoring and Measuring Success
After implementation, it is imperative to monitor performance and measure success. This phase allows businesses to evaluate the effectiveness of their machine learning initiatives.
- Key Performance Indicators (KPIs): Establish relevant KPIs to track progress. Metrics might include engagement rates, conversion rates, and return on investment (ROI).
- Data Collection: Use analytics tools to gather data continuously. Analyzing data over time provides insights into trends and areas for improvement.
- Feedback Loops: Implement feedback mechanisms. Regular assessments can help refine strategies based on ongoing performance, ensuring adjustments can be made as needed.
The journey into machine learning for marketing is not simply about technology; it is about a strategic alignment that empowers data-driven decision-making.
In summary, starting with machine learning in marketing requires a clear identification of business objectives, careful selection of tools and technologies, and a structured approach to monitoring success. This structured process helps organizations maximize their potential and achieve strategic goals.