Unlocking Opportunities in Spatial Data Science Careers
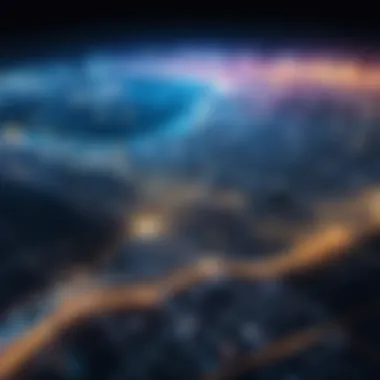
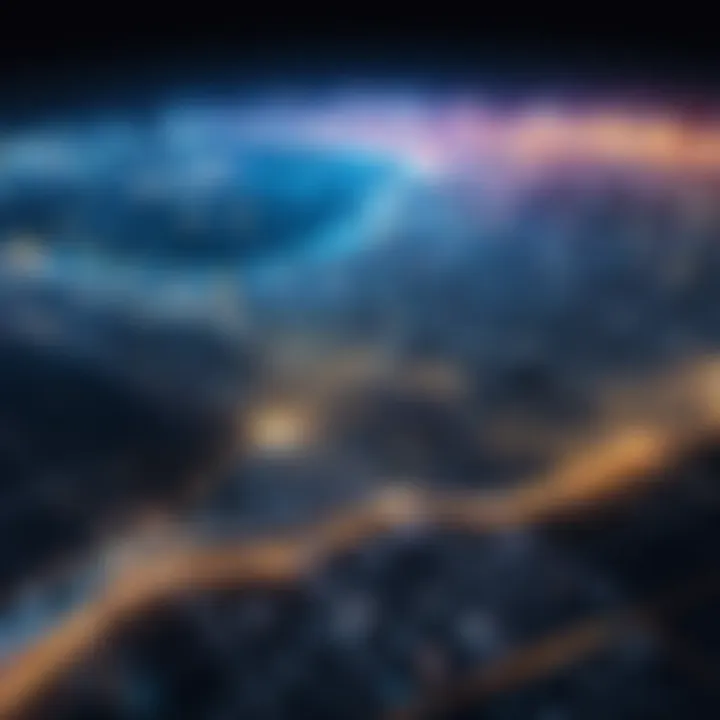
Tech Trend Analysis
The landscape of spatial data science jobs is evolving rapidly in response to technological advancements and shifting market demands. Currently, there is a notable trend towards incorporating machine learning and artificial intelligence into spatial data analysis processes. This integration of cutting-edge technologies opens up new possibilities for optimizing data interpretation and decision-making.
Exploring this trend sheds light on the significant implications for consumers. With the enhanced capabilities provided by machine learning algorithms in spatial data analysis, consumers can expect more accurate predictions and tailored services. This trend empowers businesses to deliver personalized experiences based on precise spatial insights derived from large datasets.
Looking ahead, future predictions point towards increased automation in spatial data science jobs. As machine learning algorithms continue to improve efficiency and accuracy, there is a likelihood of a surge in demand for professionals well-versed in both spatial data analytics and emerging technologies. The possibilities are vast, with the potential for revolutionizing various industries through data-driven spatial solutions.
Product Reviews
While not directly applicable to spatial data science jobs, engaging in product reviews within the realm of spatial data analysis could involve assessing the latest software tools and platforms designed for spatial data analysis integration. Evaluating features, specifications, performance, pros, and cons of these tools helps professionals make informed decisions about which technology aligns best with their unique spatial data science requirements.
In a detailed product review, professionals can gain insights into the efficiency, usability, and compatibility of spatial data analysis tools with their existing workflows. By providing a comprehensive analysis of the user experience, ease of integration, scalability, and customer support, product reviews enable practitioners to select the most suitable solutions for optimizing spatial data analysis processes.
Recommendations based on thorough product reviews offer valuable guidance to professionals seeking to enhance their spatial data science capabilities. By highlighting the strengths and weaknesses of different tools and platforms, these reviews assist individuals in selecting the most appropriate technologies to streamline their data analysis workflows.
How-To Guides
Introduction to the topic:
- Spatial data science plays a crucial role in leveraging geographical information to extract actionable insights for decision-making. Utilized across various industries such as urban planning, transportation, agriculture, and environmental science, spatial data analysis involves handling geographic data through specialized software tools.
Step-by-step instructions:
- Define the objective: Begin by outlining the specific goal or problem that requires spatial data analysis. Whether it's optimizing resource allocation or identifying spatial patterns, clarifying the objective is essential for a focused analysis.
- Data collection and preprocessing: Gather relevant spatial datasets from reliable sources and ensure data quality through cleaning and preprocessing. Removing inconsistencies and errors improves the accuracy of subsequent analysis.
- Select appropriate tools: Choose the right software tools, such as Geographic Information System (GIS) software or spatial data analysis libraries, based on the complexity of the analysis and the types of geographic data involved.
- Implement analysis techniques: Apply spatial statistics, geostatistics, and machine learning algorithms to extract meaningful patterns and relationships from the data. Utilize visualization techniques to represent spatial insights effectively.
Tips and tricks:
- Stay updated on the latest advancements in spatial data science to leverage new techniques and technologies for enhanced analysis.
- Collaborate with professionals from diverse backgrounds to gain interdisciplinary perspectives on spatial data analysis challenges.
- Document your analysis process and results comprehensively to ensure reproducibility and facilitate knowledge sharing with peers.
Troubleshooting:
- In case of unexpected results or analysis errors, revisit the data preprocessing stage to identify and rectify any inconsistencies. Verify the accuracy of input data and parameter settings to troubleshoot analysis issues effectively.
Industry Updates
Recent developments in the tech industry:
The tech industry is witnessing rapid advancements in spatial data science, driven by the increasing demand for location-based insights across sectors. Innovations in data visualization, augmented reality, and geospatial technologies are reshaping the landscape of spatial data analysis, offering new avenues for data exploration and interpretation.
Analysis of market trends:
Market trends indicate a growing reliance on spatial data analysis for informed decision-making in industries such as retail, logistics, real estate, and healthcare. The integration of location intelligence into business operations enhances strategic planning, customer engagement, and operational efficiency, leading to competitive advantages for organizations.
Impact on businesses and consumers:

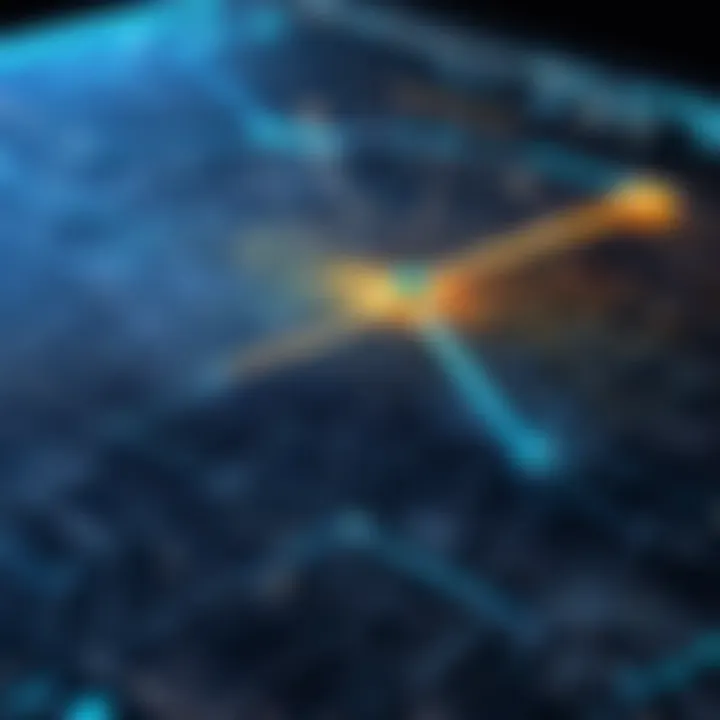
Businesses adopting spatial data analysis methodologies experience improved resource allocation, optimized logistics, targeted marketing campaigns, and enhanced customer experiences. Consumers benefit from personalized services, location-based recommendations, and seamless navigation experiences, facilitated by spatial data science applications.
Introduction
Spatial data science is a rapidly evolving field that plays a pivotal role in various sectors. From enhancing urban planning to driving decision-making processes, spatial data science is at the forefront of modern innovation. This article delves deep into the intricacies of spatial data science jobs, shedding light on the importance of this dynamic field and providing valuable insights for both tech enthusiasts and industry professionals looking to explore career opportunities in this realm.
Defining Spatial Data Science
Interdisciplinary Field
Spatial data science is a complex interdisciplinary field that combines elements of geography, data science, and technology. Its holistic approach to analyzing spatial data sets it apart as a comprehensive and unique discipline. The fusion of analytics, visualization, and problem-solving within spatial data science presents a powerful tool for exploring and understanding spatial relationships in various contexts. While the interdisciplinary nature of spatial data science offers a broad perspective, it may also pose challenges in terms of specialized skill requirements and diverse knowledge domains.
Application in Various Sectors
Spatial data science finds application across diverse sectors such as urban planning, environmental conservation, disaster management, and logistics. Its ability to leverage geospatial data for informed decision-making processes is instrumental in optimizing resource allocation and enhancing operational efficiency. By integrating spatial analysis into different fields, spatial data science enhances data-driven strategies and facilitates informed solutions. However, the versatility of spatial data science also necessitates continuous learning and adaptation to different industry standards and requirements.
Significance of Spatial Data Science Jobs
Driving Force in Decision-Making
Spatial data science serves as a driving force in decision-making processes by providing actionable insights derived from geospatial data analysis. It enables professionals to make informed choices based on spatial patterns, trends, and correlations. The ability of spatial data science to unravel complex spatial relationships empowers organizations to streamline processes, optimize workflows, and enhance overall efficiency. Nevertheless, the reliance on spatial data for critical decisions also requires a meticulous approach to data collection, analysis, and interpretation.
Impact on Urban Planning
In urban planning, spatial data science has a profound impact on creating sustainable and livable environments. By analyzing spatial data related to demographics, land use, and infrastructure, urban planners can develop cohesive strategies for urban development. The integration of spatial analysis tools in urban planning processes fosters evidence-based decisions and community-centric design solutions. Yet, the use of spatial data in urban planning raises questions related to data privacy, surveillance, and equitable access to resources and services.
Target Audience
Tech Enthusiasts
Industry Professionals
Industry professionals from diverse sectors recognize the value of spatial data science in optimizing operations and driving strategic decision-making. For professionals in fields such as real estate, transportation, and environmental management, spatial data science offers a competitive edge through enhanced spatial insights and predictive modeling. By incorporating spatial analysis techniques into their professional toolbox, industry professionals can uncover hidden patterns, identify spatial trends, and unlock innovative solutions. Nonetheless, the integration of spatial data science in professional contexts demands collaboration with data scientists, geospatial experts, and technology specialists.
Essential Skills
In the realm of spatial data science jobs, having essential skills is critical. These skills are the foundation that professionals in this field rely on to excel. Technical proficiency, analytical skills, and communication skills are vital components that shape a successful spatial data science career. Each of these skill sets plays a unique role in empowering individuals to navigate complex spatial data landscapes efficiently.
Technical Proficiency
GIS Software Knowledge
Addressing GIS software knowledge is a crucial aspect of spatial data science. This proficiency equips individuals with the ability to analyze geospatial data effectively. Understanding how to utilize Geographic Information System (GIS) software contributes significantly to decision-making processes in various industries. GIS software knowledge allows professionals to visualize, interpret, and present spatial data with precision. Its seamless integration with mapping technologies enhances data accuracy and aids in making informed decisions.
Programming Skills
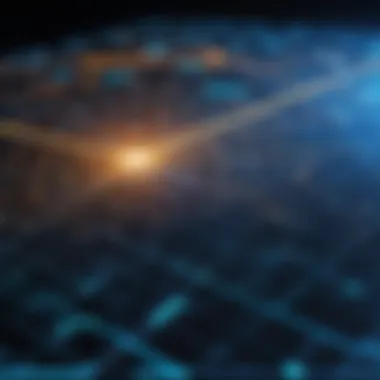
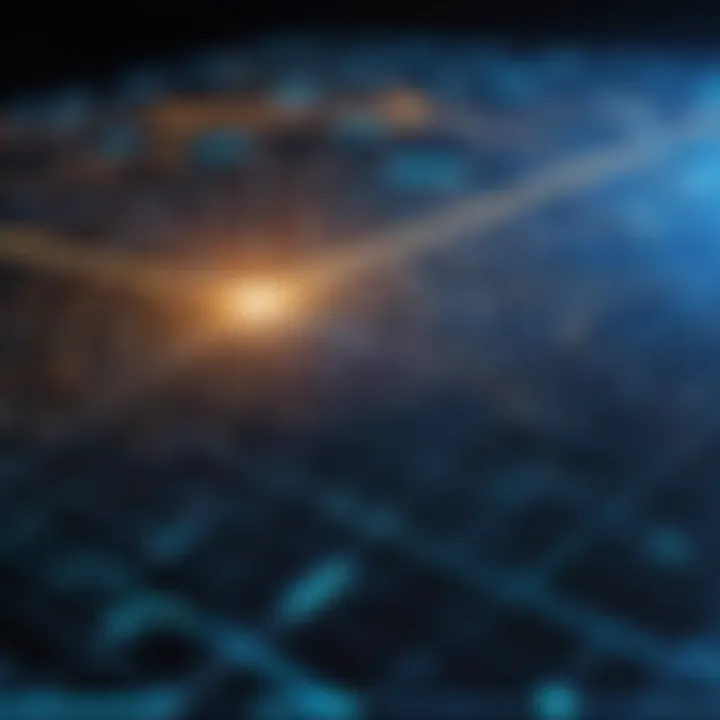
In the context of spatial data science, programming skills are indispensable. Professionals proficient in programming can develop robust algorithms for spatial analysis, streamline data processes, and create custom solutions tailored to specific spatial data needs. The utilization of programming languages like Python, R, or Java Script enables individuals to automate tasks, conduct advanced spatial modeling, and enhance data visualization capabilities. Programming skills pave the way for innovation and optimization in spatial data workflows.
Analytical Skills
Critical Thinking
Critical thinking is a fundamental skill in spatial data science that promotes logical reasoning and informed decision-making. Professionals with strong critical thinking abilities can evaluate complex spatial data sets, identify patterns, and draw meaningful conclusions. Critical thinking enhances the analysis of spatial relationships, leading to insightful interpretations and actionable recommendations. This skill fosters a deeper understanding of spatial phenomena and aids in addressing real-world spatial challenges effectively.
Problem-Solving Abilities
Effective problem-solving abilities are essential in spatial data science roles. Professionals proficient in problem-solving can tackle spatial data issues strategically, identify innovative solutions, and troubleshoot complex problems efficiently. The capacity to address spatial data discrepancies, optimize workflows, and implement creative interventions sets skilled spatial data practitioners apart. Problem-solving skills contribute to enhancing spatial data outcomes and driving continuous improvement in spatial data analysis processes.
Communication Skills
Collaboration
Collaboration is a cornerstone of success in spatial data science endeavors. The ability to collaborate effectively fosters teamwork, promotes knowledge sharing, and encourages multidisciplinary approaches to spatial data challenges. Professionals who excel in collaboration can leverage diverse perspectives, co-create solutions, and achieve collective goals within spatial data projects. Collaboration enhances synergy in spatial data teams, elevating the quality of outcomes and fostering a culture of innovation and cooperation.
Data Visualization
Data visualization plays a pivotal role in spatial data science by translating complex information into accessible insights. Professionals skilled in data visualization can represent spatial data trends, patterns, and relationships graphically, enhancing data comprehension and facilitating decision-making processes. Visual representations of spatial data enable stakeholders to grasp complex concepts easily, communicate spatial insights effectively, and derive actionable intelligence from spatial data sets. Data visualization empowers spatial data practitioners to communicate findings persuasively and engage audiences with compelling visual narratives.
Career Pathways
In this section, we delve into the critical topic of career pathways within the sphere of spatial data science. Understanding career pathways is pivotal as it elucidates the various trajectories and options available for professionals in this field. By exploring the different avenues individuals can take, we provide insights into the potential growth and development opportunities within spatial data science careers. Whether it be working for government agencies, private sector enterprises, or non-profit organizations, comprehending career pathways empowers individuals to make informed decisions regarding their professional journey.
Government Agencies
Urban Planning Departments
Urban planning departments play a vital role in spatial data science by utilizing data to design and develop sustainable urban environments. With a focus on urban infrastructure, zoning regulations, and land use planning, urban planning departments leverage spatial data to make informed decisions for the betterment of cities. The key characteristic of urban planning departments lies in their ability to integrate data analytics with urban development, creating more liveable and efficient cities. Choosing a career in urban planning departments offers professionals the advantage of directly impacting urban landscapes through data-informed strategies, although challenges may arise in balancing socio-economic factors with urban design.
Environmental Protection Agencies
Environmental protection agencies are crucial in spatial data science as they use data to monitor and preserve natural ecosystems. By analyzing spatial data related to environmental factors, these agencies work towards mitigating environmental degradation and promoting sustainable practices. The standout feature of environmental protection agencies is their commitment to safeguarding the environment through data-driven approaches. Opting for a career in environmental protection agencies provides professionals with the benefit of contributing to environmental conservation efforts, though complexities may emerge in balancing economic interests with environmental concerns.
Private Sector
Tech Companies
Tech companies are instrumental in spatial data science by developing innovative technologies and solutions that leverage spatial data for various applications. With a focus on technological advancements, data analytics, and geospatial tools, tech companies push the boundaries of spatial data science capabilities. The key characteristic of tech companies is their ability to drive technological innovations in spatial data science, making them a popular choice for professionals seeking cutting-edge opportunities. Engaging in a career at tech companies offers professionals the advantage of working on groundbreaking projects that shape the future of spatial data science, although challenges may arise in staying ahead of rapid technological advancements.
Consulting Firms
Consulting firms play a vital role in spatial data science by providing expertise and guidance to businesses and organizations seeking to leverage spatial data effectively. By offering strategic advice, data analysis, and problem-solving solutions, consulting firms aid clients in maximizing the potential of spatial data for their operations. The unique feature of consulting firms lies in their ability to offer specialized services tailored to individual client needs, making them a beneficial choice for professionals looking to diversify their experience. Pursuing a career in consulting firms equips professionals with the advantage of working across various industries and projects, though challenges may arise in meeting diverse client expectations and delivering innovative solutions.
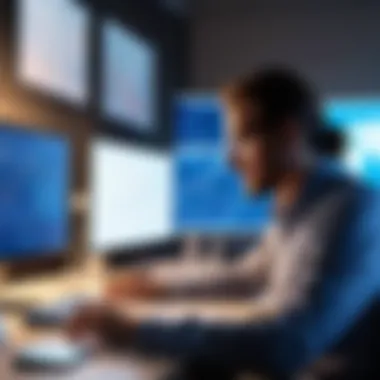
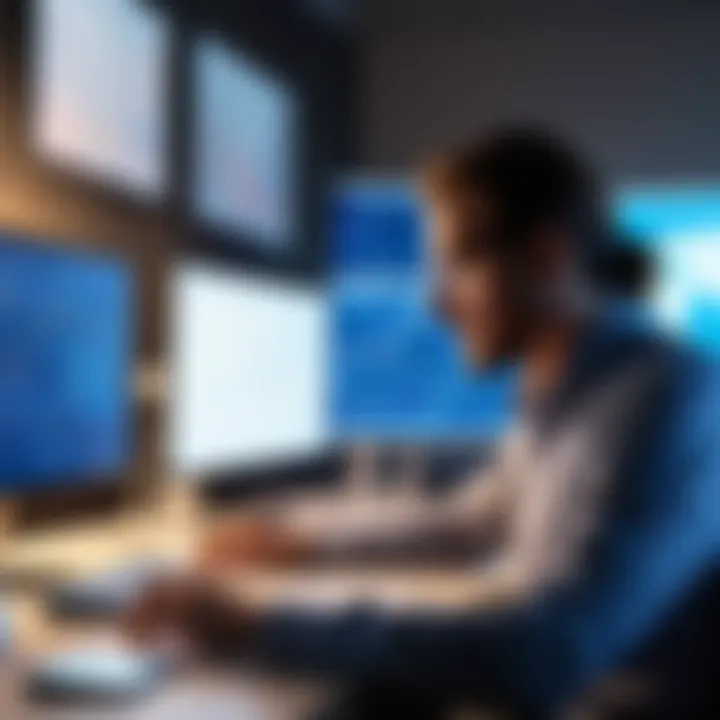
Non-Profit Organizations
Research Institutes
Research institutes play a crucial role in spatial data science by conducting in-depth research and studies on spatial data applications across different domains. With a focus on exploration, experimentation, and knowledge dissemination, research institutes contribute significantly to advancing spatial data science practices. The key characteristic of research institutes is their dedication to pushing the boundaries of spatial data science through rigorous research methodologies, making them a popular choice for professionals passionate about scientific inquiry. Engaging in a career at research institutes provides professionals with the advantage of delving deep into specialized research areas, though challenges may arise in securing funding for research projects and navigating academic hierarchies.
Humanitarian Aid Agencies
Humanitarian aid agencies play a crucial role in spatial data science by using data to address humanitarian crises and support vulnerable populations around the globe. By employing spatial data for disaster response, resource allocation, and needs assessment, humanitarian aid agencies work towards providing life-saving assistance in humanitarian emergencies. The unique feature of humanitarian aid agencies lies in their mission-driven approach to leveraging spatial data for humanitarian purposes, making them an invaluable choice for professionals dedicated to making a difference in the world. Choosing a career in humanitarian aid agencies offers professionals the advantage of working on impactful projects that have a direct positive impact on communities, although challenges may arise in working in high-stress environments and navigating complex geopolitical landscapes.
Salary Insights
In the realm of spatial data science jobs, one pivotal aspect that deserves keen attention is Salary Insights. Understanding the salary landscape is crucial for both aspiring professionals and seasoned experts in this field. By delving into Salary Insights, individuals can gain a clearer perspective on the financial rewards and incentives that come with pursuing a career in spatial data science.
Exploring average salaries provides a foundational understanding of the remuneration packages offered for entry-level positions in this dynamic industry. The knowledge of average salaries serves as a benchmark for professionals to gauge their earning potential and negotiate fair compensation. This information empowers individuals to make informed decisions about their career paths based on financial considerations.
Considering growth opportunities within the spatial data science sector is equally imperative. Examining the potential for career advancement and increased earning capabilities enhances the long-term sustainability and attractiveness of roles in this field. Growth opportunities not only signify upward mobility but also indicate the evolving nature of spatial data science jobs, presenting avenues for continuous learning and professional development.
Entry-Level Positions
Average Salaries
Average Salaries encompass a crucial aspect of entry-level positions in spatial data science job domains. The significance of average salaries lies in providing a baseline understanding of the financial rewards offered to professionals at the onset of their careers. Understanding average salaries helps individuals assess their worth in the job market, negotiate effectively, and make informed decisions regarding job offers. By delving deep into the intricacies of average salaries, aspiring professionals can set realistic financial goals and align their expectations with industry standards.
Growth Opportunities
Growth Opportunities in entry-level positions play a pivotal role in shaping the career trajectories of individuals venturing into spatial data science roles. These opportunities signify the potential for career advancement, skill enhancement, and increased responsibilities. By exploring growth avenues from the onset, professionals can strategically plan their career progression, identify areas for upskilling, and work towards achieving their long-term objectives. Embracing growth opportunities early on sets the foundation for a fulfilling and rewarding career journey in spatial data science.
Senior Roles
Management Positions
The realm of spatial data science offers various senior roles, including Management Positions that demand strategic vision, leadership capabilities, and decision-making expertise. Management Positions are instrumental in driving organizational success, overseeing project implementation, and ensuring efficient resource utilization. Professionals in these roles lead teams, steer projects towards successful outcomes, and contribute significantly to the growth and innovation within their organizations. By delving into the intricacies of Management Positions, individuals gain insights into the responsibilities, challenges, and rewards associated with senior leadership roles in spatial data science.
Industry Specializations
Industry Specializations within senior roles in spatial data science cater to the diverse needs of different sectors, including urban planning, environmental conservation, and technological innovation. Specializing in a particular industry vertical allows professionals to deepen their domain expertise, leverage industry-specific knowledge, and make tailored contributions that drive sectoral growth. By exploring industry specializations, experienced professionals can position themselves as subject matter experts, create impact within specific sectors, and align their career trajectories with their passion and interests. Embracing industry specializations opens avenues for targeted career growth and demonstrates a commitment to driving industry-specific innovations and advancements.
Future Prospects
In the vast landscape of spatial data science careers, understanding future prospects is crucial for professionals and enthusiasts alike. The evolution of technology continuously shapes the industry, making it imperative to grasp potential advancements and trends. By focusing on future prospects, individuals can align their skills and knowledge with emerging technologies, ensuring relevance and proficiency in this dynamic field. Future prospects not only provide a glimpse into upcoming opportunities but also offer valuable insights to strategize career growth. Recognizing the significance of adapting to technological advancements is fundamental in staying competitive in the ever-changing spatial data science domain.
Emerging Technologies
IoT Integration
When delving into emerging technologies within spatial data science, Io T integration stands out as a prominent aspect with far-reaching implications. IoT, or the Internet of Things, revolutionizes data collection and analysis by connecting devices and systems to streamline processes and enable real-time decision-making. The key characteristic of IoT integration lies in its ability to enhance data accuracy, efficiency, and automation, thereby optimizing spatial data science workflows. This technological innovation is a popular choice for its ability to facilitate data-driven insights and improve operational efficiencies, making it a valuable asset for professionals navigating the complexities of spatial data science jobs. Despite its advantages, challenges such as data security and privacy concerns require careful consideration when implementing IoT integration in spatial data science applications.
Machine Learning Applications
Within the context of spatial data science employment, machine learning applications offer a powerful tool for data analysis and predictive modeling. The essence of machine learning lies in its capability to analyze large datasets, identify patterns, and make informed predictions without explicit programming. This key characteristic empowers spatial data scientists to extract valuable insights from complex data sets, fostering informed decision-making and innovative solutions. The unique feature of machine learning applications is their adaptability, enabling continuous learning and optimization to enhance data analysis outcomes in spatial data science roles. While the advantages of machine learning applications are abundant, challenges like model interpretability and bias mitigation require attention to ensure ethical and accurate utilization within spatial data science applications.