Unlocking the Potential of Automated Machine Learning Solutions in Tech
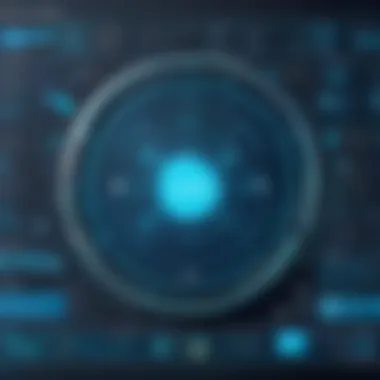
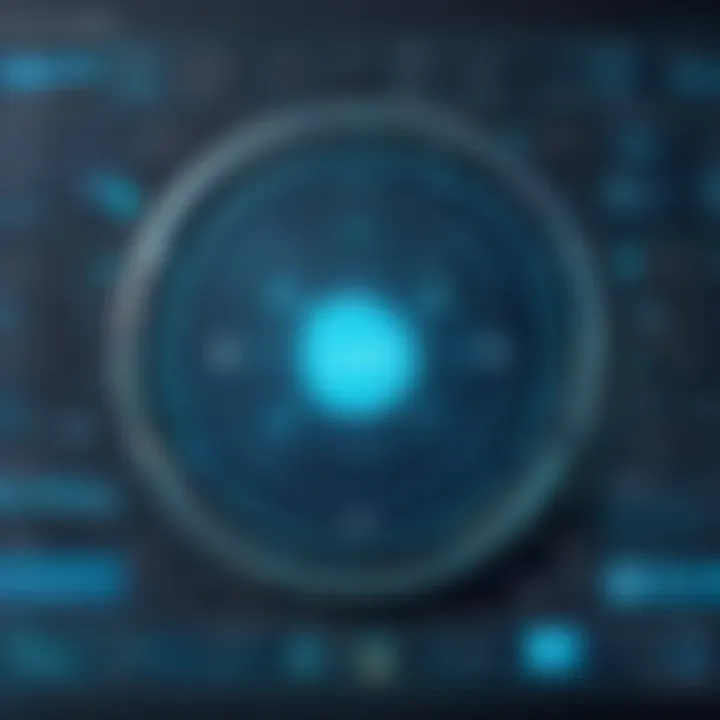
Tech Trend Analysis
Overview of the current trend
In the ever-evolving landscape of technology, automated machine learning tools have emerged as a pivotal trend. These tools signify a shift towards efficiency and innovation, streamlining complex processes within the tech industry. Their automated nature simplifies tasks, empowering both data scientists and businesses with advanced predictive modeling capabilities.
Implications for consumers
The advent of automated machine learning tools has profound implications for consumers. By harnessing the power of automation, these tools enhance user experiences, optimize decision-making processes, and drive operational excellence across various industries. Consumers benefit from more accurate predictions, personalized recommendations, and streamlined operations, leading to enhanced satisfaction and efficiency.
Future predictions and possibilities
Looking ahead, the future of automated machine learning tools appears promising and transformative. As technology continues to advance, these tools are expected to become even more sophisticated, catering to a wider range of applications and industries. From healthcare to finance, intelligent automation through machine learning is poised to revolutionize the way businesses operate, ultimately shaping the future of consumer experiences and industry standards.
How-To Guides
Intro to the topic
Embark on a journey into the realm of automated machine learning tools with this comprehensive how-to guide. Explore the fundamentals of these tools, understand their significance in the tech ecosystem, and uncover their potential for revolutionizing predictive modeling and data analysis processes.
Step-by-step instructions
Delve into the step-by-step instructions for harnessing the power of automated machine learning tools effectively. From setting up the tools to refining predictive models, this guide provides detailed insights into maximizing efficiency and accuracy in your machine learning endeavors.
Tips and tricks
Discover expert tips and tricks for optimizing your usage of automated machine learning tools. Learn how to fine-tune your models, interpret results effectively, and leverage the full potential of these tools to elevate your data science capabilities and drive meaningful insights.
Troubleshooting
Navigate common challenges and roadblocks encountered when working with automated machine learning tools. This troubleshooting guide equips you with practical solutions and best practices to overcome obstacles, ensuring a seamless and productive experience in your machine learning projects.
Industry Updates
Recent developments in the tech industry
Stay informed about the latest trends and advancements in the tech industry related to automated machine learning tools. Explore industry updates, groundbreaking innovations, and emerging technologies that are reshaping the landscape of data science and predictive analytics.
Analysis of market trends
Gain valuable insights into the market trends surrounding automated machine learning tools. Analyze market dynamics, competitive landscapes, and consumer behaviors to stay ahead of the curve and capitalize on new opportunities within the rapidly evolving tech sector.
Impact on businesses and consumers

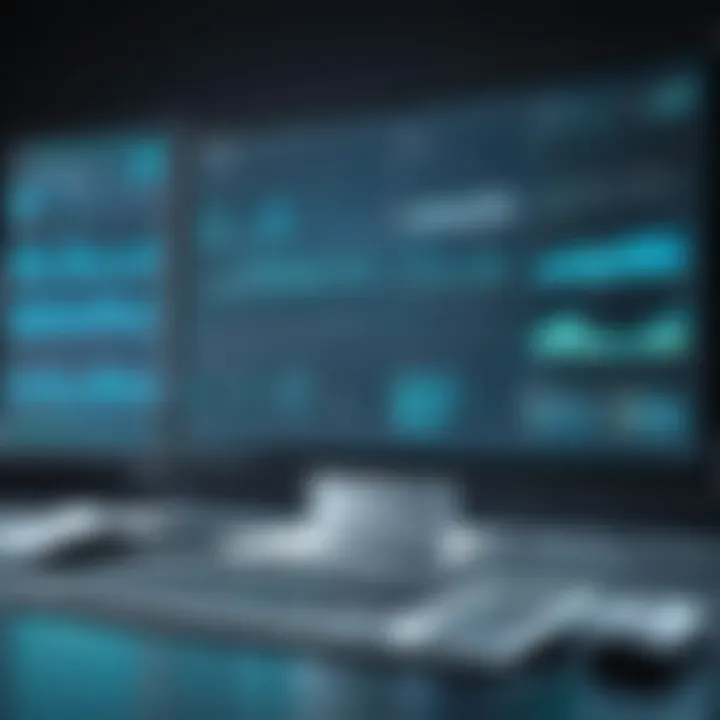
Understand the profound impact of automated machine learning tools on businesses and consumers alike. From driving operational efficiencies to enhancing customer experiences, these tools play a vital role in shaping the future of industries, fostering innovation, and facilitating growth and competitive advantage.
Intro to Automated Machine Learning Tools
Automated Machine Learning tools have emerged as a transformative force in the tech landscape, revolutionizing how data-driven tasks are approached. These tools streamline processes, enhance predictive modeling capabilities, and redefine the roles of data scientists and businesses in machine learning tasks. By automating repetitive tasks and optimizing model performance, Automated Machine Learning tools enable users to focus on strategic decision-making and insights generation. The significance of these tools extends beyond mere efficiency improvements; they represent a paradigm shift in how organizations leverage data for informed decision-making.
Understanding the Concept of Automated Machine Learning
Automated Machine Learning refers to the process of automating the design and implementation of machine learning models. This approach encompasses defining objectives, data preprocessing, algorithm selection, hyperparameter tuning, and model interpretation. At its core, Automated Machine Learning aims to democratize machine learning by making complex analytical techniques accessible to a broader audience. Its emphasis on efficiency and scalability makes it a popular choice for organizations striving to maximize their data analytics potential.
Definition and Fundamentals
The Definition and Fundamentals of Automated Machine Learning establish the groundwork for understanding the core principles driving this technology. By automating tedious tasks like data preprocessing and model selection, Automated Machine Learning accelerates model development and deployment. This characteristic is particularly beneficial for organizations looking to scale their machine learning operations without compromising accuracy or reliability. However, the reliance on automated algorithms raises concerns about the interpretability of models and potential biases introduced during automated decision-making processes.
Evolution in the Tech Landscape
The Evolution in the Tech Landscape surrounding Automated Machine Learning reflects the ongoing advancements and innovations in the field of artificial intelligence. As new algorithms and techniques emerge, Automated Machine Learning tools adapt to incorporate these developments, ensuring optimal performance and predictive accuracy. The key characteristic of this evolution lies in its ability to adapt to changing data landscapes, providing users with cutting-edge solutions to complex analytical challenges. While these advancements offer enhanced capabilities, they also pose challenges in terms of model complexity and transparency.
Benefits of Leveraging Automated Machine Learning
The benefits of leveraging Automated Machine Learning tools are multi-faceted, ranging from improved efficiency and productivity to empowering non-experts in machine learning. These tools empower users to expedite model development, reduce time-to-insight, and enhance decision-making processes through advanced predictive analytics.
Improved Efficiency and Productivity
Automated Machine Learning significantly enhances the efficiency and productivity of data scientists by automating time-consuming tasks such as data preprocessing and model optimization. This streamlining of processes translates into cost savings, quicker model deployment, and increased time for experimentation and innovation. However, the automated nature of these tools raises concerns about the lack of human oversight in critical decision points, potentially affecting model accuracy and robustness.
Empowering Non-Experts in Machine Learning
Automated Machine Learning democratizes the field of machine learning by providing non-experts with user-friendly tools to develop and deploy models without extensive technical knowledge. This democratization fosters innovation and diversity in machine learning applications, opening doors for cross-disciplinary collaboration and knowledge sharing. Nonetheless, the reliance on automated processes may lead to over-reliance on black-box models, hindering users' understanding of model outputs and limiting interpretability.
Challenges and Limitations
Despite its numerous benefits, Automated Machine Learning faces challenges and limitations that warrant careful consideration to mitigate risks and drawbacks. From ensuring model transparency to adapting to complex data structures, these challenges underscore the importance of ethical considerations and regulatory compliance in automated decision-making processes.
Ensuring Model Transparency
One of the critical challenges in Automated Machine Learning is ensuring model transparency, as automated algorithms may generate models that are difficult to interpret and explain. Transparent models are essential for building trust among stakeholders and ensuring compliance with regulatory frameworks. The unique feature of ensuring model transparency lies in the need for organizations to implement interpretability tools and methodologies to unravel the decision-making process behind automated models. However, this focus on transparency may come at the cost of sacrificing predictive performance or model complexity.
Adapting to Complex Data Structures
Automated Machine Learning tools often struggle to adapt to complex data structures, especially in cases where data quality is poor or features are highly correlated. Adapting to complex data structures requires a nuanced approach that balances model performance with data integrity. The unique feature of this challenge lies in the necessity to preprocess data effectively and design robust models that can generalize to diverse datasets. However, the automated nature of these tools may oversimplify complex data relationships, leading to suboptimal model outcomes.
Key Features and Functionality
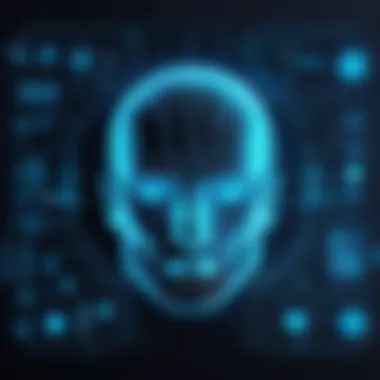
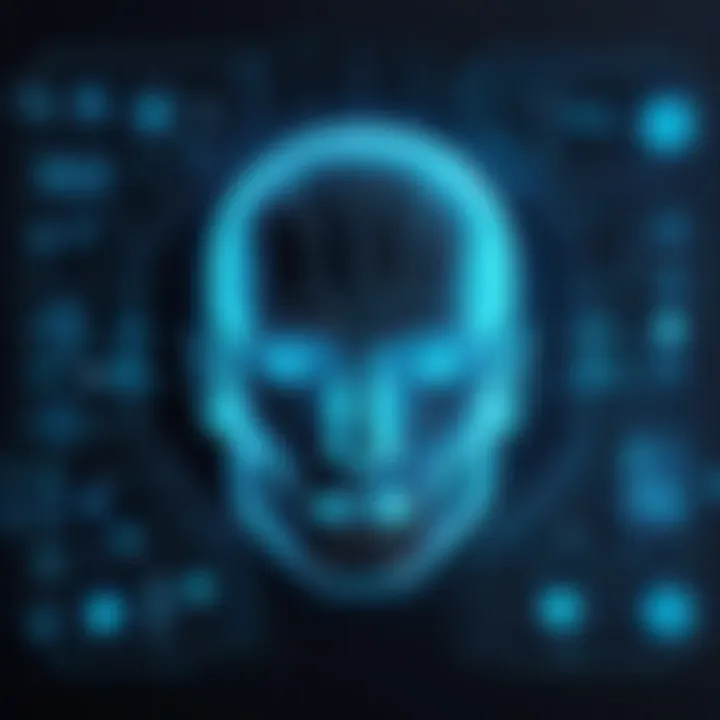
Automated machine learning tools are at the forefront of revolutionizing the tech industry with their significant impact on streamlining processes and enhancing predictive modeling capabilities. One crucial element in this article is understanding the key features and functionality of automated machine learning tools. By focusing on these specific elements, we can unravel the benefits and considerations that come with utilizing such tools in the realm of machine learning.
Automated Data Preprocessing
Handling Missing Values
The handling of missing values plays a pivotal role in the overall data preprocessing phase. Emphasizing the key characteristic of identifying and managing missing data, this aspect proves to be a popular choice in enhancing model accuracy and reliability. However, it is essential to note the unique feature of handling missing values can also pose challenges, such as potential biases or incorrect imputations. Despite these disadvantages, the strategic management of missing values remains crucial in ensuring the data's integrity and the model's performance in this article.
Encoding Categorical Variables
Another fundamental aspect in data preprocessing is the encoding of categorical variables. By highlighting the key characteristic of transforming categorical data into numerical format, this process becomes essential for machine learning algorithms to interpret the data accurately. The unique feature of encoding categorical variables lies in its ability to prevent biases introduced by categorical data representation. Nevertheless, this approach may also have limitations, such as potential information loss or increased dimensionality. Understanding the advantages and disadvantages of encoding categorical variables is vital in leveraging this technique effectively within this article.
Algorithm Selection and Hyperparameter Tuning
Optimizing Model Performance
Optimizing model performance is a critical aspect contributing to the overarching goal of predictive accuracy. By focusing on key characteristics like fine-tuning algorithm parameters and configurations, this optimization step becomes a popular choice for enhancing model efficiency. The unique feature of model performance optimization lies in its ability to adapt models to specific datasets, thereby improving generalization. However, challenges may arise, such as increased computational complexity or overfitting. Balancing these advantages and disadvantages is essential in optimizing model performance within the context of this article.
Enhancing Prediction Accuracy
Enhancing prediction accuracy is a crucial objective tied to the success of machine learning models. By highlighting key characteristics like selecting appropriate algorithms and tuning hyperparameters effectively, this aspect becomes beneficial in achieving reliable predictions. The unique feature of enhancing prediction accuracy rests in its potential to uncover intricate patterns within the data, leading to more precise forecasts. Nonetheless, pitfalls such as model overfitting or increased training times should be carefully considered. Acknowledging these pros and cons is essential in maximizing prediction accuracy within the scope of this article.
Model Interpretability and Explainability
Interpreting Model Decisions
Interpreting model decisions is vital in gaining insights into the reasoning behind algorithmic outputs. By emphasizing key characteristics like post-hoc analysis and decision attribution, this aspect becomes a popular choice for understanding model behavior. The unique feature of interpreting model decisions lies in its capacity to unveil complex relationships within the data, aiding in decision-making processes. Despite these advantages, challenges such as model complexity or interpretability limitations may arise. Striking a balance between these factors is crucial in enhancing model interpretability within this article.
Addressing Bias and Fairness
Addressing bias and fairness in machine learning models is a paramount consideration in ethical AI development. By highlighting key characteristics like fairness metrics and bias mitigation techniques, this aspect becomes essential in promoting equitable decision-making. The unique feature of addressing bias and fairness lies in its potential to rectify discriminatory outcomes and promote inclusivity. However, challenges such as algorithmic transparency or conflicting fairness criteria must be carefully navigated. Understanding these nuances is crucial in fostering unbiased and fair models within the framework of this article.
Applications in Various Industries
In the realm of automated machine learning, the applications in various industries play a pivotal role in transforming operational processes and enhancing decision-making capabilities. By leveraging automated machine learning tools, industries such as finance, banking, healthcare, biotechnology, e-commerce, and marketing witness a significant shift towards efficiency and innovation. These tools revolutionize tasks, streamline operations, and optimize outcomes, contributing extensively to the tech industry's evolution.
Finance and Banking Sector
- Risk Assessment: Within the finance and banking sector, automated machine learning tools offer a sophisticated approach to risk assessment. They efficiently analyze vast amounts of data to identify potential risks, allowing financial institutions to make informed decisions swiftly. The key characteristic of automated risk assessment lies in its ability to predict and mitigate financial hazards, ensuring robust risk management strategies. The unique feature of automated risk assessment is its real-time monitoring and adaptive nature, which provides continuous insights to optimize risk mitigation strategies.
- Fraud Detection: Automated machine learning tools are instrumental in fraud detection within the finance and banking sector. These tools can detect anomalous patterns in transactions, behavior, and account activities, aiding in the swift identification of fraudulent activities. The key characteristic of automated fraud detection is its proactive approach to detecting and preventing fraud, safeguarding financial assets and interests. The unique feature of automated fraud detection is its scalability and adaptability, allowing for the customization of fraud detection algorithms to suit specific business needs.
Healthcare and Biotechnology
- Patient Diagnosis: In healthcare and biotechnology, automated machine learning tools significantly contribute to accurate and timely patient diagnosis. These tools analyze patient data, medical records, and diagnostic tests to assist healthcare professionals in making informed diagnoses. The key characteristic of automated patient diagnosis is its ability to process vast amounts of data rapidly, leading to prompt and precise diagnostic outcomes. The unique feature of automated patient diagnosis is its integration of advanced algorithms that enhance diagnostic accuracy while minimizing errors.
- Drug Discovery: Automated machine learning tools streamline the process of drug discovery in healthcare and biotechnology. By analyzing molecular structures, chemical properties, and biological interactions, these tools expedite the identification of potential drug candidates. The key characteristic of automated drug discovery is its high-throughput screening capabilities, enabling researchers to explore a wide range of compounds efficiently. The unique feature of automated drug discovery is its predictive modeling, which accelerates the identification of novel drug targets and therapeutic interventions.
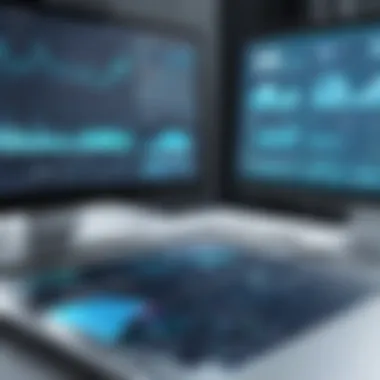
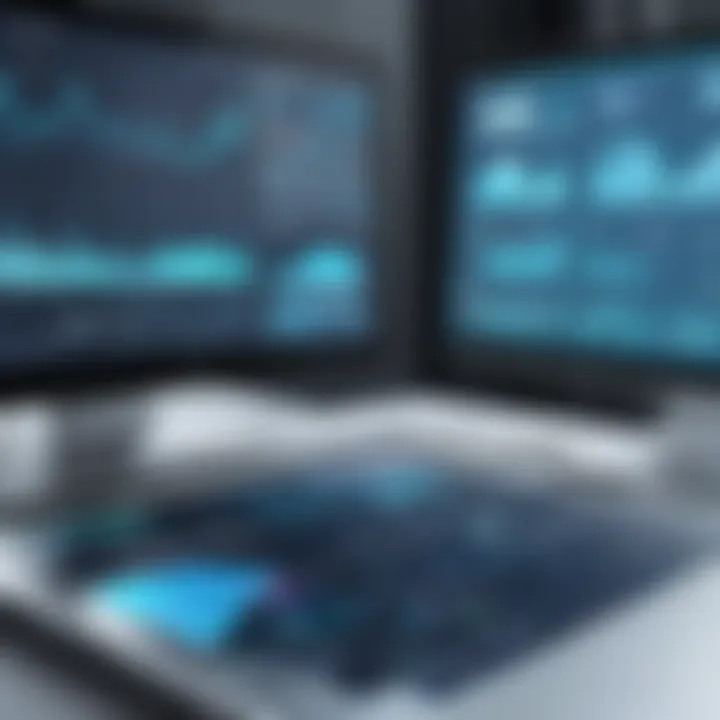
E-commerce and Marketing
- Personalized Recommendations: E-commerce and marketing benefit significantly from automated machine learning tools, particularly in providing personalized recommendations to customers. These tools analyze customer behavior, preferences, and purchase history to offer tailored product suggestions, enhancing the overall shopping experience. The key characteristic of personalized recommendations is their ability to drive customer engagement and retention by delivering relevant content. The unique feature of automated personalized recommendations is their adaptive algorithms, continually learning from user interactions to improve recommendation accuracy.
- Customer Segmentation: Automated machine learning tools play a crucial role in customer segmentation within e-commerce and marketing strategies. By categorizing customers based on demographics, behavior, and preferences, these tools enable personalized marketing campaigns and targeted messaging. The key characteristic of customer segmentation tools is their capacity to divide a vast customer base into distinct segments for tailored marketing approaches. The unique feature of automated customer segmentation is its dynamic segmentation criteria, allowing businesses to refine and adjust segmentations based on evolving market trends and customer preferences.
Future Trends and Innovations
In the vast landscape of automated machine learning tools, Future Trends and Innovations play a pivotal role in shaping the trajectory of technological advancement. As the tech industry continues to evolve rapidly, staying abreast of the latest trends is imperative for professionals and businesses alike. Future trends encompass a spectrum of developments, from cutting-edge algorithms to novel applications, driving the field towards unprecedented growth. Innovations in this realm not only optimize existing processes but also pave the way for revolutionary breakthroughs, setting the stage for a new era in machine learning.
Enhanced Automation and Integration
AI-Driven Feature Engineering
AI-driven feature engineering stands out as a fundamental aspect of automated machine learning, propelling models towards enhanced performance and accuracy. The intrinsic value of this approach lies in its ability to autonomously extract salient features from raw data, streamlining the feature selection process. By leveraging sophisticated algorithms and cognitive computing, AI-driven feature engineering empowers models to discern patterns and insights that may elude traditional methods.
One notable characteristic of AI-driven feature engineering is its adaptability to diverse datasets, ensuring robust performance across varying data structures. Its proficiency in identifying complex relationships within data sets elevates model efficiency, contributing to more precise predictions and insights. While its sophisticated nature enhances predictive capabilities, implementing AI-driven feature engineering necessitates a comprehensive understanding of algorithm intricacies and data nuances.
Continuous Learning Models
Continuous learning models represent a paradigm shift in machine learning, enabling systems to evolve and improve iteratively. Unlike conventional models that require periodic updates, continuous learning models possess the capacity to assimilate new information in real-time, refining their predictive prowess with each data input. This perpetual learning loop ensures that models adapt dynamically to changing environments, fostering adaptability and responsiveness.
The key strength of continuous learning models lies in their ability to mitigate obsolescence, as they remain primed to accommodate emerging trends and patterns seamlessly. By fostering a continuous feedback loop, these models refine their predictions iteratively, enhancing precision and reliability over time. Despite their advantages, integrating continuous learning models requires robust infrastructure and data management protocols to ensure seamless model recalibration.
Ethical Considerations and Regulatory Compliance
Ensuring Data Privacy
Ensuring data privacy stands as a cornerstone of ethical considerations in automated machine learning, necessitating stringent measures to safeguard sensitive information. The essence of data privacy lies in protecting individual rights and confidentiality, particularly in an age where data breaches and cyber threats abound. Implementing stringent data encryption, access controls, and anonymization techniques are imperative to uphold data privacy standards.
A key characteristic of ensuring data privacy is its role in fostering trust and integrity within data-driven ecosystems, instilling confidence among users regarding data handling practices. By prioritizing data privacy, organizations not only comply with regulatory mandates but also cultivate a culture of transparency and accountability, enhancing stakeholder trust and loyalty. However, the complexities of data privacy regulations necessitate ongoing compliance monitoring and adaptation to evolving privacy frameworks.
Mitigating Algorithmic Biases
Mitigating algorithmic biases emerges as a critical initiative in combating unfair discriminatory outcomes generated by machine learning algorithms. As algorithms exhibit predispositions based on training data biases, addressing algorithmic biases is paramount to ensure equitable decision-making processes. Leveraging bias detection tools, fairness metrics, and diverse training data sets can help mitigate biases and promote algorithmic equity.
A distinctive feature of mitigating algorithmic biases is its proactive approach toward rectifying historical biases entrenched in machine learning models. By identifying and rectifying biases at the model development stage, organizations can foster inclusivity and fairness in algorithmic outcomes, reflecting a commitment to ethical AI practices. However, achieving bias mitigation requires a nuanced understanding of algorithmic dynamics and continual monitoring to uphold fairness standards.
Collaborative Development and Open-source Initiatives
Community-driven Tools
Community-driven tools epitomize collaboration and knowledge sharing within the realm of automated machine learning, harnessing collective expertise and innovation. These tools rely on the collective efforts of diverse stakeholders, including developers, data scientists, and enthusiasts, to co-create and enhance machine learning solutions. The collaborative nature of community-driven tools fosters knowledge exchange, peer review, and iteration, culminating in robust and versatile toolsets.
A key characteristic of community-driven tools is their inclusivity, welcoming contributions from a global community of practitioners with varied skill sets and experiences. This collaborative ecosystem accelerates innovation, enabling rapid problem-solving and solution dissemination across diverse domains. While community-driven tools promote democratization of AI and foster learning opportunities, maintaining version control, documentation, and quality assurance is crucial for sustaining community engagement and tool longevity.
Knowledge Sharing Platforms
Knowledge sharing platforms serve as repositories of insights, best practices, and resources within the automated machine learning domain, facilitating continuous learning and skill development. These platforms aggregate expertise from industry thought leaders, researchers, and practitioners, curating a wealth of knowledge accessible to a broad audience. By enabling robust documentation, tutorials, and case studies, knowledge sharing platforms empower learners to navigate complex machine learning concepts effectively.
The key characteristic of knowledge sharing platforms is their role in democratizing education and fostering a culture of continuous learning in machine learning. Through interactive forums, webinars, and collaboration spaces, these platforms engender a sense of community and camaraderie among learners, nurturing a conducive environment for knowledge exchange and growth. While knowledge sharing platforms open avenues for skill enhancement and networking, maintaining curated content relevance and user engagement are paramount for platform sustainability and user retention.