Unveiling the Transformative Power of Open Data Science in Tech
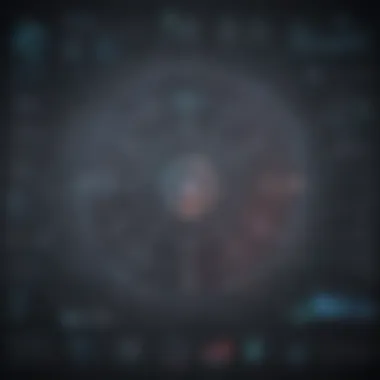
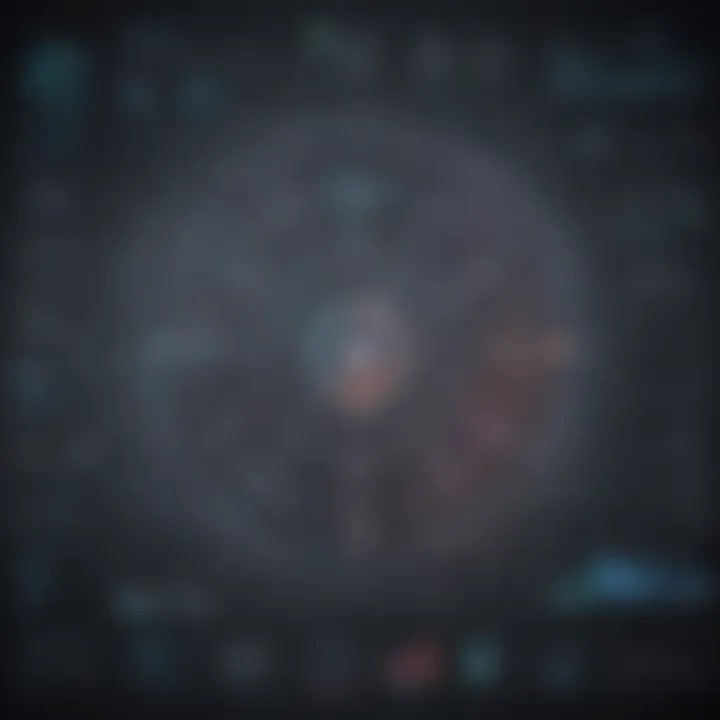
Tech Trend Analysis
When delving into the realm of Open Data Science in the Tech World, it becomes essential to analyze the current trends shaping this field. Understanding the evolution of technologies in data science provides invaluable insights for tech enthusiasts and industry professionals alike. By tracking the trajectory of open data science, one can anticipate its implications on consumer experiences and the innovative possibilities it offers. Looking ahead, discerning future predictions and exploring the potential advancements that open data science may bring to the tech industry becomes crucial to stay at the forefront of technological innovation.
Product Reviews
In the landscape of Open Data Science, product reviews play a pivotal role in guiding consumers and professionals towards making informed decisions. These reviews offer a comprehensive overview of the products available in the open data science domain, highlighting their features, specifications, and unique selling points. Through detailed performance analysis, individuals can gain a nuanced understanding of how these products fare in practical scenarios. Evaluating the pros and cons of each product ensures a well-rounded perspective, enabling readers to make sound judgments and informed choices. Finally, a well-considered recommendation based on the review findings aids in steering consumers and professionals towards selecting the most suitable open data science products for their needs.
How-To Guides
Introducing novices and experts alike to the intricacies of Open Data Science, How-To Guides serve as educational resources that offer step-by-step instructions for mastering various aspects of this field. From basic concepts to advanced techniques, these guides break down complex ideas into digestible chunks, facilitating a smoother learning curve for enthusiasts. Additionally, valuable tips and tricks shared in these guides enhance the learning experience, providing insights and strategies for optimizing one's practice in open data science. Troubleshooting sections further address common challenges faced by practitioners, offering practical solutions and workarounds to enhance efficiency and productivity.
Industry Updates
Keeping pace with the ever-evolving tech industry requires a keen eye on the latest developments and trends. Industry updates offer a holistic view of the current landscape, shedding light on new technologies, emerging market trends, and impactful innovations. Through in-depth analysis, professionals can discern the implications of these updates on businesses and consumers, foreseeing the potential disruptions or opportunities they may bring. By staying abreast of industry developments, individuals can strategically position themselves to leverage upcoming trends and advancements, ensuring they remain competitive and adaptive in the dynamic tech environment.
Introduction to Open Data Science
Open Data Science is a pivotal component within the tech world, embodying a revolutionary approach towards handling and leveraging data. Its essence lies in promoting transparency, collaboration, and innovation. By embracing Open Data Science, organizations can tap into a wealth of insights and opportunities that traditional data methodologies often overlook. This section will delve deep into the fundamental concepts that underpin Open Data Science, shedding light on its impact and relevance within the tech industry.
Defining Open Data Science
Conceptual Framework
A cornerstone of Open Data Science, the Conceptual Framework provides a structured outline for data analysis and interpretation. It acts as a guide, steering practitioners towards a comprehensive understanding of complex datasets. The adaptability and scalability of this Framework make it a popular choice, offering a systematic approach to deriving meaningful insights from raw data. Despite its advantages, the Conceptual Framework may face challenges in handling unstructured data, requiring additional tools for optimization.
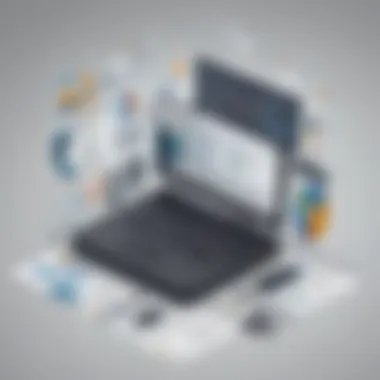
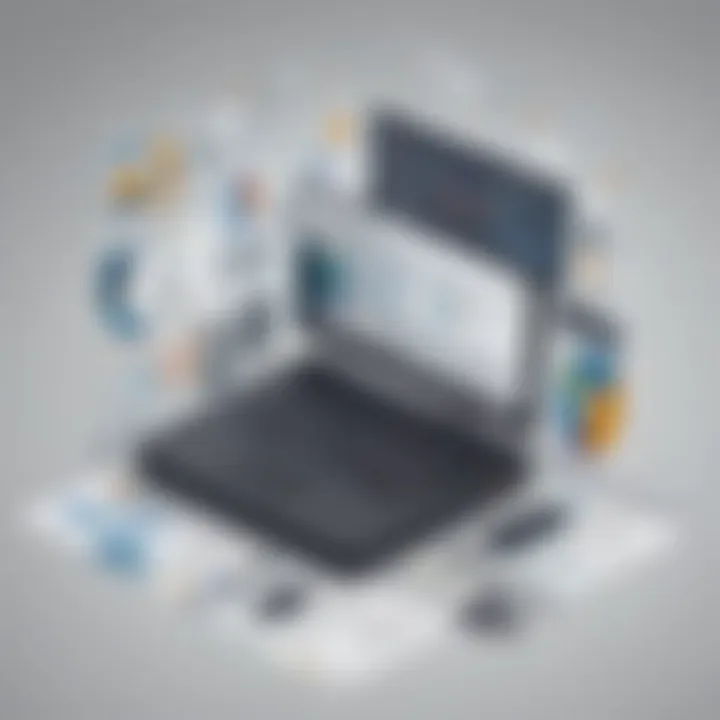
Key Principles
Integral to the foundation of Open Data Science, Key Principles serve as guiding philosophies that shape data practices. They reflect the ethical considerations, best practices, and theories that govern data processes. The resilience and responsiveness inherent in these Principles make them indispensable in navigating the evolving landscape of data science. While Key Principles prioritize data integrity and accuracy, there may be limitations in applying them universally across diverse datasets.
Evolution of Open Data Science
Historical Background
The historical roots of Open Data Science trace back to the early days of data processing and analysis. Its evolution mirrors the advancements in technology and the increasing demand for data-driven decision-making. Understanding the origins of Open Data Science provides valuable insights into its growth trajectory and long-standing relevance in modern tech environments. However, historical perspectives may sometimes restrict adaptive strategies in rapidly changing data landscapes.
Technological Advancements
Technological Advancements have been pivotal in shaping the landscape of Open Data Science. Innovations in data storage, processing speed, and algorithm development have propelled the field towards greater efficiency and accuracy. The integration of cutting-edge technologies like machine learning and artificial intelligence has revolutionized data analytics, opening new avenues for exploration. While these advancements enhance productivity and prediction capabilities, they also pose challenges in terms of data privacy and security.
Applications of Open Data Science
Open Data Science plays a fundamental role in revolutionizing the tech industry by enabling advanced data analysis and insightful visualizations. It serves as the backbone for driving innovation and decision-making within tech companies. The utilization of open data science enhances the efficiency of complex data processing tasks and facilitates the extraction of valuable insights from vast datasets. In this article, we delve deep into the critical aspects of data analysis and visualization, shedding light on predictive analytics and data interpretation.
Data Analysis and Visualization:
Predictive Analytics:
Predictive analytics, a pivotal component of data analysis, focuses on utilizing historical data to forecast future trends and behaviors. Its key characteristic lies in its ability to identify patterns and trends, aiding businesses in making informed decisions. Predictive analytics stands out as a popular choice within the tech world due to its predictive accuracy and the capability to optimize operations. However, one of its disadvantages is the challenge of interpreting complex algorithms for non-technical users. Despite this, its advantages in enhancing business strategies and forecasting outcomes make it an indispensable tool.
Data Interpretation:
Data interpretation involves extracting meaningful insights from data sets, enabling organizations to comprehend and act upon the information available. Its key characteristic lies in transforming raw data into actionable intelligence, guiding strategic business moves. Data interpretation is a beneficial choice for this article as it empowers readers to understand the significance of data-driven decision-making in the tech sector. While its unique feature lies in providing a narrative around data, one of the disadvantages is the potential for biased interpretations. Nevertheless, its advantages in facilitating informed decision-making and enhancing operational efficiency make it an essential component of open data science.
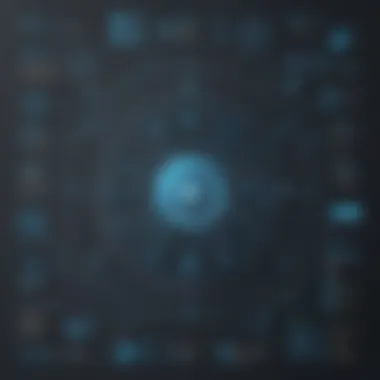
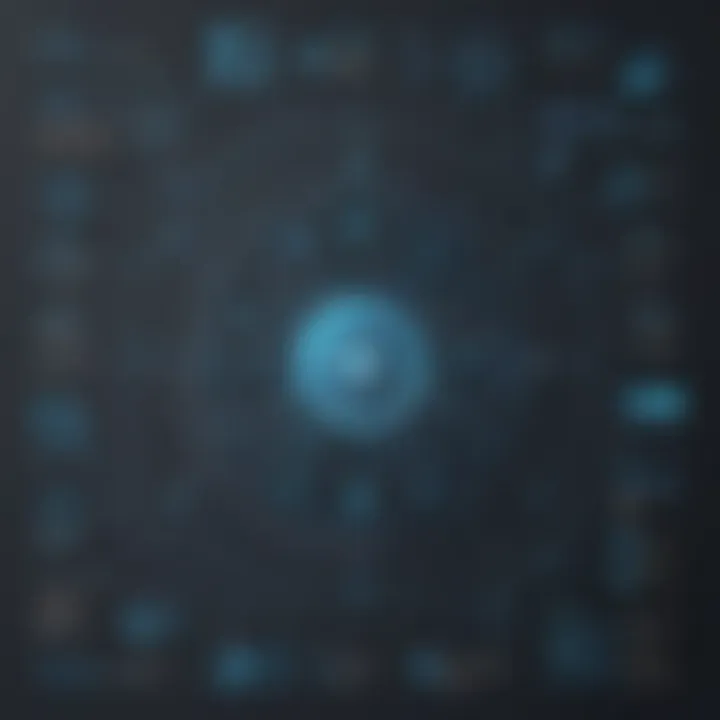
Machine Learning and AI:
Deep Learning Models:
Deep learning models form the backbone of artificial intelligence applications, enabling machines to learn complex patterns and make decisions independently. Their key characteristic lies in their ability to process vast amounts of data and recognize intricate patterns, revolutionizing various industries. Deep learning models are a popular choice for this article due to their unmatched accuracy and efficiency in handling unstructured data. Their unique feature lies in continuous learning and self-improvement, although limitations include the requirement of extensive computational resources. Nonetheless, the advantages of deep learning models in facilitating automation and enhancing predictive capabilities make them indispensable in open data science.
Natural Language Processing:
Natural Language Processing (NLP) focuses on enabling computers to understand, interpret, and generate human language. Its key characteristic lies in bridging the gap between human communication and machine understanding, enabling seamless interactions. NLP is a beneficial choice for this article as it showcases the transformative power of language in driving AI applications. The unique feature of NLP lies in sentiment analysis and language translation, with challenges including contextual nuances and language variations. Despite these challenges, the advantages of NLP in enhancing customer experiences and automating processes underscore its crucial role in open data science.
Impact of Open Data Science
Open Data Science plays a pivotal role in transforming the tech landscape, influencing innovation and collaboration across industries. The integration of open data methodologies empowers organizations to harness the power of data-driven decision-making, propelling them towards competitive advantages and sustainable growth. By embracing open data principles, businesses can unlock new possibilities for research, development, and problem-solving in the digital era.
Innovation in Tech Industry
Breakthrough Technologies
Breakthrough Technologies represent cutting-edge advancements that revolutionize the way technology functions, offering unprecedented capabilities and efficiencies. These innovations drive significant progress in various sectors by introducing novel solutions to complex problems. Their adaptability and scalability make them a preferred choice for enterprises seeking to stay ahead in the competitive tech landscape. Despite the benefits these technologies bring, they also pose challenges such as integration complexities and cybersecurity risks.
Collaborative Research
Collaborative Research fosters a culture of knowledge exchange and cooperation among industry peers, fueling collective intelligence and driving innovation. By pooling resources and expertise, organizations can tackle intricate technological challenges more effectively and expedite the development of groundbreaking solutions. The key characteristic of collaborative research lies in its ability to unite diverse perspectives and skill sets towards a common goal, optimizing research outcomes and enhancing industry standards. However, navigating collaborative research landscapes requires careful coordination and consensus-building to ensure equitable contributions and mutual benefits.
Data Privacy and Ethics
Transparency Issues
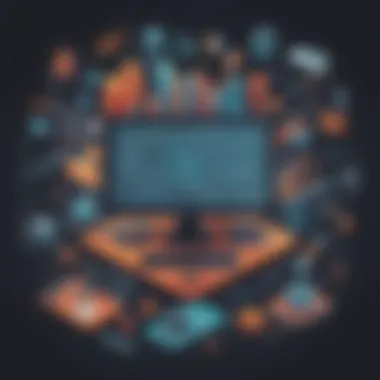
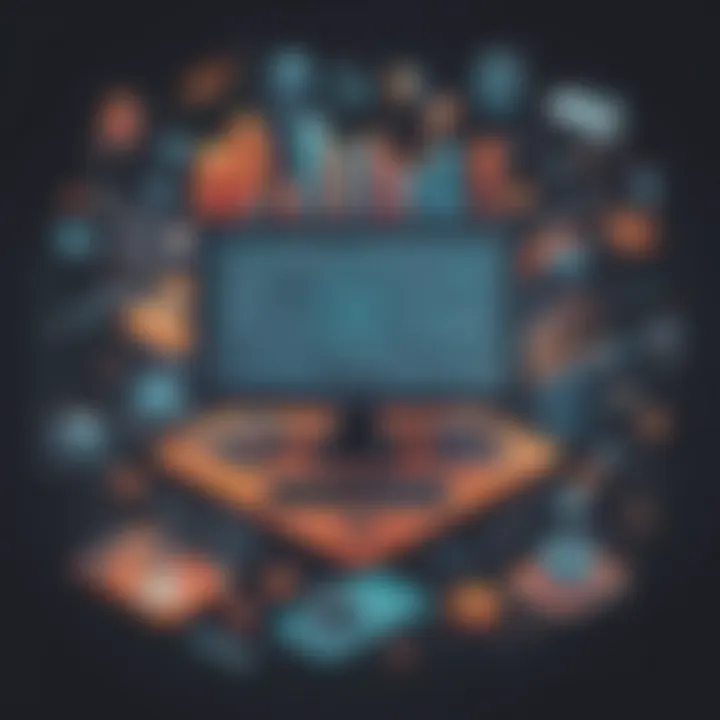
Transparency Issues underscore the critical need for organizations to maintain openness and accountability in their data practices. Addressing transparency concerns is essential for fostering trust among stakeholders and ensuring data integrity and reliability. By enhancing transparency, businesses can mitigate risks associated with data misuse and demonstrate a commitment to ethical data management. Despite its advantages, transparency initiatives may encounter challenges related to data security vulnerabilities and stakeholder resistance.
Regulatory Challenges
Regulatory Challenges encompass the legal and compliance barriers that organizations face when handling data, particularly in highly regulated industries. Navigating these challenges requires a deep understanding of legal frameworks and a proactive approach to compliance management. By adhering to regulatory requirements, businesses can protect sensitive information, mitigate legal risks, and uphold the highest standards of data governance. However, regulatory compliance efforts may strain operational resources and impede innovation if not managed efficiently.
Challenges and Future Trends
Open Data Science in the tech world delves into a realm filled with discoveries and challenges. Addressing the future trends in this field is crucial for shaping the trajectory of technology advancements. It is imperative to understand the complexities surrounding data security concerns and how they impact the open data science landscape. Unraveling the challenges and future trends is like navigating a maze of possibilities and potential pitfalls waiting to be mitigated for a brighter and secured technological future.
Data Security Concerns
In the realm of open data science, data security concerns are paramount. Cyber threats pose a significant risk to the data integrity and privacy of organizations. These threats encompass a myriad of malicious activities aiming to exploit vulnerabilities within systems. Understanding cyber threats is pivotal in fortifying defenses against potential breaches and data leaks. Implementing robust security measures is imperative to thwart cyber-attacks and safeguard sensitive information from falling into the wrong hands.
Cyber Threats
Discussing cyber threats unveils a cloak of uncertainty and vulnerability within the tech domain. The sophistication and evolving nature of cyber-attacks pose a formidable challenge to data security professionals. Cyber threats encompass a wide range of malicious activities such as phishing, malware, and ransomware. These threats exploit weaknesses in software systems and human errors to gain unauthorized access to confidential data. Being cognizant of the evolving landscape of cyber threats is essential to stay ahead of potential security breaches.
Privacy Risks
Privacy risks accentuate the importance of safeguarding personal and sensitive information in the digital age. With the proliferation of data collection and sharing practices, privacy risks have become a pervasive issue in the tech industry. Unauthorized access to personal data, data breaches, and inadequate data protection measures contribute to privacy risks. Mitigating privacy risks involves implementing stringent data protection policies, encryption protocols, and user consent mechanisms. Prioritizing data privacy is crucial in fostering trust and accountability among users and organizations.
Advancements in Data Governance
Data governance plays a pivotal role in ensuring the ethical and regulatory compliance of data practices within organizations. Advancements in data governance frameworks are instrumental in fostering transparency, accountability, and trust in data-driven decision-making processes. Understanding the intricacies of regulatory frameworks and compliance standards is essential for organizations to navigate the complex landscape of data governance effectively.
Regulatory Frameworks
Regulatory frameworks establish guidelines and best practices for organizations to adhere to legal requirements concerning data management and protection. Compliance with regulatory frameworks ensures that organizations operate within the boundaries of data privacy laws and regulations. Regulatory frameworks also promote standardization and harmonization of data practices across industries, enhancing interoperability and data exchange. Embracing regulatory frameworks is key to building a solid foundation for ethical data governance and fostering trust among stakeholders.
Compliance Standards
Compliance standards set forth the benchmarks and procedures for ensuring adherence to regulatory requirements and industry standards. Compliance standards encompass a wide array of guidelines related to data security, privacy, and accessibility. Implementing compliance standards within organizations enhances data integrity, reliability, and confidentiality. Adherence to compliance standards not only mitigates risks but also demonstrates a commitment to ethical data practices and regulatory compliance. Striving for compliance excellence is essential in upholding the integrity and credibility of organizations in today's data-centric environments.