Unlocking the Path to Entry-Level Data Science Jobs: A Comprehensive Guide
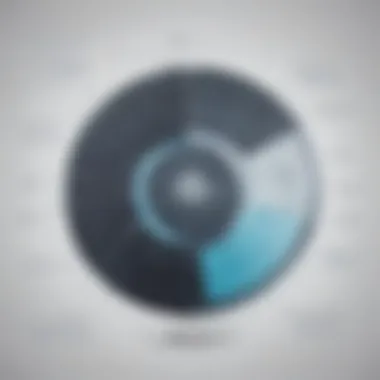
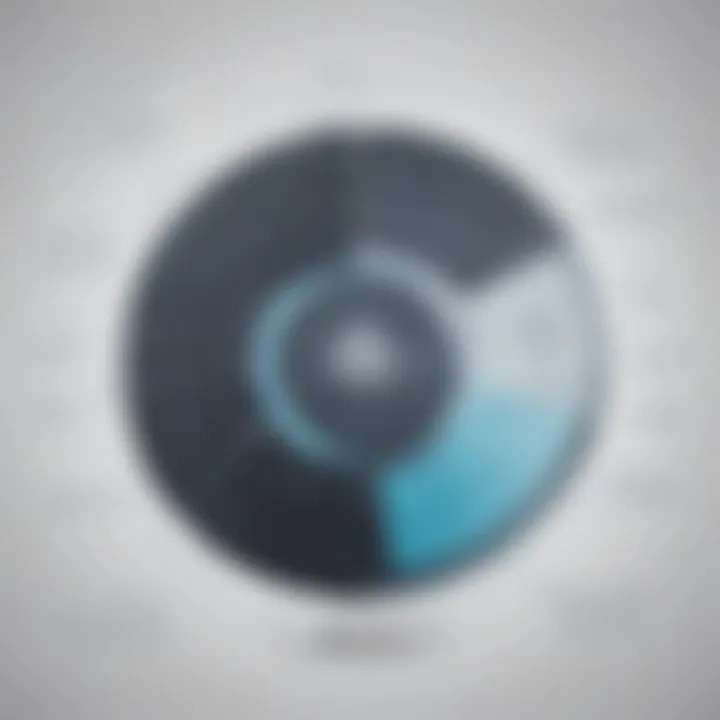
Tech Trend Analysis
When analyzing the tech trends related to entry-level data science jobs, it becomes evident that the demand for skilled professionals in this field continues to escalate. This escalation is primarily fueled by the rapid digitization of industries across the globe, emphasizing the importance of data-driven decision-making. As businesses strive to gain a competitive edge through data analytics, the need for entry-level data scientists becomes more pronounced. The implication for consumers revolves around experiencing enhanced products and services tailored to their preferences, as companies leverage data science to make informed strategic choices. Looking into the future, possibilities abound for entry-level data scientists to capitalize on the growing trend, opening new avenues for career advancement and professional development.
Product Reviews
Moving on to product reviews in the realm of entry data science jobs, it is vital to emphasize the importance of honing specific skills and acquiring relevant knowledge to excel in this domain. The 'product' here signifies the amalgamation of technical expertise, problem-solving abilities, and a keen analytical mind required for success in the field. Delving deeper into the features and specifications essential for aspiring data scientists, one must possess a strong foundation in programming languages such as Python and R, statistical analysis, machine learning techniques, and a familiarity with data visualization tools. Performance analysis in this context pertains to how effectively an entry-level data scientist can apply theoretical knowledge to real-world scenarios, showcasing their proficiency in handling complex data sets and deriving actionable insights. While the pros of entering the data science domain are vast - including lucrative career prospects, stimulating work environments, and the opportunity to drive innovation - one must also be cognizant of the cons, such as the steep learning curve and the constant need for upskilling to stay relevant in a rapidly evolving field. A recommendation for aspiring data scientists is to engage in hands-on projects, participate in online courses and bootcamps, and network with industry professionals to enhance their portfolio and stay abreast of industry developments.
How-To Guides
In crafting comprehensive how-to guides tailored for individuals venturing into entry-level data science roles, it is essential to provide a structured approach that simplifies complex concepts and tasks. The introduction to the topic should focus on demystifying the various facets of data science, elucidating its fundamental principles and significance in today's data-driven landscape. Moving on to step-by-step instructions, aspiring data scientists should start by mastering programming languages commonly used in data science like Python and R, followed by delving into statistical analysis and machine learning algorithms. Tips and tricks for success in this domain encompass practicing regularly on data science platforms, engaging in online communities to seek guidance and mentorship, and working on real-world projects to build a robust portfolio. Troubleshooting techniques should focus on addressing common challenges faced by entry-level data scientists, such as debugging code, optimizing model performance, and interpreting results accurately. By following these meticulous instructions, individuals can pave a clear path towards a successful career in data science.
Industry Updates
Considering the dynamic nature of the tech industry, staying abreast of industry updates is indispensable for individuals embarking on entry-level data science careers. Recent developments in technology often dictate the evolution of data science practices, with new tools and frameworks constantly reshaping the landscape. Analysis of market trends provides valuable insights into the demand for specific skill sets, allowing aspiring data scientists to tailor their learning path accordingly. The impact of these updates on businesses and consumers is far-reaching, influencing decision-making processes, product development strategies, and overall market competitiveness. By immersing themselves in industry updates and understanding the implications of technological advancements, entry-level data scientists can position themselves as valuable assets in a rapidly evolving digital economy.
Introduction to Entry Data Science Jobs
In the realm of data science, the introductory phase holds immense significance for aspiring professionals eager to embark on a journey into the world of analytics and insights. The section titled 'Introduction to Entry Data Science Jobs' serves as the gateway to understanding the foundational aspects of this field. By shedding light on various entry-level opportunities, this section enables individuals to grasp the basic requirements and expectations associated with such roles. Exploring the entry-level landscape equips budding data scientists with essential knowledge that forms the bedrock of their career progression.
What Are Entry Data Science Jobs?
The term 'Entry Data Science Jobs' encapsulates a diverse array of positions tailored for individuals with foundational knowledge in data analytics and machine learning. These roles typically require candidates to possess a fundamental understanding of statistical analysis, programming languages, and database management. Entry-level data science jobs serve as stepping stones for beginners wishing to acquaint themselves with the practical applications of data-driven decision-making. These positions often involve tasks such as data cleaning, visualization, and basic predictive modeling, laying a strong foundation for career advancement in the field.
Importance of Entry Data Science Roles
The significance of entry data science roles manifests in their ability to bridge the gap between theoretical knowledge and hands-on experience. These positions offer newcomers a unique opportunity to apply their learning in real-world scenarios, honing their skills through practical projects and challenges. By engaging with entry-level data science roles, individuals can gain valuable insights into the industry dynamics, data interpretation, and problem-solving methodologies. Moreover, these roles facilitate professional growth by fostering collaboration, learning from experienced professionals, and navigating complex data tasks with precision and efficiency.
Scope of Entry Data Science Positions
The scope of entry data science positions extends beyond mere job titles, encompassing a vast landscape of opportunities for emerging talents. From data analysts to business intelligence analysts and machine learning engineers, the variety of roles available in the entry-level segment caters to diverse skill sets and interests. Professionals entering this domain can explore avenues such as data visualization, predictive modeling, and trend analysis, contributing meaningfully to business strategies and decision-making processes. The broad scope of entry data science positions ensures that individuals can find roles aligned with their expertise and aspirations, enabling them to delve deeper into the realms of data-driven innovation.
Key Skills Required for Entry Data Scientists
In the dynamic realm of data science, possessing the right set of skills is paramount for individuals eyeing entry-level positions. Key skills required for entry data scientists encompass both technical prowess and soft skills, each playing a crucial role in ensuring success in this field. Technical skills form the backbone of a data scientist's toolkit, from programming languages proficiency to statistical analysis prowess and proficient database management. These abilities enable data scientists to manipulate and derive insights from vast quantities of data, a fundamental aspect of their role. On the other hand, soft skills such as critical thinking, effective communication, and problem-solving abilities are indispensable for interpreting data findings, collaborating with teams, and devising innovative solutions to complex problems. A combination of technical acumen and soft skills distinguishes exceptional data scientists from their counterparts and significantly influences career advancement and job performance.
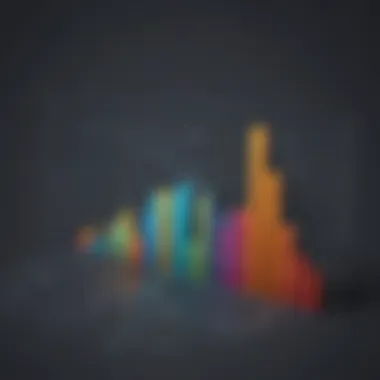
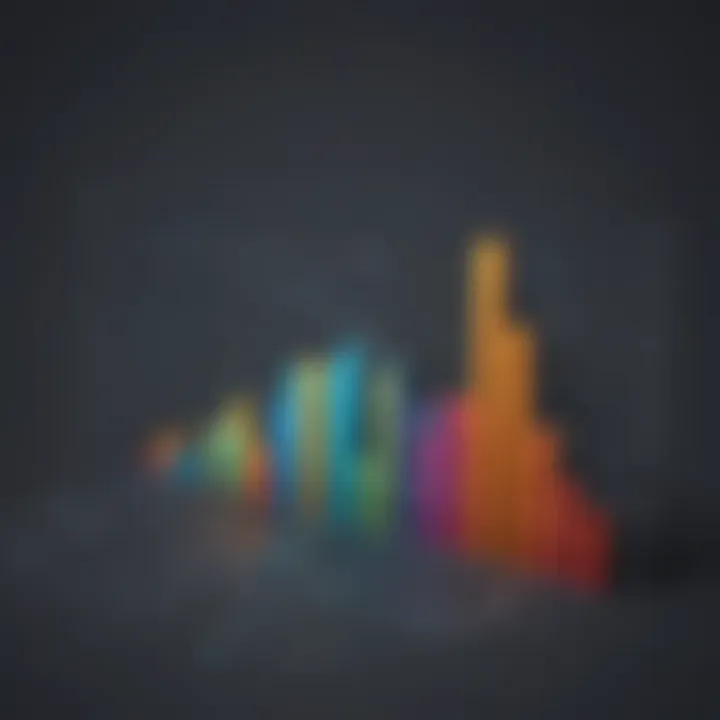
Technical Skills
Programming Languages Proficiency
Fluency in programming languages is a cornerstone skill for entry-level data scientists. Mastering languages such as Python, R, and SQL is crucial for data manipulation, statistical analysis, and machine learning algorithms. Programming language proficiency allows data scientists to extract, clean, and model data effectively, facilitating advanced analytics and insightful visualizations. The versatility of programming languages like Python enables data scientists to develop code for data projects, making it a popular choice in the data science domain. Its extensive libraries and intuitive syntax streamline the development process, offering a robust platform for data analysis. However, while Python's flexibility empowers data scientists, complexities in memory management may pose challenges in memory-intensive applications.
Statistical Analysis Skills
Statistical analysis skills are the foundation of data science, guiding data scientists in drawing meaningful insights from datasets. Proficiency in statistical techniques like hypothesis testing, regression analysis, and exploratory data analysis equips individuals to interpret data patterns and make informed decisions. Statistical analysis skills play a pivotal role in predictive modeling, identifying trends, and assessing the significance of findings. Despite the availability of advanced tools and software, a strong grasp of statistical concepts empowers data scientists to validate the accuracy of results and generate reliable solutions. However, the complexity of statistical analysis may require continuous learning and adaptation to evolving methodologies.
Database Management
Efficient database management is essential for storing, organizing, and retrieving data seamlessly in data science projects. Expertise in database management systems like MySQL, MongoDB, or Hadoop is invaluable for handling large datasets and ensuring data integrity. Data scientists rely on databases to access information swiftly, perform queries, and extract meaningful insights for analysis. Proper database management enhances data security, facilitates data retrieval, and supports collaboration among team members. While databases streamline data operations and enhance productivity, challenges may arise in managing complex relationships, optimizing query performance, and safeguarding data against security threats.
Soft Skills
Critical Thinking
Critical thinking is a pivotal soft skill for entry-level data scientists, enabling them to assess situations objectively, analyze problems critically, and propose well-founded solutions. Data scientists with strong critical thinking abilities can dissect complex issues, evaluate data discrepancies, and identify patterns that inform strategic decision-making. Critical thinking fosters innovation, encourages data-driven insights, and cultivates a holistic perspective towards problem-solving. The ability to leverage critical thinking enhances the quality of data analysis, drives continuous improvement, and positions data scientists as invaluable assets in their organizations. However, cultivating critical thinking skills requires practice, open-mindedness, and willingness to challenge conventional viewpoints.
Communication Skills
Effective communication is indispensable for data scientists to convey complex findings, collaborate with diverse stakeholders, and present recommendations cohesively. Strong communication skills enable data scientists to articulate technical concepts in a clear and understandable manner, facilitating seamless knowledge transfer and fostering collaboration across teams. Data scientists proficient in communication bridge the gap between technical and non-technical individuals, ensuring that data insights are communicated effectively and utilized to drive business decisions. However, mastering communication skills demands active listening, empathy, and the ability to tailor messages based on the audience's comprehension levels.
Problem-Solving Abilities
Problem-solving abilities are vital for data scientists to tackle intricate challenges, devise innovative solutions, and navigate uncertainties inherent in data projects. Data scientists proficient in problem-solving can approach complex problems systematically, identify root causes, and implement strategic solutions that optimize outcomes. Problem-solving skills empower data scientists to think analytically, experiment with approaches, and adapt methodologies to address evolving data requirements. A strong problem-solving acumen enhances data interpretation, streamlines decision-making processes, and fosters a culture of continuous improvement within data science teams. Nonetheless, honing problem-solving skills involves a blend of creativity, resilience, and willingness to embrace experimentation and learn from failures.
Educational Background and Qualifications
Educational background and qualifications play a pivotal role in the landscape of entry-level data science jobs. In this article, we delve into the critical aspects surrounding educational requirements for aspiring data scientists. A solid educational foundation serves as the bedrock upon which individuals can build their data science careers. When considering educational qualifications, candidates should prioritize degrees and certifications that equip them with relevant skills and knowledge needed in the field of data science. These credentials not only enhance one's competencies but also signal to potential employers a level of expertise and commitment to the field. Additionally, possessing the right educational background can open doors to a myriad of opportunities and career growth prospects in the competitive realm of data science.
Academic Requirements
The academic requirements for entry-level data science roles typically vary based on the specific job and industry sector. However, a common thread among these positions is the emphasis on a solid educational foundation in STEM fields. Candidates are often expected to hold a bachelor's degree in fields such as Computer Science, Statistics, Mathematics, or related disciplines. Additionally, in some cases, employers may prefer candidates with advanced degrees such as a Master's or Ph.D. in a relevant field. These academic prerequisites are essential in providing individuals with the necessary theoretical knowledge and analytical skills required to excel in data science roles. By meeting these academic requirements, aspiring data scientists can demonstrate their readiness to tackle complex data challenges and contribute effectively to their organizations.
Relevant Degrees and Certifications
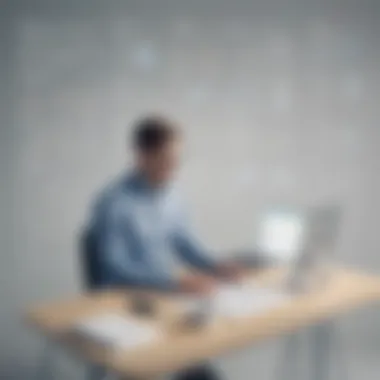
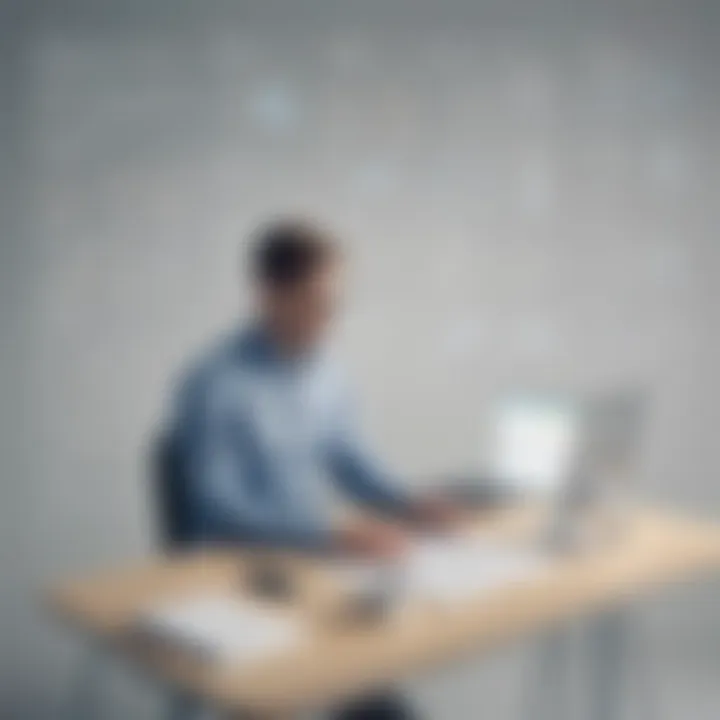
In the realm of data science, possessing relevant degrees and certifications can significantly bolster one's credibility and competencies. Degrees such as a Bachelor of Science in Data Science, Applied Statistics, or Computer Engineering are highly valued in the industry. Furthermore, certifications from reputable institutions or organizations, such as Microsoft Certified: Azure Data Scientist Associate or Google's Professional Data Engineer certification, can further validate an individual's expertise in specific areas of data science. These credentials serve as tangible proof of one's commitment to continuous learning and professional development, enhancing their marketability and attractiveness to potential employers seeking skilled data science professionals.
Internship and Project Experience
Internship and project experiences are invaluable components of a data scientist's educational journey. While formal education provides the foundational knowledge, internships and hands-on projects offer practical insights and real-world applications of data science concepts. Engaging in internships at tech companies, research institutions, or data science-focused organizations allows individuals to hone their skills, work on real projects, and gain industry exposure. Similarly, undertaking data-related projects independently or collaboratively can showcase one's problem-solving abilities, analytical skills, and creativity to potential employers. These experiences not only supplement formal education but also demonstrate a candidate's practical readiness to take on data science roles, making them stand out in a crowded job market.
Exploring Entry Data Science Job Titles
In this section, we will delve into the significance of exploring entry data science job titles within the broader landscape of data science career opportunities. Understanding the various job titles in the field is crucial for aspiring data scientists as it provides insights into different roles they can pursue. By exploring entry data science job titles, individuals can gain clarity on the specific responsibilities, skills required, and career progression within each role. This exploration allows them to align their career goals with the most suitable job titles, maximizing their potential for success in the data science industry.
Data Analyst
The role of a data analyst is fundamental in the realm of data science. Data analysts are responsible for collecting, organizing, and analyzing large sets of data to uncover valuable insights that can drive informed business decisions. They utilize statistical techniques and data visualization tools to present their findings in a clear and understandable manner. Aspiring data analysts should focus on honing their proficiency in programming languages, statistical analysis, and database management to excel in this role. Additionally, strong critical thinking and problem-solving abilities are vital for interpreting data effectively.
Business Intelligence Analyst
Business intelligence analysts play a crucial role in helping organizations leverage data to make strategic decisions. These professionals are tasked with transforming complex data into actionable insights that drive business growth. By utilizing various tools and technologies, business intelligence analysts identify trends, patterns, and opportunities that enable businesses to optimize performance and enhance competitiveness. To succeed in this role, individuals need strong communication skills to collaborate with different stakeholders and convey data-driven recommendations effectively.
Machine Learning Engineer
Machine learning engineers design and implement machine learning algorithms to develop AI-powered applications and systems. These engineers work at the intersection of data science and computer science, utilizing their expertise in programming and mathematics to build predictive models and algorithms. In-depth knowledge of machine learning techniques, software development, and algorithm optimization is essential for success in this role. Machine learning engineers should continuously update their skills to stay abreast of cutting-edge advancements in AI and machine learning, ensuring they remain at the forefront of innovation in the field.
Job Search Strategies for Entry Data Scientists
Entry-level data scientists need effective job search strategies to land desirable roles in the competitive field. Understanding the importance of targeted approaches is crucial for success. By focusing on Job Search Strategies for Entry Data Scientists, individuals can optimize their efforts, increase visibility to potential employers, and secure lucrative opportunities.
Building a Strong Portfolio
Building a strong portfolio is a critical component of standing out in the data science job market. Entry-level data scientists should showcase their projects, expertise, and problem-solving skills to prospective employers. A well-curated portfolio demonstrates practical application of technical knowledge and can set candidates apart from their peers.
Networking and Professional Connections
Networking and fostering professional connections are integral to advancing in the field of data science. Engaging with industry professionals, attending conferences, and participating in online communities can open doors to new opportunities. By nurturing relationships and seeking mentorship, entry-level data scientists can gain valuable insights and access to potential job openings.
Utilizing Job Boards and Platforms
Utilizing job boards and online platforms tailored for data science roles can significantly enhance the job search process. These platforms offer a centralized hub for job listings, industry insights, and networking opportunities. Entry-level data scientists can leverage these resources to stay informed about the latest trends, connect with potential employers, and secure interviews for relevant positions.
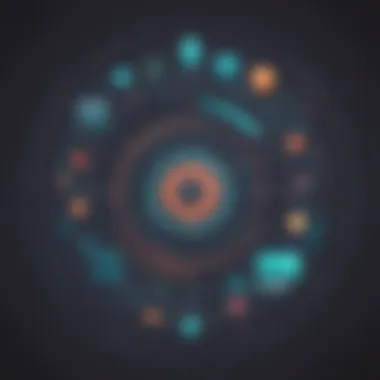
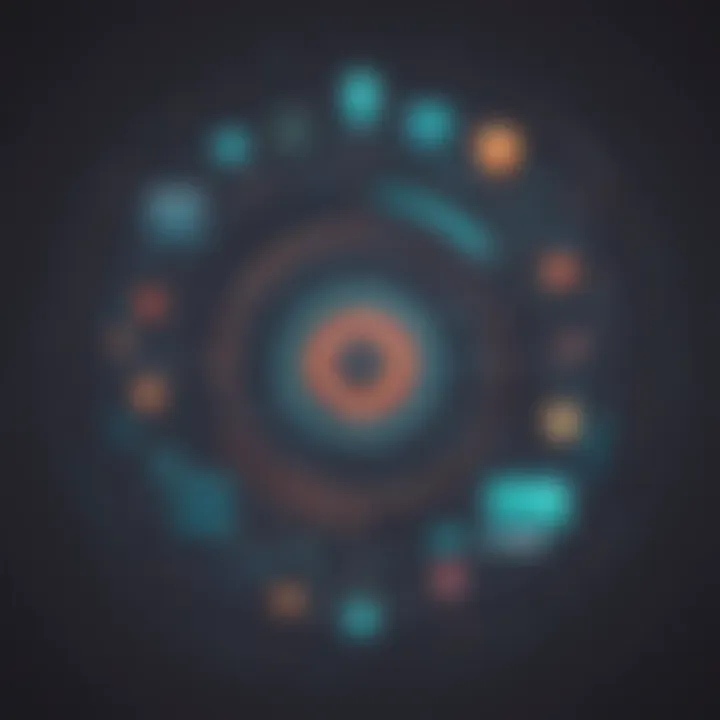
Salary Expectations and Growth Opportunities
In the realm of data science careers, understanding the landscape of salary expectations and growth opportunities holds paramount importance for aspiring professionals. The discussion surrounding salary expectations delves into the monetary compensation that entry-level data scientists can anticipate at the beginning of their careers. It serves as a crucial motivator and indicator of the value that organizations place on data-driven decision-making. Growth opportunities, on the other hand, shed light on the potential career advancements and professional development prospects available within the field of data science. By comprehensively exploring both salary expectations and growth opportunities, individuals can make informed decisions and chart strategic career paths.
Entry-Level Salary Range
When embarking on a career in data science, grasping the entry-level salary range is crucial for setting realistic expectations and gauging financial stability. Typically, entry-level data science roles offer a competitive yet varied salary spectrum, with factors such as location, industry, and company size influencing the compensation package. Entry-level data scientists can expect to earn anywhere from $X to $Y annually, with variations based on skill set, experience, and demand in the job market. It is essential for aspiring data scientists to research industry standards and analyze their qualifications to negotiate a fair salary aligned with their expertise.
Career Progression Paths
As data scientists navigate their professional journey, understanding the diverse career progression paths available within the data science domain is indispensable. Career progression in data science extends beyond vertical promotions to encompass skill enhancements, leadership opportunities, and specialization in niche areas. With a solid foundation in foundational skills, entry-level data scientists can advance to roles such as senior data scientist, data science manager, or data science architect. Embracing continuous learning, adapting to technological advancements, and showcasing real-world impact are pivotal for organic career growth within the dynamic realm of data science.
Advanced Roles in Data Science
At the pinnacle of a data scientist's career trajectory lie advanced roles that signify expertise, leadership, and innovation in the field. Advanced data science roles offer seasoned professionals the chance to spearhead groundbreaking projects, drive strategic decision-making, and mentor upcoming talents. Roles such as Chief Data Officer (CDO), Data Science Director, or AI Research Scientist showcase the culmination of years of experience, domain knowledge, and technical prowess in data science. Transitioning to advanced roles requires a blend of technical acumen, domain expertise, and visionary leadership to steer organizations towards data-driven success.
Challenges Faced by Entry Data Scientists
In the dynamic landscape of data science, entry-level professionals encounter a myriad of challenges that shape their career trajectory. Understanding and addressing these hurdles is imperative for aspiring data scientists to thrive in the competitive industry. This section delves deep into the multifaceted challenges faced by entry data scientists, shedding light on crucial aspects that pave the way for success.
Overcoming Lack of Experience
Navigating the realm of data science as a novice often entails grappling with the lack of hands-on experience. For entry-level data scientists, bridging this experience gap is a fundamental step towards establishing credibility and competence in the field. Strategies such as undertaking internships, participating in industry projects, or engaging in online learning platforms can serve as effective measures to augment one's experiential foundation. By actively seeking opportunities to apply theoretical knowledge to real-world scenarios, budding data scientists can expedite their professional growth and mitigate the challenges posed by limited experience.
Navigating Complex Data Projects
Effective navigation through complex data projects stands as a significant obstacle for entry data scientists due to the intricate nature of data analyses and interpretations. Engaging with large datasets, implementing sophisticated analytical techniques, and deriving actionable insights present formidable challenges that require adept problem-solving skills. By honing their analytical capabilities, staying abreast of emerging data technologies, and seeking mentorship from experienced professionals, entry-level data scientists can surmount the complexities of intricate data projects and emerge as proficient practitioners in the field.
Staying Updated with Industry Trends
In the fast-evolving sphere of data science, staying abreast of industry trends and technological advancements is pivotal for sustaining relevance and competitiveness. Entry data scientists face the challenge of continuously upskilling themselves to align with the latest tools, methodologies, and best practices prevailing in the industry. Investing time in attending seminars, workshops, enrolling in online courses, and following reputable data science publications can aid professionals in remaining informed and adaptable. By cultivating a habit of lifelong learning and embracing a proactive approach towards professional development, entry-level data scientists can navigate the ever-evolving landscape of data science with acumen and agility.
Conclusion
In the realm of data science, the Conclusion section holds a paramount position. It serves as a crucial compass for data science aspirants, guiding them through the intricate pathways of the field. The ultimate goal of this section is to encapsulate the essence of the entire article and provide a roadmap for individuals venturing into entry-level data science roles. By synthesizing the discussions on the importance of key skills, educational backgrounds, job titles, job search strategies, salary expectations, growth opportunities, and challenges faced by entry data scientists, the Conclusion ties together the diverse threads explored throughout the article. It offers a holistic perspective on the intricacies of entry data science jobs, aiming to empower readers with the knowledge necessary to embark on a successful career journey within the data science domain.
Summary of Key Points
The Summary of Key Points section acts as a concise recapitulation of the fundamental aspects covered in the preceding sections. It condenses the essential insights regarding the requirements, job outlook, growth opportunities, and challenges associated with entry-level data science roles. By highlighting the significance of technical and soft skills, educational backgrounds, job titles, job search strategies, salary expectations, growth trajectories, and challenges encountered by entry data scientists, this section offers a comprehensive snapshot of the diverse facets of entering the data science industry. Through a systematic elucidation of critical information, the Summary of Key Points equips readers with a quick reference guide to the core elements essential for navigating the landscape of entry data science jobs.
Embracing the Journey of Data Science Careers
Embracing the Journey of Data Science Careers embodies a profound exploration of the transformative odyssey embarked upon by aspiring data scientists. This section delves into the broader narrative of pursuing a career in data science, elucidating the intrinsic motivations, challenges, and triumphs associated with carving a niche in this dynamic field. By encouraging individuals to embrace the inherent uncertainties and continual evolution within data science careers, this section fosters a mindset of growth, adaptability, and resilience. Through a reflective lens, Embracing the Journey of Data Science Careers sparks a contemplative dialogue on the personal and professional growth that unfolds as individuals navigate the intricacies of data science, inspiring them to embark on this expedition with a sense of curiosity, passion, and purpose.