Unveiling the Myriad Dimensions of Artificial Intelligence: A Comprehensive Exploration
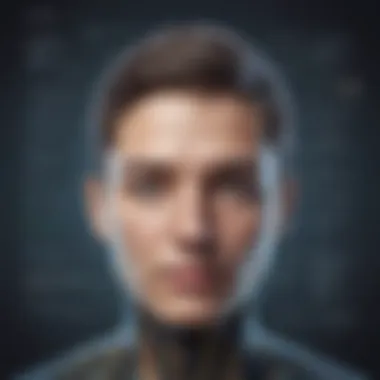
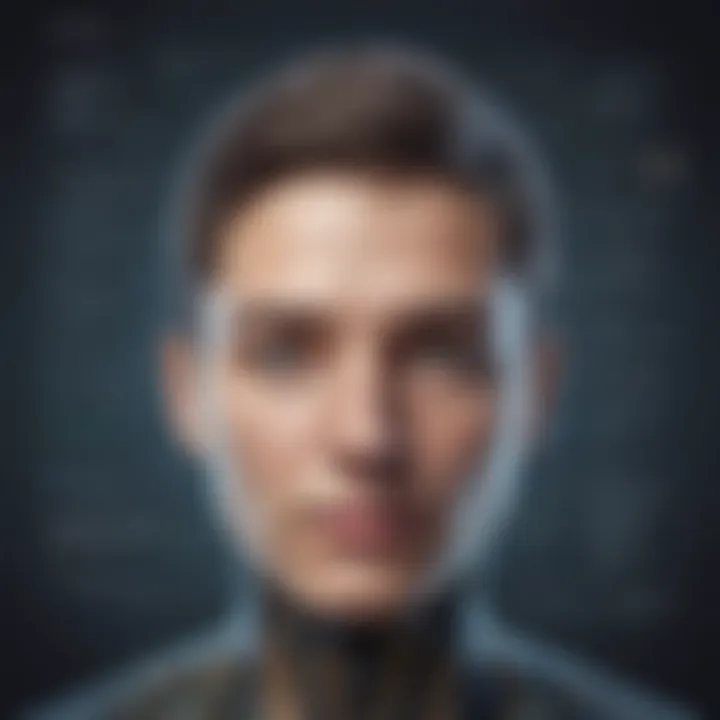
Tech Trend Analysis
Artificial Intelligence (AI) is currently at the forefront of technological advancements, revolutionizing various industries with its innovative applications. From predictive analytics to autonomous vehicles, AI is reshaping how businesses operate and consumers interact with technology. The implications of AI for consumers are vast, ranging from personalized recommendations in e-commerce to enhanced user experiences in virtual assistants. As AI continues to evolve, future predictions and possibilities suggest even greater integration into daily life, promising increased efficiency and automation across sectors.
Product Reviews
When delving into the different branches of Artificial Intelligence, it is essential to review the products driving AI innovation. These products come equipped with cutting-edge features and specifications designed to optimize performance and deliver impactful results. Performance analysis reveals the effectiveness of AI algorithms in various applications, highlighting their strengths and limitations. Understanding the pros and cons of each product enables informed decision-making, aiding in the selection of the most suitable AI technology for specific needs. Ultimately, recommendations based on thorough product reviews empower consumers and businesses to leverage AI effectively in their operations.
How-To Guides
Introducing individuals to the intricacies of Artificial Intelligence involves providing comprehensive how-to guides that simplify complex concepts into actionable steps. Beginner-friendly instructions break down the AI learning process into manageable tasks, guiding users through practical applications and examples. Tips and tricks enhance understanding by offering valuable insights and best practices for optimal AI utilization. Furthermore, troubleshooting guides address common challenges and errors, equipping readers with the knowledge to overcome technical hurdles effectively. By offering detailed how-to guides, individuals can enrich their AI knowledge and practical skills, fostering confidence in navigating the AI landscape.
Industry Updates
Keeping abreast of recent developments in the tech industry is crucial for understanding the evolving landscape of Artificial Intelligence. Through analysis of market trends, professionals can gain valuable insights into the trajectory of AI adoption and its impacts on businesses and consumers. Industry updates offer a comprehensive view of new AI applications, emerging technologies, and disruptive innovations. By monitoring industry developments, organizations can strategize effectively, anticipating market shifts and adapting their business models to leverage AI trends successfully. The dynamic nature of the tech industry underscores the importance of continuous learning and adaptation to stay competitive in the rapidly evolving world of Artificial Intelligence.
Introduction to Artificial Intelligence
Artificial Intelligence is a revolutionary field that has garnered significant attention in recent years due to its vast potential for transforming various industries. In this article, we will delve into the key aspects of Artificial Intelligence, focusing on understanding its importance, applications, and impact on modern society.
Understanding AI
Definition of Artificial Intelligence
Artificial Intelligence, commonly known as AI, refers to the simulation of human intelligence processes by machines, emphasizing the creation of intelligent agents that can perform tasks requiring human intelligence. This fundamental aspect of AI sets the stage for complex problem-solving, pattern recognition, and decision-making capabilities in machines. The unique feature of AI lies in its ability to adapt and learn from data, enabling it to continuously improve performance over time. While the advantages of AI are numerous, such as enhancing automation and efficiency, there are also considerations regarding ethical implications and the impact of AI on the job market.
Evolution of AI
The evolution of AI traces back to the mid-20th century when pioneers like Alan Turing and John McCarthy laid the groundwork for its development. Over the decades, AI has undergone significant advancements, transitioning from rule-based systems to more sophisticated machine learning algorithms. The key characteristic of AI's evolution is its progression from narrow AI applications to the emergence of general AI, capable of performing tasks across various domains. While the benefits of AI evolution are evident in improved accuracy and scalability, challenges such as ethical dilemmas and biased algorithms continue to be areas of concern.
Importance of AI in Modern Society
The importance of AI in modern society cannot be overstated, as it permeates sectors like healthcare, finance, and transportation, revolutionizing traditional practices. AI plays a pivotal role in improving medical diagnostics, optimizing financial operations, and enhancing the safety of autonomous vehicles. Its key characteristic lies in its ability to analyze vast amounts of data swiftly and accurately, enabling better decision-making and innovative solutions. However, the ethical considerations surrounding AI, including data privacy and algorithmic bias, underscore the need for responsible integration and governance of AI technologies.
Applications of AI
AI in Healthcare
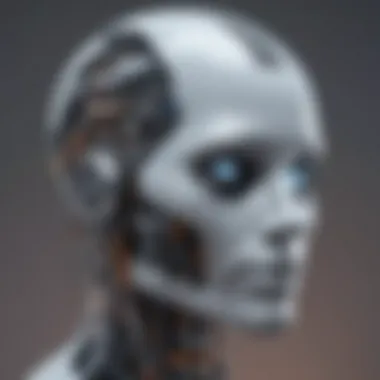
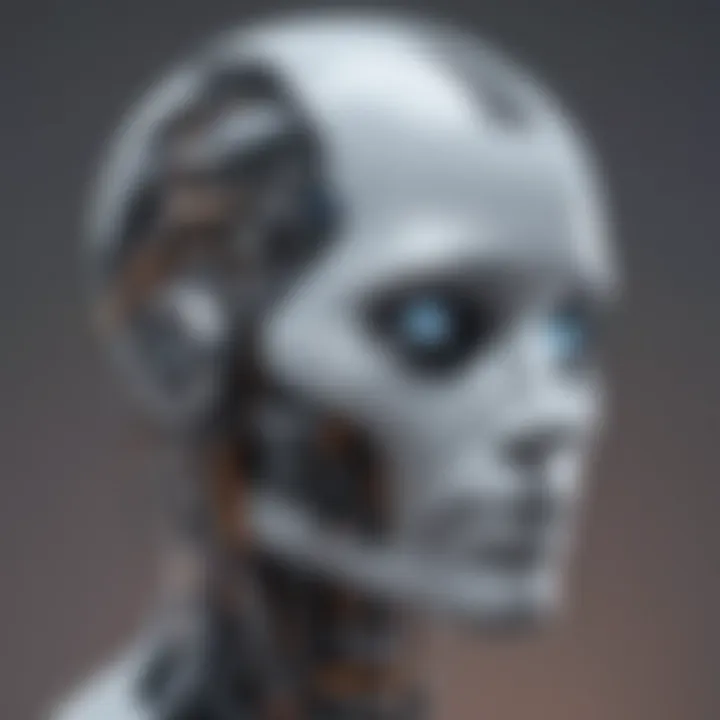
AI's application in healthcare holds immense promise for revolutionizing patient care and medical research. By leveraging machine learning algorithms, AI can assist in diagnosing diseases, personalizing treatment plans, and predicting patient outcomes. The key characteristic of AI in healthcare is its potential to enhance clinical decision-making and streamline healthcare workflows, contributing to improved efficiency and patient outcomes. Despite its advantages, challenges related to data security and regulatory compliance remain pivotal considerations in the integration of AI in healthcare settings.
AI in Finance
In the financial sector, AI is utilized for tasks such as algorithmic trading, risk assessment, and fraud detection, enhancing operational efficiency and decision-making processes. The key characteristic of AI in finance lies in its ability to analyze complex financial data rapidly and accurately, enabling organizations to mitigate risks and capitalize on market opportunities. While AI offers benefits like predictive analytics and automated trading, potential risks like algorithmic bias and cybersecurity vulnerabilities require vigilant oversight and regulatory frameworks within the financial industry.
AI in Autonomous Driving
AI plays a crucial role in the development of autonomous driving technologies, transforming the transportation industry with advancements in self-driving vehicles. By employing computer vision and sensor fusion technologies, AI enables vehicles to perceive the environment, make real-time decisions, and navigate routes autonomously. The key characteristic of AI in autonomous driving is its potential to improve road safety, alleviate traffic congestion, and enhance overall transportation efficiency. However, challenges related to regulatory compliance, ethical dilemmas, and public acceptance pose significant hurdles in the widespread adoption of autonomous driving systems.
Branches of Artificial Intelligence
Artificial Intelligence (AI) is a vast field with several branches that play a crucial role in driving innovation and technological advancements across various industries. Understanding the different branches of AI is essential to comprehend the diverse applications and capabilities of this rapidly evolving technology. Machine learning, natural language processing, computer vision, robotics, expert systems, cognitive computing, and AI ethics and governance are key branches that shape the landscape of AI.
Machine Learning
Machine learning is a fundamental branch of artificial intelligence that focuses on developing algorithms capable of learning from and making predictions or decisions based on data. Within machine learning, there are three main types: supervised learning, unsupervised learning, and reinforcement learning.
Supervised Learning
Supervised learning involves training a model on a labeled dataset to predict outcomes accurately. This approach relies on providing the algorithm with input-output pairs to learn the mapping function. Supervised learning is advantageous as it can make precise predictions based on historical data, but it may struggle with unseen data patterns.
Unsupervised Learning
In contrast, unsupervised learning aims to find hidden patterns or intrinsic structures in unlabeled data. Without predefined outcomes, unsupervised learning algorithms explore data to identify similarities or anomalies. Unsupervised learning is beneficial for clustering data points and discovering meaningful relationships, yet it may lack the interpretability of supervised approaches.
Reinforcement Learning
Reinforcement learning involves training agents to make sequential decisions through interactions with an environment. By rewarding desired behaviors and penalizing incorrect actions, reinforcement learning models learn optimal strategies over time. This branch is well-suited for dynamic environments and scenarios where an agent must learn through trial and error.
Natural Language Processing
Natural Language Processing (NLP) focuses on enabling machines to understand, interpret, and generate human language. Various tasks within NLP, such as text classification, sentiment analysis, and language generation, contribute to enhancing communication between humans and machines.
Text Classification
Text classification involves categorizing text documents into predefined classes. This task is essential for organizing and structuring textual data, enabling efficient information retrieval and analysis. Text classification models can automate processes like spam detection, content categorization, and sentiment classification.
Sentiment Analysis
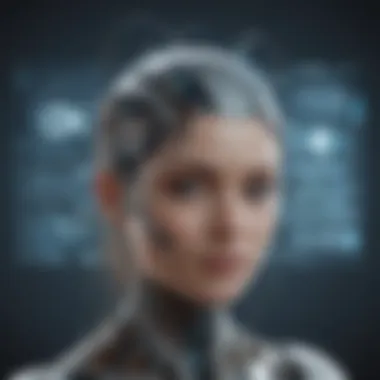
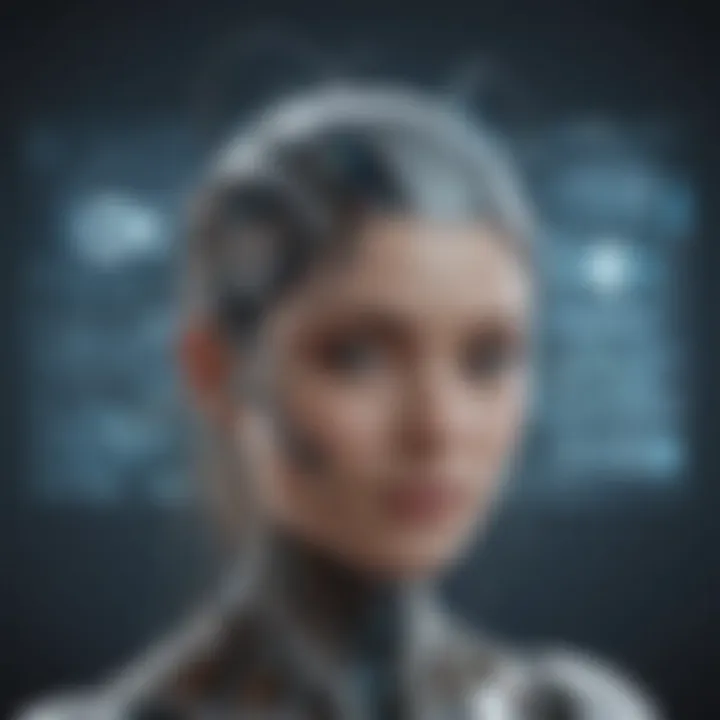
Sentiment analysis aims to determine the emotional tone or polarity of text. By analyzing the sentiment behind words or phrases, machines can gauge public opinion, customer feedback, and social media responses. Sentiment analysis is valuable for understanding user sentiments towards products, services, or topics.
Language Generation
Language generation pertains to the automatic creation of coherent and contextually relevant human-like text. This capability is essential for chatbots, virtual assistants, and content generation tasks. Language generation models use techniques like recurrent neural networks or transformers to produce natural language responses.
Computer Vision
Computer vision involves teaching machines to interpret and analyze visual information, similar to how humans perceive the world. Image recognition, object detection, and facial recognition are key components of computer vision that drive innovations in areas like surveillance, healthcare, and augmented reality.
Image Recognition
Image recognition focuses on identifying objects, scenes, or actions within images. By recognizing patterns and features in visual data, machines can categorize and classify images accurately. Image recognition is extensively used in industries ranging from autonomous vehicles to medical imaging.
Object Detection
Object detection goes a step further by not only identifying objects in an image but also locating and delineating their boundaries. This capability is crucial for applications like video surveillance, industrial automation, and augmented reality, where precise object localization is essential.
Facial Recognition
Facial recognition technology analyzes facial features to recognize and authenticate individuals. This technology finds applications in security systems, access control, and personalized customer experiences. Facial recognition algorithms can detect faces, verify identities, and enhance user convenience.
Robotics
Robotics integrates AI with mechanical engineering to create intelligent machines that can perform tasks autonomously or interact with their environment. Through branches like autonomous robots, robot perception, and human-robot interaction, robotics revolutionizes industries such as manufacturing, healthcare, and exploration.
Autonomous Robots
Autonomous robots are designed to operate independently, executing tasks without external intervention. These robots navigate, sense their surroundings, and make decisions without human control. Autonomous robots are employed in domains like agriculture, logistics, and space exploration for their efficiency and reliability.
Robot Perception
Robot perception enables machines to sense and comprehend their environment through sensors and cameras. By processing sensory data, robots can map their surroundings, recognize objects, and navigate complex spaces. Robot perception is critical for enhancing the autonomy and safety of robotic systems.
Human-Robot Interaction
Human-robot interaction focuses on creating seamless communication and collaboration between humans and machines. This branch explores interfaces, gestures, and feedback mechanisms that facilitate intuitive interactions. Human-robot interaction enhances user experience in settings like assistive robotics, entertainment, and customer service.
Expert Systems
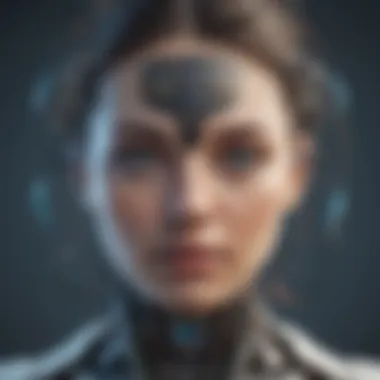
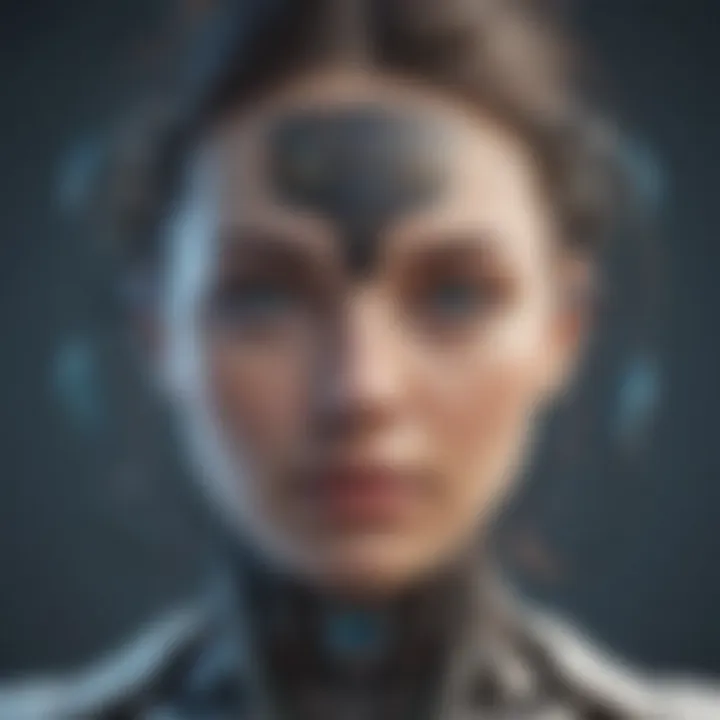
Expert systems are AI applications that mimic human expertise to solve complex problems or provide decision support in specialized domains. Components like knowledge representation, inference engines, and knowledge bases empower expert systems to analyze data, make deductions, and offer insights in fields like healthcare, finance, and engineering.
Knowledge Representation
Knowledge representation involves structuring information in a format that machines can manipulate and reason with. By representing knowledge in a logical form, expert systems can encode rules, facts, and relationships to facilitate problem-solving. Knowledge representation enhances the interpretability and efficiency of AI systems.
Inference Engine
An inference engine processes rules and knowledge in an expert system to derive logical conclusions or make decisions. This engine executes inferencing mechanisms, such as forward or backward chaining, to draw inferences from available information. Inference engines play a central role in simulating human-like reasoning processes.
Knowledge Base
The knowledge base stores domain-specific knowledge used by an expert system to provide intelligent responses. This repository contains facts, rules, and heuristics that guide the system's decision-making processes. A well-structured knowledge base is essential for accurate problem-solving and effective decision support.
Cognitive Computing
Cognitive computing seeks to emulate human thought processes by combining artificial intelligence and advanced analytics. Through functionalities like emotion analysis, contextual understanding, and decision-making, cognitive computing systems enhance problem-solving, learning, and communication capabilities.
Emotion Analysis
Emotion analysis involves identifying and understanding human emotions expressed through text, speech, or facial cues. By associating emotions with users' interactions, machines can tailor responses and experiences accordingly. Emotion analysis is beneficial for personalizing services, detecting fraud, and improving user engagement.
Contextual Understanding
Contextual understanding focuses on interpreting the context surrounding information to derive accurate meanings. By analyzing situational cues, data dependencies, and linguistic nuances, machines can grasp the subtleties of human communication. Contextual understanding enhances the relevance and accuracy of automated systems.
Decision Making
Decision-making algorithms aim to replicate human decision processes by evaluating options, predicting outcomes, and selecting optimal choices. These algorithms assist in complex scenarios where data-driven decisions are required. Decision-making systems aid in risk assessment, resource allocation, and strategic planning.
AI Ethics and Governance
AI ethics and governance address the moral, legal, and societal implications of artificial intelligence technologies. Concerns such as bias in AI, transparency and accountability, and ethical decision-making are pivotal in shaping ethical frameworks, policies, and responsible AI practices.
Bias in AI
Bias in AI refers to the unfair, unjust, or discriminatory outcomes produced by artificial intelligence systems. Biases in training data or algorithm design can perpetuate inequities and reinforce prejudices. Addressing bias in AI is crucial to ensure fairness, equality, and ethical use of AI technologies.
Transparency and Accountability
Transparency and accountability in AI advocate for clear, explainable, and accountable practices in AI development and deployment. Ensuring that AI systems operate transparently and are accountable for their decisions builds trust and safeguards against unethical use. Transparency promotes user understanding and ethical responsibility in AI applications.
Ethical Decision-Making
Ethical decision-making in AI involves embedding ethical principles and values into AI systems to guide their behavior and choices. Aligning AI practices with ethical standards and cultural norms fosters responsible AI innovation. Ethical decision-making frameworks support the development of AI technologies that respect human rights and societal well-being.