Unveiling the Depths of Descriptive Analytics in Data Science
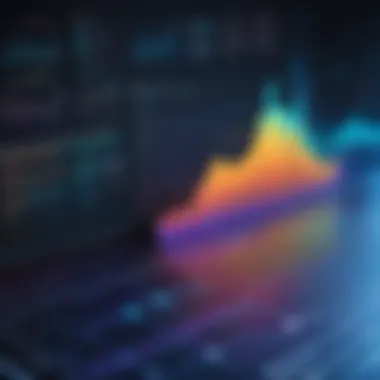
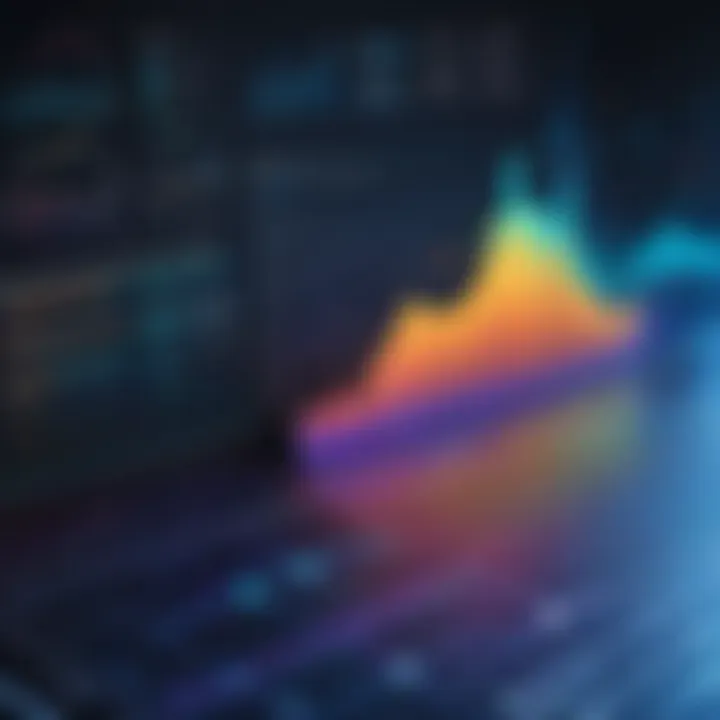
Tech Trend Analysis
Data science, a pivotal component of the tech ecosystem, is experiencing a significant upward trend. As businesses increasingly recognize the value of data-driven insights📈, the demand for descriptive analytics has surged. This trend is reshaping how companies operate and make strategic decisions. Consumers, on the other hand, stand to benefit from more personalized services and products tailored to their preferences🛍️. Looking ahead, the future of descriptive analytics holds promising advancements in automation and scalability, paving the way for enhanced efficiency and decision-making. Embracing this trend is crucial for staying competitive in the dynamic tech landscape. More Data for a Brighter Future!
Product Reviews
Exploring Descriptive Analytics tools unveils a vast array of options, each offering unique features🔧 and capabilities. From user-friendly interfaces to advanced visualization techniques, these tools cater to diverse analytical needs. The performance analysis of these tools showcases their efficacy in handling vast datasets📈, extracting actionable insights, and streamlining data processing workflows⚙️. While some tools boast robust data visualization capabilities, others excel in data preprocessing and statistical analysis. Understanding the pros and cons of each tool is essential for selecting the most suitable option for your analytical requirements. Mapping Out The Best Tools for Descriptive Analytics!
How-To Guides
Embarking on the journey of descriptive analytics requires a solid foundation in data preprocessing and visualization techniques. By familiarizing yourself with key concepts such as data aggregation, summarization, and visualization📊, you can enhance your analytical skills and extract meaningful insights from data. Step-by-step instructions simplify the process of data exploration and interpretation, enabling you to derive actionable conclusions from complex datasets. Additionally, leveraging tips and tricks from experienced practitioners enhances your proficiency in descriptive analytics✨. Troubleshooting common challenges in data analysis ensures smoother workflows and accurate results. Enhance Your Analytical Skills Today!
Industry Updates
The tech industry is abuzz with rapid advancements and groundbreaking innovations in the realm of descriptive analytics. Recent developments have seen the integration of machine learning algorithms into analytical tools, revolutionizing data interpretation and prediction capabilities🔮. Market trends indicate a shift towards real-time data processing and predictive modeling, empowering businesses to make proactive decisions and stay ahead of the curve. This evolution in descriptive analytics is reshaping industries, from e-commerce to healthcare, driving efficiency and innovation. The impact on businesses and consumers is profound, enabling better-informed decisions and personalized experiences. Embracing the Latest Trends in Descriptive Analytics!
Introduction to Descriptive Analytics
Descriptive analytics holds a vital position in the realm of data science, serving as the foundation for insightful data interpretation and decision-making processes. In this article, we embark on a journey to unravel the nuances of descriptive analytics, shedding light on its significance and practical implications in the digital era. By delving into the intricate world of descriptive analytics, we aim to equip tech enthusiasts and industry professionals with the necessary knowledge to harness the power of data-driven insights effectively. Through a detailed exploration of various descriptive analytics concepts and methodologies, readers will gain a comprehensive understanding of its role in transforming raw data into actionable intelligence.
Understanding Descriptive Analytics
Definition and Purpose
The cornerstone of descriptive analytics lies in its ability to summarize and present historical data in a comprehensible format, enabling stakeholders to extract valuable insights and trends. By elucidating the past behaviors and performance metrics of a system, definition, and purpose of descriptive analytics facilitate a deeper understanding of the underlying dynamics. Its systematic approach to data analysis not only elucidates trends and patterns but also aids in forecasting future outcomes based on historical trends. Although derived from structured data, this method offers an organized framework to interpret large datasets efficiently.
Key Components
Anchored in a robust framework, the key components of descriptive analytics encompass statistical measures, data visualization techniques, and clustering methodologies. These components play a pivotal role in transforming intricate datasets into actionable intelligence by uncovering patterns and anomalies hidden within the data. Through the application of summary statistics like mean, median, and mode, organizations can derive key insights into the central tendencies and variability of their data. Furthermore, data visualization tools such as charts, heatmaps, and scatter plots facilitate the intuitive representation of complex data relationships, aiding in effective decision-making processes.
Role in Data Science
Within the broader landscape of data science, descriptive analytics serves as the foundational layer that sets the stage for more advanced analytical processes. Its role in data science is indispensable, as it forms the basis for exploratory data analysis, enabling data scientists to delve deep into the intricacies of raw data. By providing a systematic framework for data interpretation and pattern recognition, descriptive analytics paves the way for predictive modeling and prescriptive analytics, thereby driving strategic decision-making. This pivotal role underscores the significance of descriptive analytics as a catalyst for deriving actionable insights from vast datasets, propelling organizations towards data-driven success.
Techniques in Descriptive Analytics
In the realm of data science, techniques in descriptive analytics play a vital role in unraveling insights from vast datasets. Understanding the nuances of descriptive analytics tools and methodologies is crucial for extracting meaningful information. These techniques form the cornerstone of data analysis, offering a structured approach to interpreting and summarizing data. By diving deep into summary statistics, data visualization, and clustering techniques, data scientists can uncover patterns, trends, and relationships hidden within the data.
Summary Statistics
Mean, Median, Mode
Mean, Median, and Mode are fundamental measures in descriptive analytics that provide key insights into the central tendency of a dataset. The mean represents the average value of a dataset, the median signifies the middle value when the data is arranged in ascending order, and the mode denotes the most frequent value. These measures offer a comprehensive view of the data distribution and help analysts in understanding the dataset's characteristics. While the mean is sensitive to outliers, the median is robust in the presence of skewed data. Understanding these measures is essential for accurate data interpretation in various domains.
Standard Deviation
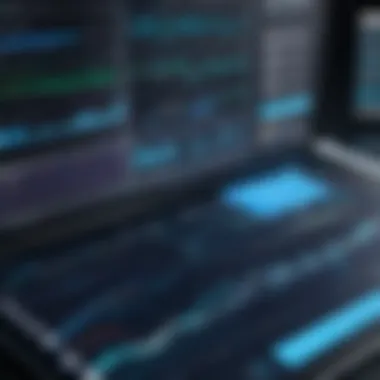
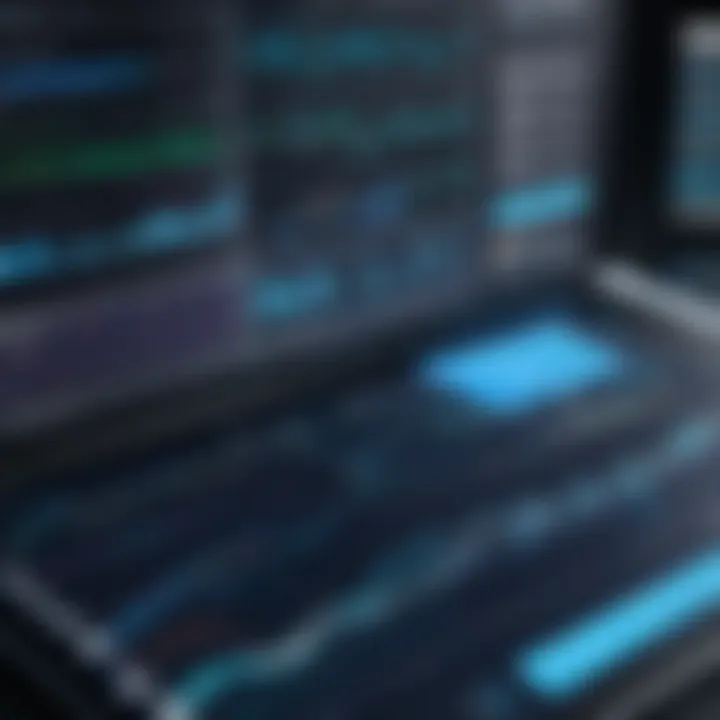
Standard deviation indicates the dispersion of data points around the mean and provides insights into the dataset's variability. It quantifies the spread of values and highlights the degree of deviation from the average. A higher standard deviation suggests greater variability, while a lower value indicates data points are closer to the mean. This measure aids in assessing the consistency and stability of data, enabling analysts to make informed decisions based on the data's reliability and predictability.
Variance
Variance complements standard deviation by offering a squared measure of the average deviation from the mean. It quantifies the dispersion of values and provides a comprehensive understanding of the dataset's spread. While standard deviation offers the variability in the original units, variance offers a squared unit of measure, aiding analysts in evaluating the overall distribution of data. Understanding the variance allows analysts to gauge the dataset's diversity and identify patterns or anomalies that may impact the analysis process.
Data Visualization
Types of Charts and Graphs
Data visualization techniques such as charts and graphs are indispensable tools in descriptive analytics for communicating complex data insights effectively. Different types of visualizations, including bar charts, line graphs, and pie charts, offer unique perspectives on the data, making patterns more apparent and facilitating quick interpretation. By harnessing the power of visual representations, analysts can uncover trends, outliers, and correlations within the data, leading to informed decision-making and actionable insights.
Heatmaps and Scatter Plots
Heatmaps and scatter plots are advanced data visualization techniques that enable analysts to explore relationships and dependencies between variables. Heatmaps visually represent data values through color gradients, providing a clear overview of patterns and correlations. Scatter plots, on the other hand, depict the relationship between two variables through plotted points, allowing analysts to identify clusters or trends within the data. These visualization tools enhance the data exploration process, aiding in pattern recognition and hypothesis generation.
Dashboard Creation
Dashboard creation plays a pivotal role in data visualization by consolidating multiple visual elements into a unified interface. Dashboards offer a holistic view of key metrics and KPIs, allowing users to monitor performance, track trends, and gain actionable insights at a glance. By customizing dashboards with interactive features and dynamic visualization components, analysts can create intuitive interfaces for data exploration and decision-making. Dashboards streamline the data analysis process, empowering users to extract meaningful insights efficiently.
Clustering Techniques
K-Means Clustering
K-Means clustering is a widely used unsupervised algorithm in descriptive analytics for grouping similar data points into clusters. By iteratively assigning data points to the nearest cluster center, K-Means identifies patterns and structures within the dataset. This technique is valuable for segmenting data into distinct groups based on similarity, enabling analysts to uncover hidden patterns and relationships. K-Means clustering is robust, scalable, and efficient, making it a popular choice for exploratory data analysis and data segmentation tasks.
Hierarchical Clustering
Hierarchical clustering is another powerful clustering technique that organizes data points into a hierarchical tree-like structure. By iteratively merging or splitting clusters based on similarity, hierarchical clustering reveals the underlying relationships among data points. This technique enables analysts to visualize cluster hierarchies and explore nested structures within the data. Hierarchical clustering offers flexibility in cluster interpretation and allows analysts to delve deeper into the dataset's structure, facilitating comprehensive data understanding and pattern recognition.
DBSCAN
DBSCAN (Density-Based Spatial Clustering of Applications with Noise) is a density-based clustering algorithm that identifies clusters of varying shapes and sizes within the data. By grouping data points based on their density reachability, DBSCAN can effectively segregate noise from meaningful clusters. This algorithm is robust against outliers and can discover clusters of arbitrary shapes, making it suitable for datasets with irregular patterns and varying densities. DBSCAN is adept at handling large datasets and is widely utilized in anomaly detection and spatial data analysis tasks.
Applications of Descriptive Analytics
In the realm of data science and analysis, the Applications of Descriptive Analytics play a pivotal role in extracting valuable insights and patterns from data sets. Understanding and harnessing the power of Descriptive Analytics is crucial for making informed decisions, evaluating performance metrics, and identifying trends. By utilizing various techniques in Descriptive Analytics, businesses and industries can gain a competitive edge by leveraging data-driven strategies to optimize operations and drive growth. The exploration of Applications of Descriptive Analytics in this comprehensive guide sheds light on the significance of this methodology in the broader context of data science.
Business Intelligence
Market Analysis
Market Analysis is a fundamental aspect of Business Intelligence that involves evaluating market trends, consumer behavior, and competitive landscapes. This analysis helps enterprises to understand market dynamics, identify opportunities for growth, and make strategic business decisions based on data-driven insights. Market Analysis enables organizations to assess market demand, develop targeted marketing campaigns, and enhance customer engagement. While Market Analysis provides valuable market insights, it requires accurate and up-to-date data for reliable decision-making.
Customer Segmentation
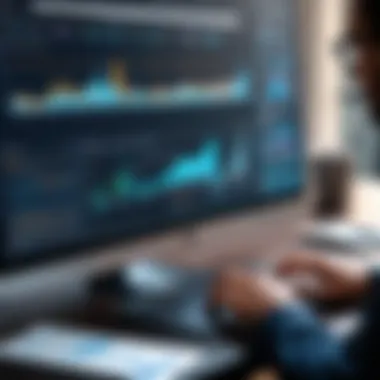
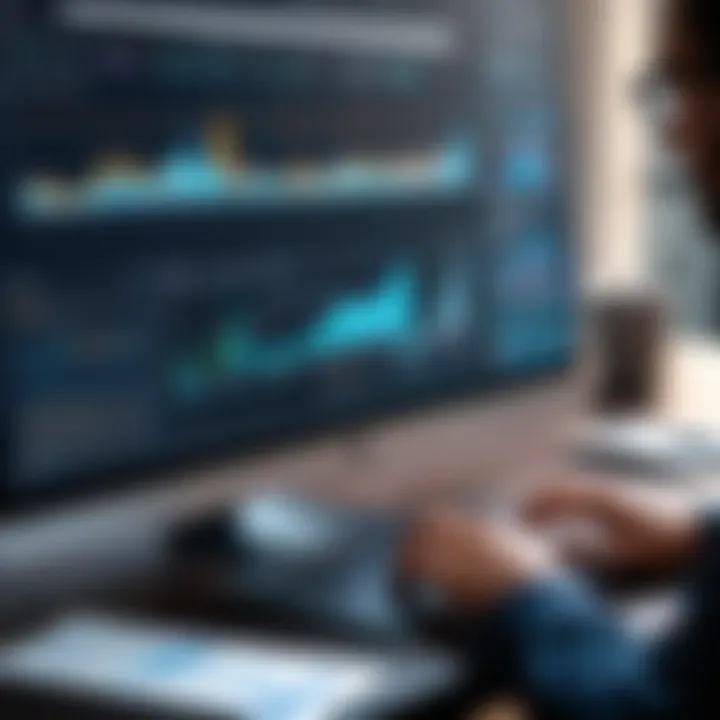
Customer Segmentation is a strategic approach within Business Intelligence that involves dividing a customer base into distinct groups based on specific criteria such as demographics, behavior, or preferences. This segmentation allows businesses to tailor their products and services to better meet the needs of different customer segments, thereby improving customer satisfaction and loyalty. By understanding the unique characteristics of each customer segment, companies can personalize marketing strategies, optimize pricing strategies, and increase conversion rates. However, effective Customer Segmentation requires detailed customer data and sophisticated analytical tools for accurate segmentation.
Competitor Benchmarking
Competitor Benchmarking is a critical component of Business Intelligence that involves evaluating the performance of competitors in the market to assess strengths, weaknesses, and market positioning. By analyzing competitor strategies, product offerings, and customer feedback, businesses can gain valuable insights to refine their own strategies and differentiate themselves in the market. Competitor Benchmarking enables organizations to identify market gaps, benchmark performance metrics, and drive innovation by learning from industry leaders. However, effective Competitor Benchmarking requires access to reliable competitor data and a comprehensive competitive analysis framework.
Healthcare Analytics
Patient Diagnosis
Patient Diagnosis is a key aspect of Healthcare Analytics that focuses on using data analysis and medical records to identify and diagnose medical conditions in patients. By leveraging advanced analytical tools and algorithms, healthcare providers can streamline diagnosis processes, reduce diagnostic errors, and improve patient outcomes. Patient Diagnosis plays a crucial role in personalized medicine, early disease detection, and treatment planning. While Patient Diagnosis enhances healthcare decision-making, it requires comprehensive patient data, medical expertise, and ethical considerations for patient privacy.
Treatment Optimization
Treatment Optimization is a pivotal area within Healthcare Analytics that aims to enhance treatment protocols, medication plans, and healthcare interventions based on data insights. By analyzing patient responses, treatment outcomes, and clinical pathways, healthcare professionals can optimize treatment regimens to deliver personalized and effective care. Treatment Optimization enables healthcare providers to reduce treatment costs, minimize adverse effects, and improve patient adherence to treatment plans. However, effective Treatment Optimization necessitates integrated health data systems, evidence-based practices, and continuous monitoring for treatment efficacy.
Epidemiological Studies
Epidemiological Studies are essential in Healthcare Analytics, focusing on the patterns, causes, and effects of diseases within populations. By conducting epidemiological research and analyzing health datasets, researchers can identify disease trends, risk factors, and public health challenges. Epidemiological Studies provide valuable insights for disease prevention, health policy development, and healthcare resource allocation. While Epidemiological Studies contribute to evidence-based decision-making in public health, they require robust data sources, statistical modeling expertise, and interdisciplinary collaborations to address complex health issues effectively.
Predictive Maintenance
Equipment Monitoring
Equipment Monitoring is a critical aspect of Predictive Maintenance, involving continuous tracking of machine performance, operational metrics, and maintenance requirements. By deploying sensor technologies, Io T devices, and predictive algorithms, organizations can monitor equipment health in real-time, predict maintenance issues, and prevent costly downtime. Equipment Monitoring enables proactive maintenance strategies, predictive analytics, and condition-based maintenance scheduling. While Equipment Monitoring enhances operational efficiency and asset reliability, it necessitates data integration, sensor calibration, and predictive maintenance expertise for effective equipment monitoring.
Failure Prediction
Failure Prediction is a key component of Predictive Maintenance that focuses on predicting equipment failures before they occur, allowing organizations to prevent downtime and optimize maintenance schedules. By analyzing historical performance data, failure patterns, and maintenance logs, predictive models can forecast potential equipment failures, recommend maintenance actions, and minimize disruptions to operations. Failure Prediction empowers organizations to implement predictive maintenance strategies, reduce maintenance costs, and extend the lifespan of assets. However, effective Failure Prediction requires accurate failure data, predictive modeling techniques, and collaboration between maintenance teams and data scientists.
Downtime Reduction
Downtime Reduction is a crucial objective of Predictive Maintenance, aiming to minimize unplanned downtime, increase equipment availability, and enhance overall operational efficiency. By implementing predictive maintenance strategies, condition monitoring systems, and reliability-centered maintenance practices, organizations can proactively address maintenance issues, optimize asset performance, and maximize production uptime. Downtime Reduction strategies facilitate continuous production processes, cost savings, and improved reliability of critical equipment. While Downtime Reduction enhances operational resilience, it requires proactive maintenance planning, real-time data analytics, and cross-functional collaboration to achieve sustainable downtime reduction.
Challenges and Limitations
When delving into descriptive analytics in data science, it is crucial to acknowledge and address the challenges and limitations that come with this analytical approach. Understanding these aspects is paramount for ensuring the accuracy and reliability of data-driven insights. By exploring the challenges and limitations in this article, readers can grasp the complexities involved and the considerations required for effective use in real-world applications.
Data Quality Issues
Incomplete Data
Incomplete data poses a significant obstacle in the realm of descriptive analytics. Its presence can skew analysis results, leading to inaccurate conclusions. Incomplete data refers to missing or unavailable information within a dataset, hindering the comprehensive understanding of the underlying trends or patterns. Despite its drawbacks, incomplete data can offer valuable insights into data collection processes and the need for improved data management strategies.
Inconsistent Data
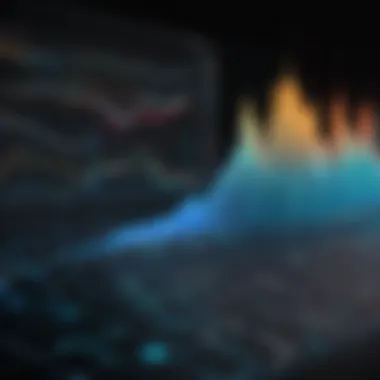
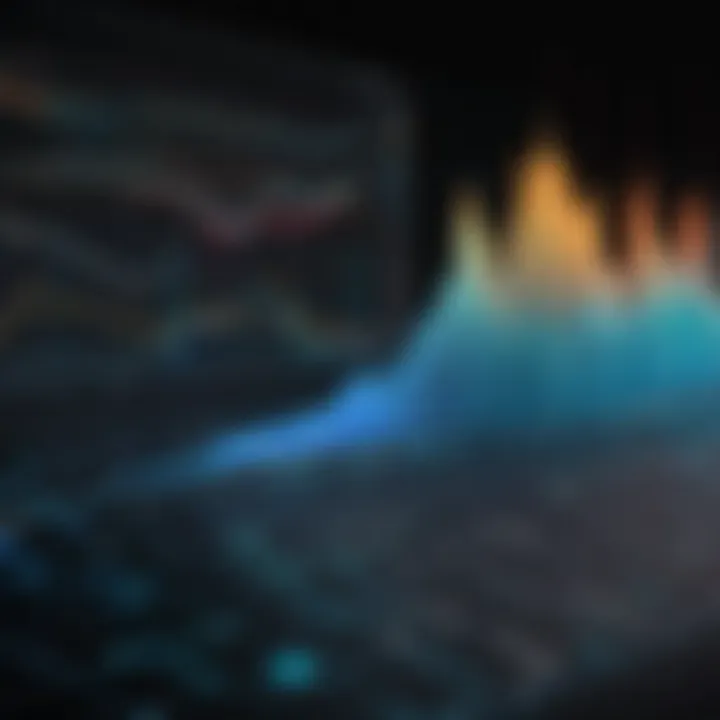
Inconsistent data introduces variation and irregularities that impede the reliability of analytical outcomes. In descriptive analytics, consistency is pivotal for drawing meaningful interpretations from datasets. The presence of inconsistent data can create challenges in data processing and validation, affecting the overall integrity of the analytical processes. Understanding the origins of inconsistent data and implementing data normalization techniques are key steps in mitigating this limitation.
Biased Data
Biased data reflects disproportionate representation within a dataset, leading to skewed analyses and biased outcomes. In the context of descriptive analytics, recognizing and addressing biases in data is crucial for extracting accurate insights. Biased data can result from various sources, such as sampling methods or data collection processes. By identifying and mitigating biases, analysts can enhance the reliability and relevance of descriptive analytics findings.
Interpretation Challenges
Correlation vs. Causation
Distinguishing between correlation and causation is a prominent interpretational challenge in descriptive analytics. While correlation identifies relationships between variables, causation establishes a cause-and-effect link between them. The distinction is critical for avoiding misleading interpretations and drawing accurate conclusions from analytical findings. Acknowledging this challenge prompts analysts to apply rigorous statistical methods and experimental design to discern causality effectively.
Overfitting
Overfitting occurs when a model captures noise in the data rather than underlying patterns, resulting in poor generalization to new datasets. In descriptive analytics, overfitting can lead to overly complex models that excel in training data but perform poorly in real-world applications. Mitigating overfitting involves balancing model complexity and dataset size, employing regularization techniques, and validating models on independent datasets to ensure robust and accurate outcomes.
Data Privacy Concerns
Data privacy concerns encompass the ethical and regulatory considerations surrounding the collection, storage, and use of sensitive information in analytics. In the age of increasing data breaches and privacy regulations, ensuring data privacy is paramount for maintaining trust and compliance. Descriptive analytics heightens these concerns due to the volume and granularity of data involved, emphasizing the importance of implementing robust privacy measures and transparent data practices to safeguard individual privacy rights and organizational trust.
Future Trends in Descriptive Analytics
data science plays a pivotal role in transforming the landscape of insights and decision-making, paving the way for a more robust and dynamic approach to data analysis. Future Trends in Descriptive Analytics stand at the forefront of this revolution, driving innovation and pushing boundaries in the realm of data science. This section focuses on the evolution of descriptive analytics and its integration with cutting-edge technologies to enhance predictive capabilities and streamline operations. By exploring the future trends in descriptive analytics, we gain a deeper understanding of the ever-evolving data ecosystem and its implications for tech enthusiasts and industry professionals.
AI Integration
Machine Learning Models
Machine Learning Models are a core component of AI Integration, revolutionizing the way data is analyzed and patterns are identified. Their proactive learning ability and adaptability make them a cornerstone of predictive analytics, optimizing performance and accuracy in data-driven decision-making processes. The intricate algorithms and model architectures of Machine Learning Models offer scalability and efficiency, catering to the complex data landscapes encountered in modern data science practices. While Machine Learning Models present significant advantages in enhancing predictive capabilities and automation, they also pose challenges related to interpretability and model explainability.
Natural Language Processing
Natural Language Processing (NLP) leverages computational techniques to interpret and analyze human language, enabling machines to interact with, understand, and generate natural language. Its application in descriptive analytics enhances text mining, sentiment analysis, and language translation, thereby enriching data insights and streamlining communication processes. The versatile nature of NLP makes it a valuable tool in data science, facilitating data processing and insights extraction from unstructured textual data. However, the complexity of language nuances and context poses challenges in accuracy and bias mitigation when implementing NLP solutions.
Image Recognition
Image Recognition technology empowers machines to interpret and process visual information, revolutionizing image classification, object detection, and pattern recognition. Its integration in descriptive analytics enhances data visualization capabilities and enables deeper insights extraction from visual data sources. The robust algorithms and neural network architectures of Image Recognition systems facilitate image understanding and feature extraction, amplifying the utility of image data in data science applications. Despite its advancements in visual data processing, Image Recognition technology faces challenges related to data quality, scalability, and computational resource requirements in implementing sophisticated image analysis solutions.
Real-time Analytics
Stream Processing
Stream Processing plays a crucial role in Real-time Analytics, enabling the continuous processing and analysis of data streams to capture insights and trends in real time. Its low latency and high throughput capabilities make it ideal for monitoring and analyzing dynamic data sources, such as Io T devices and sensor networks. The micro-batch processing and event-driven architecture of Stream Processing systems ensure timely data processing and decision-making, facilitating rapid response to emerging data patterns and events. While Stream Processing excels in real-time data processing and analytics, it also faces challenges in managing data stream fluctuations and ensuring fault tolerance in stream processing pipelines.
Event Detection
Event Detection algorithms detect anomalies and significant events in data streams, providing critical insights into sudden changes and patterns in real-time data. Its adaptive algorithms and detection mechanisms enable proactive monitoring of data streams, alerting users to deviations from expected patterns and thresholds. Event Detection enhances predictive capabilities and operational efficiency by enabling timely interventions and decision-making based on real-time data insights. However, event detection algorithms require robust validation and tuning processes to minimize false positives and optimize detection accuracy in dynamic data environments.
Dynamic Visualization
Dynamic Visualization tools offer interactive and real-time visualization capabilities, transforming complex data sets into intuitive and actionable insights. Their dynamic charts, graphs, and dashboards allow users to explore data trends, correlations, and anomalies, enhancing data comprehension and decision-making processes. Dynamic Visualization enhances data storytelling and communication, enabling stakeholders to gain deep insights into data patterns and relationships. Despite its benefits in data exploration and analysis, Dynamic Visualization tools require optimal data presentation design and user experience considerations to ensure effective data communication and engagement.