Unlocking the Potential of Cloud Data Analytics Tools for Advanced Insights
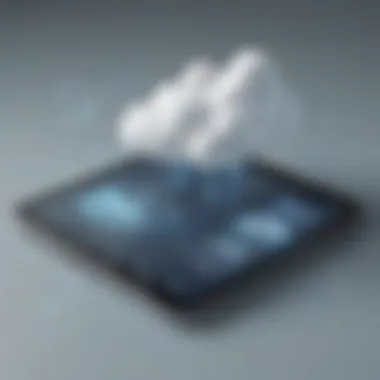
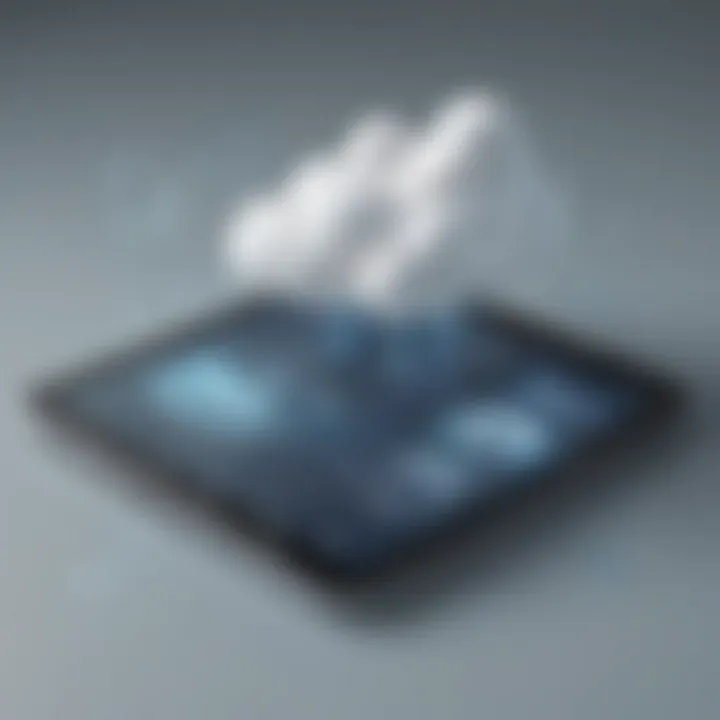
Tech Trend Analysis
Cloud data analytics tools have seen a surge in popularity in recent years with the advancement of technology. Organizations are increasingly adopting these tools to streamline their data analysis processes and gain valuable insights from their vast data repositories. This trend is reshaping the way businesses approach data analysis, leading to more efficient decision-making processes and improved operational outcomes. Consumers are benefiting from these tools by accessing real-time data analytics capabilities that enhance their overall user experience with various products and services. Looking towards the future, the possibilities for cloud data analytics tools are boundless, with upcoming developments expected to revolutionize how data is analyzed and utilized across industries.
Product Reviews
When delving into the realm of cloud data analytics tools, it is crucial to assess the key players in the market. Leading products such as 'Tool A' and 'Tool B' offer a wide range of features and specifications designed to meet the diverse needs of users. 'Tool A' boasts advanced data visualization capabilities, real-time data processing, and seamless integration with popular cloud platforms. On the other hand, 'Tool B' stands out for its robust machine learning algorithms, customizable dashboards, and user-friendly interface. In terms of performance, both tools exhibit high processing speeds and accuracy rates, making them reliable options for data analysis tasks. However, 'Tool A' shines in terms of ease of use and accessibility, while 'Tool B' excels in handling complex data sets efficiently. To aid consumers in their decision-making process, 'Tool A' is recommended for beginners and intermediate users due to its intuitive interface, while 'Tool B' is ideal for advanced users seeking in-depth analytics capabilities. Consider the pros and cons of each tool carefully before choosing the one that aligns best with your data analytics requirements.
How-To Guides
For individuals looking to leverage cloud data analytics tools effectively, a comprehensive how-to guide can serve as a valuable resource. To begin, it is essential to understand the basics of data analysis and the role of cloud-based solutions in this process. Step-by-step instructions can help users navigate through setting up their data analytics environment, importing data sets, and configuring analytics models. Additionally, offering tips and tricks on optimizing data visualization, interpreting analytical results, and establishing data connections can enhance the overall user experience. In the event of encountering technical challenges or errors, a troubleshooting section can provide insights into common issues and solutions, ensuring a seamless user experience throughout the data analytics journey. By following these guidelines diligently, users can make the most of cloud data analytics tools and unlock their full potential for data-driven decision-making.
Industry Updates
The tech industry is dynamic and constantly evolving, with frequent updates and innovations shaping the landscape of cloud data analytics tools. Recent developments have focused on enhancing the scalability and security of these tools, catering to the increasing demand for reliable data analysis solutions. Market trends indicate a growing preference for cloud-based data analytics tools among businesses of all sizes, underscoring the importance of data-driven insights in today's competitive market environment. These tools have a significant impact on businesses and consumers alike, driving operational efficiencies, facilitating resource optimization, and fostering innovation across various sectors. As the industry continues to evolve, staying informed about the latest trends and advancements in cloud data analytics tools is paramount for tech enthusiasts and industry professionals seeking to stay ahead of the curve.
Introduction
In the realm of data analytics, the emergence of cloud-based solutions has revolutionized the way organizations analyze and derive insights from vast datasets. This section serves as a gateway to understanding how cloud data analytics tools are reshaping the landscape of data analysis. By harnessing the power of the cloud, companies can now access scalable and flexible analytics resources, leading to enhanced operational efficiencies and strategic decision-making.
Understanding Cloud Data Analytics
Definition and Scope
One of the fundamental aspects of cloud data analytics is its expansive Definition and Scope. This refers to the parameters and boundaries within which data analysis occurs in a cloud environment, encompassing data collection, processing, and visualization. The key characteristic of Definition and Scope lies in its ability to handle massive volumes of data with efficiency and agility. Its adaptability to varying data types and structures makes it a preferred choice for modern analytical tasks. Despite its advantages in scalability and speed, challenges may arise in ensuring data security and regulatory compliance within this framework.
Benefits of Cloud-based Analytics
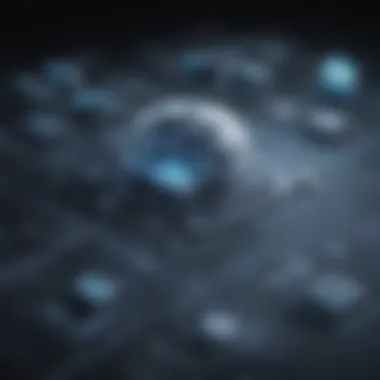
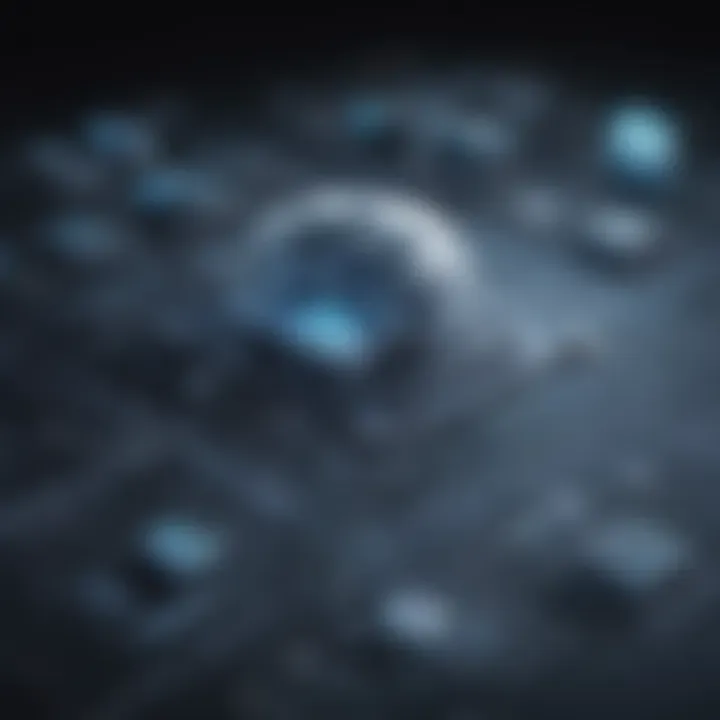
Delving into the Benefits of Cloud-based Analytics opens a world of advantages for organizations looking to leverage data for strategic purposes. The key characteristic of these analytics lies in their ability to provide real-time insights, adaptive data processing, and seamless integration with other data platforms. This unique feature empowers businesses to make data-driven decisions swiftly and effectively. However, while the benefits are numerous, challenges such as data privacy concerns and integration complexities need to be carefully navigated to maximize the full potential of cloud-based analytics.
Impact on Business Operations
The Impact on Business Operations stemming from cloud data analytics is profound and multifaceted. By incorporating cloud analytics tools into their operations, businesses witness improvements in decision-making processes, cost efficiencies, and overall performance. The key characteristic of this impact is the alignment of data insights with strategic objectives, enabling organizations to respond dynamically to market changes and consumer demands. While the advantages are substantial, businesses must address challenges related to data governance, skill gaps, and legacy system integration to fully harness the transformative potential of cloud analytics in their operations.
Key Features of Cloud Data Analytics Tools
When delving into the realm of cloud data analytics tools, understanding the key features is paramount to grasping the essence of this advanced technology. The pivotal aspects of scalability and flexibility stand out as foundational pillars in the efficiency and effectiveness of these tools. Scalability enables the system to handle varying workloads seamlessly, essential in today's dynamic business landscape, while flexibility allows for adaptability to changing data processing needs. These features are crucial in optimizing data analytics capabilities and empowering businesses to make informed strategic decisions based on real-time insights and comprehensive analysis.
Scalability and Flexibility
Adaptive Data Processing
In the context of cloud data analytics tools, adaptive data processing plays a critical role in enhancing overall data processing efficiency. This specific aspect focuses on the system's ability to dynamically adjust its processing capabilities according to the volume and complexity of the data being analyzed. The key characteristic of adaptive data processing lies in its capacity to allocate resources intelligently, ensuring consistent performance even during peak data processing periods. This flexibility is a sought-after attribute in modern analytics tools, catering to the ever-changing demands of data-driven decision-making processes.
Moreover, the unique feature of adaptive data processing lies in its intelligent resource allocation mechanism, which optimizes performance without compromising on data accuracy or processing speed. However, like any technological innovation, adaptive data processing also poses certain challenges, such as the need for sophisticated algorithms to manage resource allocation effectively and potential scalability limitations in extremely high-demand scenarios.
Real-time Monitoring Capabilities
Real-time monitoring capabilities represent another crucial facet of cloud data analytics tools, offering immediate insights into data processes and performance metrics. This characteristic enables users to track data flows, identify anomalies, and respond promptly to emerging trends or issues. The key benefit of real-time monitoring lies in its ability to enhance decision-making by providing up-to-the-minute information for agile and data-driven strategies.
The uniqueness of real-time monitoring capabilities lies in their responsiveness to changes in data patterns and the swift generation of actionable insights. However, incorporating real-time monitoring features may introduce complexities in system configuration and require robust data integration frameworks to ensure seamless connectivity across diverse data sources.
Integration with Big Data Platforms
Integration with big data platforms is a fundamental feature that underpins the comprehensive data analytics ecosystem. This aspect focuses on the seamless connectivity between cloud analytics tools and expansive big data repositories, enabling organizations to leverage diverse data sources for advanced analytics. The key characteristic of this integration lies in its ability to harmonize data from various sources, facilitating comprehensive analysis and holistic insights.
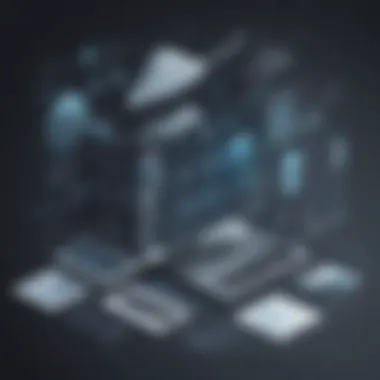
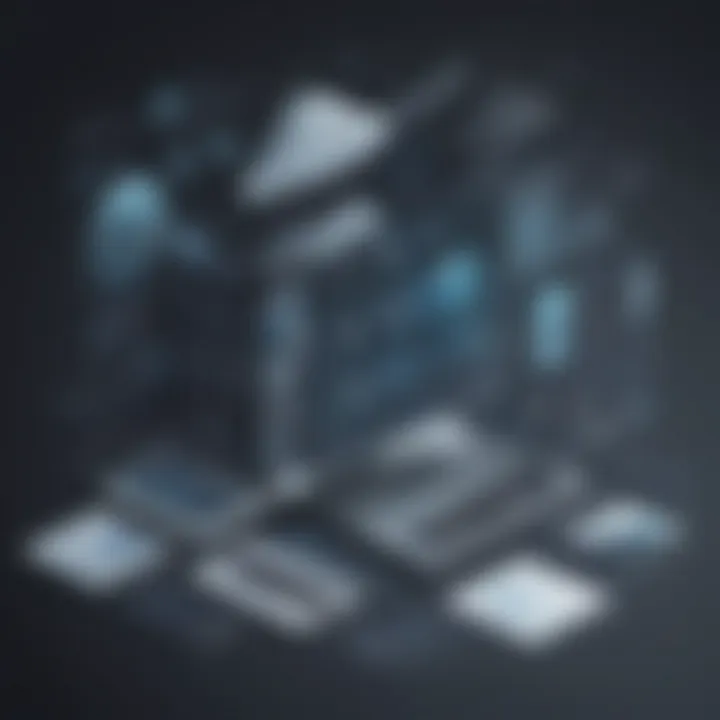
The distinctive feature of integration with big data platforms is its capacity to support extensive data volumes and diverse data types, fostering a comprehensive understanding of organizational data landscapes. Nonetheless, challenges may arise in managing complex data integration processes and ensuring data consistency across disparate platforms, requiring robust data governance frameworks and adept data management strategies.
Popular Cloud Data Analytics Tools
In this section, we delve into the significance of Popular Cloud Data Analytics Tools within the context of this comprehensive guide on cloud data analytics. These tools play a pivotal role in empowering organizations to harness the power of data analytics efficiently and effectively. By analyzing specific elements such as scalability, real-time monitoring capabilities, and integration with big data platforms, readers can gain a deep understanding of how these tools can drive data-driven decision-making processes and enhance operational efficiency.
Amazon Redshift
Data Warehousing Capabilities
Data Warehousing Capabilities are a fundamental aspect of Amazon Redshift, offering organizations the ability to store and manage large volumes of data for analytical purposes. The key characteristic of this feature lies in its robust infrastructure that enables users to optimize query performance and streamline data processing tasks seamlessly. Its unique feature of columnar storage enhances data retrieval speed and overall performance, making it a preferred choice for organizations seeking efficient data warehousing solutions. Despite its advantages in accelerating query performance and facilitating complex analytics, there may be challenges related to managing massive datasets efficiently in this context.
Query Optimization Techniques
Query Optimization Techniques in Amazon Redshift focus on enhancing query performance through intelligent optimization algorithms. By automatically tuning queries and indexing data for faster retrieval, this feature contributes significantly to the overall efficiency of data processing and analysis. Its key characteristic lies in its adaptive query optimizer that adapts to changing workloads, ensuring optimal performance under varying conditions. The unique feature of Query Optimization Techniques lies in its ability to reduce query execution time and boost overall system efficiency. However, complexities may arise in fine-tuning optimization parameters to align with specific workload requirements.
Integration with AWS services
Integration with AWS services is a notable aspect of Amazon Redshift, allowing seamless connectivity with a wide range of AWS tools and services for enhanced data processing and analysis capabilities. The key characteristic of this integration is its ability to leverage the scalability and flexibility of AWS resources, enabling organizations to scale their analytics operations effortlessly. The unique feature of this integration lies in its cost-effective utilization of AWS services, providing a comprehensive analytics environment within a single platform. While the advantages include streamlined data workflows and efficient resource allocation, potential challenges may arise in optimizing data transfer speeds and managing interdependent AWS services effectively.
Implementation Strategies for Cloud Data Analytics Tools
In the realm of cloud data analytics, effective implementation strategies are paramount for maximizing the benefits of data analysis tools. These strategies play a crucial role in enhancing operational efficiency, decision-making processes, and overall business outcomes. By implementing cloud data analytics tools strategically, organizations can leverage the power of data to gain insightful and actionable intelligence. The implementation strategies encompass a wide array of considerations, ranging from data security to scalability and seamless integration with existing systems. In this article, we delve into the importance of implementing cloud data analytics tools thoughtfully to harness the full potential of data-driven insights.
Data Security Considerations
Encryption Protocols
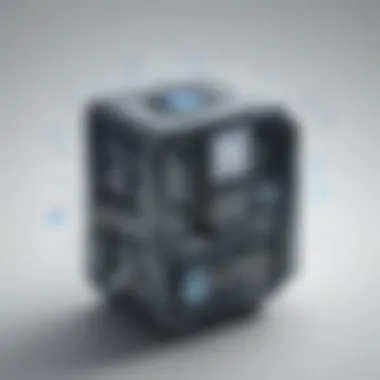
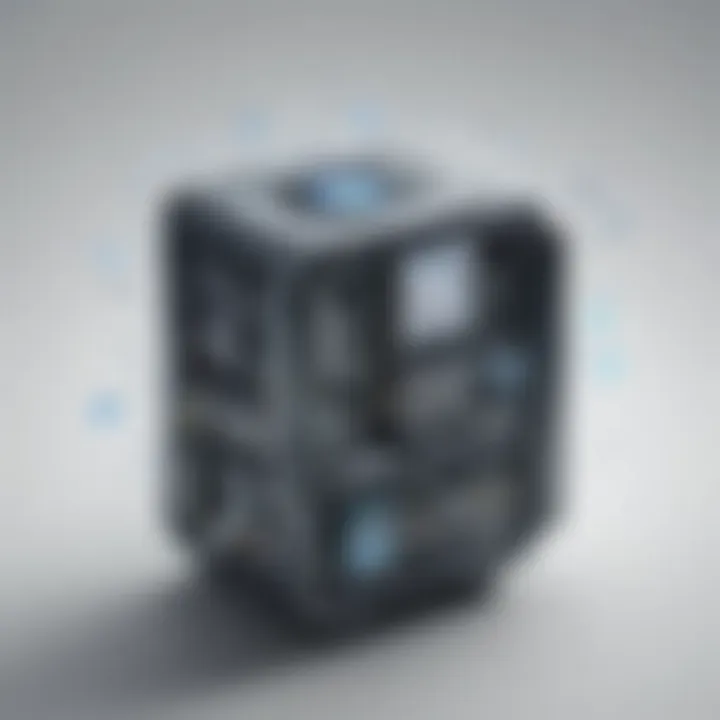
Encryption protocols represent a critical aspect of data security in cloud data analytics. These protocols utilize cryptographic algorithms to encode sensitive information, ensuring that data remains confidential and secure during transmission and storage. The key characteristic of encryption protocols lies in their ability to transform plaintext data into encrypted format, making it indecipherable to unauthorized individuals. This encryption method is a popular choice for safeguarding data integrity and confidentiality in the context of this article.
The unique feature of encryption protocols lies in their versatile nature, allowing organizations to tailor encryption schemas to align with specific security requirements. While encryption enhances data privacy and mitigates cybersecurity risks, it also introduces challenges such as performance overhead and key management complexities. Despite these drawbacks, the advantages of encryption protocols in ensuring data confidentiality make them indispensable for maintaining robust data security in cloud data analytics.
Access Control Mechanisms
Access control mechanisms play a pivotal role in regulating user permissions and defining levels of data access within cloud data analytics environments. These mechanisms enforce security policies by restricting unauthorized users from accessing sensitive data, thereby reducing the risk of data breaches and unauthorized disclosures. The key characteristic of access control mechanisms is their ability to authenticate user identities and assign appropriate access rights based on predefined rules and policies.
Access control mechanisms serve as a beneficial choice for controlling data access and protecting confidential information in this article. The unique feature of access control mechanisms lies in their granular control capabilities, enabling organizations to tailor access privileges to different user roles and responsibilities. While access control enhances data security, it may introduce complexities in managing access permissions and maintaining access logs effectively. Nonetheless, the advantages of access control mechanisms in safeguarding data integrity and confidentiality are indispensable for securing cloud data analytics environments.
Compliance with Data Regulations
Compliance with data regulations is a critical aspect of data governance in cloud data analytics. Organizations must ensure that their data analytics practices align with regulatory requirements and industry standards to avoid legal repercussions and data privacy breaches. The key characteristic of compliance with data regulations lies in the adherence to laws such as GDPR, HIPAA, and CCPA to protect consumer privacy rights and uphold data protection principles.
Compliance with data regulations is a popular choice for maintaining regulatory compliance and fostering trust among data subjects in this article. The unique feature of compliance with data regulations lies in its proactive approach to data governance, emphasizing transparency, accountability, and data subject rights. While compliance requirements may entail additional overhead in terms of documentation and auditing, the advantages of complying with data regulations far outweigh the risks posed by non-compliance, ensuring ethical data handling practices and regulatory adherence in cloud data analytics.
Trends Shaping the Future of Cloud Data Analytics
Cloud data analytics tools are at the forefront of transforming how businesses analyze and utilize data. As technology continues to evolve, understanding the trends shaping the future of cloud data analytics becomes crucial. The integration of Artificial Intelligence (AI) is a key trend that is revolutionizing the field, offering advanced capabilities to extract insights from vast datasets. AI is paving the way for more efficient data processing, enhanced decision-making processes, and precise predictive analytics. Embracing AI in cloud data analytics opens up new possibilities for businesses to drive innovation and stay competitive in today's rapidly changing digital landscape. Furthermore, the fusion of AI with cloud data analytics fosters a deeper understanding of consumer behaviors, enabling organizations to tailor their products and services according to market demands. This synergy propels companies towards agility and strategic growth, positioning them as industry leaders in the age of digital transformation.
Artificial Intelligence Integration
Machine Learning Algorithms:
Machine learning algorithms play a pivotal role in enhancing data analysis capabilities within cloud environments. These algorithms utilize statistical models and computational algorithms to enable systems to learn from data patterns, make predictions, and adapt to changing scenarios. One key characteristic of machine learning algorithms is their ability to discern intricate patterns within datasets that human analysis often overlooks. This capability empowers businesses to extract meaningful insights, predict future trends, and optimize decision-making processes effectively. The unique feature of machine learning algorithms lies in their adaptability and scalability, making them a popular choice for organizations seeking to leverage data analytics tools efficiently. While machine learning algorithms offer significant advantages in enhancing data interpretation and guiding strategic decisions, challenges such as model complexity and data bias need to be carefully addressed to maximize their potential impact.
Cognitive Analytics Applications:
Cognitive analytics applications mark a significant shift in how businesses harness the power of data intelligence. By incorporating principles of psychology and cognitive science, these applications enable data analytics tools to emulate human thought processes, perception, and learning mechanisms. The key characteristic of cognitive analytics applications is their ability to unravel complex data sets, identify correlations, and generate insightful recommendations autonomously. This innovative approach streamlines data interpretation, accelerates problem-solving, and enriches business intelligence capabilities. The unique feature of cognitive analytics applications lies in their intuitive nature, making data analytics more accessible to a broader audience within organizations. While these applications offer enhanced data processing and interpretation capabilities, potential challenges related to data privacy, ethical considerations, and algorithm transparency must be carefully navigated to ensure responsible and effective implementation.
Predictive Modeling Techniques:
Predictive modeling techniques represent a progressive methodology for forecasting future trends and outcomes based on historical data patterns. These techniques utilize statistical algorithms, machine learning models, and data mining processes to make informed predictions and optimize decision-making processes. The key characteristic of predictive modeling techniques is their ability to provide actionable insights, anticipate market trends, and mitigate potential risks in real-time. By leveraging historical data and advanced analytical tools, organizations can enhance forecasting accuracy, streamline resource allocation, and drive operational efficiency. The unique feature of predictive modeling techniques lies in their adaptability to dynamic business environments, enabling organizations to proactively respond to market fluctuations and capitalize on emerging opportunities. While predictive modeling techniques offer significant benefits in strategic planning and risk management, considerations such as data quality, model validation, and interpretation nuances must be carefully evaluated to ensure reliable and actionable insights.