Enterprise AI Companies: Key Players and Innovations
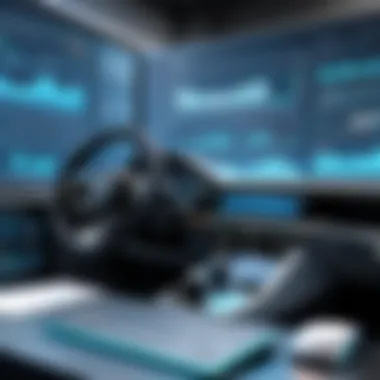
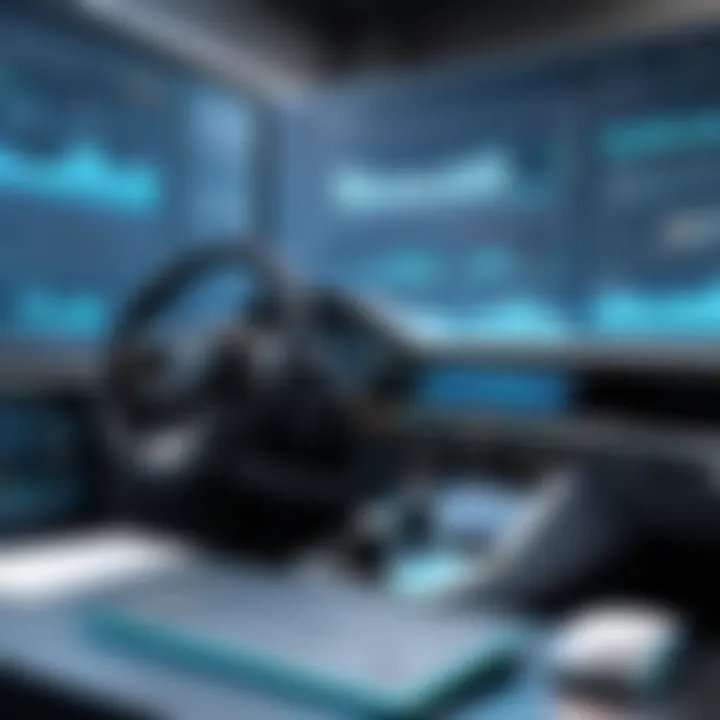
Intro
In the rapidly evolving world of business, enterprise AI companies are forging new paths that fundamentally change the way we work and think. This landscape is not just a passing trend; it represents a seismic shift in how decisions are made, workflows are optimized, and ultimately, how value is generated in various sectors.
The ever-increasing velocity of data, fueled by digital transformation and the Internet of Things (IoT), is the beating heart of modern enterprises. With artificial intelligence at the helm, organizations can harness this data, drawing actionable insights that were once buried beneath piles of information. Here, we are not just talking about automating tasks; we are discussing a complete overhaul of operational methodologies.
In the pages that follow, we'll navigate through the key players driving these changes, delve into the technology that enables them, and analyze real-world applications that illustrate the transformative potential of AI. Ethical considerations will also come into play, as businesses grapple with the implications of advanced technologies—balancing innovation with responsibility. This exploration not only encompasses current standings but also anticipates future trajectories for enterprise AI.
As we embark on this journey, it’s vital to understand that the importance of enterprise AI cannot be overstated. It’s not merely about improving efficiency; it’s about redefining the very fabric of business strategies and customer engagement. With this in mind, let’s dive headfirst into the first section, focusing on current trends shaping the enterprise AI landscape.
Preamble to Enterprise AI
In today's tech-driven landscape, Enterprise AI has carved a prominent niche, influencing how organizations strategize and operate on a multifaceted level. This segment delves into what exactly constitutes Enterprise AI, exploring its foundations and spotlighting its significance for modern businesses. The very definition sets the stage for understanding its far-reaching implications.
Definition of Enterprise AI
Enterprise AI refers to the deployment of artificial intelligence technologies at scale within an organization, aiming to enhance efficiency, streamline operations, and improve decision-making processes. This is not just about employing basic machine learning tools; it embraces sophisticated algorithms, natural language processing, and data analytics to tackle complex challenges faced by large enterprises. Essentially, it’s about embedding AI deeply into the business fabric to drive agility and innovation.
To put it simply, think of it as a modern toolkit allowing companies to sift through mountains of data, automating numerous tasks while deriving actionable insights. Just as you can’t have a well-run bakery without the right equipment, businesses today can't thrive without harnessing meaningful AI tools.
Importance in Modern Business
The importance of Enterprise AI in contemporary business cannot be overstated. In a nutshell, it equips organizations with the means to navigate a fast-paced environment, make informed decisions, and cater to the rapidly evolving demands of consumers. Here are some key points to consider:
- Enhanced Efficiency: With AI taking on time-consuming tasks, teams can shift their focus to strategic initiatives.
- Data-Driven Insights: Enterprises can harness a wealth of data, transforming it into insights that guide their next steps.
- Personalized Customer Experiences: AI enables businesses to tailor offerings to individual preferences, shifting from one-size-fits-all approaches.
- Competitive Advantage: Companies leveraging AI often find themselves a step ahead, as they can respond more swiftly to market shifts.
"Adopting AI technologies can differentiate the wheat from the chaff in today’s competitive marketplace."
In recap, Enterprise AI serves as a catalyst for transformation in businesses. Rather than simply reacting to changes, companies employing AI are proactively shaping their futures. As organizations continue to integrate these tools, the landscape promises to evolve further, making it critical to understand the fundamentals of this transformative technology.
Key Players in the Field
In the realm of enterprise AI, understanding who the significant players are is crucial for grasping the landscape. The companies that dominate this industry shape technology trends, influence market directions, and define standards in the AI domain. Recognizing these key players helps businesses identify potential partnerships, gain insights into competitive advantages, and keep abreast of cutting-edge innovations.
Industry Giants
When we think of industry giants in enterprise AI, several names stand out. Companies like Google, Microsoft, and IBM have established themselves at the forefront of this technological revolution. They offer robust AI platforms that serve various industries.
- Google Cloud AI harnesses an array of tools, such as AutoML, that allow businesses to create custom machine learning models, catering to specific needs without extensive expertise.
- Microsoft Azure AI provides a suite of services, from cognitive capabilities to advanced analytics, making it easier for enterprises to integrate AI into their existing workflows.
- IBM Watson is noteworthy with its foundations in natural language processing. Organizations leverage Watson for customer support, data analytics, and healthcare solutions.
These giants not only invest heavily in research but also acquire smaller firms to bolster their offerings. Their scale and resources enable them to push the envelope on what’s possible with AI. Moreover, partnerships with other tech firms and academic institutions have fostered innovation and accelerated development in AI technologies.
"The interplay between industry giants and emerging startups can create a dynamic ecosystem that nurtures innovation and facilitates knowledge transfer."
Emerging Startups
While the giants dominate, a wave of emerging startups is reshaping the enterprise AI landscape. These companies often operate with agility and a fresh perspective, addressing niche problems and unmet needs in the market. For example, firms like UiPath in Robotic Process Automation and DataRobot in automated machine learning bring specialized solutions to the table.
- UiPath focuses on enhancing productivity through robotic process automation, allowing companies to automate repetitive functions while optimizing human resource allocation.
- DataRobot democratizes AI by enabling companies, regardless of their size or expertise, to harness machine learning without understanding the underlying complexities.
Startups can outpace their larger counterparts by quickly adapting to market demands and customer feedback. Many of these companies have demonstrated explosive growth, attracting significant venture capital investments, which enables them to innovate faster.
All in all, recognizing the blend of established industry giants and nimble startups provides a complete picture of the enterprise AI landscape. Each plays a crucial role in driving advancements, challenging conventional practices, and fostering a culture of innovation. This understanding is not only beneficial for tech enthusiasts but also invaluable for industry professionals looking to navigate the ever-evolving enterprise AI ecosystem.
Technological Innovations Driving Enterprise AI
The realm of enterprise AI rests firmly on a foundation of technological innovations. These advancements shape how businesses harness artificial intelligence, making operations not just effective but also more intelligent and responsive. Understanding these technologies unlocks insights into how companies can exploit AI's capabilities to drive growth, improve efficiency, and adapt to fast-changing market dynamics.
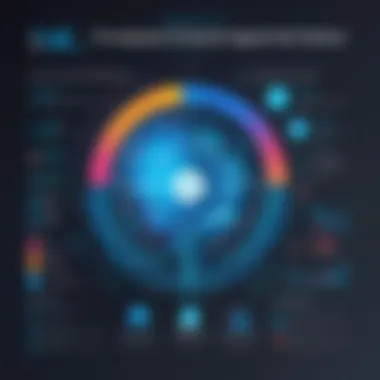
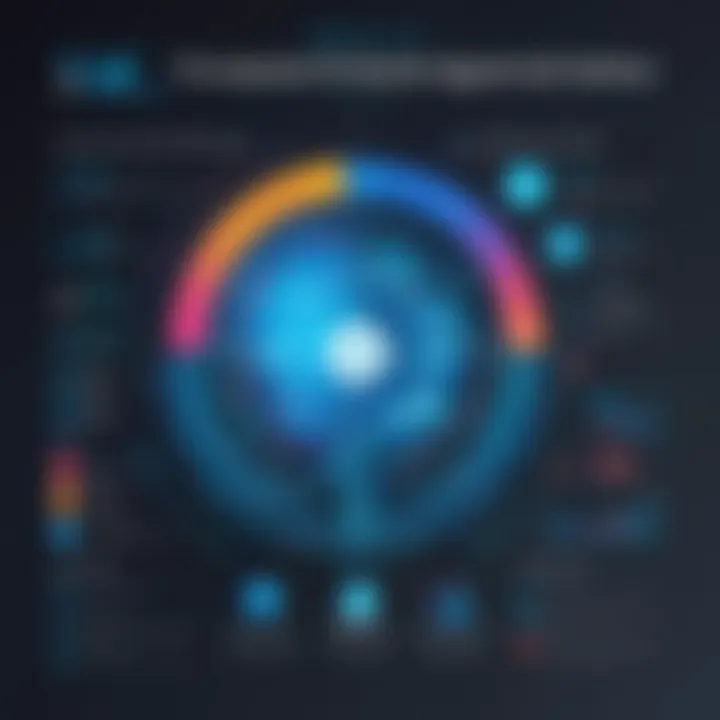
Machine Learning Algorithms
At the core of many AI applications are machine learning algorithms. These are the tools through which systems learn from data inputs and make informed decisions. Imagine a retail company using customer purchase data to predict future buying behavior. By employing algorithms like regression or clustering, they can tailor marketing strategies accordingly. This ability to draw actionable insights from data is paramount.
The growth of machine learning has seen significant innovations in algorithms:
- Supervised Learning: Trains models on labeled datasets. This method provides a clear path from input to output, enabling businesses to make predictions that are often quite accurate.
- Unsupervised Learning: Focuses on unlabeled data and enables the identification of hidden patterns, beneficial for market segmentation or discovering new product features.
- Reinforcement Learning: This technique learns from past actions and rewards, ideal for dynamic environments like gaming or robotics.
The versatility of these algorithms means they can be adapted for various applications. Just think about fraud detection in finance. Institutions deploy machine learning to sift through transactions in real-time, notifying them of suspicious activities based on learned behavior patterns.
Natural Language Processing
Natural Language Processing (NLP) enables machines to understand and interact using human language. This technology is changing the game in customer service, providing tools for chatbots that can handle queries without human intervention. For instance, a bank may utilize NLP to allow customers to check balances or transfer funds just by chatting in their preferred language.
NLP incorporates various approaches:
- Sentiment Analysis: Gauges public sentiment through reviews or social media, hence steering marketing efforts.
- Text Classification: Categorizes large volumes of text, useful for tagging emails, for example.
- Named Entity Recognition: Identifies names, locations, or organizations in texts, assisting in data extraction.
The implications for businesses are profound. Firms can improve customer interactions, often increasing satisfaction where a human touch may falter. Automated insights can help understand trending topics, leading to quicker pivots in strategy.
Robotic Process Automation
Robotic Process Automation (RPA) is another innovative pillar of enterprise AI technology. It allows companies to automate mundane tasks that are typically repetitive and rule-based, such as data entry or invoice processing. By delegating these tasks to software bots, staff are freed up to engage in more value-added activities, effectively boosting productivity.
RPA can be utilized for:
- Financial Reporting: Automating data collection from multiple sources, providing faster and more accurate reports.
- Customer Onboarding: Streamlining processes through automatic data verification, ensuring customers are registered quickly and without hassle.
- Supply Chain Management: Monitoring inventories in real-time to trigger automatic reorders, thus keeping just the right stock.
This technology doesn't just save time; it reduces errors and enhances accuracy in tasks often prone to human mistakes. Unquestionably, RPA enables firms to operate more smoothly while remaining competitive.
"AI has the potential to enhance efficiency across many sectors, fundamentally changing how organizations operate and strategize."
In summary, the technological innovations represented by machine learning algorithms, natural language processing, and robotic process automation are at the forefront of driving enterprise AI. They provide tools for deeper insights, improved interactions, and enhanced operational efficiency, thus facilitating a transformative journey for modern enterprises.
Applications of AI Across Industries
The integration of artificial intelligence in various sectors marks a significant turning point in how businesses function. AI technologies are not just buzzwords; they drive efficiency, enhance decision-making, and offer tailored experiences that resonate with consumers and stakeholders alike. Understanding the myriad applications of AI helps demystify its impact on industries ranging from healthcare to retail. Here, we’ll briefly touch on some key sectors and highlight how AI is reshaping the landscape.
Healthcare Transformations
In the realm of healthcare, AI is like a breath of fresh air. It helps hospitals and clinics optimize operations and enhance patient care through predictive analytics, improving diagnosis rates and treatment plans. For instance, AI algorithms analyze vast datasets, identifying patterns that can lead to early detection of diseases.
Some specific applications include:
- Predictive Analytics: Anticipating patient admissions and outcomes based on historical data.
- Personalized Medicine: Tailoring treatment plans that fit the individual characteristics of patients.
- Telemedicine: Using AI-powered chatbots to screen patients and provide initial consultations, making healthcare more accessible.
“AI can sift through mountains of data, uncovering insights that would take humans much longer to visualize.”
These applications not only improve efficiency but bring a level of precision that can potentially save lives. Furthermore, as this technology continues to evolve, we can expect even broader implementations, leading to reduced healthcare costs and improved patient satisfaction.
Financial Services Enhancement
When it comes to financial services, AI is a game changer. Financial institutions leverage AI to enhance security, streamline operations, and augment customer service. Algorithms built for fraud detection can analyze transaction patterns much quicker than any human could. The result is a robust defense mechanism against fraudulent activity.
Key advancements in this sector include:
- Risk Assessment: Automating credit scoring by analyzing a customer’s financial behavior and transaction history.
- Algorithmic Trading: Real-time data analysis to guide investment strategies.
- Chatbots for Customer Support: Providing clients with immediate responses to inquiries, thus improving customer satisfaction.
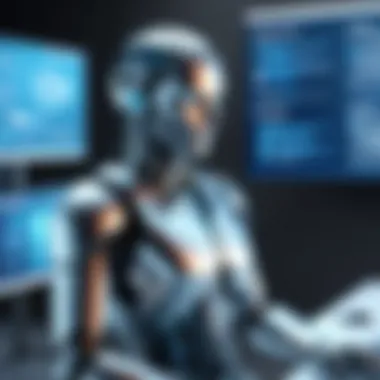
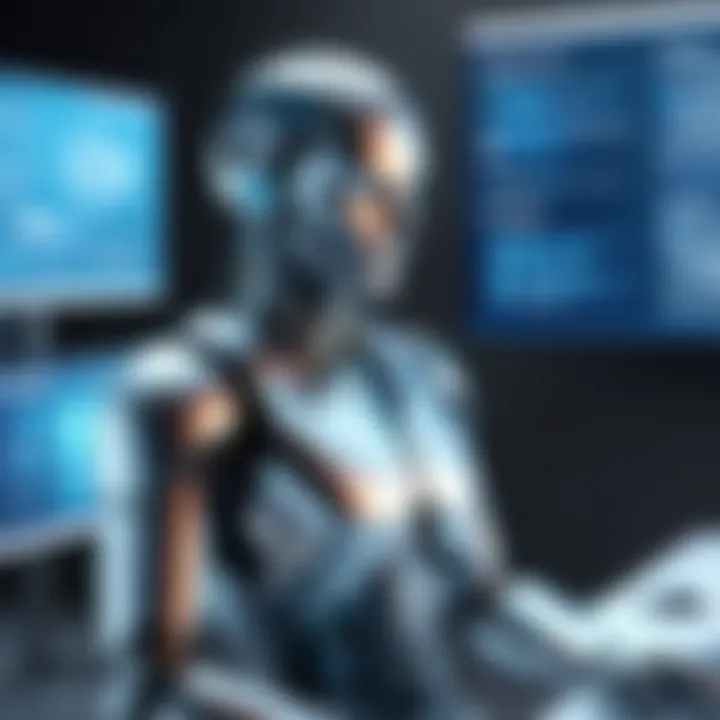
AI's influence on the financial sector isn't just about efficiency; it's also about cultivating better relationships with clients through customized offerings based on real-time analytics. As firms work towards integrating machine learning models, the future looks promising for enhanced predictive capabilities.
Retail and Customer Experience
In retail, AI has become the secret sauce for business growth. It personalizes shopping experiences, predicts inventory needs, and enhances the overall customer journey. With consumer preferences constantly shifting, AI provides retailers with state-of-the-art tools to stay ahead of the curve.
Some significant uses in retail include:
- Recommendation Engines: Suggesting products based on past purchases and browsing behavior, which boosts sales.
- Inventory Management: Predicting stock levels based on consumer trends, thus minimizing excess inventory.
- In-store Virtual Assistants: Enhancing the shopper's experience through interactive queries and navigation help.
With these implementations, retailers not only enhance sales figures but also cultivate loyalty among their consumers by offering experiences tailored to individual preferences.
In summary, AI's applications across different industries highlight its importance as a transformative technology. By adopting AI, sectors can achieve more than operational efficiency; they can also create lasting relationships with clients and patients, adapting to their needs like never before.
Case Studies: Success in Action
When examining the landscape of enterprise AI, specific implementations reveal just how transformative this technology can be across various industries. Case studies serve as compelling narratives that not only spotlight significant achievements but also offer a practical guide for other companies venturing into AI adoption. They embody the blend of ambition and strategic foresight that defines successful AI projects. As businesses grapple with the complexities of AI, case studies become essential in illustrating the practical benefits, pitfalls, and overall importance of embracing artificial intelligence.
Noteworthy Implementations
Several enterprises have effectively leveraged AI technology to challenge conventional methods and significantly enhance their operational processes. A standout example can be found in the healthcare sector, where IBM’s Watson Health has revolutionized diagnostics. By analyzing vast amounts of medical data, Watson provides insights that support clinical decision-making. This is not merely an improvement in efficiency; it directly influences patient outcomes, demonstrating how AI can shift the paradigm in critical areas like health management.
In the world of retail, Amazon stands tall with its dynamic inventory management. By utilizing machine learning algorithms, the company optimizes stock levels based on consumer trends. This has led to a reduction in both overstock and understock situations, demonstrating how companies can streamline operations effectively. Not only does it enhance customer satisfaction, but it also drives down costs, making it a win-win for both the business and the consumer.
Another notable case comes from the finance sector. JPMorgan Chase employed AI to automate document review processes, significantly decreasing the time needed for compliance checks. Such implementations underline AI's capability to process information faster than human counterparts, allowing the workforce to focus on more complex, value-adding tasks. This example highlights the importance of automation in enhancing productivity and ensuring compliance without sacrificing quality.
"AI isn't just a tool; it's a catalyst for change. The right implementations can redefine industry standards."
Lessons Learned
Delving into these case studies, several lessons become apparent.
- Integration is Key: Successful AI adoption doesn't happen in a vacuum. Companies need to ensure that AI solutions integrate seamlessly with existing systems. For instance, JP Morgan's success stemmed from using AI to enhance rather than replace human processes, fostering collaboration.
- Data Quality Matters: AI’s effectiveness hinges on the quality and quantity of data. Inaccurate or insufficient data leads to skewed insights. IBM Watson’s reliance on comprehensive and diverse datasets illustrates that feeding AI with robust data fortifies its predictive capabilities.
- Change Management: Resistance often arises during transitions to AI. Companies need to engage employees, demonstrating how AI will enhance their roles rather than replace them. Amazon prioritized training programs to ensure their workforce embraced new technologies.
- Iterative Approach: Many enterprises learned that deploying AI isn’t a one-off event. Continuous assessment and adjustment based on real-world results are vital. These iterative improvements allow businesses to refine their AI models, ensuring that they stay relevant amid rapidly evolving market conditions.
Challenges and Limitations
Discussing the challenges and limitations in enterprise AI is crucial for understanding its true potential and boundaries. Businesses often seek to leverage this technology to gain a competitive edge, yet they face significant hurdles. Recognizing these issues early on can save companies time, money, and frustration in their quest for AI integration. This section delves into two primary challenges: data privacy concerns and integration difficulties.
Data Privacy Concerns
Data privacy is increasingly at the forefront of conversations around enterprise AI. With businesses accumulating vast amounts of data, the responsibility to protect sensitive information has never been greater. Companies must navigate a labyrinth of regulations such as the General Data Protection Regulation (GDPR) in Europe and the California Consumer Privacy Act (CCPA) in the United States.
To truly grasp the significance of this challenge, consider these points:
- Regulatory Compliance: Organizations must stay compliant with these regulations, which can change rather quickly. Non-compliance can lead to hefty fines and damage to reputation.
- Customer Trust: If customers feel that their data isn't secure, they might be hesitant to engage with a company. Trust is invaluable, and a single breach can unravel years of goodwill.
- Implementation of Security Measures: Integrating robust security measures often comes at an additional cost. Businesses need to invest in technology and training to ensure employees understand how to handle sensitive data properly.
"The protection of data is an ongoing struggle, requiring both technological upgrades and a culture shift within organizations."
The implications of poor data privacy practices extend beyond legal consequences; they can erode consumer confidence and cripple a company's market standing.
Integration Difficulties
Integrating AI systems into existing business operations can be as tricky as navigating a minefield. While the potential for enhanced efficiency and decision-making is alluring, the road to integration is fraught with challenges. Here are some notable obstacles:
- Legacy Systems: Many organizations still rely on outdated technology that is not compatible with newer AI solutions. This can lead to costly overhauls or incomplete integrations.
- Skill Gaps: The tech landscape is evolving faster than many professionals can keep up with. A lack of skilled personnel can stymie projects, as companies struggle to find experts who can implement and manage AI systems.
- Cultural Resistance: Sometimes, the biggest hurdle is the culture within a business. Employees may resist changes or feel threatened by AI, fearing job losses or additional pressures in their roles.
By acknowledging and addressing these integration challenges, businesses can strategically approach their AI initiatives, aligning them with long-term goals without falling into common traps.

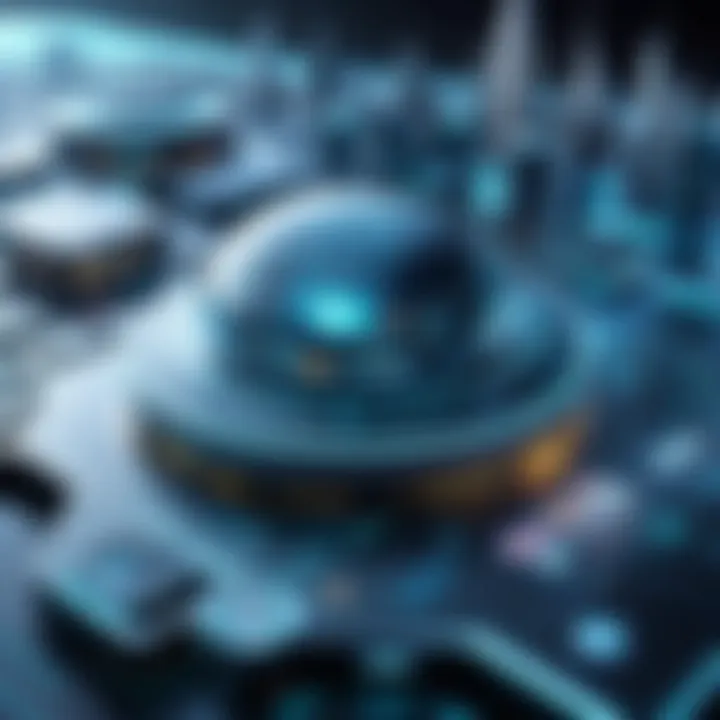
Ethical Considerations in AI Deployment
The rapidly evolving landscape of enterprise AI presents not just a wealth of opportunities but also a myriad of ethical dilemmas that demand careful consideration. As organizations increasingly rely on AI to streamline processes and enhance decision-making, they find themselves at the intersection of technology and morality. This section delves into the critical ethical aspects of AI deployment, underscoring the importance of addressing biases and ensuring transparency.
Bias and Fairness
At the heart of AI ethics lies the concept of bias. The potential for AI systems to perpetuate or even exacerbate existing biases is a mounting concern. AI models are typically trained on historical data, and if this data contains biases—whether related to race, gender, or socioeconomic status—those biases may be unwittingly reinforced in the system's output. This not only hampers the fairness of decisions made by AI but could also lead to legal ramifications for organizations.
To counteract this, companies should prioritize diverse datasets that accurately reflect the representative demographics of their target audience. It's imperative to continually evaluate AI systems for possible biases, employing methodologies such as algorithm auditing and fairness assessments. Doing so ensures that all stakeholders are treated fairly, fostering trust and acceptance among users.
"The greatest risk is not that AI will become conscious and decide to harm us, but that it will replicate the biases of its creators."
Moreover, training staff to recognize and rectify their own biases can have a substantial impact on the development process of AI technologies. Cultivating an inclusive company culture goes a long way in advancing fairness in AI deployment. Organizations should create awareness and initiate discussions on bias, championing ethics training as an integral part of the AI development framework.
Transparency and Accountability
Transparency in AI systems is not just a buzzword; it’s a cornerstone principle that promotes accountability. Businesses utilizing AI must be candid about how decisions are made. Understanding AI workflows is critical for both users and stakeholders. If a company relies on an AI algorithm to assess a loan application, for instance, it’s crucial they explain how inputs lead to outputs or decisions.
Lack of transparency can lead to skepticism and distrust from customers, which in turn can tarnish a company's reputation. Implementing measures like explainable AI, where algorithms can elucidate their reasoning processes, can bridge this gap. Moreover, accountability structures need to be in place—who bears responsibility when an AI system makes a mistake?
Creating a culture of accountability involves documenting AI development processes and outcomes rigorously. This could include establishing oversight committees that review AI systems regularly, focusing on risk management and compliance with ethical standards.
Ultimately, prioritizing transparency and accountability not only builds trust but also positions companies as leaders in ethical AI deployment.
In sum, as enterprise AI continues its upward trajectory, addressing ethical considerations is not just essential; it’s a necessity. By actively tackling bias and ensuring transparency, organizations can responsibly harness the power of AI to create fair and equitable outcomes.
Future Trends in Enterprise AI
As the digital age continues to evolve, enterprise AI stands at the forefront of innovation and strategic development within numerous industries. Understanding the future trends in this domain not only helps organizations stay competitive but also sheds light on potential challenges and opportunities that lie ahead. The increasing reliance on AI technologies highlights the urgency for businesses to adapt and harness the advantages offered by advancements in this field. Several key elements will shape the future of enterprise AI, and they're worth looking into.
Advancements in AI Technologies
The landscape of AI technology is changing rapidly. From enhanced machine learning algorithms to improvements in natural language processing, the technological advancements are nothing short of remarkable. Here are some notable trends:
- Deep Learning: The sophistication of deep learning models is growing, allowing for more accurate predictions and classifications. Techniques such as reinforcement learning are becoming pivotal in developing applications like autonomous systems and robotics.
- Federated Learning: Privacy has emerged as a major concern, and federated learning addresses this by allowing machine learning models to be trained across multiple decentralized devices without sharing sensitive data. This method not only safeguards privacy but also leads to more robust AI models.
- AI Ethics and Governance: As AI technologies advance, the need for proper governance frameworks is crucial. Developing ethical guidelines for AI deployment will help organizations navigate complex moral landscapes, ensuring fair outcomes and increasing public trust.
- Edge AI: The shift towards edge computing is significant. By processing data closer to its source, businesses can achieve lower latency and improved response times, which is particularly vital for applications in sectors like healthcare and manufacturing.
"The continued evolution of AI technologies will redefine operational capabilities, pushing companies to rethink their strategies."
Expected Market Growth
The enterprise AI market is anticipated to witness substantial growth. Various factors contribute to this bullish outlook:
- Increased Investments: Venture capitalists and companies alike are pouring funds into AI startups and projects, fueling further innovation and development. Major players like Microsoft and Google are continuously investing in AI research, pushing boundaries and setting new standards.
- Demand for Automation: With growing labor costs and the need for efficiency, businesses are turning to AI for automating routine tasks. From customer service chatbots to advanced data analytics, automation not only cuts costs but also improves accuracy and speeds up processes.
- Widespread Adoption Across Industries: AI is no longer limited to tech companies. Industries such as healthcare, finance, and agriculture are embracing AI solutions. For example, predictive analytics is being used in healthcare for patient diagnosis and treatment recommendations, illustrating the versatility and applicability of AI.
- Global Business Environment: The COVID-19 pandemic has accelerated the digital transformation of enterprises forcing them to adapt to new technologies including AI to remain competitive. As organizations continue investing in digital capabilities, the demand for AI solutions will grow correspondingly.
The convergence of these trends surrounding both advancements in AI technologies and market growth is paving the way for significant shifts within the enterprise AI arena. Businesses that stay ahead of the curve will be better positioned to leverage these changes, fostering innovation and enhancing overall operational efficiency.
Closure
In wrapping up our exploration of enterprise AI companies, it becomes clear that understanding this landscape is pivotal for stakeholders across various sectors. The integration of artificial intelligence into business operations not only streamlines processes but also unlocks unprecedented opportunities for innovation. As enterprises harness the capabilities of AI, they find themselves not just in a race for efficiency, but also in a quest for enhanced decision-making and competitive edge.
Recapitulation of Insights
Reflecting on the insights discussed, several key points emerge:
- Industry Dominance: Major players like Google and IBM continue to lead the charge, driving advancements in AI technologies and setting benchmarks in performance and reliability.
- Startup Ecosystem: Emerging startups are injecting fresh ideas into the market, offering niche solutions that cater to specific industry needs.
- Technological Foundations: From machine learning to natural language processing, the underlying technology continues to evolve, enabling more sophisticated applications across industries like healthcare, finance, and retail.
- Case Studies: Taking a closer look at case studies reveals not only success stories but also pivotal lessons that emphasize the transformative processes of AI integration.
- Ethical Considerations: The topic of bias and fairness surfaces repeatedly, underlining the importance of responsible AI deployment in mitigating risks associated with data privacy and algorithmic discrimination.
This synthesis offers a comprehensive viewpoint; however, it serves only as a launchpad for further inquiry into the evolving dynamics of enterprise AI.
Final Thoughts on the Future of Enterprise AI
As we peer into the future, it's evident that enterprise AI will keep shaping the landscape of business operations. The anticipated market growth signifies that companies will increasingly prioritize AI solutions as a cornerstone of their strategic plans.
Furthermore, advancements in AI technology promise more robust, user-friendly tools that facilitate seamless integration into existing systems. Considering this trajectory, businesses that embrace AI proactively will likely reap the rewards, while those that hesitate may find themselves sluggish in comparison.
Ultimately, enterprise AI's ongoing evolution beckons not only for adaptation but also for a conscientious approach, ensuring that the benefits distributed align with the moral imperatives of fairness and accountability. The future holds boundless prospects, yet navigating its contours with due diligence will be key for sustained success.