Exploring the Cutting-Edge: A Deep Dive into Distributed Streaming Platforms
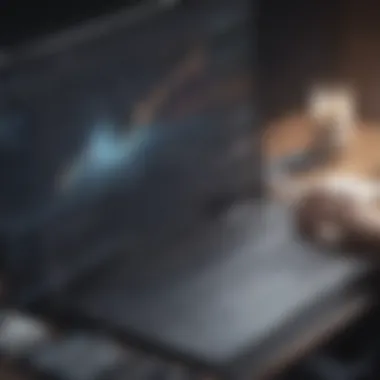
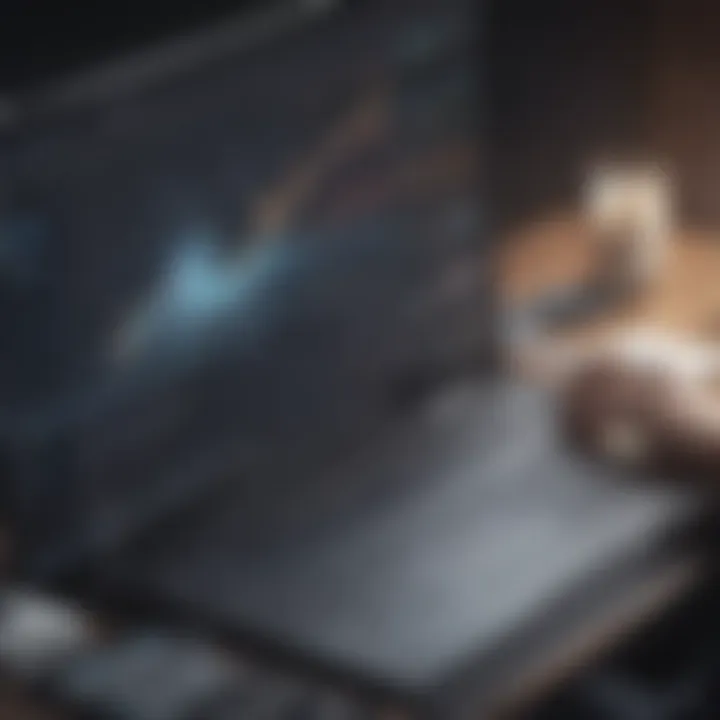
Tech Trend Analysis
As we delve into the fascinating world of distributed streaming platforms, it becomes evident that this technology is at the forefront of modern data processing revolution. The current trend in the tech industry showcases a significant shift towards real-time data processing and analysis. This shift has profound implications for consumers, leading to enhanced user experiences through faster and more accurate data insights. Looking towards the future, the predictions and possibilities surrounding distributed streaming platforms are truly promising, offering unprecedented levels of scalability, efficiency, and reliability in handling vast amounts of data.
Product Reviews
In our exploration of distributed streaming platforms, it is crucial to examine the key products driving this technological advancement. These platforms typically encompass a wide range of features and specifications tailored to meet the diverse needs of users. From seamless data integration to advanced analytics capabilities, distributed streaming platforms excel in performance and scalability. Conducting a detailed performance analysis reveals their strengths and limitations, highlighting the pros and cons of each product. Based on this evaluation, a clear recommendation can be made to guide consumers and industry professionals in selecting the most suitable platform for their data processing requirements.
How-To Guides
To master the intricacies of distributed streaming platforms, it is essential to begin with a comprehensive introduction to the topic. Understanding the fundamental principles and underlying concepts lays a strong foundation for users to navigate through the complexities of data processing. Through step-by-step instructions, individuals can learn how to harness the power of distributed streaming platforms effectively. In addition to the basics, valuable tips and tricks are shared to optimize performance and maximize results. Should any challenges arise, a detailed troubleshooting guide is provided to assist users in addressing common issues and refining their data processing strategies.
Industry Updates
Keeping abreast of the latest developments in the tech industry sheds light on the evolving landscape of distributed streaming platforms. Recent trends indicate a rapid progression towards enhanced functionality and interoperability within these platforms. Analyzing market trends offers valuable insights into the implications for businesses and consumers alike. The increasing adoption of distributed streaming platforms is reshaping traditional data processing methods, paving the way for advanced capabilities and unprecedented data-driven decision-making. Industry professionals are encouraged to stay informed and adapt to these changes to remain competitive in a data-driven world.
Introduction
Diving into the realm of distributed streaming platforms is akin to embarking on a journey through the intricate landscape of modern data processing. In this digital era, where data reigns supreme, the concept of distributed streaming platforms holds a significant position. As technology rapidly evolves, the need for real-time data processing becomes paramount, driving the demand for efficient and scalable solutions. This section sets the stage for a deep dive into the core components, mechanisms, and applications of distributed streaming platforms, offering a comprehensive guide for enthusiasts and professionals seeking insight into this cutting-edge technology.
Defining Distributed Streaming Platforms
Overview of Distributed Systems
Unveiling the essence of distributed systems unveils a paradigm shift in data processing methodologies. Leveraging multiple interconnected nodes to store and process data, distributed systems revolutionize traditional computing paradigms. The key allure lies in their ability to enhance performance, fault tolerance, and scalability by distributing workload across a network of nodes. This approach fundamentally transforms the landscape of data processing, paving the way for real-time analytics, dynamic data processing, and streamlined data dissemination. Despite some inherent complexities, the benefits of leveraging distributed systems outweigh the challenges, making them an indispensable resource for modern data-centric applications.
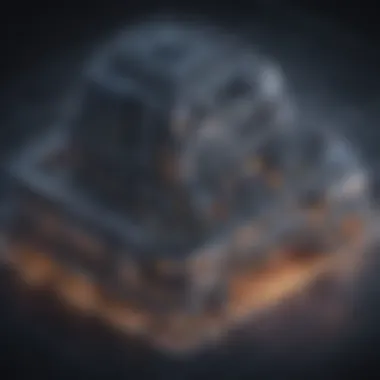
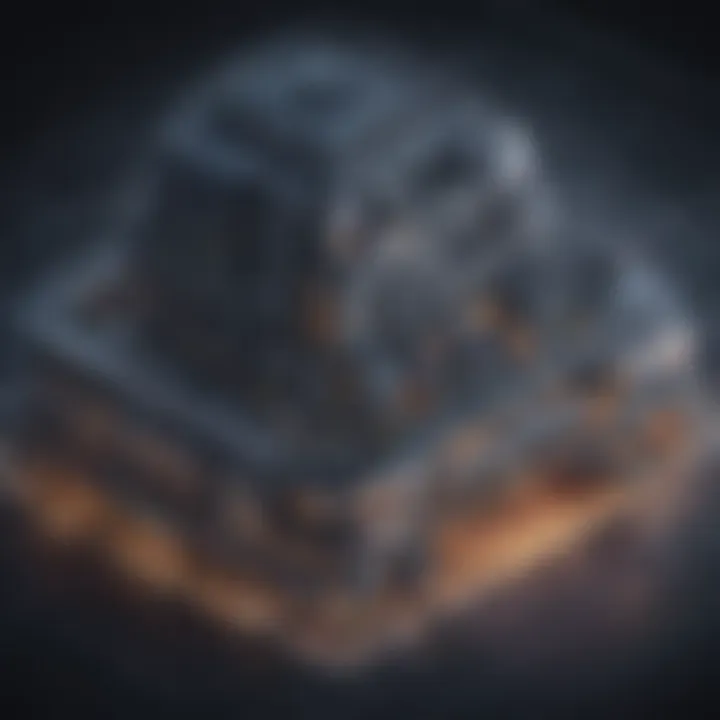
Evolution of Streaming Technology
The evolution of streaming technology marks a pivotal advancement in the realm of data processing. From batch processing to real-time analytics, the shift towards instantaneous data processing has reshaped how organizations harness information. Streaming technology enables continuous data ingestion and analysis, facilitating timely decision-making and actionable insights. Its real-time nature allows for immediate responses to incoming data streams, opening avenues for predictive analytics, anomaly detection, and dynamic data processing. While presenting unparalleled benefits in speed and efficiency, streaming technology also poses challenges related to resource utilization, data consistency, and system complexity, requiring adept management and strategic implementation to unlock its full potential.
Architecture of Distributed Streaming Platforms
In the realm of modern data processing, the architecture of distributed streaming platforms plays a pivotal role. It serves as the backbone that enables real-time data processing and analysis, catering to the increasing demands of today's data-driven landscape. Distributed streaming platforms offer a scalable and fault-tolerant infrastructure that harnesses the power of distributed systems to process data streams efficiently. The intricate architecture comprises core components like Stream Processors, Message Brokers, and Distributed Storage, each playing a unique role in ensuring seamless data flow and processing.
Core Components
Stream Processors
Stream Processors are instrumental in the real-time processing of data streams within distributed streaming platforms. These components handle the continuous flow of data, applying various operations such as filtering, transformations, and aggregations in a distributed manner. A key characteristic of Stream Processors is their ability to handle data streams with low latency, enabling near-instant analytics and responses. Their parallel processing capabilities make them a popular choice for organizations handling large volumes of real-time data, allowing for quick decision-making and actionable insights.
Message Brokers
Message Brokers act as intermediaries that facilitate communication between different components within the distributed streaming platform. They play a crucial role in ensuring reliable message delivery, message queuing, and load balancing. One of the key characteristics of Message Brokers is their ability to decouple the sender and receiver of data, enhancing system resilience and fault tolerance. This decoupling feature makes them a beneficial choice for managing high volumes of data streams efficiently.
Distributed Storage
Distributed Storage forms the foundational layer for persistent data storage within distributed streaming platforms. These storage systems are designed to handle large volumes of data across different nodes in a distributed fashion, ensuring data durability and availability. A key characteristic of Distributed Storage is its scalability, allowing organizations to store and retrieve data seamlessly as per their requirements. While offering high availability and fault tolerance, Distributed Storage systems also come with the challenge of managing data consistency and ensuring data integrity.
Data Flow Mechanism
Real-time data ingestion
Real-time data ingestion mechanisms play a critical role in capturing and ingesting data streams as they are generated. These mechanisms ensure that data is collected in a timely manner, feeding into the processing pipeline for real-time analysis. A key characteristic of real-time data ingestion is its efficiency in handling high-velocity data streams, enabling organizations to keep pace with the continuous influx of data from various sources.
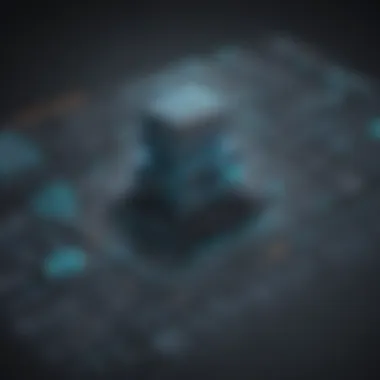
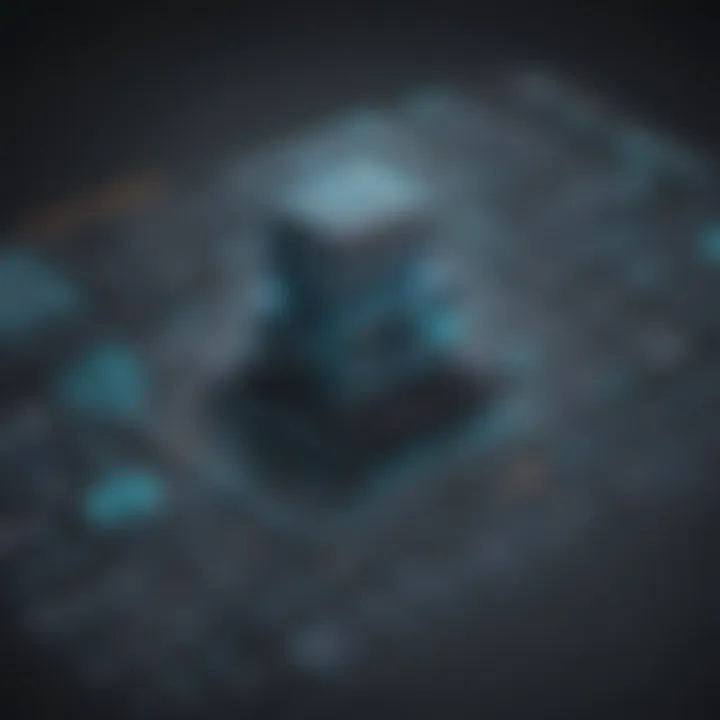
Stream processing
Stream processing involves the analysis and manipulation of data streams in real-time, allowing organizations to extract valuable insights and take immediate action. Stream processing systems are equipped with the capability to apply complex event processing, pattern matching, and windowed computations to streaming data. A key characteristic of stream processing is its ability to provide low-latency processing, enabling organizations to gain real-time visibility into their data and make informed decisions promptly.
Key Features of Distributed Streaming Platforms
Distributed streaming platforms play a pivotal role in modern data processing by offering scalability, fault tolerance, and low latency processing capabilities. Scalability is a key feature that allows these platforms to expand seamlessly as data volume grows. Horizontal scaling, a fundamental aspect, involves adding more resources to distribute the workload evenly, ensuring efficient performance. This approach is highly beneficial as it enables the platform to handle increasing data loads without compromising speed or accuracy. However, horizontal scaling requires careful planning to maintain consistency and avoid bottlenecks. On the other hand, partitioning divides the data into manageable subsets, optimizing processing efficiency. While partitioning enhances performance, it also introduces complexities in data retrieval and synchronization, necessitating robust strategies for seamless operation.
Fault tolerance is another critical feature that ensures system resilience against failures. Data replication involves duplicating data across multiple nodes to prevent data loss in case of hardware or network failures. This redundancy enhances data durability and availability, safeguarding against potential disruptions. However, data replication can impact storage capacity and increase network overhead, requiring a balance between redundancy and resource utilization. Automatic recovery mechanisms play a vital role in restoring system functionality after incidents, minimizing downtime and ensuring continuous operation. While automatic recovery streamlines the recovery process, it may overlook specific conditions or dependencies, necessitating comprehensive recovery protocols to address potential limitations.
Low latency processing is essential for real-time analytics and near-instant response times. Real-time analytics enable timely insights on streaming data, empowering businesses with instant decision-making abilities. The capability to process data instantaneously ensures that organizations can keep pace with dynamic data streams, capitalize on emerging opportunities, and address challenges promptly. However, real-time analytics may strain computational resources and require optimized data pipelines to support rapid processing. Near-instant response times enhance user experience by offering rapid feedback or actions based on incoming data. This feature is crucial for interactive applications or services that demand immediate responses to user inputs, driving engagement and satisfaction. Nevertheless, achieving near-instant response times necessitates efficient data processing frameworks and streamlined communication channels to minimize delays and latency issues.
Use Cases and Applications
In the landscape of distributed streaming platforms, understanding the significance of use cases and applications is paramount. These components serve as the practical application of the theoretical framework, showcasing how these platforms are utilized in real-world scenarios. This section delves into the specific elements that drive the adoption of distributed streaming platforms in various industries. By exploring the benefits and considerations of different use cases and applications, readers can grasp the versatility and adaptability of this technology across domains.
Real-time Data Analytics
Predictive maintenance
Predictive maintenance stands out as a key facet of real-time data analytics within distributed streaming platforms. Its primary function lies in the proactive identification of potential equipment failures based on data patterns, enabling preemptive maintenance actions. The key characteristic of predictive maintenance is its ability to forecast maintenance needs before issues arise, thereby minimizing downtime and optimizing operational efficiency. The unique feature of predictive maintenance is its utilization of machine learning models to predict equipment failures, offering an edge in predictive accuracy. While advantageous in enhancing equipment reliability and lifespan, a potential drawback lies in the complexity of implementing and managing predictive maintenance within the distributed streaming platform ecosystem.
Fraud detection
Within the realm of real-time data analytics, fraud detection plays a critical role in ensuring data integrity and security. It involves the continuous monitoring of transactions and activities to identify anomalous patterns indicative of fraudulent behavior. The key characteristic of fraud detection is its capacity to detect suspicious activities in real-time, enabling immediate intervention to mitigate potential risks. The unique feature of fraud detection lies in its adaptive algorithms that evolve with emerging threats, enhancing detection capabilities over time. Despite its benefits in safeguarding against fraudulent activities, challenges such as false positives and intricate data validation processes can pose limitations in maintaining operational efficiency.
IoT Data Processing
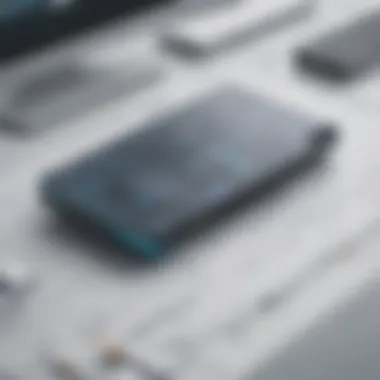
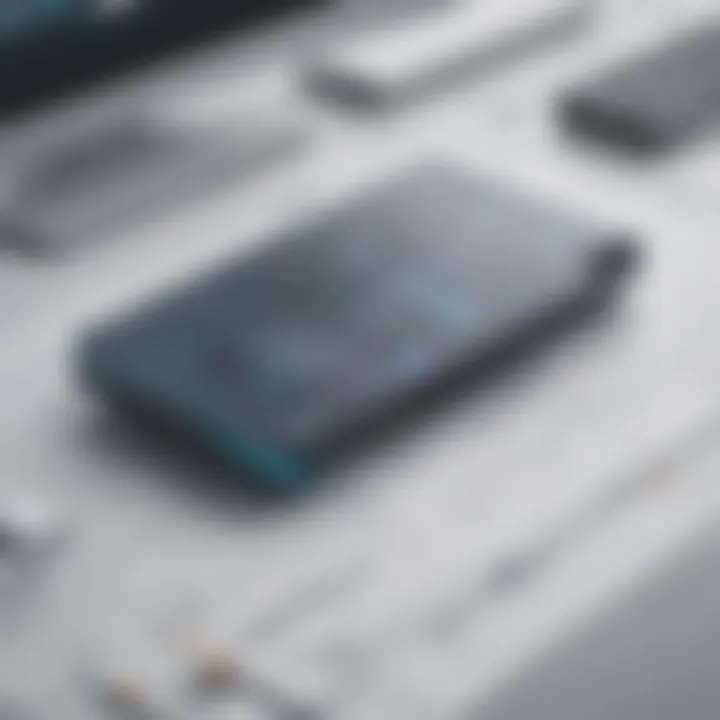
Smart home devices
Smart home devices represent a pivotal aspect of Io T data processing within distributed streaming platforms. These devices, equipped with sensors and connectivity features, enable the collection and analysis of data for enhancing home automation and efficiency. The key characteristic of smart home devices is their ability to interact with users and adapt to preferences, streamlining daily routines. The unique feature of smart home devices lies in their integration with AI-driven algorithms for personalized experiences, catering to diverse user needs. While advantageous in transforming traditional homes into smart living spaces, issues related to data privacy and connectivity vulnerabilities may pose challenges in widespread adoption.
Industrial IoT
Industrial Io T (IIoT) emerges as a foundational element in IoT data processing within distributed streaming platforms, focusing on industrial applications and automated processes. IIoT leverages interconnected devices and sensors to optimize manufacturing, supply chain management, and industrial operations. The key characteristic of industrial IoT is its emphasis on machine-to-machine communication and data exchange to drive seamless automation and decision-making. The unique feature of IIoT is its integration with Industry 4.0 principles, enabling predictive maintenance, resource efficiency, and agile production practices. While beneficial in revolutionizing industrial processes, concerns related to cybersecurity and interoperability across diverse systems may present challenges in implementing large-scale IIoT solutions.
Challenges and Best Practices
In the realm of distributed streaming platforms, navigating the landscape of challenges and implementing best practices is paramount for ensuring optimal performance and scalability. This section serves as a crucial pillar in understanding how to harness the full potential of these advanced systems. By addressing specific elements such as data consistency, fault tolerance, and system resilience, tech enthusiasts and industry professionals can fortify their knowledge and expertise in orchestrating robust distributed streaming solutions.
Data Consistency
Distributed Transactions
Distributed transactions play a pivotal role in maintaining data integrity and coherence across multiple nodes in a distributed streaming environment. The essence of distributed transactions lies in their ability to ensure atomicity, consistency, isolation, and durability (ACID properties) even when operating within a decentralized system. This characteristic makes distributed transactions a preferred choice for handling complex data operations that require reliability and transactional guarantees. However, it is essential to note that the inherent complexity of coordinating transactions across distributed nodes can introduce latency overhead and potential synchronization challenges, which must be carefully managed to avoid performance bottlenecks.
Eventual Consistency
On the other hand, eventual consistency offers a contrasting approach to maintaining data coherence within distributed streaming platforms. Instead of enforcing immediate consistency across all nodes, eventual consistency allows for temporary discrepancies between replicas, with the assurance that convergence will be achieved over time. This relaxed consistency model prioritizes availability and partition tolerance over strong consistency, making it well-suited for scenarios where real-time data synchronization is not a strict requirement. While eventual consistency enhances system availability and fault tolerance, it may introduce operational complexities related to conflict resolution and reconciliation mechanisms, influencing the overall performance and data correctness in distributed environments.
Monitoring and Performance Tuning
In the dynamic ecosystem of distributed streaming platforms, effective monitoring and performance tuning are indispensable practices for optimizing system efficiency and throughput. By implementing robust metrics collection mechanisms, organizations can gain valuable insights into resource utilization, throughput patterns, and system health indicators. These metrics serve as key performance indicators (KPIs) for evaluating system performance, identifying potential bottlenecks, and proactively addressing operational inefficiencies.
Metrics Collection
Metrics collection encompasses the systematic gathering, storage, and analysis of performance metrics from various components within a distributed streaming platform. By leveraging dedicated monitoring tools and frameworks, organizations can track key performance indicators such as data ingestion rates, processing latencies, and resource utilization across distributed nodes. This enables proactive performance monitoring, capacity planning, and workload optimization, essential for ensuring reliable and scalable data processing in real-time.
Optimization Techniques
Optimization techniques form the cornerstone of enhancing the overall efficiency and agility of distributed streaming platforms. By leveraging advanced optimization strategies such as query optimization, data partitioning, and parallel processing, organizations can streamline data processing workflows, improve computational efficiency, and reduce latency overhead. These techniques empower developers and system architects to fine-tune system parameters, optimize resource allocation, and harness the full potential of distributed streaming platforms for mission-critical applications. However, it is imperative to strike a balance between optimization efforts and system complexity to avoid diminishing returns and maintain operational simplicity in highly dynamic environments.