Deep Learning's Impact on Diverse Industries
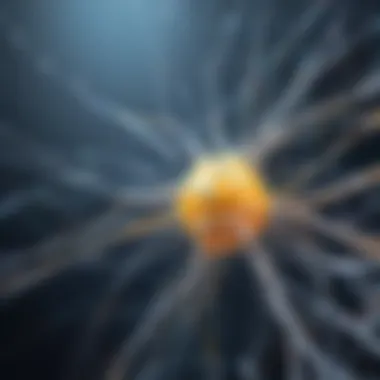
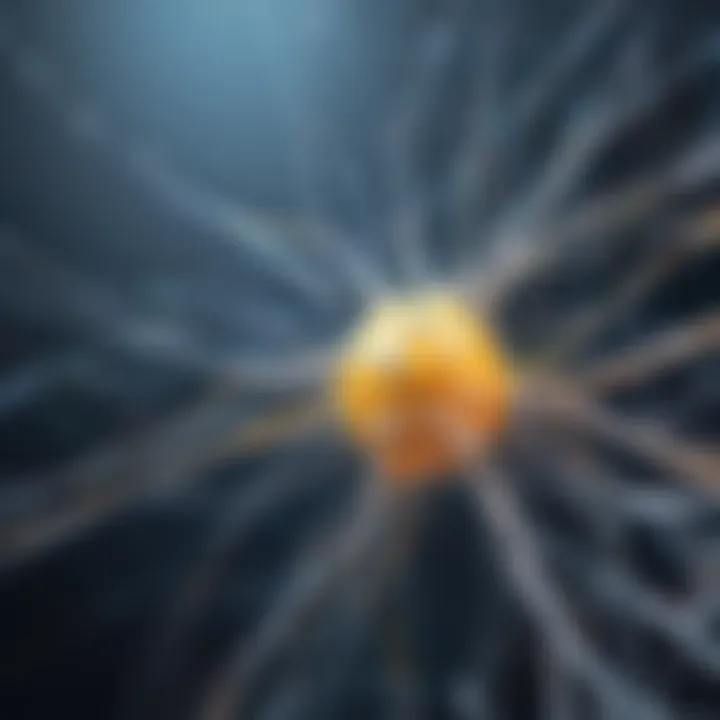
Intro
Artificial Intelligence (AI) is a term that’s been buzzing around for quite some time, but when you drop in the term deep learning, things get a little more intriguing. This subset of AI is like the secret sauce, providing the ability to analyze vast amounts of data in a way that mimics human cognition. The practical uses of deep learning span across various industries, innovating everything from healthcare diagnostics to financial forecasting.
As we delve deeper into applications of deep learning, it’s essential to understand that this technology leverages complex neural networks, resembling the way our brains operate. From analyzing patterns in images to translating languages instantaneously, the capabilities are not just impressive; they’re a game changer.
Tech Trend Analysis
Overview of the current trend
Deep learning has made significant strides recently. The surge in data availability spurred by IoT devices has become the backbone for the effectiveness of deep learning algorithms. In healthcare, AI-powered tools are revolutionizing diagnostic procedures by comparing patient images and predicting diseases more accurately than ever before.
Implications for consumers
For everyday consumers, the advantages of deep learning are hard to ignore. Think about personal assistants like Siri or Google Assistant—they utilize deep learning to understand your commands better and respond in a more human-like manner. Furthermore, if you’ve shopped online, you’ve likely seen recommendations pop up based on your past behaviors; this personalization is the work of sophisticated deep learning models.
Future predictions and possibilities
Looking ahead, the future seems bright for deep learning. Analysts predict more integration of this technology across different sectors. Autonomous vehicles, for instance, rely heavily on deep learning to interpret real-time data from their surroundings, enhancing safety and efficiency. Moreover, advancements in natural language processing will likely lead to even more intuitive human-computer interactions.
"The technological evolution driven by deep learning is not just a fad—it's poised to redefine our daily lives and the structure of industries altogether."
Industry Updates
The tech industry is buzzing with recent developments related to deep learning. Major players like Nvidia and Google are investing heavily in AI research, exploring new architectures that promise to increase training efficiency and model accuracy.
A notable update is the focus on reducing energy consumption while improving the performance of neural networks. This is crucial as the energy demands of training these models can be quite high, presenting a challenge amidst growing concerns over climate change.
Analysis of market trends
The market for deep learning applications is experiencing rapid growth. According to various forecasts, the global deep learning market is expected to surpass several billion dollars in the coming years. This growth partly stems from increased demand in sectors like healthcare, where AI-driven diagnostics are proving invaluable.
Impact on businesses and consumers
For businesses, adapting to this trend means investing in AI technologies to stay competitive. The timely insights gained from deep learning can offer a competitive edge, driving better decision-making. Meanwhile, consumers benefit from enhanced experiences—be it smarter home devices or improved online services that understand and predict their needs better than ever before.
Understanding Deep Learning
In the rapidly evolving discipline of artificial intelligence, understanding deep learning is like having a compass in uncharted waters. This segment sheds light on the fundamental aspects that define deep learning, which is crucial for comprehending its practical applications. Recognizing this topic's significance sets the stage for exploring its multifaceted implementations across various sectors.
Deep learning, a subset of machine learning, boasts a hierarchy of algorithms modeled after the human brain's neural architecture. This sets it apart from traditional machine learning methods in handling vast datasets and extracting meaningful insights. Here, we examine how deep learning mimics human cognition and why its insights are indispensable for contemporary AI applications.
Defining Deep Learning
At its core, deep learning involves training models on large amounts of data to recognize patterns and make predictions. This process relies on artificial neural networks, where multiple layers enable the network to learn increasingly abstract representations of the data. With the rise of computational power and availability of massive data sets, this approach has significantly transformed various industries, making it a buzzword in the AI realm.
Neural Networks Explained
Deep learning revolves around neural networks, which are the backbone of its functionality. These networks consist of interconnected nodes, or neurons, that process data in layers. Each layer extracts features, contributing to the overall understanding of the input data.
Basic Structure of Neural Networks
The basic structure of a neural network involves an input layer, one or more hidden layers, and an output layer. Each node in the hidden layers applies a mathematical operation to the inputs it receives, passing the result to the next layer. This structure is advantageous because it allows the network to learn complex patterns in data, which simpler models might overlook. The hierarchical design enables a stepwise extraction of features, making it a popular choice for tasks ranging from image recognition to natural language processing.
Types of Neural Networks
When it comes to types of neural networks, various architectures cater to different applications. Convolutional Neural Networks (CNNs), for instance, excel in image processing, while Recurrent Neural Networks (RNNs) shine in sequential data analysis such as speech recognition. Each type has its unique set of advantages based on the specific task at hand, allowing practitioners to select the best fit for their requirements. While CNNs are great for extracting spatial hierarchies in images, RNNs are designed to remember information over time, a crucial factor for tasks with dependencies.
Activation Functions
Activation functions are critical components of neural networks, determining whether a neuron should be activated or not based on its input. Popular options like ReLU (Rectified Linear Unit) and sigmoid play different roles in influencing the network’s learning dynamics. These functions introduce non-linearity to the model, allowing it to learn complex functions. Choosing the right activation function can greatly impact a model's performance, making it essential to experiment with various options during model training.
The Evolution of Deep Learning Techniques
Understanding the evolution of deep learning is key to grasping its current capabilities. Innovations have emerged over time, changing how we approach problem-solving in AI. This historical context is instrumental for anyone seeking to utilize deep learning effectively today.
Historical Context
Deep learning's roots trace back to the 1940s with the development of the first neural models. However, it wasn't until the 2010s that deep learning gained mainstream traction, propelled by advances in computational power and the advent of big data. This context highlights not only the resilience of the concept but also the technological advancements that have fueled its resurgence.
Milestones in Development
Key milestones, such as breakthroughs in CNNs and the advent of specialized hardware for deep learning, have shaped this field. The introduction of the AlexNet architecture in 2012 marked a pivotal moment in computer vision, leading to widespread adoption of deep learning techniques across various domains. This progress has brought about tools and frameworks that make deep learning more accessible than ever before, allowing even those with limited expertise to engage with this complex technology.
Current Trends in Research
As of now, deep learning research is brimming with innovations. From advancements in unsupervised learning approaches to self-supervised learning, researchers are constantly pushing boundaries. Current trends emphasize efficiency and interpretability, addressing concerns over the opacity of deep models. By focusing on these areas, the community seeks to enhance the practicality of deep learning applications, ensuring they are not just powerful but also understandable.
Deep learning is transforming industries and reshaping our technological landscape, opening doors to capabilities previously thought impossible.
Applications Across Various Sectors
In the rapidly evolving world of technology, the impact of deep learning stretches across various sectors, creating a ripple effect that enhances efficiency, accuracy, and innovation. The adoption of deep learning techniques is not just a trend but a necessary stride toward modernizing industries from healthcare to finance and beyond, making it crucial to understand these diverse applications.
Healthcare Innovations
Medical Imaging
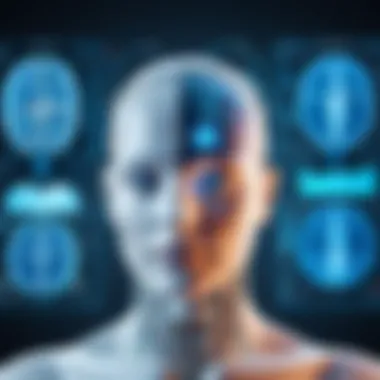
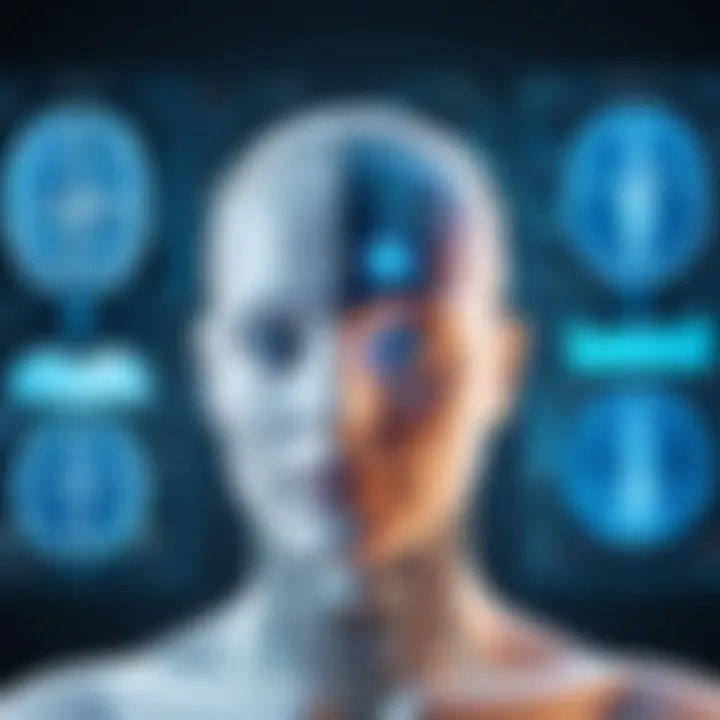
Medical imaging has undergone a transformation with the advent of deep learning algorithms. These algorithms analyze images at a level of detail that was previously unimaginable, identifying patterns that a human eye might overlook. For instance, deep learning models have been pivotal in detecting anomalies in X-rays and MRIs, significantly contributing to faster diagnosis and treatment.
A standout feature of medical imaging is its ability to improve accuracy and reduce human error. As a result, healthcare providers are facing lower misdiagnosis rates and more timely interventions. While the technological advancements promise a brighter future, it also raises concerns regarding data privacy and the need for rigorous validation of these algorithms, ensuring trust in their outcomes.
Predictive Analytics in Patient Care
Predictive analytics powered by deep learning is changing how patient care is approached. By interpreting vast amounts of data—from patient history to real-time health metrics—these models can forecast potential health issues before they escalate into severe conditions. For instance, predictive tools can identify patients at risk of readmission, thereby allowing for targeted interventions.
What makes predictive analytics distinctive is its proactive nature—it seeks to prevent rather than respond. However, the reliance on historical data can sometimes introduce biases, making it essential for healthcare providers to carefully consider data sources and the training of their models to provide equitable care.
Drug Discovery
Deep learning models are revolutionizing drug discovery by simulating and predicting the reactions of compounds. This application not only accelerates the traditional trial-and-error method but also offers insights into the molecular interactions that could lead to the development of new drugs. For instance, companies like Atomwise are using neural networks to screen millions of compounds in a fraction of the time it would take human researchers.
A key characteristic of drug discovery through deep learning is its potential to significantly lower costs and timeframes associated with bringing new drugs to market. Nevertheless, integrating machine learning in pharmaceutical research is still in its infancy, and while promising, the technology must overcome challenges pertaining to the reproducibility of results and regulatory hurdles.
Deep Learning in Finance
Algorithmic Trading
Deep learning has carved a niche in algorithmic trading, leveraging complex models to predict market trends and execute trades automatically. By analyzing patterns in historical data, these systems can identify lucrative trading opportunities with incredible speed. The trading firms that utilize deep learning tools can make decisions based on comprehensive market analysis without human intervention.
The unique feature of algorithmic trading is its capacity to analyze data in real-time, reacting to market changes seconds faster than a human trader could. However, this brings its own set of challenges, particularly around market volatility and the potential for errors in automated decisions, which can lead to drastic financial implications.
Risk Assessment Models
Deep learning is instrumental in enhancing risk assessment models, helping financial institutions mitigate potential losses. By analyzing vast datasets—like credit scores, transaction history, and consumer behavior—these models can predict the likelihood of default and adjust risk profiles accordingly. Utilizing deep learning models aids banks in making informed lending decisions and minimizing risks.
The attractiveness of these models lies in their ability to process nonlinear relationships within data, which traditional models may miss. Nonetheless, shadows of bias can creep in if historical data reflects systemic issues, necessitating a thoughtful approach to data governance and model training.
Fraud Detection Systems
Fraud detection systems are another hallmark application, utilizing deep learning to identify fraudulent activities not easily discernible by traditional means. By continuously analyzing transaction patterns and flagging anomalies, these systems can prevent substantial financial losses. For instance, firms like PayPal are employing such technologies to enhance their fraud detection processes.
The critical characteristic here is the adaptive learning capabilities of these systems, improving over time as they encounter more data. Yet, even advanced models can struggle with false positives, where legitimate transactions are flagged as fraud, leading to inconvenience and potential loss of customer trust.
Autonomous Vehicles
Sensor Fusion
In the realm of autonomous vehicles, deep learning plays a vital role, particularly in sensor fusion which involves combining various data sources for better decision-making. By analyzing information from cameras, LiDAR, and radar, self-driving systems can create an accurate perception of their environment. This fusion of data enhances the vehicle's ability to navigate complex scenarios.
A notable aspect of sensor fusion is its capability to provide a comprehensive view of the surroundings, enhancing safety and reliability. However, it also demands significant computational resources and poses challenges in real-time data processing.
Object Detection and Recognition
Object detection and recognition are pivotal for autonomous vehicles, allowing them to identify pedestrians, road signs, and other vehicles. Deep learning continues to improve these technologies, creating models that can differentiate between various objects accurately. This is crucial for making driving decisions, thereby enhancing safety.
What makes this application crucial is the ability to improve over time with more data, allowing for better situational awareness. However, like many technologies, the risk of adversarial attacks—where misleading inputs significantly affect model outputs—remains a concern that the industry is working to address.
Path Planning Algorithms
Path planning algorithms use deep learning to determine the optimal route for navigation. By considering various factors—like traffic conditions and road constraints—these algorithms can make real-time adjustments to enhance safety and efficiency. For example, companies like Waymo are heavily investing in such technologies to improve their autonomous driving solutions.
The unique feature here is the real-time adaptability, which is paramount for safe navigation. Balancing efficiency with safety remains a challenge, as unforeseen circumstances on the road can necessitate immediate changes to planned routes.
Natural Language Processing Applications
Sentiment Analysis
In the realm of sentiment analysis, deep learning serves as a tool to gauge public sentiment based on text data from social media, reviews, and forums. By training models on vast amounts of textual data, businesses can understand consumer feelings towards products or services, allowing them to make informed decisions.`
Sentiment analysis is attractive because it provides insight into customer opinions at scale. However, the nuances of language and context can sometimes lead to misinterpretation, necessitating ongoing improvements in model training.
Chatbots and Virtual Assistants
Chatbots and virtual assistants leverage deep learning to understand and respond to user inquiries intelligently. By recognizing user intent and context, these technologies deliver more accurate and relevant responses, enhancing customer experience. Organizations like OpenAI have developed sophisticated models leading to better human-computer interactions.
One key feature of these assistants is their unique ability to learn from interactions, continuously improving their service quality. Nonetheless, the challenge still exists in addressing ambiguities in user requests, which can lead to misunderstandings.
Machine Translation Systems
Machine translation systems, powered by deep learning, translate text across different languages with increasing proficiency. Technologies like Google Translate use complex algorithms to enhance both accuracy and fluency in translations.
The strength of machine translation lies in its ability to improve continually with usage and data. Nevertheless, issues surrounding cultural nuances and context may lead to mistranslations, showing that human oversight is still valuable.
Deep Learning in Creative Industries
Generative Art
Generative art has tapped into deep learning to produce visually striking pieces that push the boundaries of creativity. Artists employ algorithms to generate original artwork based on specified parameters, allowing for unique artistic expressions.
A distinct characteristic is the collaborative relationship between the artist and the algorithm, leading to artwork that might not have been possible without machine input. However, debates surrounding authorship and originality are prevalent in this space, questioning the role of the artist.
Music Generation
Deep learning is also reshaping the music industry by enabling the generation of music compositions. Companies like OpenAI with their MuseNet model are crafting pieces in various styles, showing how technology can augment creativity.
The unique aspect here is the ability to generate music that resonates with human emotions and creativity. Yet, concerns about authenticity and the emotional depth of AI-generated music remain a topic of discussion.
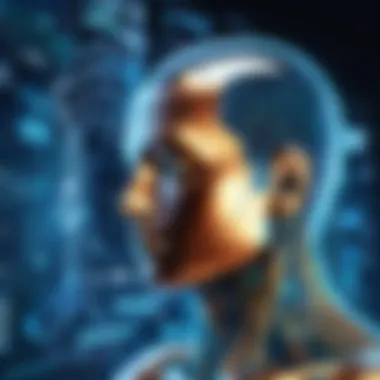
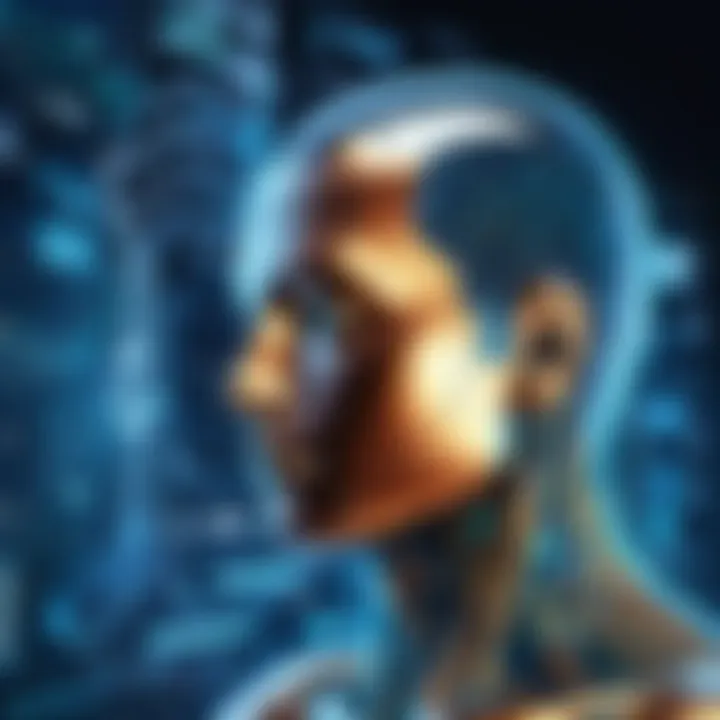
Content Creation Automation
Content creation has extensively adopted deep learning, where algorithms automate writing tasks like blog posts, marketing content, and even news articles. Tools powered by AI can generate coherent and engaging text, helping businesses scale their content efforts efficiently.
What makes content creation automation appealing is its capacity to save time and resources for content creators. However, striking a balance between quality and quantity remains a crucial challenge, as not all outputs may meet the expected standards.
Technical Challenges and Solutions
In the ever-evolving landscape of artificial intelligence, the application of deep learning brings a wealth of opportunities, but it also surfaces numerous technical challenges that warrant thorough examination. Understanding these difficulties helps stakeholders make informed decisions while advancing their AI projects, ultimately leading to superior outcomes. Identifying and addressing the critical obstacles such as data requirements, computational demands, and ethical considerations formulates a roadmap to enhance the overall efficacy of deep learning technologies.
Data Requirements and Quality
Data is often dubbed the lifeblood of deep learning algorithms. However, it’s not just about quantity; the quality of this data can make or break the success of any AI initiative.
Big Data Integration
The integration of big data stands as a cornerstone for effective deep learning applications. It allows models to harness an extensive volume of information, which fuels better accuracy and enhances predictive capabilities. The essence of big data is its ability to compile vast datasets that traditional methods would struggle to process. Notably, big data can capture the nuances present in diverse datasets, which can drastically boost model performance.
However, while the advantages are evident, it is crucial to navigate the complexities involved in big data integration. These complexities include issues related to storage, processing power, and data access that can be daunting for developers. The challenge is finding the right infrastructure that can handle the heavy lifting of big data without compromising performance.
Data Labeling Challenges
Data labeling, on the other hand, introduces its own set of hurdles that can stall projects if not carefully managed. This process transforms unstructured data into a format suitable for training models—think of it as teaching a child to recognize objects through examples. The primary challenge lies in the scale of data that needs to be labeled accurately and consistently. A poorly labeled dataset will lead to biased models, ultimately skewing the insights derived.
The high cost and time involved in manual data labeling can also push teams to seek alternative methods, yet while automation tools promise efficiency, they may also introduce errors or misclassifications.
Synthetic Data Solutions
Amidst the challenges of data procurement and labeling emerges the concept of synthetic data, offering a fresh perspective in the equation. Synthetic data is designed to mirror real-world scenarios while overcoming the limitations linked to real data collection, such as privacy concerns and data scarcity. This kind of data can stimulate richer datasets for various applications.
Nevertheless, this approach is not without caveats. The core advantage of synthetic datasets is their flexibility and cost-effectiveness, but if not generated properly, they might lack vital attributes of real-world data, leading to models that don’t perform well in live environments. Ensuring that the synthetic datasets maintain sufficient fidelity to real-world data is paramount for their successful adoption.
Computational Demands
The need for robust computational resources cannot be overstated when it comes to deep learning applications. The success of these sophisticated models hinges significantly on their computational capabilities.
Hardware Limitations
Hardware limitations pose significant constraints in creating and training deep learning models. Many companies experience bottlenecks due to inadequate computational power, particularly when dealing with intricate datasets or deploying large models. The key characteristic of these limitations is often rooted in the need for powerful GPUs, which may not be readily available to smaller enterprises.
Additionally, the investment required for advanced hardware can deter organizations from pursuing ambitious deep learning projects. Thus, while superior hardware can dramatically enhance performance, access remains a challenge for many.
Energy Efficiency Concerns
Efficient energy usage is another pressing issue that often slips under the radar. Training deep learning models, especially complex ones, often entails considerable energy consumption, raising both costs and environmental concerns. This characteristic reflects how sustainability is becoming a focal point as organizations strive to reduce their carbon footprint.
Moreover, balancing performance with energy efficiency is a delicate act. Innovations that target more efficient models are necessary to mitigate the energy concerns while maintaining the accuracy that deep learning promises.
Optimizing Algorithms
To tackle computational issues, optimizing algorithms has surfaced as an invaluable strategy. Various techniques like pruning, quantization, and knowledge distillation streamline models, which not only improves computational efficiency but also reduces the memory footprint.
The primary advantage of optimizing algorithms lies in its capability to retain model accuracy while using fewer resources. This optimization can lead to significant enhancements in response times, making deployments more practical in real-world applications. Nonetheless, one must remain cautious, as excessive optimization can sometimes lead to deteriorated performance across diverse tasks.
Bias and Ethics in AI
As deep learning continues to permeate various sectors, understanding bias and ethical implications becomes indispensable for responsible use. The methods adopted in model training can inadvertently perpetuate harmful biases present within the datasets.
Identifying Bias in Training Data
Recognizing bias in training data is essential to prevent the reinforcement of stereotypes or systematic discrimination. It is crucial to review the datasets for representation, as imbalanced data can distort the learning process of an AI model. Being aware of and identifying these biases ensures that the outcomes produced by the AI are fair and reflective of diverse perspectives.
The challenge lies in collecting data that encompasses all facets of a target population comprehensively. This is a key step toward bridging the gap between ideal AI use and practical limitations.
Ethical AI Deployment
The deployment of AI also raises ethical concerns that tech professionals must contend with fiercely. Decisions on how AI systems act in society can have profound implications. Ethical AI deployment incorporates principles like transparency, accountability, and fairness. The endeavor is to build systems that not only serve functional purposes but also uphold moral standards. The key here is understanding that it’s not just about what AI can do, but rather what it should do.
Nevertheless, adhering to ethical practices can be challenging when various stakeholders have differing priorities. Thus, organizations need robust frameworks for ethical AI deployment that maintain a balance between innovation and ethical responsibility.
Regulatory Considerations
The current regulatory landscape presents a multifaceted dilemma for AI developers. In light of growing public scrutiny over data use and privacy, understanding regulatory considerations is paramount. Regulations such as GDPR or CCPA shape how AI systems handle data, and compliance with these regulations is not merely optional. The unique feature here is the evolving nature of regulations worldwide, which creates an environment of uncertainty and unpredictability.
While regulations ensure a semblance of protection for users, they also impose additional hurdles. Companies may face challenges in navigating these complex legal frameworks, risking delays in deployment or achieving compliance. Still, successful navigation can strengthen consumer trust, catalyzing long-term growth and success for AI initiatives.
Looking Ahead: The Future of Deep Learning
The horizon for deep learning is not just bright; it’s brimming with possibilities. Understanding future trends is essential for anyone invested in artificial intelligence, as these advancements could redefine entire industries. This section will delve into emerging trends like transfer learning, explainable AI, and federated learning, which are set to shape the landscape significantly.
The significance of these trends cannot be overstated, as they promise not only to enhance the efficiency of AI systems but to address some of the ethical issues that have dogged the field. These developments highlight the necessity of advancement while ensuring that technology remains accessible and comprehensible.
Emerging Trends
Transfer Learning
Transfer learning stands out as a game-changer in deep learning. It's the practice of taking a pre-trained model and fine-tuning it for a specific task. The powerhouse behind many success stories in AI, transfer learning sheds light on how much can be achieved with relatively little data.
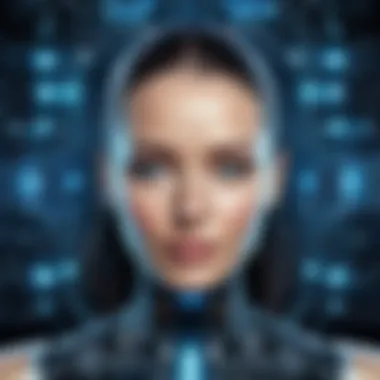
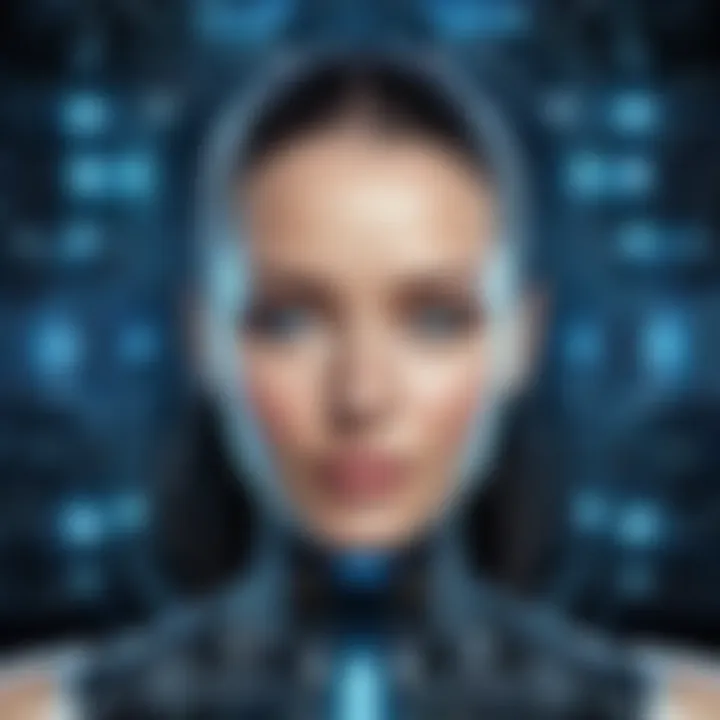
The key characteristic of transfer learning is its ability to leverage existing knowledge from one domain and apply it to another, which removes a chunk of the heavy lifting in model training. It’s particularly beneficial when working with limited data, allowing models to perform well without needing to start from scratch.
However, while transfer learning certainly has its advantages, it comes with challenges. Fine-tuning can be a tricky business; if the original model and the new task vary too much, it may perform poorly. Nevertheless, the unique capacity for leveraging previous work is its undeniable edge.
Explainable AI
Explainable AI (XAI) addresses the critical need for transparency in AI systems. As deep learning models have become more complex, understanding their decision-making processes has become increasingly challenging. XAI provides insights into how models arrive at their conclusions, which is crucial for trust and accountability in AI applications.
The core appeal of explainable AI lies in its ability to make the inner workings of deep learning accessible to users and developers alike. This transparency leads to better debugging and gives stakeholders confidence, particularly in sensitive areas like healthcare or finance.
However, the journey to achieving XAI isn’t without obstacles. While striving for transparency, explainable models may trade off some predictive performance, as they might rely on simpler models that are easier to interpret but not as powerful. Despite this, the initiative for better understanding remains a top priority as we move forward.
Federated Learning
Federated learning is a fresh approach that changes the way models are trained by keeping data decentralized. Instead of collecting data in a centralized server, models learn from data stored locally across devices. This method can enhance privacy and security while maintaining performance.
Its major characteristic is that it mitigates risks related to data breaches. Organizations can collaborate without exposing sensitive data, making it a safe option for sectors where privacy is paramount, like finance or healthcare.
Nonetheless, federated learning poses technical challenges, such as ensuring model accuracy when working with disparate data sources and potential communication overhead. Yet its ability to support privacy concerns while still fostering model improvement offers a promising avenue for future deep learning.
Integration with Other Technologies
IoT and Deep Learning
The integration of the Internet of Things (IoT) with deep learning takes data-driven applications up a notch. IoT devices generate massive amounts of data, and deep learning models can sift through all this information to extract actionable insights. This relationship enables smarter cities and more efficient systems.
The interesting aspect of IoT and deep learning is the symbiotic improvement they offer; as more devices become interconnected, the quality of data increases, permitting deeper analysis and more sophisticated models. This situation means enhanced functionality for sectors like transportation and healthcare.
On the flip side, challenges arise due to the vastness and variable quality of data produced by IoT devices. Handling this complexity requires robust infrastructure and strategies, which may not always be readily available.
Blockchain Applications
Blockchain technology’s rise is paving new paths for integrating security in deep learning applications. Smart contracts, tokenization, and decentralized systems are fostering innovative uses of AI where transparency and trust are key elements.
The primary characteristic of blockchain in AI is its integrity; it creates a tamper-proof ledger, ensuring that the data fed into models is secure and reliable. This dual alliance can be a game changer, especially in finance and supply chain.
Nevertheless, blending these technologies isn't without hurdles. The computational overhead required for blockchain can slow processes down. Plus, establishing synergy between different technologies can be a complex affair, but the potential rewards are worthwhile.
Quantum Computing Impact
Quantum computing is a thrilling frontier with huge implications for deep learning. It's an emerging technology that could exponentially boost computational power, enabling the processing of vast datasets at unprecedented speeds and complexities.
The exciting aspect of quantum computing is its compatibility with AI; it allows for more complex algorithms that can solve problems beyond the reach of classical computers. This synergy has the potential to revolutionize optimization tasks, enabling AI systems to make better, faster decisions.
However, quantum computing remains in its infancy, and many challenges lie ahead. Development costs are high, and the technological ecosystem is still not robust. Yet, as progress continues, its impact on deep learning could be profoundly transformative.
Global Impact and Urbanization
Smart Cities
The concept of smart cities thrives on the interplay between technology and urban living. Using deep learning in city management can lead to enhanced efficiency, improved public services, and a better quality of life for residents.
A standout characteristic of smart cities is their interconnected framework, where analytics derived from deep learning models helps in decision-making processes. This can manifest in traffic management, energy distribution, and public safety initiatives.
Despite the benefits, smart city projects can face challenges such as data privacy concerns and the potential for inequity in technological implementation across different communities. Addressing these challenges is crucial to ensure that smart cities serve all their citizens.
Sustainability Initiatives
Sustainability initiatives are increasingly leveraging deep learning to tackle environmental challenges. Models can optimize resource management, reduce waste, and conserve energy through smart algorithms that analyze consumption patterns.
The main advantage here is applying data to encourage responsible practices, driving down carbon footprints and promoting everyone’s quality of life. However, the challenges revolve around integrating these AI-driven approaches into existing frameworks, which can be resource-intensive and complex.
Enhancing Education Through AI
Education stands to benefit from deep learning applications tremendously. Personalizing learning experiences through adaptive algorithms can lead to improved student outcomes and engagement levels.
The key feature of enhancing education with AI is its capability to tailor experiences to individual learner needs. This flexibility can bridge gaps in understanding and create inclusive environments.
However, issues of accessibility and the digital divide remain significant hurdles in this area. Ensuring that all students have access to these advanced technologies is essential to realize the potential benefits fully.
Finale
In this section, we summarize the critical insights discussed throughout this article and emphasize the transformative potential of deep learning in artificial intelligence.
Recap of Key Insights
Throughout the article, we have explored numerous applications of deep learning across varied sectors. In healthcare, machine learning and deep learning models are revolutionizing patient care through advanced medical imaging techniques and predictive analytics. The finance sector benefits through algorithmic trading and robust fraud detection systems, ensuring not just efficiency but security in transaction processing. Essentially, these applications underscore a broader trend: the ability of deep learning to process expansive datasets quickly and accurately, making complex decision-making feasible where you might once have hit a wall.
- Healthcare: From medical imaging to drug discovery.
- Finance: Risk assessment models and fraud detection systems.
- Autonomous Systems: Advancements in self-driving cars via object detection.
These instances not only highlight the technological advancements within sectors but also reveal an ongoing evolution in how we may interact with AI systems.
The Ongoing Role of Deep Learning in AI
Deep learning plays an undeniable role as the backbone of numerous artificial intelligence applications. This technology is now embedded in everyday tools, from voice recognition in smartphones to recommendation systems on streaming platforms. Deep learning's ability to learn from data patterns propels its use in innovative solutions that define modern life.
The integration of deep learning into AI systems does not merely enhance performance; it fundamentally alters the capabilities of these systems. For example, as deep learning techniques advance, they pave the way for smarter algorithms capable of performing tasks that once required human intelligence. To grasp the nuances of this change, consider how customer service has transformed with the advent of chatbots; as they evolve, their interactions become more intuitive, improving user satisfaction.
Final Thoughts on Future Developments
As we look toward the future, it’s critical to recognize the promise that deep learning technologies hold. Emerging trends such as federated learning and explainable AI are at the forefront of discussion among tech experts, opening avenues that improve both accessibility and transparency. The collaboration between these technologies could lead to more personalized and trustworthy AI solutions. In the not-so-distant future, we may even witness greater integration with other technologies like blockchain and IoT, shaping a landscape where AI facilitates smarter cities and sustainable initiatives.
"The power of deep learning lies not just in its ability to analyze data but in its potential to redefine how we understand and interact with technology."
In essence, deep learning’s integration into artificial intelligence is not just a trend; it's a trajectory towards a future where AI systems can augment human capability and understanding, offering the promise of better decision-making, increased efficiency, and enhanced creativity across all fields.