Understanding Data Science Manager Salaries: A Comprehensive Guide
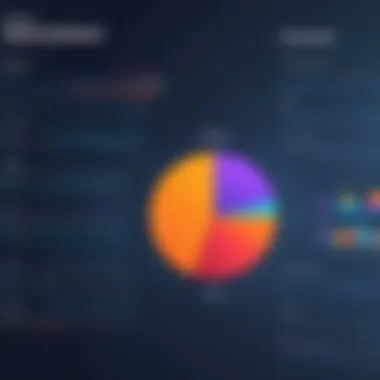
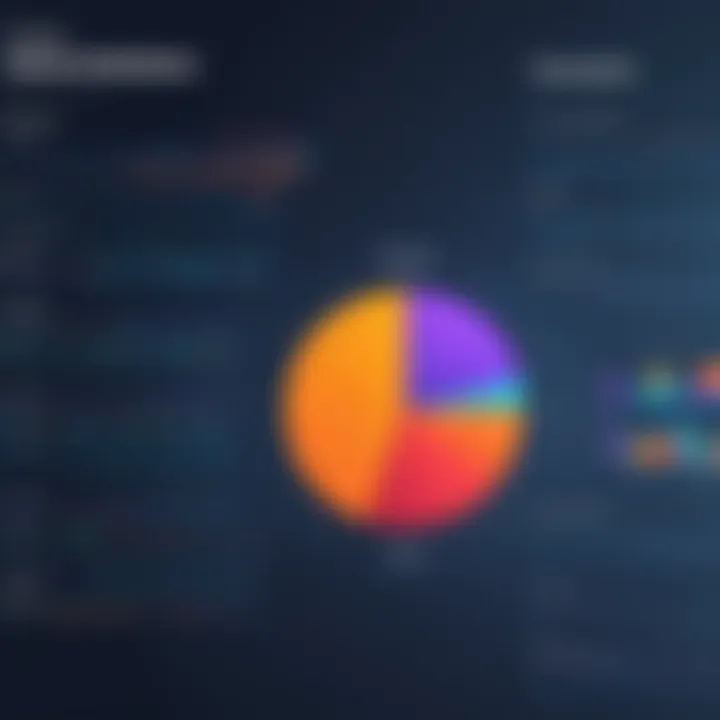
Intro
Understanding the compensation landscape for data science managers serves as a crucial undertaking for numerous professionals within the tech industry. As organizations increasingly recognize the value of data-driven decision-making, the demand for skilled managers in this realm has surged. This article embarks on a comprehensive examination of the factors influencing salaries in this field, delving into geographical variances, industry specifics, levels of experience, and educational qualifications, among others.
Additionally, we will scrutinize related job roles and draw comparisons that may illuminate the evolving salary dynamics. This exploration is aimed at both individuals considering a career path in data science management, as well as current practitioners wishing to gauge their remuneration against industry standards. By aggregating and interpreting extensive data, this article aims to provide clarity, thus empowering readers to make informed decisions regarding their careers and compensation expectations.
Prelude to Data Science Management
The domain of data science has emerged as a cornerstone for businesses aiming to leverage data for strategic advantage. Understanding data science management is vital, as it encompasses both the technical and managerial aspects of overseeing data science teams. A data science manager plays a crucial role in translating data insights into actionable strategies, aligning them with business objectives. Their responsibilities include not only managing data scientists but also ensuring that projects meet deadlines, budget constraints, and quality standards.
In the context of this article, we explore the various salaries associated with the role of a data science manager. The salary landscape is influenced by multiple facets such as geographic location, varying industry standards, and individual career progression. Having a clear comprehension of data science management allows aspiring professionals to gauge their potential earnings and understand the factors that contribute to compensation differences.
Defining the Role of a Data Science Manager
A data science manager's role is multidisciplinary. They serve as the bridge between technical teams and business leadership. This requires a blend of skills in data analysis, project management, and effective communication. Their duties typically include:
- Team Leadership: Guiding data scientists in their projects and fostering collaboration within the team.
- Project Oversight: Ensuring that data projects are aligned with organizational goals and delivered on time.
- Stakeholder Communication: Acting as the point of contact between data teams and other departments or executive leadership.
- Strategic Planning: Developing data-driven strategies that align with business objectives and enhance decision-making processes.
Due to these responsibilities, a strong managerial foundation combined with technical knowledge is essential for success in this role.
Importance of Data Science in Modern Businesses
Data science has become increasingly essential in various industries, as organizations turn to data to gain insights, improve efficiencies, and make informed decisions. Here are several key elements that highlight why data science is critical:
- Enhanced Decision-Making: Data-driven insights allow organizations to move beyond intuition, leading to smarter, calculated decisions.
- Operational Efficiency: Through analyzing data processes, businesses can streamline operations and identify areas of improvement.
- Customer Insights: Understanding customer behavior and preferences through data analytics informs marketing strategies and product development.
Given its impact, companies that invest in data science often gain a competitive edge. This underscores the significance of the role of a data science manager in guiding organizations through the complexities of data utilization.
Overview of Data Science Manager Salaries
Understanding the landscape of data science manager salaries is crucial for both aspiring professionals and current managers. This section sheds light on various dimensions that determine compensation in this vital role. Grasping the nuances of salary metrics can aid in strategic career decisions, be it understanding rightful compensation or benchmarking against industry standards.
Average Salary Insights
The average salary for data science managers can vary significantly based on several factors. Industry research often cites an average salary range between $120,000 and $200,000 per year. This disparity stems from variances in geographic locations, industries, and individual qualifications. Therefore, when analyzing average salaries, it is paramount to consider the source of this data.
Factors like company size also contribute to salary differences. Large tech firms like Google or Facebook typically offer high salaries compared to smaller startups. Additionally, companies that value advanced technical skills may offer more competitive compensation. For the most accurate insight into salary averages, platforms such as Glassdoor or Payscale provide valuable statistics, aggregated from employee-reported data.
Salary Distribution Across Experience Levels
Salary distribution reveals a significantly progressive curve as data science managers accumulate experience. Entry-level managers often start with salaries around $90,000. However, as professionals move up the ranks, their potential earnings rise sharply. Mid-level managers can expect to earn between $130,000 and $160,000, while senior professionals often surpass $200,000.
A few key considerations regarding salary growth are:
- Experience: Managers with more than ten years of experience often hold positions with increased responsibilities, thereby commanding higher salaries.
- Reputation: Strong reputations within the industry can translate to better offers, regardless of experience level.
- Skill Set: Proficiency in advanced analytics, machine learning, and data strategy often plays a crucial role in determining compensation levels.
"In data science, experience and continuous learning are essential. Keeping skills updated can significantly influence salary potential."
Overall, understanding salary distribution empowers data science managers to negotiate effectively, ensuring they receive compensation reflective of their skills and contributions.
Geographical Impact on Salaries
Understanding the geographical impact on salaries is crucial for anyone in the field of data science management. Compensation can vary widely based on location, influenced by factors like cost of living, demand for data science professionals, and the concentration of tech firms in a given area. With these dynamics in play, recognizing regional payment trends becomes essential for both current managers and those aspiring to enter the profession.
Salaries by Country
In different countries, salaries for data science managers can differ significantly. For instance, in the United States, the salaries tend to be quite high, especially in major tech hubs such as San Francisco and New York. Reports have shown that data science managers in these cities can earn upwards of $150,000 annually, while positions in smaller cities may offer closer to $100,000 or less.
Countries like Germany and the United Kingdom also offer competitive salaries, often ranging from €70,000 to £80,000 respectively for similar roles. However, countries in Southeast Asia may provide lower salaries, typically between $30,000 to $50,000, reflecting different economic conditions and market demands.
It's imperative to analyze salary reports by reputable sources to get a clearer picture of compensation trends by country. Detailed surveys and reports can be found on platforms like en.wikipedia.org or britanica.com.
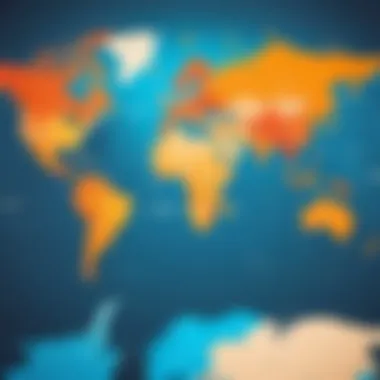
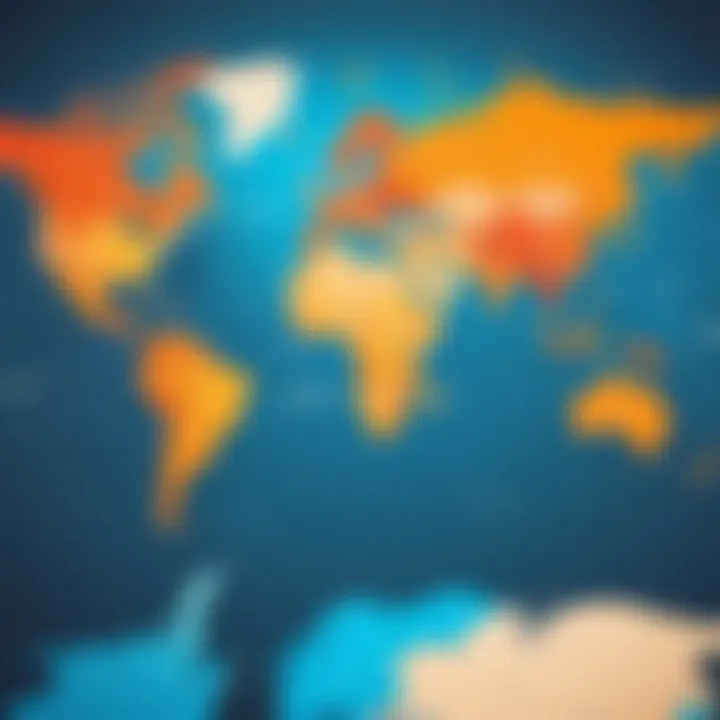
Urban vs. Rural Salary Differences
The urban-rural divide in salaries is another important aspect to consider. Generally, urban areas provide higher salaries than rural locations due to a few key factors.
- Higher Cost of Living: Urban centers often have a significantly higher cost of living, which can drive up salaries. Employers usually compensate for this to attract talent.
- Greater Job Opportunities: Urban regions tend to have more businesses and tech companies, increasing competition for skilled managers. Higher demand typically results in higher salaries.
- Network and Resources Access: Professionals in urban settings often have better access to industry networks, professional development opportunities, and resources that can further enhance their career growth.
In contrast, rural areas may offer lower salaries due to smaller company sizes, less competition, and lower overall living costs. For aspiring data science managers, understanding these geographical salary trends equips them with valuable information for making informed career decisions.
Industry-Specific Salary Variations
When evaluating data science manager salaries, it is crucial to consider the industry in which a data science manager operates. Different industries not only offer varying salary ranges, but they also have unique demands and expectations for professionals in the role. Understanding these variations helps aspiring managers to set realistic salary expectations and informs companies on how to structure compensation packages effectively.
Tech Industry Salaries
In the tech sector, data science managers typically earn some of the highest salaries compared to other industries. This is largely due to the high demand for data-driven decision-making within technology companies. Big firms like Google, Facebook, and Amazon often lead the market with generous compensation packages. For example, the average salary for a data science manager in these companies can exceed $150,000 annually, often supplemented by substantial stock options and bonuses.
Factors that influence these high salaries include:
- Company size: Larger tech companies usually have more resources, allowing them to offer higher salaries.
- Demand for talent: With the rapid growth of AI and machine learning, managers with expertise in these areas are particularly sought after.
- Location: Tech hubs like Silicon Valley are known for their inflated salaries due to the high cost of living and competition for skilled professionals.
Finance and Healthcare Comparisons
In contrast to the tech industry, salary figures for data science managers in finance and healthcare sectors can present a different picture. Both industries recognize the value of data science, yet the salary ranges can vary significantly.
In finance, data science managers often earn competitive salaries, typically ranging from $120,000 to $170,000. Investment firms and banks invest heavily in analytics to devise strategies, manage risks, and optimize portfolios. As a result, they often require data science managers who can manage large datasets and provide actionable insights.
On the other hand, in healthcare, the average salary for a data science manager may be a bit lower, ranging from $100,000 to $140,000. Despite this, the role is increasingly important as the industry adopts more data-driven approaches to patient care and operational efficiency.
"The need for actionable insights in healthcare continues to rise, but compensation lags behind that of other industries, highlighting a potential area for salary growth."
Overall, when exploring salary variations across industries, it’s clear that domain-specific knowledge and skills can greatly impact a data science manager's earning potential. Aspiring managers should consider these industry dynamics when shaping their career paths.
Educational Background and Salary Correlation
Educational background plays a significant role in shaping the salary landscape for data science managers. The higher the educational attainment, the more it typically influences salary levels. This correlation stems from various reasons.
Firstly, advanced degrees often represent a deeper understanding of complex concepts and practices related to data science. Holding a master's degree in data science or a related field can enhance one’s credibility. Employers tend to favor candidates with advanced degrees, particularly in competitive industries. This preference often translates into higher starting salaries for those who hold such qualifications.
Secondly, many companies have formalized salary structures that assign compensation ranges based on educational qualifications. This practice means that candidates with advanced education likely start at a higher pay band compared to those without.
In addition, data science managers frequently deal with intricate projects requiring extensive analytical skills. Higher education can also equip individuals with a more robust theoretical foundation in statistical methods, programming, and machine learning. As a result, these skills contribute to their overall effectiveness, justifying higher compensation.
Furthermore, the experience associated with higher education should not be overlooked. Many advanced degree holders engage in significant projects during their studies, including internships, research, and practical applications. These experiences enhance their skill set and marketability.
"Employers often see advanced degrees as indicators of commitment and analytical ability which are crucial in data science roles."
In summary, educational background significantly correlates with salary outcomes in data science management. The emphasis on advanced degrees fosters higher compensation, reflecting both market demands and skill enhancement.
Impact of Advanced Degrees
An advanced degree, such as a master's or Ph.D., plays a pivotal role in determining salary levels for data science managers. Numerous studies indicate that individuals with advanced qualifications earn, on average, higher salaries than their counterparts with only a bachelor's degree. This phenomenon occurs for multiple reasons.
- Specialized Knowledge: Advanced degrees facilitate deeper domain knowledge in data science and analytics. Understanding these intricate subjects can lead to more effective decision-making in managerial roles.
- Research Opportunities: Many master's and doctoral programs offer research opportunities. Engaging in research helps build a network, which can be invaluable for salary negotiations and future job opportunities.
- Career Advancement: Advanced degrees often serve as a stepping stone for career progression. Professionals seeking senior roles or moves into strategic positions benefit from the knowledge gained during their studies.
Certifications and Specialized Training
Certifications and specialized training are crucial elements that can enhance salary prospects for data science managers. While a degree may open doors, certifications often provide specific skills that directly align with industry needs.
- Industry Recognition: Certifications from reputable organizations, such as Microsoft Certified: Azure Data Scientist or Certified Analytics Professional, are recognized across industries. This recognition increases a candidate’s value in the job market.
- Skill Enhancement: Specialized training often focuses on current tools and technologies, keeping professionals updated with the latest advancements. This training not only ensures relevance but also increases efficiency and problem-solving capabilities.
- Networking and Community Engagement: Many certification programs connect professionals with broader networks. Engaging with communities often leads to unforeseen opportunities.
Ultimately, certifications and specialized training serve as tools for professional development while also influencing competitive salary offerings. For data science managers, such credentials can lead to better job positions and salary enhancements.
Experience Level and Career Progression
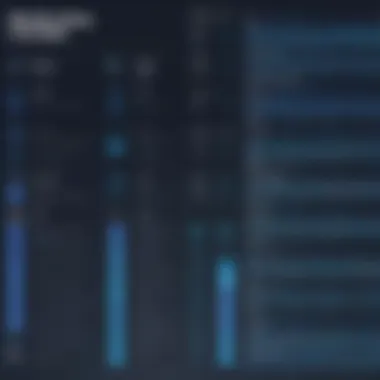
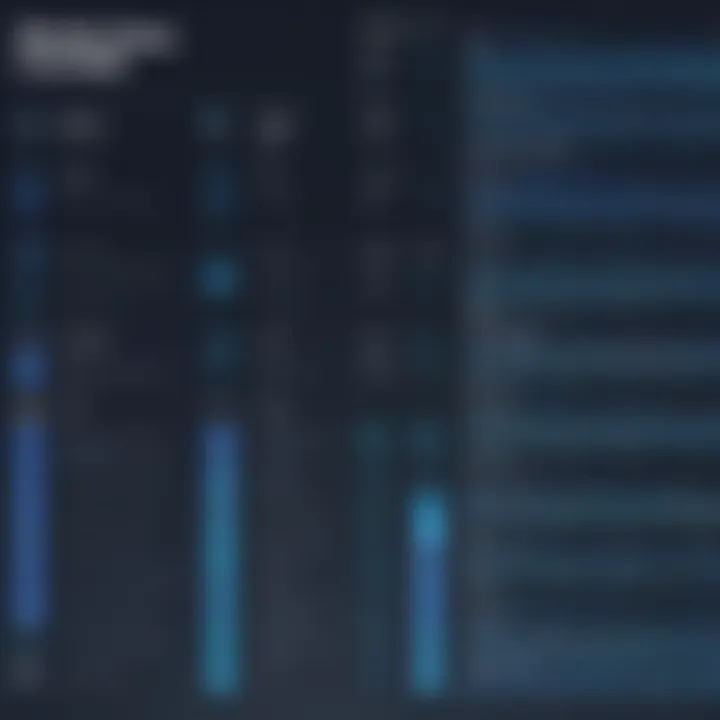
The topic of experience level and career progression is crucial when evaluating data science manager salaries. Understanding how experience influences compensation helps professionals identify their worth in the marketplace. Different experience levels provide varying responsibilities, skill sets, and expectations, greatly impacting overall salary.
A data science manager with advanced experience typically commands a higher salary than their entry-level counterparts. As one progresses in their career, factors such as leadership abilities, project management skills, and familiarity with advanced data methodologies become critical. This progression not only reflects a deepening of expertise but also the ability to drive business success through effective data utilization.
Entry-Level vs. Senior Roles
Entry-level data science managers often start their careers with compensation that might seem competitive but falls short when compared to senior roles. An entry-level position generally offers a salary range that allows for insights into the field but lacks substantial financial rewards relative to responsibilities. For example, an entry-level manager may earn between $80,000 to $100,000, depending on the location and the organization.
In contrast, a senior data science manager's expertise and capacity to lead teams can push salaries well above $150,000. These roles require proven track records in project success and team leadership, which typically results in higher pay.
- Entry-Level Characteristics:
- Senior Role Characteristics:
- Limited experience in management
- Possible support roles to senior teams
- Focus on learning and adapting to business needs
- Extensive experience managing teams
- Direct impact on company strategy
- Established networks and stakeholder relations
Role of Project Management Experience
Project management experience plays an integral role in salary determination for data science managers. Professionals who possess strong project management skills are often seen as valuable assets to their organizations. Employers favor those who can ensure projects meet deadlines, adhere to budgets, and deliver quality outcomes.
For data science managers, this experience can increase their salary potential. A solid background in project management indicates not only technical expertise but also an ability to handle various stakeholders, align project goals with business objectives, and foster team collaboration.
The influence of project management in compensation is notable:
- Managers with project management certifications, such as PMP or Agile, may benefit from bonuses or higher base salaries.
- Organizations are likely to offer higher pay to candidates with a proven track record of successful project completions.
Benefits and Compensation Packages
In the realm of data science management, salary is only one facet of overall compensation. Many factors impact the total value of a compensation package. Understanding the various components of benefits and compensation is crucial for data science managers at any stage of their career. The right benefits can enhance job satisfaction, improve work-life balance, and ultimately contribute to higher productivity.
Health and Wellness Benefits
Health and wellness benefits are essential in attracting and retaining top talent. For data science managers, these packages often include medical, dental, and vision insurance. Managers might also have access to mental health resources and wellness programs. Such offerings can reflect an employer’s commitment to employee well-being.
Moreover, companies may also provide gym memberships or wellness stipends. These health benefits contribute to overall job satisfaction and can differentiate one company from another in a competitive job market. The importance of health benefits cannot be overstated. Employees tend to feel valued when their employers prioritize their health needs.
Stock Options and Bonuses
Stock options and bonuses are potent incentives for many data science managers. These financial perks link an employee's profitability to the company’s performance. When the company does well, managers benefit directly through stock price increases.
Bonuses may come in various forms, such as performance-based bonuses or end-of-year incentives. Data science managers may receive bonuses tied to their own KPIs, making these payments a way to motivate and reward high performance.
Overall, understanding these components of a compensation package offers critical insights into one’s total remuneration as a data science manager. It provides a fuller picture beyond base salary and helps individuals navigate job offers or negotiations more effectively.
Understanding the full spectrum of benefits and compensation can provide leverage during salary negotiations. Measures that consider both financial and non-financial elements are key for long-term career satisfaction.
Salary Trends and Future Outlook
The examination of salary trends and future outlook is crucial for understanding the evolving landscape of data science manager compensation. This section delves into the elements that shape salary expectations, the implications for professionals in the field, and the dynamic nature of employment in data science. Observing these trends can assist individuals to make informed career decisions and negotiations.
Emerging Trends in Data Science Compensation
Several emerging trends are currently influencing compensation within the data science domain. Automation and artificial intelligence play a significant role in enhancing productivity, which in turn impacts salary structures. Companies often seek data science managers who can leverage these technologies to create efficient processes. As a result, those with skills in machine learning and advanced analytics may find their market value increasing.
Additionally, there is a noticeable rise in the demand for remote work opportunities. Organizations have adapted to a more flexible work paradigm, which often broadens the talent pool. This flexibility frequently corresponds with competitive compensation packages, as companies aim to attract top talent regardless of geographical constraints.
Lastly, the growing emphasis on data-driven decision-making across industries results in a higher demand for skilled professionals. This push increases salary benchmarks as businesses recognize the strategic advantages of employing effective data science managers.
Demand for Data Science Managers
The demand for data science managers continues to rise due to various factors. First and foremost, organizations are increasingly relying on data to guide their strategic decisions. As data becomes more central to business operations, the need for adept professionals to navigate this complexity grows.
According to recent statistics, the job market for data science roles is projected to expand significantly in the coming years. This growth indicates that companies are investing heavily in data initiatives, which directly impacts salary expectations for managerial positions. Firms are often willing to pay a premium for individuals who can demonstrate a solid track record in managing data-driven projects and teams.
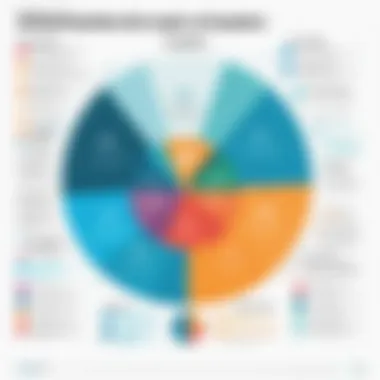
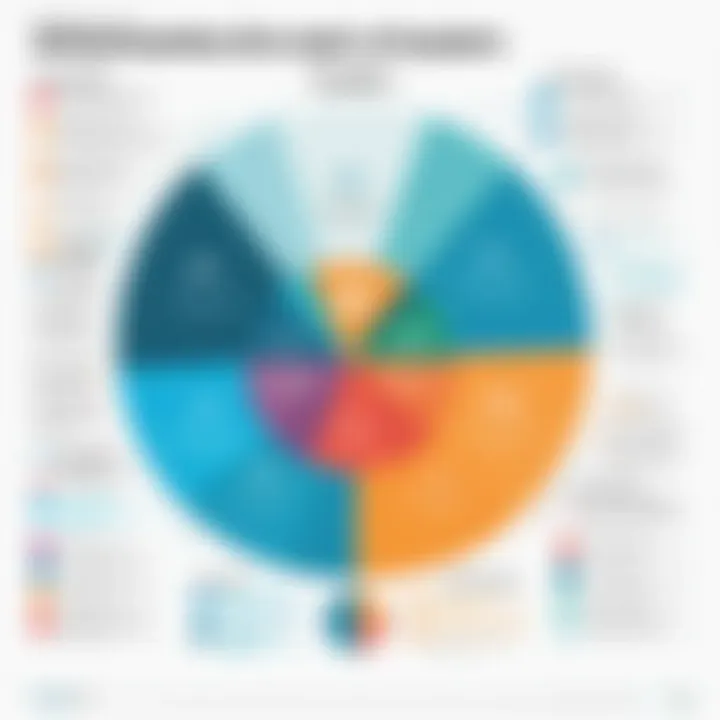
Moreover, the capability to align data science efforts with business goals is highly sought after. Data science managers who can communicate effectively with stakeholders and bridge technical expertise with business acumen often command higher salaries. The combination of leadership skills and technical know-how significantly enhances their value in any organization.
"As the demand for data science roles continues to grow, so does the salary potential for those in managerial positions."
Negotiating Salaries
Negotiating salaries is a critical aspect of career development for data science managers. It not only influences one's immediate financial compensation but can also impact long-term career prospects and job satisfaction. Understanding how to advocate for oneself in salary discussions can lead to better offers and alignment with market standards. Given the competitive landscape of data science, where skills are in high demand, being well-prepared to negotiate can set a candidate apart.
Understanding Your Market Value
Determining your market value involves several key factors. It requires research and analysis of current salary trends, which can vary significantly based on geographical location, industry, and experience level. Useful resources for this research include sites like Glassdoor and Payscale, where data science salaries are extensively covered.
Consider the following steps in assessing your value:
- Research Salary Ranges: Familiarize yourself with the average salaries for data science managers in your region and sector. Make sure to gather information on entry-level, mid-level, and senior positions to get a comprehensive view.
- Evaluate Your Skills and Experience: Take into account your specific technical skills, years of experience, and contributions to previous projects. Unique qualifications or certifications from recognized institutions can also elevate your worth.
- Industry Comparisons: Different sectors pay differently. Data science managers in technology firms may earn more than those in non-profits. Researching industry-specific salary information can provide critical insight.
Being equipped with this information allows for more informed discussions during salary negotiations, reinforcing the value you bring to the organization.
Strategies for Successful Negotiation
Successfully negotiating a salary requires strategic thinking and clear communication. Here are some effective tactics to employ:
- Set a Target Salary Range: Establish a salary range based on your market research and personal requirements. It’s essential to remain flexible but also assertive in your expectations.
- Practice Your Pitch: Prepare to articulate your value. Highlight your achievements, specific skills, and how they contribute to the company’s objectives. Being confident in your presentation can make a significant difference.
- Timing Matters: Initiate salary discussions when the company is performing well or during performance reviews, as this may increase the chances of a positive outcome.
- Don't Just Focus on Salary: Consider the entire compensation package, including bonuses, benefits, and work-life balance options. Sometimes the overall value may outweigh a higher base salary.
- Maintain Professionalism: Approach negotiations with professionalism. Be ready for pushback and maintain open lines of conversation. Showing willingness to find common ground can help foster a positive dialogue.
"Negotiation is not about victory or defeat; it's about reaching a mutually beneficial agreement."
By applying these strategies, data science managers can effectively negotiate salaries that reflect their true market value. This can lead to greater job satisfaction and career advancement, thus contributing positively to the broader landscape of data science management.
Case Studies: Salary Comparisons
Importance of Salary Comparisons
Case studies involving salary comparisons provide essential insights into the compensation landscape for data science managers. These studies allow potential candidates to understand how various factors converge to influence salaries. Factors such as geographic location, industry sector, and experience level manifest differently in each case. By analyzing real-world examples, it is possible to illuminate patterns that would otherwise be obscured by aggregate data alone.
Additionally, these comparisons serve as benchmarks for current professionals looking to evaluate their own compensation relative to others in the field. Candidates can leverage such data to negotiate better pay and benefits during their job searches. Thus, understanding salary comparisons is critical for anyone navigating or contemplating a career in data science management.
Profiles of Successful Data Science Managers
The profiles of successful data science managers reveal valuable lessons about career growth and salary trajectories. For instance, consider Janice, a data science manager at Google. Her journey involved starting as a data analyst, progressing through roles that emphasized technical skills and leadership. She holds a Master's degree from Stanford University, which contributed significantly to her career advancement.
Another example is Paul, a manager at a healthcare startup. His background in computational biology, combined with industry experience, helped him command a higher salary than peers in less specialized sectors. Both Janice and Paul's experiences highlight that continuous learning and adaptability foster significant salary gains in this competitive field.
Salary Breakdown by Location and Experience
Location plays a crucial role in determining salaries for data science managers. For example, in San Francisco, salaries can exceed $150,000 annually due to the high cost of living and competitive market. Conversely, a position in a smaller city may offer around $100,000 or less, showcasing a substantial variance based on geographic choices.
Similarly, experience significantly impacts salary. Here are some insights:
- Entry-Level: Data science managers with less than two years of experience may start at $80,000 to $100,000.
- Mid-Level: With five to seven years of experience, salaries can rise to between $120,000 and $150,000.
- Senior-Level: Those with over ten years often command salaries upwards of $160,000, especially in tech-centric hubs.
This shows that both location and experience are vital considerations when assessing potential earnings, making it clear why candidates must consider these factors when seeking employment opportunities.
The End
Understanding the compensation dynamics of data science managers is essential for professionals navigating their careers in this field. This article has appraised various factors influencing data science manager salaries, equipping readers with valuable insights. Through comprehensive analysis, the findings establish a framework for understanding how geographical location, industry sector, and individual qualifications contribute to salary variation.
Recap of Key Salary Insights
In summarizing the key salary insights, we observe that:
- Average Salaries: Generally, data science managers earn significantly higher than the average salary across many industries, often exceeding six figures.
- Experience Levels: Salaries show a clear progression path. Entry-level positions can expect lower compensation, while senior roles command much higher wages.
- Geographical Disparities: Salaries are notably higher in urban tech hubs such as San Francisco and New York compared to rural settings.
- Industry Differences: The tech sector tends to offer the highest salaries for data science managers, closely followed by finance and healthcare sectors.
To encapsulate these elements, today’s data science managers must continuously upgrade their skills to meet the evolving demands of their roles.
Paths Forward for Aspiring Data Science Managers
For those aspiring to become data science managers, several paths can be beneficial:
- Education and Training: Pursuing advanced degrees, such as a master's in data science or analytics, can greatly enhance earning potential. Certifications from recognized bodies also add significant weight to one’s profile.
- Gaining Diverse Experience: Engaging in roles that cover various aspects of data science, including analytics, machine learning, and project management, is advantageous. This broad experience can prepare candidates for the complexity of managerial tasks.
- Developstrong Networking Skills: Building a professional network can open doors to job opportunities and increase visibility in the industry.
- Continuous Learning: Staying updated with the latest technologies and methodologies is crucial. Data science is a rapidly changing field, and being adaptable can provide a competitive edge.