Unveiling the Crucial Role of a Data Science Architect in the Tech Realm
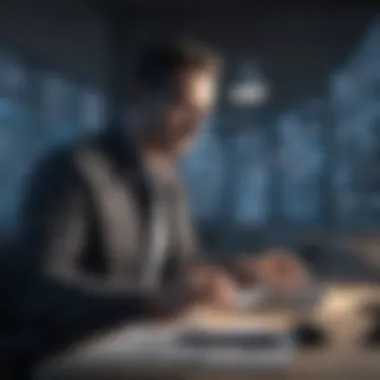
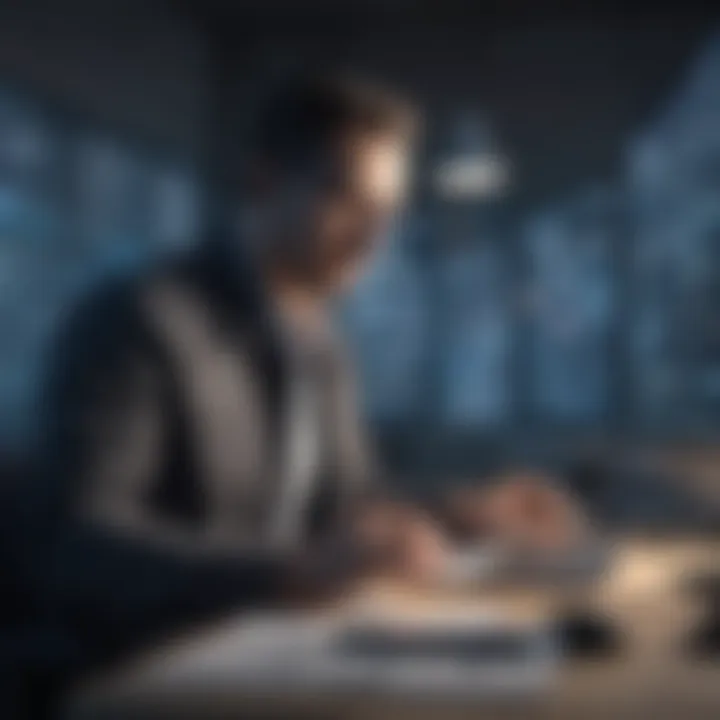
Tech Trend Analysis
In delving into the intricate world of data science architecture within the dynamic tech industry, it is paramount to examine the current trends shaping the landscape. The role of a data science architect transcends traditional data analytics, necessitating a profound understanding of emerging technologies and methodologies. As Big Data continues to revolutionize industries, data science architects stand at the forefront of harnessing its power for driving innovation and gaining strategic insights. Anticipating future trends is integral to their strategic vision, enabling them to navigate complexities and stay ahead of the technological curve.
Product Reviews
Within the realm of data science architecture, a scrutiny of the tools and technologies pivotal to a data science architect's repertoire is indispensable. From reviewing industry-leading data analysis platforms to exploring the nuances of machine learning algorithms, an in-depth analysis of each product's functionalities and compatibility within the data architecture ecosystem is imperative. This scrutiny extends to evaluating the performance, scalability, and user-friendliness of these tools, shedding light on their pros and cons. By providing nuanced recommendations based on empirical assessments, data science architects can optimize their toolkits for maximum efficiency and efficacy.
How-To Guides
For individuals aspiring to embark on a career in data science architecture or enhance their existing skill set, a comprehensive guide delineating the essential steps and strategies is paramount. From introducing fundamental concepts in data science to offering step-by-step instructions on building predictive models, this guide aims to equip enthusiasts with practical insights and expertise. In addition, garnering tips and tricks from seasoned professionals can augment learning outcomes, offering unique perspectives and enhancing problem-solving abilities. Addressing common troubleshooting dilemmas ensures a holistic understanding of data science architecture, empowering practitioners to overcome challenges proficiently.
Industry Updates
Amidst the rapid evolution of the tech industry, staying abreast of recent developments and trends is pivotal for data science architects. Analyzing market shifts, regulatory updates, and disruptive innovations enables them to adapt their strategies and methodologies effectively. Understanding the impact of these updates on businesses and consumers is intrinsic to making informed decisions and driving operational efficiencies. By synthesizing industry updates with practical insights and foresight, data science architects can chart a course for sustained success and innovation in an ever-evolving technological landscape.
Introduction
In the fast-evolving landscape of technology, the role of a Data Science Architect stands out as a pivotal one, driving innovation and spearheading data-driven strategies. This article will delve deep into the intricate responsibilities and skills required in this dynamic field. Understanding the essence of data science architecture is crucial to comprehending the transformative power it holds within the tech industry.
Navigating the complex terrain of big data, machine learning, and statistical analysis, a Data Science Architect bridges the gap between raw data and actionable insights. Their expertise in designing robust data architectures empowers organizations to glean valuable business intelligence, enhancing decision-making processes and predicting future trends with a high degree of accuracy. The significance of this role cannot be overstated, as it shapes the technological advancements that steer companies towards sustainable growth and competitive advantage.
Embarking on an exploration of the Definition of Data Science Architecture, we unravel the layers of intricacies involved in structuring data ecosystems to extract maximum value. Key Components such as Data Ingestion Systems, Data Storage Solutions, and Data Processing Frameworks form the backbone of any data architecture, highlighting the importance of meticulous planning and execution in ensuring seamless data flow and accessibility.
The subsequent discussion on the Role of Data Science Architect in Designing Architecture illuminates the strategic vision and critical thinking prowess demanded by this position. From conceptualizing data strategies to optimizing data governance frameworks, a Data Science Architect operates at the intersection of technology and business, driving excellence and fostering a culture of innovation.
Understanding Data Science Architecture
Understanding Data Science Architecture is fundamental in grasping the intricate role of a data science architect within the tech industry. This section sheds light on the core concepts related to data science architecture, emphasizing its significance in developing innovative data solutions, and leveraging big data for valuable business insights. Delving into Understanding Data Science Architecture provides readers with a comprehensive outlook on the framework that underpins data science initiatives in the tech realm.
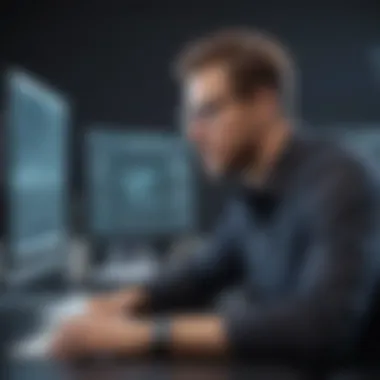
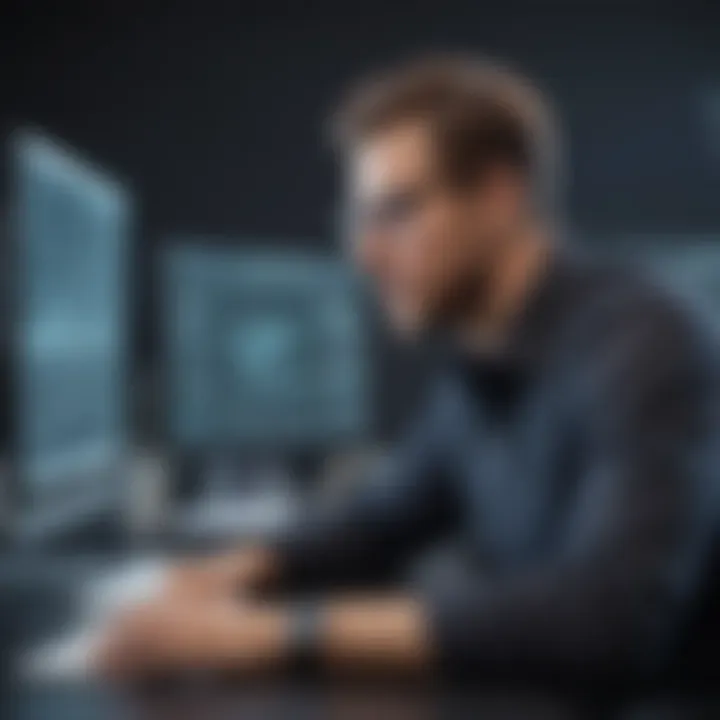
Definition of Data Science Architecture
Data Science Architecture refers to the overall structure and system design that facilitates the collection, storage, processing, and analysis of large volumes of data for deriving meaningful insights. It encompasses the integration of various technologies and methodologies to create a robust data ecosystem that supports data-driven decision-making processes within an organization.
Key Components of Data Science Architecture
Data Ingestion Systems:
Data Ingestion Systems play a crucial role in efficiently collecting and importing data from diverse sources into the data ecosystem. They streamline the process of gathering data, ensuring its accurate and timely integration into the analytical systems. Data Ingestion Systems are characterized by their ability to handle vast amounts of data in different formats, making them a preferred choice for organizations aiming to capitalize on their data assets. Despite their efficiency, Data Ingestion Systems may face challenges in handling real-time data streams seamlessly, which can impact data quality and processing speed.
Data Storage Solutions:
Data Storage Solutions are essential components of Data Science Architecture, providing a scalable and secure infrastructure for storing and managing large datasets. These solutions enable data persistence, accessibility, and retrieval, supporting analytical processes and ensuring data integrity. The key characteristic of Data Storage Solutions lies in their capacity to handle massive data volumes efficiently, making them indispensable for organizations dealing with big data. However, while offering high performance and scalability, Data Storage Solutions may pose challenges related to data redundancy and storage costs.
Data Processing Frameworks:
Data Processing Frameworks form the backbone of data analytics operations, offering tools and resources for processing, transforming, and analyzing data. These frameworks enable data scientists to perform complex computations, extract insights, and derive patterns from large datasets effectively. The primary advantage of Data Processing Frameworks lies in their distributed computing capabilities, which enhance processing speed and scalability. Nevertheless, selecting the appropriate framework and optimizing it for specific tasks can be a daunting task, requiring expertise and careful consideration from data science architects.
Role of Data Science Architect in Designing Architecture
The role of a Data Science Architect in designing data architecture is paramount to the successful implementation of data science projects. These professionals are responsible for conceptualizing and developing data strategies, overseeing data governance frameworks, collaborating with cross-functional teams, and driving innovation in data science initiatives. By leveraging their technical proficiencies, leadership skills, and domain knowledge, data science architects play a pivotal role in shaping the architecture that underpins modern data-driven practices in the tech industry.
Skills and Qualifications of a Data Science Architect
In the dynamic landscape of the tech industry, the paramount significance of acquiring diverse skills and qualifications as a Data Science Architect cannot be overstated. The convergence of technical proficiencies, leadership acumen, and effective communication prowess defines the core competencies expected from a Data Science Architect. These multidimensional skills and qualifications are not merely desirable but are imperative for navigating the complexities of modern data science. The blend of domain expertise and versatile skills equips Data Science Architects to lead with insight and innovation.
Technical Proficiencies
Machine Learning
Exploring the realm of Machine Learning illuminates a foundational aspect of Data Science Architecture. Machine Learning, a subset of artificial intelligence, empowers data systems to autonomously learn and improve from experience without explicit programming. Its ability to analyze vast datasets and derive meaningful insights makes it a cornerstone in contemporary data science endeavors. The adaptability and scalability of Machine Learning algorithms render them invaluable for predictive modeling, data pattern recognition, and decision-making processes within data architecture. Despite its computational intensity, the predictive capabilities of Machine Learning algorithms bolster the efficacy of data-driven strategies, enhancing predictive analytics and optimizing operations.
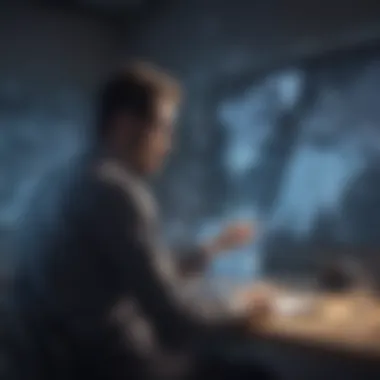
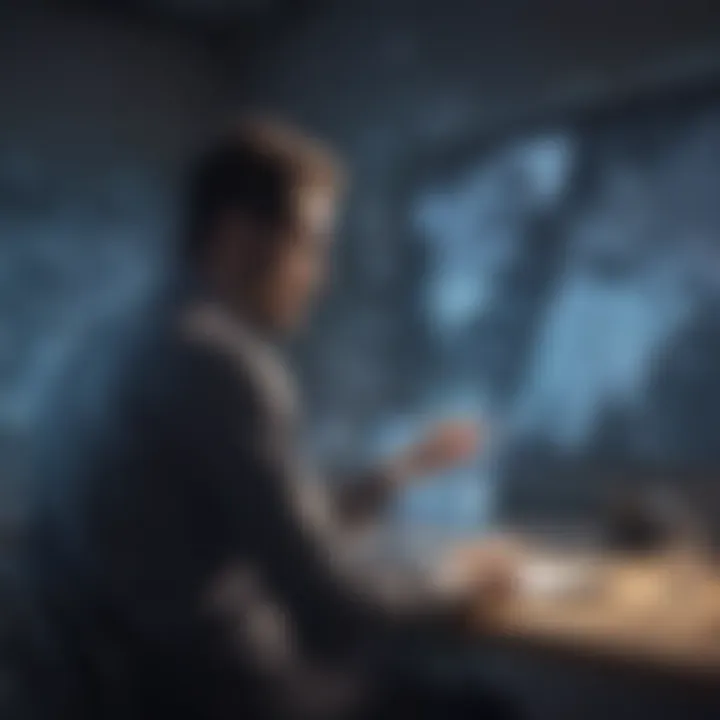
Data Visualization
Data Visualization serves as a pivotal tool for Data Science Architects in elucidating complex data sets into visually compelling representations. By leveraging graphical elements such as charts, graphs, and infographics, Data Visualization enhances data comprehension and communication, fostering informed decision-making. The visual narrative constructed through Data Visualization enables stakeholders to identify trends, outliers, and patterns within data sets, thereby facilitating strategic insights and actionable data-driven recommendations. The interactive nature of Data Visualization tools amplifies user engagement and augments data exploration, allowing for immersive data storytelling and user interaction.
Statistical Analysis
Statistical Analysis constitutes an indispensable component of the Data Science Architect's skill set, underpinning the rigorous evaluation and interpretation of data. By employing statistical methods such as hypothesis testing, regression analysis, and significance testing, Data Science Architects derive robust conclusions and insights from empirical data. Statistical Analysis enables the quantification of uncertainty, identification of relationships between variables, and validation of data-driven hypotheses, providing a systematic framework for data exploration and decision support. The statistical rigor applied by Data Science Architects ensures the reliability and validity of analytical findings, fortifying the evidential basis for strategic recommendations and business decisions.
Leadership and Communication Skills
"Connecting the technical acumen of data science with the human element of leadership and communication is where the Data Science Architect excels. Effective leadership empowers teams to collaborate seamlessly, navigate complex challenges, and drive innovation in data-driven projects. Beyond technical expertise, strong leadership skills encompass strategic vision, team motivation, and conflict resolution, cultivating a cohesive and high-performing data team. The ability to communicate insights, recommendations, and outcomes clearly and persuasively to stakeholders is paramount for translating data complexities into actionable strategies and ensuring organizational alignment on data initiatives."
Educational Background
"The educational foundation of a Data Science Architect is instrumental in shaping their expertise and proficiency in data science disciplines. While diverse paths may lead to a career in data architecture, formal education in computer science, statistics, mathematics, or a related field provides the requisite knowledge and theoretical framework for data analysis and interpretation. Advanced degrees such as a Master's in Data Science or Computer Engineering offer specialized training in data modeling, machine learning algorithms, and statistical methods, augmenting the analytical capabilities of Data Science Architects. Continuous learning, professional certifications, and participation in data science workshops further enrich the educational background of Data Science Architects, ensuring ongoing skill development and adaptability to evolving industry trends."
Responsibilities of a Data Science Architect
In the realm of data science architecture, the role of a Data Science Architect holds immense significance. The Responsibilities of a Data Science Architect encompass a wide array of crucial tasks that drive the successful implementation of data-related projects. One key element involves developing data strategies that align with the overarching business objectives and goals. By meticulously formulating data strategies, the Data Science Architect sets the foundation for leveraging data effectively to derive actionable insights and drive decision-making processes. Moreover, through their expertise and strategic foresight, these professionals play a pivotal role in shaping how organizations harness the power of data in a rapidly evolving technological landscape.
Developing Data Strategies
Developing data strategies is a fundamental aspect of a Data Science Architect's role. This task involves crafting detailed plans to manage and utilize data effectively to meet organizational objectives. It includes outlining methods for data collection, analysis, and utilization to derive valuable insights that can propel a business forward. By developing comprehensive data strategies, Data Science Architects ensure that data processes are streamlined, efficient, and aligned with the organization's overarching goals. Additionally, these strategies lay the groundwork for implementing advanced data analytics techniques, enhancing operational efficiency, and maximizing the potential of available data resources.
Overseeing Data Governance
Overseeing data governance is a critical responsibility that falls within the purview of a Data Science Architect. Data governance entails establishing policies, procedures, and controls to ensure the accuracy, security, and integrity of organizational data. By overseeing data governance practices, Data Science Architects guarantee that data is managed in a compliant manner, adhering to regulatory standards and best practices. This oversight helps mitigate risks related to data breaches, privacy concerns, and data quality issues, ultimately safeguarding the organization's valuable information assets.
Collaborating with Cross-Functional Teams
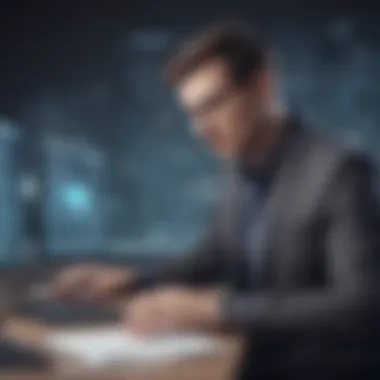
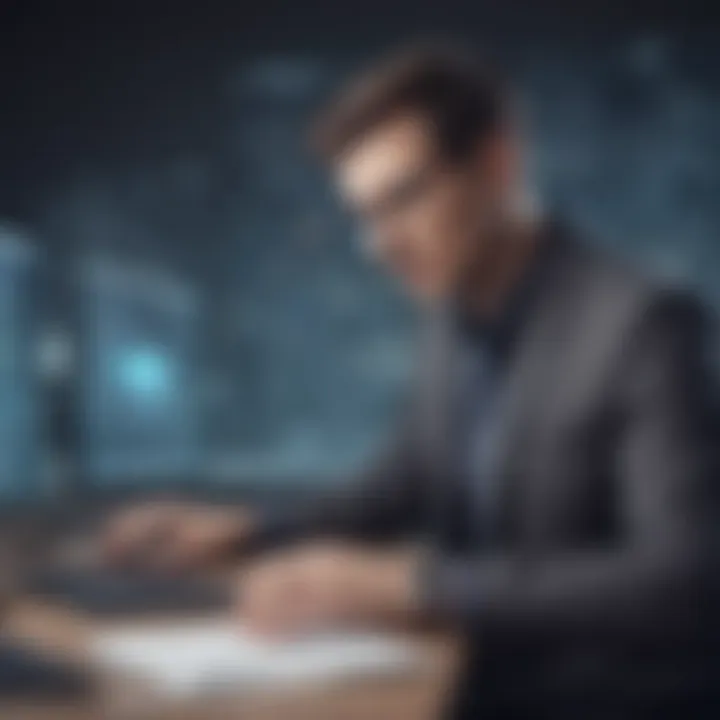
Collaborating with cross-functional teams is essential for a Data Science Architect to facilitate the seamless integration of data-driven initiatives across different departments. Effective collaboration involves working closely with stakeholders from diverse functional areas such as IT, operations, marketing, and finance to ensure that data projects align with the organization's overall business objectives. By fostering collaboration and open communication, Data Science Architects can leverage the expertise of multidisciplinary teams to drive innovation, solve complex problems, and achieve transformative outcomes through data-driven decision-making processes.
Driving Innovation in Data Science Projects
Driving innovation in data science projects is a core aspect of a Data Science Architect's role. By spearheading innovation initiatives, these professionals drive the development of groundbreaking solutions that harness the power of data to drive business growth and competitive advantage. Through a combination of technical expertise, creative thinking, and strategic vision, Data Science Architects pave the way for transformative projects that push the boundaries of traditional data analytics. By driving innovation, these professionals play a key role in shaping the future of data science architecture and driving technological advancements in the ever-evolving landscape of the tech industry.
Challenges Faced by Data Science Architects
Data Science Architects encounter a multitude of challenges in the dynamic landscape of the tech industry, shaping the discourse surrounding data-driven decision-making and innovation. These challenges underscore the critical role played by these professionals in leveraging data effectively and ethically. By navigating through these hurdles, Data Science Architects elevate the significance of data science within organizations, fostering enhanced strategic decision-making and sustainable growth.
Data Security and Privacy Concerns
Data Security and Privacy Concerns stand as paramount considerations for Data Science Architects, given the sensitivities surrounding data handling and usage. Ensuring the confidentiality and integrity of data is imperative to maintain the trust of stakeholders and comply with regulatory requirements. Mitigating risks associated with data breaches and privacy violations demands robust security measures, encryption protocols, and adherence to data protection laws. Data Science Architects must intricately weave security frameworks into architectural designs to fortify data assets against cyber threats and unauthorized access.
Scaling Data Infrastructure
Scaling Data Infrastructure poses a considerable challenge for Data Science Architects due to the exponential growth of data volumes and complexities within systems. Balancing performance, scalability, and cost-efficiency while expanding data infrastructure necessitates strategic planning and implementation. Scalability issues such as storage limitations, processing delays, and network bandwidth constraints require innovative solutions like cloud computing, distributed storage, and parallel processing architectures. Data Science Architects must architect scalable infrastructure that can adapt to evolving business needs and accommodate surges in data velocity, variety, and veracity.
Keeping Abreast of Rapid Technological Changes
Data Science Architects confront the relentless pace of technological advancements, necessitating continuous learning and upskilling to remain relevant in the competitive tech landscape. Staying updated on emerging tools, frameworks, and methodologies is essential for driving innovation and optimizing data processes effectively. Adapting to new paradigms like edge computing, AI algorithms, and automation tools is crucial for enhancing data capabilities and staying ahead of market trends. Data Science Architects need to embrace a growth mindset, establish learning networks, and collaborate with industry peers to stay abreast of rapid technological changes and harness the full potential of data science for organizational success.
Future Trends in Data Science Architecture
In the rapidly evolving landscape of data science architecture, staying ahead of the curve is imperative for professionals in the tech industry. Future trends in data science architecture play a pivotal role in shaping the strategic direction of organizations and ensuring maximum efficiency in data-driven decision-making processes. Understanding and embracing these trends can give data science architects a competitive edge in a dynamic market.
Integration of AI and Machine Learning
The integration of artificial intelligence (AI) and machine learning represents a significant advancement in data science architecture. AI algorithms allow systems to learn from data, identify patterns, and make decisions with minimal human intervention. By integrating AI and machine learning technologies, data science architects can enhance data processing capabilities, improve predictive analytics, and automate complex tasks. This fusion opens doors to innovative solutions that optimize business operations and drive sustainable growth in the tech industry.
Emphasis on Real-time Data Processing
Real-time data processing has emerged as a key trend in data science architecture, enabling organizations to access and analyze data instantaneously. The ability to make decisions in real-time based on up-to-the-minute information is crucial in a fast-paced business environment. Data science architects are leveraging real-time data processing tools to gain valuable insights, detect anomalies, and respond promptly to changing market conditions. This trend ensures that organizations can capitalize on opportunities swiftly and mitigate risks effectively, enhancing overall operational efficiency and competitiveness.
Advancements in Data Visualization Techniques
Advancements in data visualization techniques are transforming the way data science insights are communicated and interpreted. Visual representations of complex data sets make it easier for stakeholders to grasp key trends, patterns, and correlations within the data. Data science architects are utilizing innovative data visualization tools to create interactive dashboards, heat maps, and graphs that provide actionable insights at a glance. By leveraging advanced data visualization techniques, organizations can streamline decision-making processes, foster data-driven cultures, and improve overall strategic planning. This trend underscores the importance of presenting data in a clear, concise manner to facilitate better understanding and informed decision-making.