Understanding the Role of a Data Management Lead
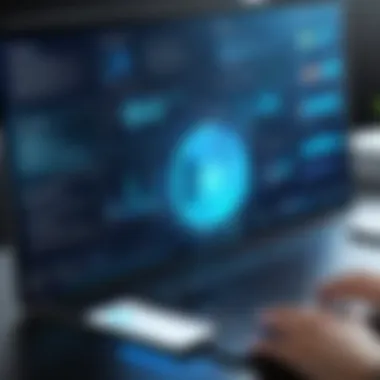
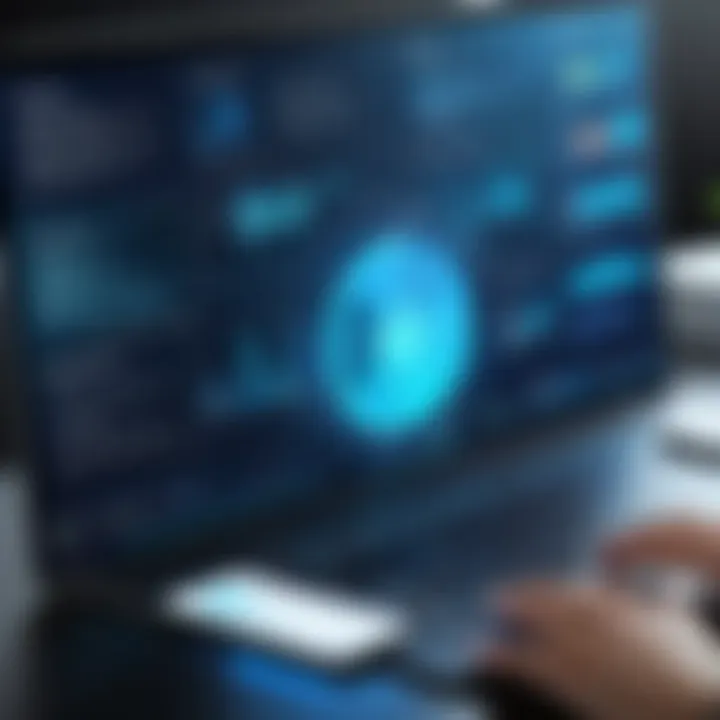
Intro
In today’s fast-paced, data-driven landscape, the role of a Data Management Lead is becoming increasingly pivotal. Organizations strive to harness data effectively to improve operations, inform decisions, and gain a competitive edge. This exploration will pull back the curtain on the responsibilities that come with this position and the expectations surrounding it.
Understanding how to manage data, secure it, and ensure its integrity is not merely a technical necessity; it’s a strategic imperative enriching the broader corporate tapestry. In this article, we will delve into the skills that mark the difference between a competent Data Management Lead and an outstanding one, the intricacies of data management strategies, and how collaboration sparks innovation across departments.
It’s essential to appreciate the complexity of this role as it straddles not only the technical requirements but also the softer skills necessary for fostering collaboration and communication throughout an organization.
Overview of Data Management Leadership
When diving into the realm of data management, the idea of leadership cannot be brushed aside. The role of a Data Management Lead is not just a position; it serves as the backbone of an organization’s data strategy. Here, we unpack the meaning and significance of this pivotal role.
Definition of Data Management Lead
A Data Management Lead is essentially the conductor of an orchestra where data is the music. Their main objective is to ensure that data flows harmoniously across the organization, facilitating effective decision-making and operational efficiency. In simpler terms, they are the ones who mold and manage the data landscape, ensuring everything is in sync.
In practical terms, this means that a Data Management Lead works closely with various teams to define data governance structures, create policies, and oversee the use of data analytics tools. They are equipped with a defined set of responsibilities that ranges from establishing best practices to leading data-driven initiatives. This role is not only technical but also heavily strategic.
Importance of the Role
The importance of a Data Management Lead cannot be overstated. Think of them as the bridge between raw data and actionable insights. With the digital landscape transforming at breakneck speed, effective data management is crucial for organizations aiming for success. Here are some specific benefits and considerations that go hand-in-hand with this role:
- Data Integrity: The lead is crucial in maintaining the accuracy and consistency of data. This is particularly very vital, as decisions are often based on data analytics, and flawed data can lead to catastrophic consequences.
- Compliance and Security: In a world rife with data privacy regulations such as GDPR, having a knowledgeable guide at the helm helps navigate these waters effectively.
- Strategic Decision-Making: A skilled Data Management Lead not only oversees data processes but also contributes to crafting strategies that align business objectives with data capabilities. This role ensures that data plays an integral part in shaping business direction.
With the right leadership in place, organizations can harness the true power of their data, transforming it into a competitive advantage.
In summary, as we delve deeper into the nuts and bolts of what makes an effective Data Management Lead, we will explore the core responsibilities and the necessary skills they need to excel in this demanding environment.
Core Responsibilities
The core responsibilities of a Data Management Lead are crucial for ensuring that an organization's data practices align with strategic goals. This role serves as a linchpin in the data landscape, ensuring that data is not only collected but also stewarded in a way that maximizes its potential. It blends oversight and execution, embracing a holistic view that wraps around various data-centric functions. A well-defined outline of these responsibilities not only helps in guiding a Data Management Lead but also sets the stage for effective collaboration across the board. Here’s a deeper look into the fundamental areas of focus:
Strategic Data Governance
Establishing Policies
Establishing data governance policies lays down the framework for how an organization approaches data management. It's not just about setting rules; it’s about creating a culture that values data. The core aspect here is ensuring that policies address both regulatory compliance and internal needs effectively. This is a beneficial choice for any organization because strong policies can protect against data mishaps and compliance issues. A unique feature of this task often lies in the need for adaptability; as laws and technologies evolve, so too must the policies. While there's an advantage in having clear guidelines, an overly rigid policy can stifle innovation or quick adaptations.
Implementing Standards
Implementing data management standards is essential to create uniformity across various processes. This involves integrating best practices that can lead to higher quality data outcomes and also streamline operations. The key characteristic of this task is its foundational role in data integrity. Adopting consistent standards can be hugely beneficial, as it enables teams to work from the same playbook, enhancing collaboration. However, it also poses challenges; the unique feature lies in the balancing act between enforcing standards and allowing flexibility for teams to innovate. If done poorly, it may lead to frustration among team members, whereas a thoughtful approach can foster a more cohesive environment.
Data Quality Management
Monitoring Data Integrity
Monitoring data integrity involves keeping a vigilant eye on data as it flows through systems. It plays a pivotal part in maintaining the accuracy and reliability of information used for decision-making. The key feature of this responsibility is its proactivity; it’s about catching issues before they snowball into larger problems. Being adept at this can prevent costly errors and reinforce trust in data among stakeholders. However, while it’s a beneficial practice, it can also be resource-intensive and may require specialized tools and personnel to manage effectively.
Conducting Audits
Conducting audits stands as a necessary aspect of assessing data quality and compliance. It serves to verify that the data used within the organization adheres to established policies and standards. The unique characteristic of audits is their periodic nature, which means they offer snapshots of data health at intervals, thus allowing teams to adjust practices as needed. This aspect is beneficial as it brings clarity to data processes and can highlight areas for improvement or training needs. Conversely, audits sometimes face resistance from teams, as they may be viewed as disruptions or as a lack of trust in day-to-day operations. Balancing informative feedback with constructive dialogue is key here.
Collaboration with Stakeholders
Interdepartmental Communication
Effective interdepartmental communication is essential in fostering an organizational culture that prioritizes data. This responsibility emphasizes the importance of open channels among different teams. The key aspect is building context and understanding across departments, as data doesn’t exist in silos. This is a popular approach since it enhances synergy and leads to quicker problem resolutions. However, one unique feature to consider is that sometimes, communication can become muddied with jargon and technical terms unfamiliar to non-technical staff. Striking the right balance and ensuring clarity can make all the difference.
Building Partnerships
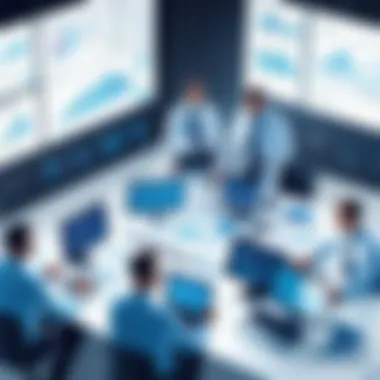
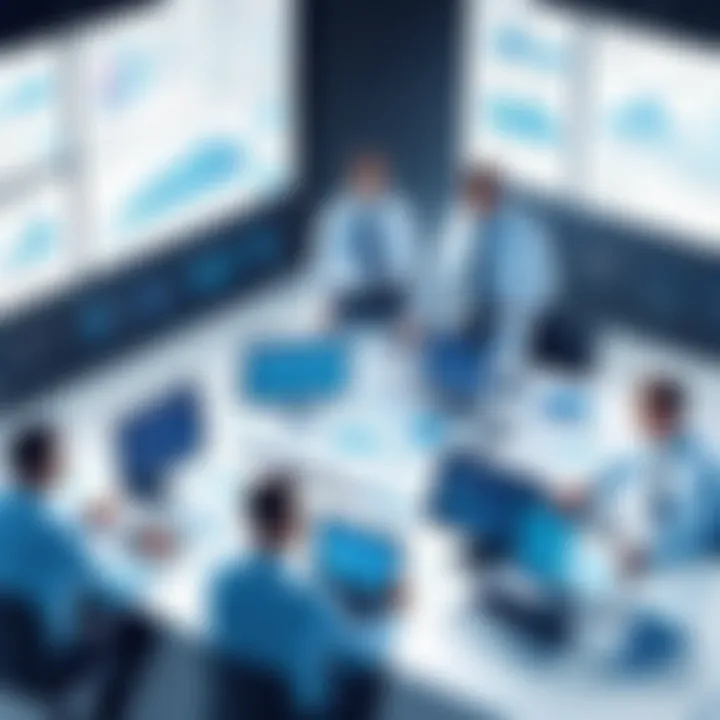
Building partnerships, both within the organization and externally, enriches the data management ecosystem. This involves understanding stakeholder needs and aligning data strategies accordingly. A key characteristic of this task is its relational focus; it’s not just about transactions but about cultivating long-term collaboration. This is a beneficial choice, as it leads to stronger alliances, ensuring that data efforts are supported at all levels. On the flip side, one challenge here can be the divergence of goals among partners. Navigating these differing objectives while maintaining a unified data strategy is crucial for sustainable success.
Required Skills and Qualifications
In the fast-paced realm of data management, having the right skills isn't just important; it's crucial. A Data Management Lead needs to embody a mix of technical acumen, analytical prowess, and social finesse. In this section, we'll dive into what these skills look like and why they're the bedrock of success in the position. Each aspect contributes to ensuring data is treated not just as numbers and text but as a vital asset for the organization.
Technical Skills
Proficiency in Data Management Tools
When a Data Management Lead is proficient in data management tools, it can make a world of difference. Proficiency here means being able to leverage these tools effectively to collect, store, and analyze data. Some commonly used tools include SQL databases, Tableau for visualization, and ETL tools like Talend.
The key characteristic of being adept with these tools lies in the ability to handle large datasets seamlessly. This capability helps in addressing challenges related to data storage and retrieval.
One unique feature of these data management tools is their ability to integrate with various data sources. This integration is extremely advantageous because it enables a consolidated view of data across the organization, providing a single source of truth. However, a disadvantage could be that learning curve often differs from tool to tool, which can take time for new users to overcome.
Understanding Data Architecture
Understanding data architecture is like having a roadmap in a city you've never been to. It allows a Data Management Lead to visualize how different data assets are structured, ensuring effective data utilization. This knowledge includes understanding databases, data warehouses, and the overall flow of data within the organization.
The key characteristic of this understanding is the insight it grants into how data can be organized and accessed efficiently. It encourages a data-driven approach to management by mapping out relationships and dependencies between datasets.
A unique feature of data architecture is its adaptability to business changes. Organizations may evolve, and so do their data needs; a well-designed architecture can accommodate this fluidity. One downside is that it can be a complex system to manage if not structured properly, leading to inefficiencies.
Analytical Abilities
Data Analysis Techniques
Data analysis techniques are essential for ferreting out insights from raw data. Employing methods like regression analysis, clustering, or machine learning, a Data Management Lead can extract actionable insights. This allows organizations to understand their data trends better, ultimately leading to informed decision-making.
The key characteristic of these techniques lies in their ability to transform ambiguous data into a coherent narrative. By effectively employing these techniques, organizations can stay ahead of their competitors.
One unique feature is that some analysis techniques can automate data interpretation, saving time and resources. However, the downside can be over-reliance on automation, which might overlook nuances that require human judgment.
Problem-Solving Skills
Problem-solving skills are indispensable in tackling data-related challenges that arise day-to-day. A Data Management Lead often encounters issues like data quality discrepancies or integration roadblocks. Thus, they need to think critically and navigate around obstacles effectively.
The key characteristic of strong problem-solving skills is the ability to develop creative solutions under pressure. This quality enables the Data Management Lead to maintain the flow of data operations without a hitch.
A unique feature of this skill is the emphasis on collaboration; good problem-solvers can rally teams to brainstorm and come up with comprehensive solutions. However, it can also lead to analysis paralysis when a plethora of options are on the table, delaying decisions.
Soft Skills
Leadership Qualities
In any organization, effective leadership can determine the success of a team. For a Data Management Lead, having solid leadership qualities is pivotal when guiding teams through the complexities of data management. This means being inspirational, approachable, and decisive.
The key characteristic of strong leadership is the ability to foster a collaborative culture where team members feel valued. For instance, by actively seeking input from team members on data strategies, a leader can spark innovation.
A unique feature of effective leadership in data management is the capacity to mentor and nurture talent within the organization. However, one downside could be navigating through differing opinions, which can sometimes create friction.
Effective Communication
Effective communication is the oil that keeps the mechanical parts of any organization running smoothly. For a Data Management Lead, translating complex data concepts into layman's terms is an essential skill. This means they can engage with both technical teams and non-technical stakeholders alike.
The key characteristic is the ability to build rapport while conveying essential information. When a Data Management Lead can articulate strategy and data findings, they reinforce the importance of data across the board.
A unique feature is that effective communication fosters a culture of transparency, which is essential, especially when sharing sensitive data observations. Yet, the downside can be situations where miscommunication leads to misunderstandings, emphasizing the need for clarity.
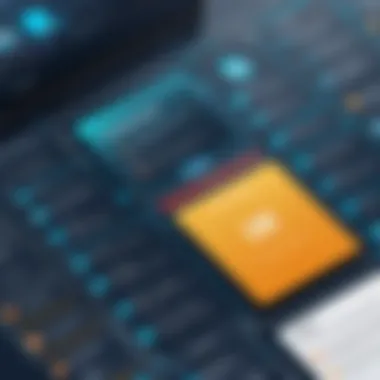
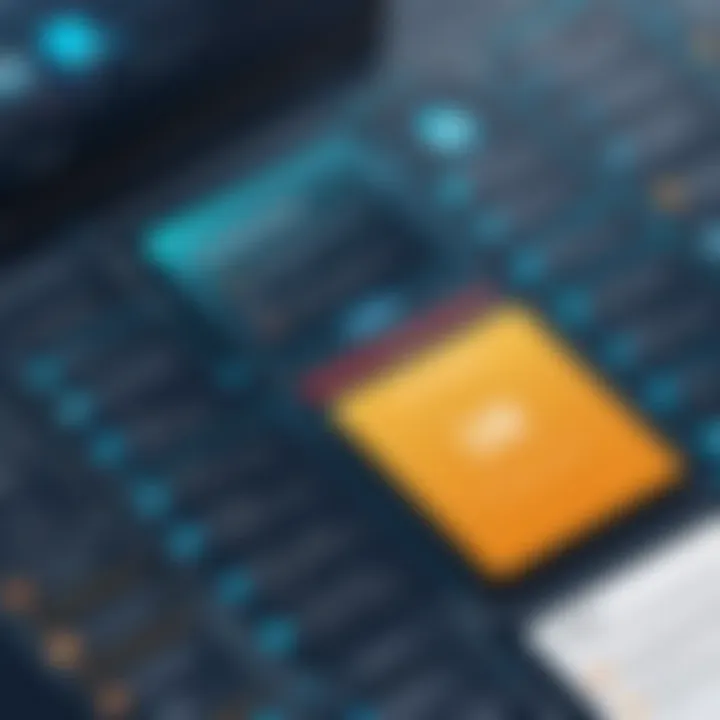
Challenges in Data Management
Data management might seem like a clean field where everything is organized and aligned, but it’s as messy as a toddler’s art project. There are numerous hurdles that data management leads must navigate, especially in today’s fast-paced digital environment. Understanding these challenges is crucial for anyone looking to become adept in this role. A data management lead’s ability to tackle these issues head-on can define the success of their organization’s data strategy and policy implementation.
Data Privacy Regulations
Compliance with GDPR
The General Data Protection Regulation, commonly known as GDPR, is a game changer in data management. It mandates strict consent protocols on how companies handle personal data of EU citizens. For data management leaders, compliance with GDPR isn't just a checkbox; it’s a substantial aspect that can shape organizational strategies. The pressing characteristic of GDPR is its emphasis on user privacy and data protection. This got companies scrambling to put measures in place to secure personal data, and non-compliance could lead to hefty fines – we're talking up to 20 million euros or 4% of a company’s global revenue.
The unique feature of GDPR is its territorial scope; it doesn’t matter where the company operates. If they handle the data of EU residents, they are fair game to these regulations. This promotes a culture of accountability and transparency across organizations, benefiting customers who feel their rights are being respected. However, for businesses, this comes with its own set of challenges. Navigating through compliance complexities can be cumbersome and could stifle operational agility.
Handling Data Breaches
The unfortunate reality is that data breaches are becoming more and more commonplace. For a data management lead, the ability to handle these situations is critical. Efficiently managing a data breach communicates an organization's competence and responsibility to stakeholders. A key characteristic here is the necessity for an established response plan; without one, companies risk their reputation and customer trust.
What sets a robust data breach management plan apart is its proactive rather than reactive approach. Immediate containment, thorough investigation, and transparent communication with affected parties are unique features that define an effective strategy. The advantages of having a solid plan are manifold: it minimizes losses, preserves customer trust, and, in many cases, can actually help mitigate legal repercussions. On the flip side, if a company neglects this aspect, the fallout can be severe, affecting not just data integrity but also the brand’s image.
Technological Changes
Adapting to New Tools
The landscape of data management is ever-changing, almost like a chameleon adjusting to its surroundings. Adapting to new tools can seem like a Sisyphean task, yet it’s essential for any data management lead. New tools often come with advanced functionalities which could revolutionize an organization's data processes. They enable better storage solutions, robust analytics capabilities, and enhanced security measures.
Successful adaptation doesn’t happen overnight; it requires ongoing training and a willingness to embrace the unfamiliar. The unique feature of this adaptability is the evolution of the workforce. A team that constantly evolves their skills with the tools makes their data management practices not just functional but also innovative. However, the challenge lies in maintaining productivity while implementing new systems, a balancing act many find difficult to perform.
Staying Current with Trends
Moreover, staying current with trends is not just a nice-to-have; it’s a must-have, especially in tech. For data management leads, ignoring upcoming trends can be detrimental. The data landscape is burgeoning with advancements in artificial intelligence, machine learning, and cloud computing, all of which are shaping how we handle data.
Following these trends allows organizations to leverage cutting-edge technologies. A proactive approach to learning about the latest innovations can yield competitive advantages, making companies more agile in their strategies. However, the downside to this is the risk of information overload. Too much information without guidance could lead to paralysis by analysis; effectively implementing insights is the antidote to this dilemma.
In a world inundated with data, the smart management of that data determines the pace and efficiency of any organization’s growth.
When paired together, these challenges create a complex environment requiring deft navigation and strategic foresight. Solving these challenges is paramount not just for safeguarding data but also for enhancing overall organizational success.
Impact on Organizational Success
A Data Management Lead plays a critical role in bridging the gap between data and decision-making processes within an organization. Their influence extends far beyond simple data oversight; it reaches into the very heart of strategic planning and operational efficiency. By effectively managing data, these leads turn raw numbers into actionable insights, directly impacting organizational success.
Data-Driven Decision Making
Enhancing Business Strategies
At the core of enhancing business strategies is the ability for organizations to leverage data to make informed choices. This aspect is crucial because it ensures that decisions aren't just made based on gut feelings but are backed by solid evidence. A key characteristic of enhancing business strategies is the transformation of analytical data into strategic initiatives. For instance, by analyzing market trends and customer behaviors, data management leads can identify new opportunities that resonate more effectively with target audiences.
The unique feature of enhancing business strategies lies in its adaptability. Businesses that utilize precise data insights are more nimble and can respond to changes in the market swiftly. The advantages of this approach include increased market share and improved customer satisfaction; however, there can be disadvantages, such as the potential for over-reliance on data, leading to paralysis by analysis.
Improving Efficiency
Improving efficiency is another pillar of how data management leads can drive success in organizations. It encompasses optimizing processes and eliminating redundancies by utilizing data analysis. The critical characteristic here is the identification of bottlenecks in workflows through data-driven insights. For example, a company might identify that certain tasks in its supply chain take longer than necessary due to lack of proper data tracking.
The unique capability of improving efficiency is its capacity to create a culture of continuous improvement. With a focus on efficiency, organizations can achieve better resource allocation and reduced costs. Yet, this also comes with its own set of challenges—implementing new data management practices requires time and employee buy-in, which can be difficult in established workflows.
Supporting Business Objectives
Aligning Data Strategies with Goals
Aligning data strategies with business objectives is mental yoga that requires careful balancing. It ensures that data management efforts sync with the broader aims of the organization. The highlight of this process is that it cultivates a sense of purpose in data initiatives that goes beyond just compliance or maintenance tasks. By actively aligning data strategies with organizational goals, companies can maximize the value derived from their data assets.
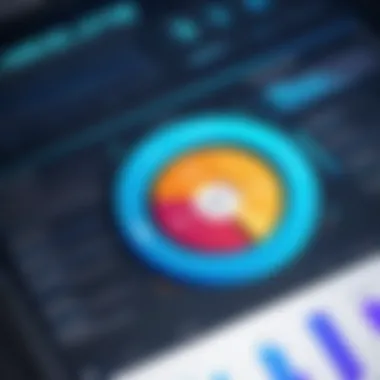
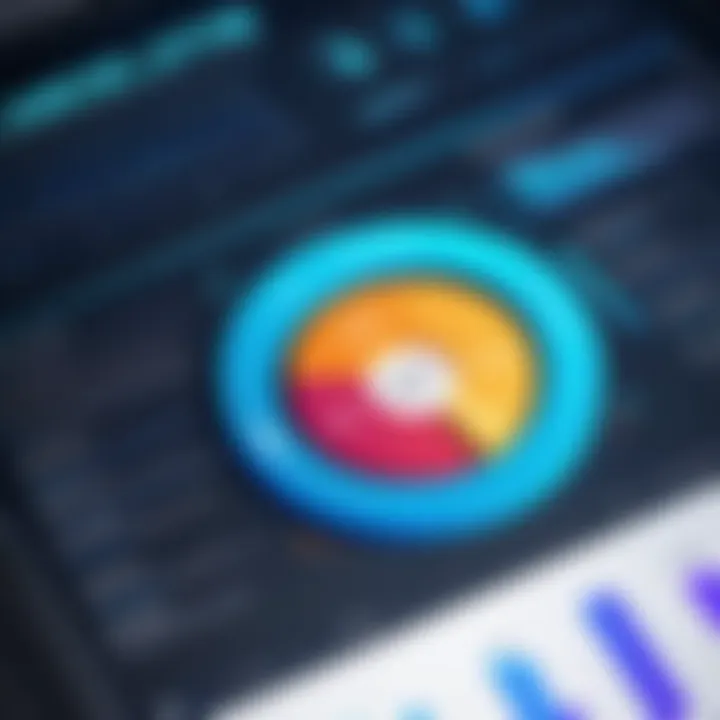
A unique feature here is the establishment of key performance indicators (KPIs) specific to data management initiatives. These KPIs not only help in tracking progress but also make teams accountable for their data-related outcomes. Advantages include clearer direction and improved focus; however, one must tread lightly as misalignment can lead to wasted resources and missed targets.
Measuring Performance
Measuring performance is integral to understanding how well an organization is meeting its data management goals. This aspect allows for an ongoing assessment of both processes and results, which can highlight areas needing attention. The key characteristic here is the continuous feedback loop incorporated into performance measuring. By analyzing datasets over time, organizations can refine their strategies and improve overall performance.
The unique trait of measuring performance lies in its capacity to build a responsive ethos within teams. With readily available data insights, teams can pivot swiftly when necessary. The benefits of this approach include sustained growth and informed decision-making, but it does present challenges in terms of ensuring accuracy and relevance in the metrics used.
Career Path and Advancement
The journey of a Data Management Lead is quite an intricate one. Numerous levels and roles exist, each building upon the last, adding layers of expertise and knowledge that contribute to the overall effectiveness of data management within an organization. This section addresses the significance of career progression in this domain. It illustrates how understanding the various entry points and senior roles can aid both aspiring data professionals and organizations in crafting clear career trajectories.
Entry-Level Positions
Data Analyst Roles
The Data Analyst role serves as a crucial starting point for many professionals in the data management landscape. An analyst typically digs deep into raw data, transforming it into insights that can guide business decisions. One key characteristic of this position is its focus on analytical skills—using various statistical tools and techniques, data analysts help decode patterns and trends within vast datasets.
This role often becomes popular among newcomers due to its accessibility. With various online courses available, many people can pick up the necessary skills without breaking the bank. A unique feature here is the direct interaction with business units, which cultivates an understanding of organizational needs and strategies early on. However, while this role is beneficial for skill-building, it can sometimes be limited in scope, leaving analysts yearning for more significant responsibilities.
Junior Data Management Positions
On a similar note, Junior Data Management Positions offer a stepping stone that can pave the way to successful careers in data management. This role typically involves supporting data governance and quality assurance initiatives. The distinct feature of a junior role is the exposure to the end-to-end data lifecycle, providing hands-on experience in how data is collected, managed, and utilized.
What makes this career path appealing is the potential for rapid learning. Junior roles often come with mentorship opportunities, allowing individuals to soak up knowledge from seasoned professionals. However, there's a caveat; these positions can sometimes lack autonomy, which may frustrate those eager to make a substantial impact.
Progression to Senior Roles
Senior Data Management Lead
As professionals build experience, they often aspire to become a Senior Data Management Lead. This role amplifies one's responsibilities, extending beyond daily management to include strategic oversight of data initiatives across an organization. A notable attribute of this position is the emphasis on leadership qualities—mentoring junior staff and driving collaborative efforts across departments.
Stepping into this role is advantageous for those who aim to make strategic decisions impacting the company as a whole. The decision-making authority provides a certain prestige and respect within the organization. However, the responsibility can be quite intense, often leading to pressure to deliver results quickly and efficiently.
Chief Data Officer
Finally, the apex of this career path is the Chief Data Officer (CDO). This role encompasses an extensive scope of responsibilities, often driving the overall data strategy and governance. The CDO is now seen as an essential part of the executive team, a position that demands a comprehensive blend of technical knowledge, business acumen, and leadership skills.
Aspiring to be a CDO provides numerous benefits, including a significant level of influence within the organization and the opportunity to shape data policies at a high level. However, it is not without its challenges; the CDO must remain hyper-aware of ever-changing regulations and technological advancements, ensuring the organization remains compliant and competitive.
In the evolving field of data management, understanding one's career path is essential for growth and success.
The exploration of these various roles outlines not only the steps to progression but also emphasizes the evolution of skills and knowledge necessary to thrive in the field. Having a roadmap can offer clarity and motivation, guiding professionals toward their ultimate career aspirations.
Finale
In wrapping up our discussion, it’s evident that a Data Management Lead holds a pivotal role in shaping how data is utilized within an organization. Their work not only influences strategic decision-making but also directly impacts operational efficiency. Understanding this role helps organizations appreciate the multifaceted nature of data management that goes beyond mere data collection and storage.
Summary of Key Points
To reiterate some of the main takeaways:
- Significance of the Role: The Data Management Lead is essential for implementing effective data governance strategies and ensuring compliance with regulations that protect sensitive information.
- Core Responsibilities: This includes overseeing data quality management, establishing data governance frameworks, and fostering communication across departments.
- Skills Required: Technical knowledge, analytical capabilities, and soft skills like leadership and communication are crucial for success in this role.
- Challenges Faced: Navigating data privacy regulations and adapting to rapid technological changes are some hurdles that require constant adaptability and foresight.
- Career Path: The pathway to becoming a Data Management Lead often starts with entry-level data positions and evolves towards senior leadership roles.
Future of Data Management Roles
Looking ahead, the landscape of data management roles is set to evolve significantly. With advancements in technology and an increasing emphasis on data-driven decision-making, the demand for skilled Data Management Leads is likely to surge.
Several trends are shaping the future of this profession:
- Artificial Intelligence: The integration of AI and machine learning in data management processes is expected to streamline data analysis and enhance decision-making capabilities.
- Focus on Data Privacy: As data breaches and privacy concerns grow, professionals in this field will likely need to prioritize the development of robust security measures and compliance frameworks.
- Cross-Functional Collaboration: Data management will increasingly require collaboration across technical and non-technical teams. This shift emphasizes the need for professionals who can communicate insights effectively to a diverse audience.
- Remote Work Adaptations: With more organizations embracing hybrid work models, data management leads must adapt to new tools that facilitate remote collaboration, ensuring data accessibility and integrity.
"The future will be shaped by those who understand data, and not just those who manage it."
By grasping the importance of these points and anticipating future developments, organizations can better prepare for the challenges and opportunities that lay ahead.