The Role of Data and Insights Analysts in Tech
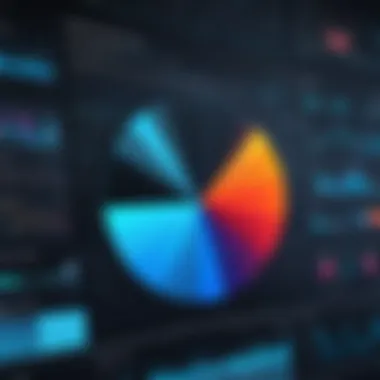
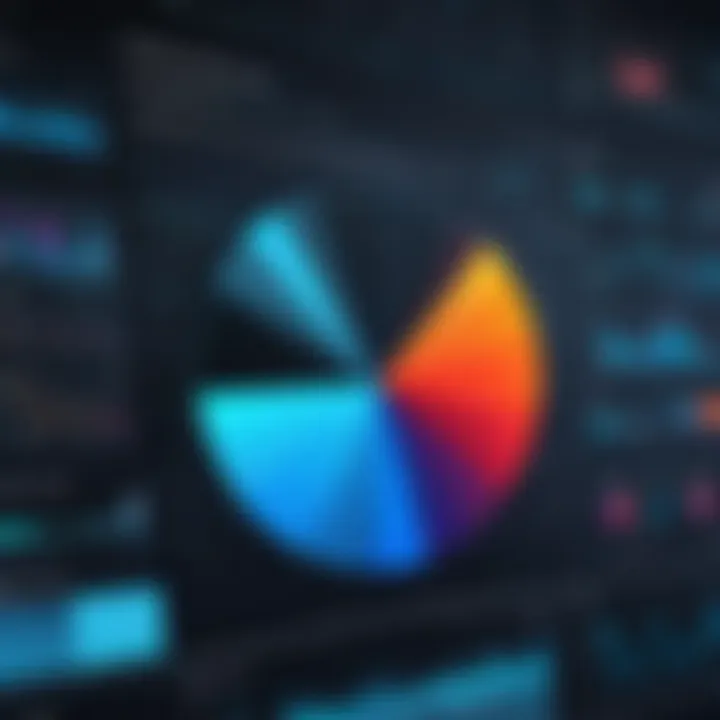
Intro
In today’s rapidly evolving world, the effects of data can hardly be overestimated. With swathes of information being created every second, organizations need individuals who can translate raw numbers into meaningful narratives. This is where data and insights analysts come into play. They are not just number crunchers; they are the navigators on the ocean of information, steering companies towards well-informed decisions.
As businesses pivot towards a more data-centric approach, the demand for analysts has surged. But what exactly do these professionals entail? They are adept at combing through vast amounts of data, extracting insights that can influence strategic directions. From identifying market trends to improving customer experiences, their role is pivotal in ensuring that a company doesn't just keep its head above water, but swims ahead.
Data analysts find themselves embroiled in various methodologies and tools, each offering unique advantages. However, as we delve deeper into their world, it’s important to acknowledge the ethical facets that often come into play in their practices. Once regarded as mere back-office support, data analysts are now front and center in shaping business strategies across industries.
This piece aspires to be a beacon for tech enthusiasts, gadget lovers, and industry professionals, providing not just a peek into the life of a data analyst, but a thorough investigation of their essential contributions in the modern tech landscape.
Tech Trend Analysis
Overview of the current trend
The data analytics industry is currently experiencing a bustling shift towards more automated tools and artificial intelligence capabilities. Gone are the days when reports were conjured up by manual processes that took ages. Nowadays, platforms like Tableau and Looker offer dynamic dashboards that respond in real time, enhancing the speed at which insights are generated.
Implications for consumers
For consumers, these trends translate into enhanced services tailored to individual preferences. Think about the last time a streaming service suggested a show you loved. Such recommendations result from data analysts sifting through user behavior and preferences. As companies leverage these refined methodologies, users benefit from more personalized experiences that meet their needs almost intuitively.
Future predictions and possibilities
Looking ahead, it's plausible that data analytics will become even more intertwined with machine learning. Analysts might find themselves focusing on refining algorithms, ensuring data is processed with the highest quality and efficiency. The potential for predictive analytics is too tempting to ignore. If companies accurately predict trends, they could stay steps ahead of their competitors. However, as the industry evolves, analysts will also need to keep an eye on emerging ethical issues, striving to balance innovation with responsibility.
Industry Updates
Recent developments in the tech industry
Data analytics is not stagnant; it morphs as new needs arise. Recently, there has been a noticeable surge in the popularity of real-time data analysis. Businesses can hardly afford to wait for weekly or monthly reports anymore, especially in sectors like finance or e-commerce where changes occur at lightning speed.
Analysis of market trends
All signs point towards a higher demand for analysts proficient with programming languages such as Python and R. While visualization skills remain critical, the needs of the market are rapidly changing. Organizations increasingly value a fearless approach to data, encouraging analysts to dive deep into their findings, often using advanced statistical techniques.
Impact on businesses and consumers
The influence of these analysts, particularly in the decision-making processes, cannot be understated. As businesses leverage insights for strategic initiatives, consumers benefit through enhanced product offerings and tailored experiences. This symbiotic relationship could foster long-term loyalty and trust.
In essence, data and insights analysts are the modern-day data warriors, fighting the good fight against ambiguity by transforming chaos into clarity.
By understanding these dynamics, both industry professionals and everyday tech enthusiasts can glean greater insight into how data continues to shape our technology landscape.
Foreword to Data and Insights Analysts
In the rapidly evolving world of technology, the role of data and insights analysts has become not just important, but essential. These professionals act as the bridge between raw data and actionable insights, helping organizations navigate the complexity of data-driven decision-making. By transforming numbers and figures into clear narratives, analysts empower businesses to make informed choices that can influence strategy, operations, and ultimately, success.
Understanding the role of data and insights analysts is like peering into the DNA of tech operations. As companies generate unprecedented amounts of data daily, the necessity for skilled individuals who can sift through this information becomes increasingly pronounced. Their expertise ensures that data is not merely stored but utilized effectively. This section sets the stage to explore what these analysts do, their significance in the tech ecosystem, and the core responsibilities they carry.
Definition and Importance in Tech
When we talk about data and insights analysts, we’re referring to professionals who specialize in interpreting complex datasets to extract meaningful insights that drive business strategies. They're not just number-crunchers but storytellers who articulate the implications of data. Their work plays a pivotal role in today’s tech landscape where decisions must be swift and evidence-based.
Analysts leverage statistical techniques and advanced tools to understand trends and patterns in data, helping various stakeholders make educated decisions. For instance, in marketing tech firms, their insights can fine-tune targeting strategies, leading to better customer engagement. Similarly, in product development, data analysis informs feature prioritization based on user feedback and usage statistics.
The importance of this role cannot be overstated. Companies that harness data effectively tend to outperform their peers, and that advantage often lies in the hands of skilled data analysts.
Overview of Responsibilities
The job description for a data and insights analyst is as diverse as it is crucial. Here’s a look at some primary responsibilities that define the role:
- Data Collection and Cleaning: Gathering data from multiple sources and ensuring its accuracy and relevance. This process often includes removing duplicate entries and correcting errors that could skew analysis.
- Data Analysis: Using statistical methods to interpret data and identify trends. Analysts need to be adept at using software tools and programming languages to execute this work effectively.
- Data Visualization: Presenting data in an easily digestible format often involves creating charts, graphs, and dashboards. This helps stakeholders grasp insights quickly and make informed decisions.
- Reporting: Communicating findings through reports or presentations. Analysts must tailor their messaging to various audiences, ensuring that technical findings resonate with non-technical stakeholders too.
- Collaboration: Working with teams across the organization, from marketing to product development, to implement data-driven strategies and ensure that the insights gathered lead to actionable outcomes.
"Data is not just numbers; it tells a story, and the analyst is the storyteller."
These responsibilities highlight the multifaceted nature of the role, which requires not only rigorous analytical skills but also the ability to communicate complex ideas simply. By understanding these core areas, it becomes clear why data and insights analysts are indispensable in harnessing the full potential of data in the tech landscape.
Skills Required for Data and Insights Analysts
In the rapidly changing landscape of technology, data and insights analysts hold a key position. Their role is critical not just in extracting data, but in deriving tangible benefits to drive business decisions and strategy. For individuals aspiring to excel in this field, specific skills are paramount. These skills do not merely enhance one's employability but are essential in navigating the complexities of the modern data environment. Let’s take a closer look at the core competencies required for success in this role.
Analytical Skills
Analytical skills are the bread and butter of any data analyst, acting as the backbone upon which their abilities rest. These skills allow analysts to sift through mountains of data, discerning patterns and trends that might evade the naked eye. For instance, consider an e-commerce company that has seen a dip in sales. A skilled analyst can dive into sales data, customer behavior, and market trends to identify not just the symptoms, but potentially the root causes of the problem.
Analytical capabilities also include statistical methods, which involve interpreting numbers accurately while keeping the big picture in mind. Tools such as R and Python facilitate this process, allowing analysts to perform complex calculations with ease. As such, nurturing a strong foundation in statistical principles is crucial; the real power lies in transforming those raw numbers into actionable insights.
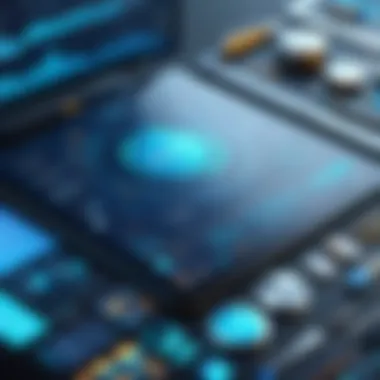
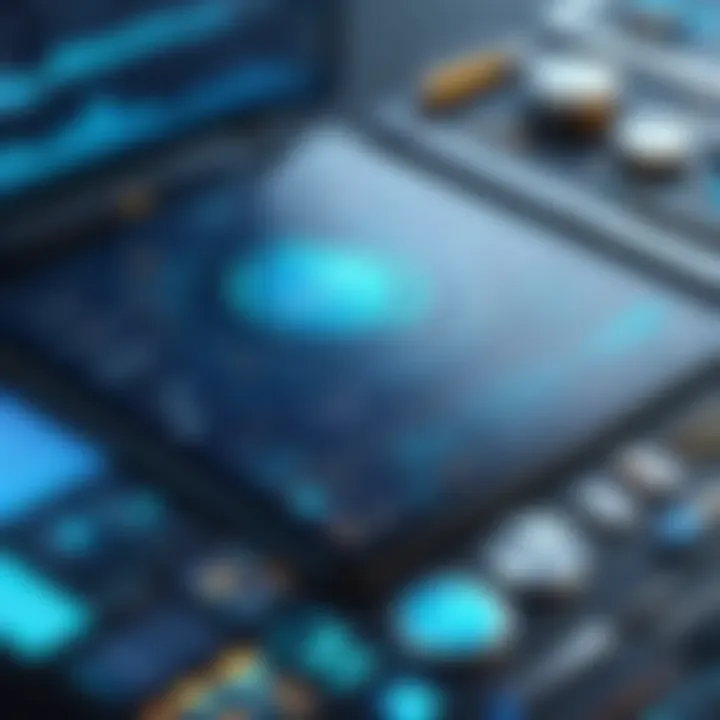
"Data isn't just numbers; it’s a story waiting to be told."
Technical Proficiency
In today's tech-savvy age, technical proficiency is non-negotiable for data analysts. This doesn’t mean you need to be a coding wizard, but familiarity with various technologies is essential. Knowing your way around SQL for database management or Excel for data manipulation is often the starting point. However, as the landscape evolves, being knowledgeable about data visualization tools like Tableau or Power BI can set you apart.
More recently, familiarity with cloud-based platforms like Google Cloud or Azure is becoming increasingly important. With organizations migrating their data storage and analysis to the cloud, those who can leverage these systems effectively are going to have a leg-up.
To sum it up, possessing the right technical skills is akin to carrying a toolkit. Each tool serves a purpose, and mastering the appropriate ones enables analysts to perform detailed investigations seamlessly.
Communication and Presentation
Analysis might be a solitary act typically done behind a computer screen, but the impact of the insights drawn can ripple across teams and organizations. This is where communication and presentation skills come into play. An analyst's ability to transform complex data into clear and coherent narratives matters immensely.
Translating findings into digestible formats for stakeholders—whether they are marketing managers or C-suite executives—is a skill that can’t be understated. Knowing how to create clear visuals, summarizing data in impactful presentations and communicating insights simply can be just as vital as performing the analysis itself.
To communicate your findings effectively, consider the audience and their level of understanding. Using jargon might alienate non-technical stakeholders, while basic explanations might bore data-savvy individuals. Balancing the two is part of the art of being an effective data analyst.
A good starting point is to practice storytelling with data; this can include techniques such as data visualization, contextual framing, and using real-world examples. What goes beyond the numbers is often what shapes decisions and drives action.
Methodologies in Data Analysis
Data analysis is a bedrock in the tech sector, providing the means to convert stacks of raw data into structured insights that fuel decision-making. The methodologies applied by data analysts, whether simple or sophisticated, significantly determine the accuracy and relevance of insights derived. These approaches aren't just academic exercises; they have real implications in shaping strategies and enhancing business performances. Let's unpack the three principal methodologies that are crucial for any data analyst's toolkit: descriptive analytics, predictive analytics, and prescriptive analytics.
Descriptive Analytics
Descriptive analytics serves as the foundation for any data-driven initiative. It revolves around summarizing past data to create a clear picture of what has happened previously. Think of it as a packed suitcase coming back from a trip — it contains everything from souvenirs to receipts, all telling a story of your travels.
Data analysts utilize descriptive statistics to generate insights about patterns or trends from historical data. This could mean analyzing sales data to identify seasonal trends or compiling user engagement metrics to inform future campaigns. The beauty of descriptive analytics is its clarity; it provides a snapshot that can often lead to informed discussions about necessary adjustments or opportunities for growth.
Key Aspects of Descriptive Analytics:
- Data Summarization: Encompasses measures like mean, median, and mode, which give a general understanding of data.
- Trend Analysis: Helps businesses see where they’ve been, potentially steering future initiatives in the right direction.
- Visualization: Tools like Tableau or Power BI can transform raw data into intuitive visuals, allowing for quick comprehension.
"Analysis without planning is like driving a car with your eyes closed."
Predictive Analytics
Moving a step beyond descriptive analytics is predictive analytics, which uses historical data to forecast future events. In simpler terms, it’s the concept of looking in a crystal ball but relying on data patterns instead of mystical powers. Organizations employ statistical algorithms and machine learning techniques to predict outcomes and behaviors.
For instance, retailers might leverage predictive analytics to gauge consumer behavior, estimating what products consumers are most likely to purchase based on previous transactions. Similarly, financial institutions use it for risk assessment, predicting defaults on loans or potential fraud.
Key Considerations for Predictive Analytics:
- Model Selection: Choosing the right algorithms is crucial for accurate predictions. Techniques can range from regression analysis to more complex machine learning models.
- Data Quality: Predictions are only as good as the data; poor quality or missing data can skew results.
- Interpretability: It’s essential that the stakeholders understand the predictions. Clear communication ensures that insights are actionable.
Prescriptive Analytics
As we stride into the realm of prescriptive analytics, it’s where data meets decision-making head-on. This methodology not only anticipates what might happen but also advises on possible actions to take. Essentially, it’s about providing guidelines on what steps to follow to achieve desired outcomes.
Prescriptive analytics uses simulation, optimization, and decision analysis to guide organizations in making better decisions. For instance, a supply chain manager could use prescriptive analytics to optimize inventory levels, recommending how much stock to order and when, based on real-time demand data and operational constraints.
Important Elements of Prescriptive Analytics:
- Scenario Analysis: Evaluates different possible future scenarios based on variations in input data.
- Decision Models: Helps define the best course of action given specific constraints and objectives.
- Real-Time Analysis: Provides insights in real-time, which can be crucial in fast-paced industries.
In a nutshell, methodologies in data analysis serve as the compass guiding organizations through the stormy seas of information. Each approach—descriptive, predictive, and prescriptive—holds its unique significance, contributing substantial value to data-driven decision-making.
Tools and Technologies for Data Analysis
In the evolving landscape where data is the new oil, understanding the tools and technologies that data and insights analysts wield becomes crucial. These tools help bridge the gap between raw data and actionable insights, leading to informed decision-making and strategic planning. Selecting the right array of tools is not just about enhancing efficiency; it’s about uncovering the narratives hidden within data sets, pinpointing trends, and facilitating precise predictions that can shape the future. The significance of these technologies cannot be overstated, as they essentially empower analysts to convert complex data into straightforward stories that businesses can act upon.
Data Visualization Tools
Effective communication of insights often hinges upon how data is presented. Data visualization tools serve as the lens through which data becomes more accessible. They aid analysts in transforming abstract numbers into visual narratives—think bar charts, line graphs, and heat maps—making the information easier to digest and interpret. Tools like Tableau and Power BI are at the forefront of this domain.
- Benefits of Data Visualization Tools:
- Immediate Insight: By visualizing data, trends that may not be perceived in raw numbers become glaringly obvious.
- Engagement Factor: Visuals tend to capture attention better than barren statistics. Presentations become engaging and can stimulate discussion around the data.
- Decision Support: Real-time dashboards can place stakeholders in the driver’s seat, enabling swift decision-making based on the most current data.
Yet, one must be wary of misleading visuals. A graph can be skewed to tell a favorable story, which can lead to erroneous interpretations. Thus, data analysts must wield these tools not only with creativity but with integrity.
Statistical Software
When it comes to deeper analysis, statistical software becomes invaluable. With tools like R and Python, analysts can dive into complex datasets, employing statistical techniques to extract meaningful insights.
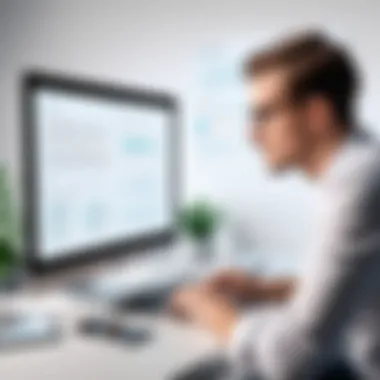
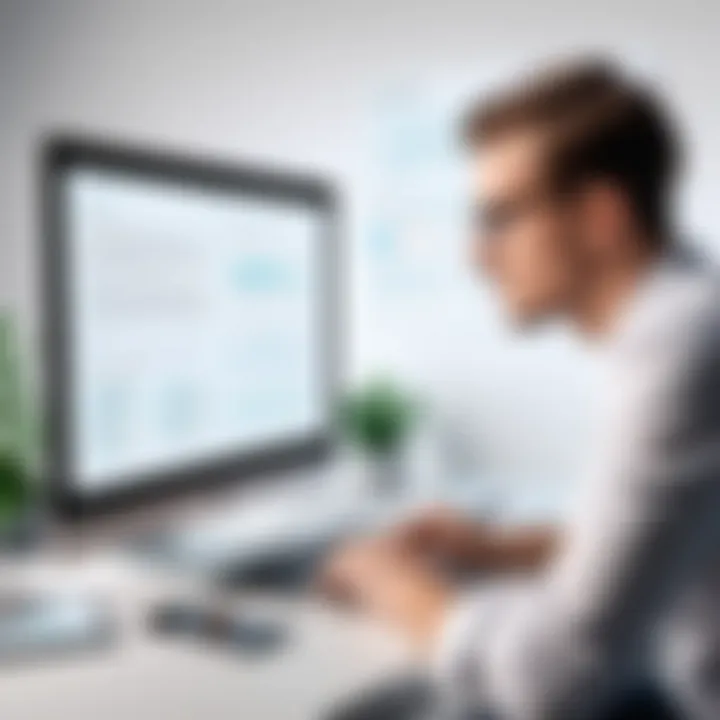
- Characteristics of Statistical Software:
- Ability to handle large data sets efficiently.
- Flexibility in applying various statistical methods for different problems, such as regression analysis, time-series analysis, and hypothesis testing.
- Extensive libraries and community support for ongoing learning and problem-solving.
Statistical software allows analysts to run various scenarios and models, which enable companies to understand potential outcomes based on their decisions. The beauty of such software s in its versatility; it caters to various domains, from finance to healthcare, proving its broad importance in analyzing varied data types.
Database Management Systems
Data without a solid backbone is akin to a house of cards. This is where Database Management Systems (DBMS) come into play. Systems such as MySQL and MongoDB provide a structured way to store, retrieve, and manage data efficiently.
- Importance of DBMS:
- Data Integrity and Security: They ensure that the data remains intact and secure, preventing unauthorized access or data corruption.
- Scalability: As businesses grow, so too does their data. A good DBMS can handle increasing amounts of data without missing a beat.
- User-Friendly Access: DBMS allow analysts to query data without needing to delve deep into coding languages, broadening access to insights.
In summary, selecting appropriate tools and technologies greatly influences the capabilities and effectiveness of data and insights analysts. By leveraging data visualization tools, powerful statistical software, and robust database management systems, analysts can elevate the practice of data analysis and ensure that insights gleaned from data remain relevant, actionable, and ethical.
Data is no longer just a collection of numbers; it's a narrative waiting to be told, and the right tools make that telling possible.
Real-World Applications of Data Insights
In today's fast-paced technology landscape, the application of data insights goes beyond mere information gathering. The ability to turn raw data into actionable insights is crucial across multiple industries. Data analysts serve a vital role by ensuring that organizations make informed decisions that can lead to tangible results, whether it be maximizing profits or enhancing customer satisfaction.
Business Intelligence
Business intelligence (BI) represents a cornerstone of effective decision-making. By analyzing historical and current data, companies can identify patterns and trends that may be otherwise overlooked. This insight enables leaders to tailor their strategies effectively, directly influencing the bottom line. For instance, a retail company might leverage BI to assess purchasing trends, helping it to optimize inventory based on predicted sales. This reduces overhead costs and ensures that popular items are in stock at the right time.
Moreover, BI tools allow organizations to visualize complex data easily. Dashboards filled with graphs and metrics make it simpler for decision-makers to digest vast amounts of information at a glance. This immediacy can mean the difference between seizing a market opportunity or missing it entirely.
Being data-driven bolsters a company’s agility and can even create a competitive edge by ensuring timely adaptations to strategies.
Market Research
When it comes to market research, insights derived from reliable data are more valuable than pure intuition. Analysts gather and interpret data on consumer behavior, market trends, and competitor actions. These insights offer organizations a clearer picture of what resonates with their customer base.
For example, through focused surveys and social media analysis, a tech startup can discover which product features are most appealing to its audience. The findings from this data allow companies not only to tailor their products accordingly but also to create targeted marketing campaigns that resonate with potential buyers.
In addition, analyzing demographic data can guide organizations as they venture into new markets. By understanding the unique characteristics and preferences of potential customers, businesses can customize their approaches, enhancing the likelihood of successful market penetration.
Customer Experience Enhancement
A positive customer experience often translates to loyalty and continued business. Data analytics plays an immense role in refining this experience. Through methods like customer segmentation and behavioral analytics, businesses gain insights that inform personalized marketing and service approaches.
An example can be found in e-commerce, where understanding the journey of a customer's online shopping experience can lead to improvements in website design and functionality. If data indicates a significant drop-off rate at a certain point in the checkout process, companies can investigate to streamline the experience and encourage conversions.
The ability to predict customer needs further empowers organizations to proactively address concerns. Making data-driven decisions keeps customers engaged, satisfied, and likely to return. Thus, personalized interactions often lead to increased customer loyalty.
"Insights from data don't just inform decisions; they often make them stronger, driving strategic gains that are hard to achieve otherwise."
In summary, utilizing data insights in real-world applications enables businesses, regardless of their sector, to stand out in a crowded marketplace. This deep understanding not only fosters informed decision-making but also encourages innovation and growth.
Challenges Faced by Data Analysts
In the fast-paced world of technology, data analysts are like the navigators of a vast, uncharted sea. They steer the ship through waves of information, ensuring that organizations don’t end up adrift or lost. However, this role comes with its fair share of challenges. Addressing these hurdles is not just important; it’s crucial. Insightful analysts can make or break a company’s strategy. Therefore, understanding these challenges helps not only in minimizing risks but also in uncovering new opportunities for growth.
Data Quality Issues
Data is only as good as the source it’s drawn from. If analysts are working with poor-quality data, they're fighting a losing battle from the get-go. Data quality issues stem from several factors, such as inconsistent formats, outdated information, or even incorrect entries. Think of a restaurant that only serves week-old ingredients—no matter how great the chef is, the meal won't be appealing.
Ensuring that the data is clean and reliable is paramount. This requires implementing stringent data management practices. Some actions analysts might consider include:
- Regular Audits: Regularly auditing data sources for accuracy.
- Automation Tools: Employing tools that help automate the cleaning process.
- Training Staff: Ensuring that everyone involved in data entry understands the importance of accuracy.
When these measures are in place, analysts can trust their findings and base their recommendations on solid ground. Without this trust, decisions can be misguided, leading to potential financial losses or missed opportunities.
Volume and Variety of Data
The sheer volume of data generated today is mind-boggling. We produce about 2.5 quintillion bytes of data every single day. With such a vast amount of information, analysts often find themselves overwhelmed. Tackling the volume is one challenge, but the variety adds another layer of complexity. Data comes from numerous sources—social media, customer interactions, sales transactions, you name it. Each source has its flavor and format, making it tricky for analysts to create a unified picture.
To navigate this sea of data, analysts must prioritize effectively. Strategies include:
- Data Categorization: Classifying data into relevant categories to filter out the noise.
- Utilizing Big Data Technologies: Leveraging technologies like Hadoop and Spark, which can handle large datasets effortlessly.
- Focus on Key Metrics: Identifying and honing in on the key metrics that matter most to stakeholders.
By focusing on the essentials and utilizing appropriate tools, analysts can streamline their work and make sense of what would otherwise be chaotic data landscapes.
Keeping Up with Technological Changes
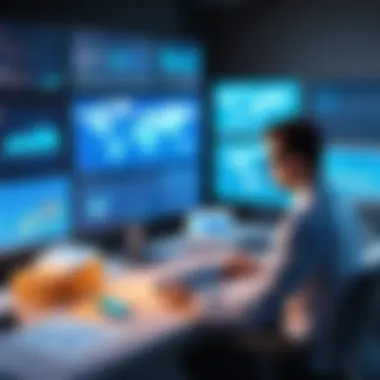
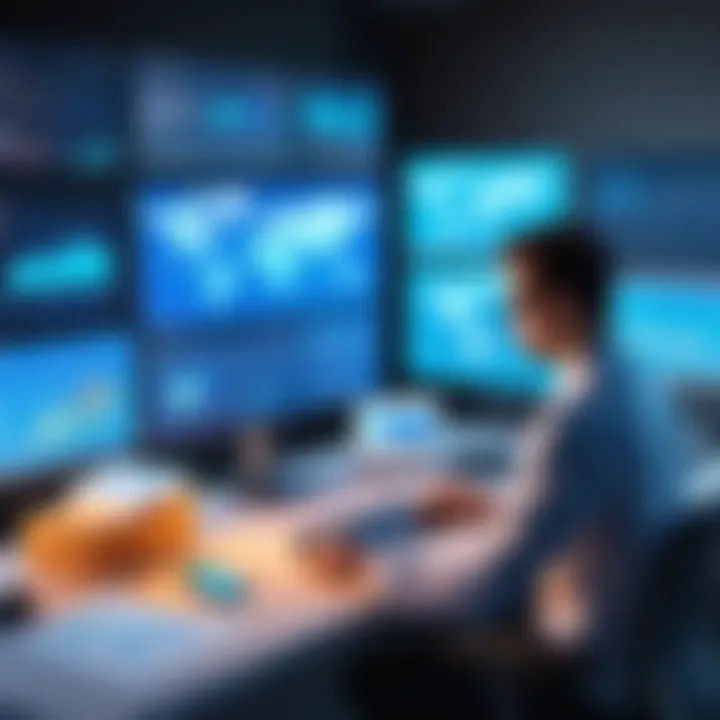
In the world of tech, change is the only constant. New tools and methodologies emerge all the time, and analysts need to keep their skills relevant. The pressure to continuously update one’s knowledge can be daunting. Technologies that seemed revolutionary a year ago may quickly become obsolete, leaving analysts scrambling to adopt new practices.
This unyielding pace of change makes ongoing education critical. Steps analysts can take include:
- Continuous Learning: Engage in online courses to stay updated on the latest data tools and techniques.
- Networking with Peers: Joining forums and discussion groups (like those found on Reddit) allows for sharing insights and experiences.
- Experimentation: It's beneficial to test new tools and approaches in a sandbox environment before applying them to real-world data.
By embracing lifelong learning and being adaptable, data analysts can keep pace with the intricacies of their evolving field, ensuring they remain valuable assets in any organization.
"In the midst of chaos, there is also opportunity." - Sun Tzu
Understanding these challenges paves the way for more efficient and effective data analysis practices, ultimately allowing businesses to thrive in a data-driven world.
Future Trends in Data Analysis
In an era where information is golden, the future of data analysis holds immense significance. The landscape is consistently evolving, and understanding these trends is crucial for industry success. Organizations that grasp these shifts not only gain a competitive advantage but also foster innovation in their processes.
Artificial Intelligence and Machine Learning Integration
The integration of artificial intelligence (AI) and machine learning (ML) into data analysis represents a seismic shift in how decisions are made and insights are derived. Analysts are now able to train algorithms to recognize patterns and predict outcomes with remarkable accuracy. This isn't just about crunching numbers anymore; it’s about empowering machines to learn from the data itself.
- Benefits:
- Considerations:
- Efficiency Gains: AI can sift through massive datasets at lightning speed, allowing analysts to focus on strategic tasks.
- Enhanced Accuracy: Algorithms that learn continuously can adapt to new data, significantly reducing errors compared to traditional methods.
- Skill Gaps: There's a pressing need for analysts to upskill to work effectively with these advanced technologies.
- Data Dependency: Successful AI models rely heavily on clean, high-quality data. This makes data governance even more essential.
Augmented Analytics
Augmented analytics is another trend reshaping the way data is analyzed and visualized. Using AI tools, analysts can uncover hidden patterns and insights without the need for deep statistical knowledge. This democratizes data, making insights accessible to all levels of a business.
- Key Features:
- Benefits:
- Natural Language Processing (NLP): Analysts can interact with data via conversational interfaces, enabling users to ask questions in plain language and receive insightful responses.
- Automated Insights: The technology can suggest insights, highlighting trends that might have gone unnoticed.
- Time-saving: Automating reports and visualizations frees analysts to focus on interpretation rather than manual compilation.
- Broader Participation: With user-friendly interfaces, more people in an organization can engage with data, fostering a culture of data-driven decision-making.
Ethical Considerations in Data Practices
As data analysis grows in sophistication, so do the ethical considerations surrounding it. Data privacy, fairness, and algorithmic bias are issues that demand attention. Data analysts not only need to deliver insights but must approach their work with a sense of responsibility.
- Data Privacy: Protecting user information is paramount. Compliance with regulations like GDPR and CCPA is non-negotiable.
- Bias and Fairness: Analysts must scrutinize the data and algorithms they use to ensure equitable outcomes. Without careful oversight, there's a risk of perpetuating existing inequalities.
In the quest for meaningful insights, ethical data practices are often overlooked, yet they can make or break trust with consumers.
The future of data analysis promises to be dynamic, with trends such as AI integration, augmented analytics, and ethical practices taking center stage. Keeping abreast of these changes will not only help analysts and organizations make informed decisions but also ensure they fully leverage the potential of data in a responsible manner.
The Vital Role of Ethics in Data Analysis
The ethical framework surrounding data analysis holds significant weight in today's highly digitized and data-driven world. With the explosion of technology, the mountains of data being generated every second creates a minefield for those tasked with interpreting it. Data and insights analysts must navigate ethical concerns, which, if ignored, could lead to detrimental outcomes for individuals and organizations alike. The importance of ethics extends beyond mere legality; it involves principles that guide analysts in making responsible decisions that protect stakeholders while achieving insight-driven outcomes.
Data Privacy
One cannot discuss ethics in data analysis without emphasizing data privacy. Nowadays, consumers expect their personal information to be safeguarded. The rise of regulations like GDPR (General Data Protection Regulation) has marked a turning point in how organizations handle user data. Analysts often have access to sensitive information, which frequenly compels them to behave with utmost discretion. By implementing robust data privacy measures, data analysts not only adhere to laws but also build trust with clientele.
If individuals feel their data is treated with respect, they are more likely to engage authentically with organizations. This trust can translate into better user experiences and loyalty, significantly benefiting the business in the long run.
"Ethics in data analysis isn’t merely a checkbox; it’s critical for fostering trust and long-term relationships with clients."
Moreover, analysts can leverage anonymization techniques to protect individual identities while still drawing substantial insights. For instance, rather than analyzing specific transaction data from users, organizations might aggregate this information to glean trends, thus maintaining privacy. Companies embracing ethical practices surrounding data privacy often find themselves at an advantage in competitive markets.
Bias and Fairness in Algorithms
As algorithms become more prevalent in decision-making, bias and fairness remain integral to ethical considerations. Bias can seep into data analysis through various channels, including flawed datasets or subjective human choices made during the selection of variables to analyze. When biases in algorithms go unchecked, they can lead to unfair treatment of specific groups—especially marginalized communities—resulting in widespread social implications.
Analysts must not only be aware of these biases but actively work toward minimizing their impact. This can entail validating data with diverse sources, ensuring representation across data inputs, or applying fairness metrics to identify and mitigate algorithmic biases.
Tech giants have faced backlash for biased algorithms, pointing to the need for accountability. As more industries incorporate data analysis in their decision-making, analysts must advocate for fairness to nurture a more inclusive future.
Detailed attention to these ethical dimensions not only benefits organizations but also ensures that the decisions made based on data are just and equitable. When ethical practices are in the forefront of data and insights analysis, it paves the way for a tech landscape where data propels innovation without compromising societal values.
Ending
In wrapping up our exploration of data and insights analysts, several key themes emerge that underscore their essential role in the modern tech landscape. Above all, these professionals are not just number crunchers; they play a pivotal role in shaping strategies that drive business growth and improve operational efficiencies. The analytical insights they provide form the backbone of decision-making processes across industries, making their work fundamentally important.
Summarizing Key Insights
The journey through this article highlights numerous invaluable aspects of being a data and insights analyst. Firstly, these analysts leverage a diverse range of skills, from meticulous analytical abilities to technical know-how, that enables them to navigate and interpret vast datasets. They employ various methodologies, including descriptive, predictive, and prescriptive analytics, each serving a unique purpose in understanding data and generating actionable insights.
Moreover, several tools and technologies are at their disposal, such as data visualization platforms like Tableau and database management systems like SQL Server. These tools not only enhance their efficiency but also ensure that insights are communicated in a meaningful way to stakeholders.
As we have discussed, real-world applications of data analysis are practically limitless. The impact they have on business intelligence, market research, and customer experience illustrates their significant influence on organizational success.
The Future Landscape for Data Analysts
Looking ahead, the future landscape for data analysts appears to be both exciting and challenging. With the integration of artificial intelligence and machine learning into analysis processes, analysts can expect a shift in how data is approached. These technologies promise not only to streamline processes but also to enhance predictive capabilities, allowing for more informative decision-making.
In addition, there is a growing emphasis on ethical considerations surrounding data practices. As the volume and variety of data increase, so do concerns regarding data privacy and algorithmic bias. Analysts must be vigilant, ensuring that best practices are in place to uphold ethical standards as they manipulate and interpret data. This not only protects user privacy but will also bolster the credibility of their insights.
In summary, data and insights analysts will continue to be at the forefront of technological innovation, playing an integral role in translating data into strategic, actionable insights that drive progress and profitability. As the landscape evolves, so too will the skills and focus of these important professionals, ensuring their relevance in helping organizations navigate the complexities of the digital age.