Exploring Big Data Analysis Companies in Today's Market
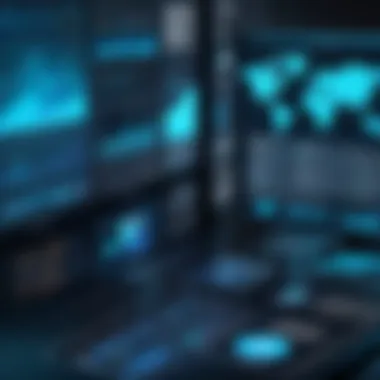
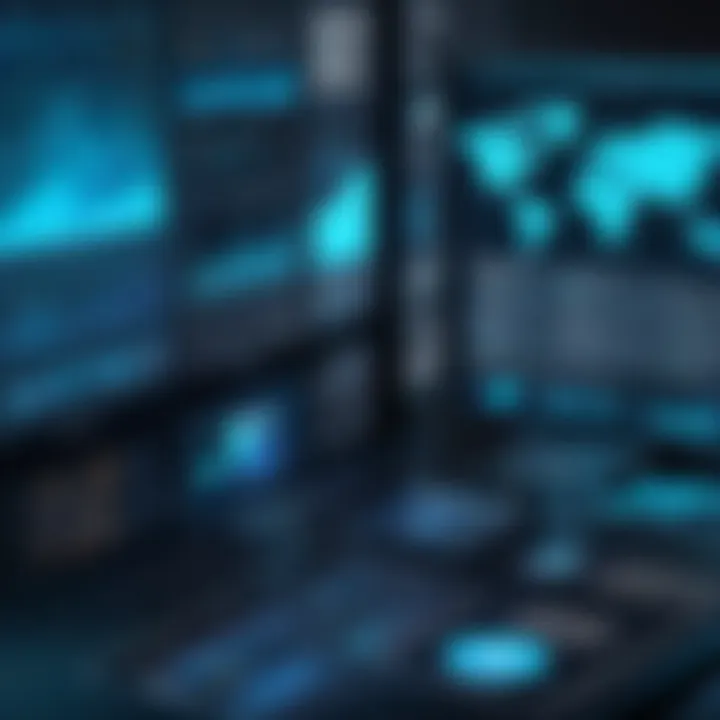
Intro
In the age of big data, companies are emerging as key players in the data-driven landscape. With mountains of information generated daily, businesses need to harness these insights to stay ahead. This article dives into the significance of big data analysis and the firms that specialize in this arena. Why do these companies matter? How are they changing the way industries operate? We will explore these questions and more, laying a foundation for understanding this critical aspect of modern business.
Tech Trend Analysis
Overview of the Current Trend
The landscape of big data analysis has evolved remarkably over the last few years. More industries, from healthcare to finance, are adopting data-centric strategies. Technologies such as artificial intelligence and machine learning are becoming commonplace. Current trends indicate a shift towards real-time data processing. Organizations are keen on extracting actionable insights at a faster pace, leading to smarter decision-making.
Implications for Consumers
Consumers are increasingly impacted by these technological advancements. With companies analyzing data, personalized experiences are becoming the norm. Think about the targeted advertisements you see or the recommendations on streaming platforms – these are results of sophisticated data analysis. Moreover, transparency in data usage is trending; consumers now have a better understanding of how their information is used, leading to trust-building between companies and customers.
Future Predictions and Possibilities
Looking ahead, we can anticipate even more integration of big data analytics in everyday life. Imagine a world where smart devices not only track your health but also provide predictive analytics to preemptively address potential health issues. As data privacy concerns grow, companies will need to navigate regulations while maximizing the benefits of insights derived from data. This dual focus on innovation and ethics will shape the future of big data analysis.
Challenges Faced in Big Data Analysis
Data Quality
One of the biggest hurdles within the sector is the quality of data itself. Poor data can lead to flawed analysis, and hence, incorrect business decisions. Companies must adapt rigorous data cleaning methodologies to ensure reliability.
Skilled Personnel
As technology evolves, so does the need for skilled analysts. There is a shortage of trained professionals capable of interpreting massive data sets and deriving actionable strategies. Companies are pouring resources into training and development to keep pace with evolving skills requirements.
Managing Volume and Speed
Another challenge is managing the sheer volume of data that companies generate and collect. The velocity with which this data arrives is daunting. Organizations must implement advanced infrastructure and tools to process and analyze data swiftly without bottlenecks.
The End
In summary, big data analysis companies are instrumental in navigating our data-driven world. By understanding the current trends, consumer implications, and challenges faced, businesses can better align their strategies to leverage data effectively. This ever-evolving field will continue to shape industries, and those who adapt will emerge victorious in the digital landscape.
Understanding Big Data
In an age where information flows faster than the snap of a finger, grasping the concept of big data is crucial. Big data is not just a technical term tossed around in board meetings; it fundamentally reshapes how businesses operate, make decisions, and connect with customers. It presents an opportunity for organizations to harness colossal volumes of data to drive growth and enhance customer experience.
Understanding big data entails comprehending its various dimensions and the characteristics that differentiate it from traditional data. It’s about recognizing how data can be both an asset and a challenge, and navigating these waters effectively can spell the difference between success and stagnation.
Definition of Big Data
Big data refers to datasets that are so large and complex that they're difficult to process using traditional data processing applications. It encompasses data generated from numerous sources, including social media, transaction records, sensors, and more. This data isn't just about its size; it's also about its velocity, variety, veracity, and value.
Characteristics of Big Data
- Volume
Volume describes the sheer size of data being generated every second across various platforms. For instance, consider the trillions of bytes produced by social media interactions, online transactions, and sensor data from IoT devices. The ability to sift through high volumes of data is not merely an operational capability — it is often where insights and trends hide. - Velocity
Velocity pertains to the speed at which data is created, stored, and analyzed. In the current landscape, data isn't stagnant. It's continually flowing, with real-time analytics becoming a necessity to remain competitive. For example, stock trading platforms require instantaneous data feedback to make rapid decisions and maintain market relevancy. - Variety
Data comes in various forms, whether structured, unstructured, or semi-structured. Social media content, emails, images, and recorded phone conversations all fall under this umbrella. This multitude of formats can enhance analytics but also complicates the process. The mixing and matching of diverse data types can lead to richer insights, provided the right tools are employed. - Veracity
Veracity refers to the reliability and accuracy of data. Without quality data, even the most sophisticated algorithms can lead to untrustworthy results. Veracity emphasizes the importance of cleaning and validating data before it’s put to use. Unchecked data can mislead organizations, causing misguided strategies based on faulty conclusions. - Value
Ultimately, the goal of collecting massive amounts of data comes down to value. It’s not enough to have data; companies must discover how to derive meaningful insights from it. The value of big data lies in identifying trends, making predictions, and informing strategic decisions that can pave the way for innovations and competitive edges in the marketplace.
Importance of Big Data Analysis
The importance of big data analysis can’t be overstated. It influences nearly every industry, from healthcare where patient data drives treatment innovations to retail where shopping behaviors help tailor marketing strategies.
Big data analysis enables organizations to forecast market trends, personalize customer experiences, and increase operational efficiency. Analyzing data assists in identifying customer preferences and behavior patterns, translating to better service and a strong competitive advantage in an increasingly crowded market.
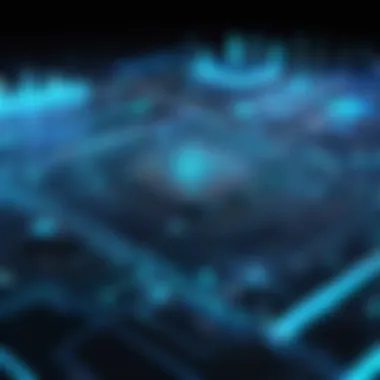
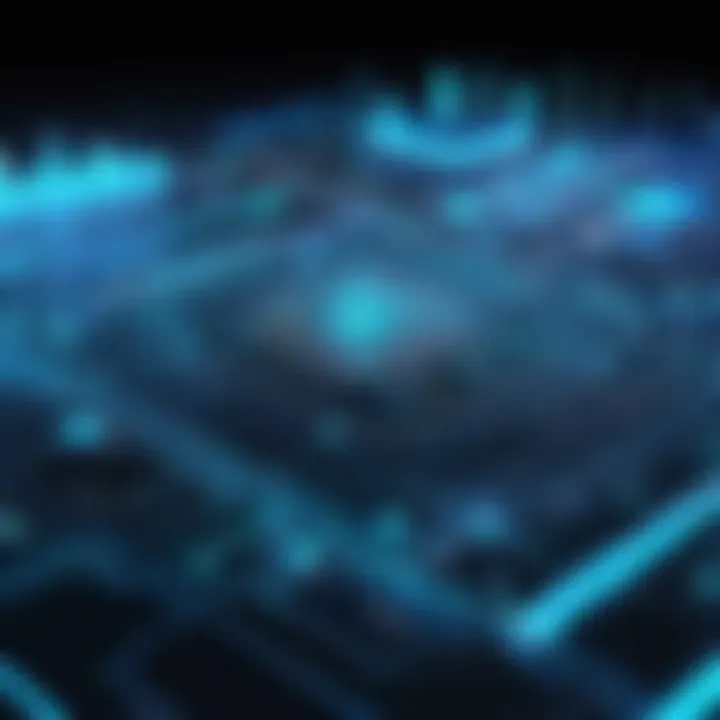
"Data is a valuable asset; harnessing it transforms not just businesses, but entire industries."
As the world becomes ever more interconnected, understanding big data is no longer optional for companies looking to thrive. It’s a fundamental building block for making informed decisions and staying relevant in today’s fast-paced digital landscape.
Big Data Analysis Companies Overview
In an ever-evolving digital sphere, big data analysis companies stand as beacons of innovation. They wield the power to sift through colossal amounts of data, extracting nuggets of wisdom that can drive business decisions and shape strategic directions. As organizations increasingly rely on data to steer their choices, the role of these companies becomes paramount not only for optimizing performance but also for gaining a competitive edge in their respective fields.
What Constitutes a Big Data Company?
A big data company typically focuses on the aggregation, analysis, and visualization of vast data sets. However, just examining company classification is more like looking at an iceberg; much lies beneath the surface. These firms are distinguished by their ability to handle the three V's of big data: volume, velocity, and variety. Companies like Cloudera and Palantir Technologies highlight innovative data management strategies essential for harnessing big data insights. Moreover, such companies often deploy advanced analytics and machine learning to unpack values hidden in data streams, making them invaluable partners to organizations aiming to leverage data effectively.
Key Players in the Industry
Major Corporations
When it comes to heavyweights in the realm of big data analysis, companies like IBM, Microsoft, and Oracle come to mind. Their influence is extensive due to their well-established infrastructures and robust service offerings. IBM, for example, has evolved its Watson platform to offer a suite of tools that facilitate data-driven decision-making in various sectors. A hallmark of such corporations is their ability to integrate various analytic tools with existing business operations, a factor that significantly boosts productivity.
The unique feature of major corporations lies in their access to extensive resources and funding, enabling them to invest in cutting-edge technologies and talent acquisition. This strength can be a double-edged sword; they sometimes lose agility to respond to rapid market changes compared to their smaller counterparts.
Startups
Starting from scratch can often lead to innovation that the larger corporations may overlook. Startups in the big data sector, such as Databricks or Snowflake, have introduced versatile data management platforms that allow companies to easily access and manipulate massive data chunks. One key characteristic of these startups is their focus on niche solutions or specific industries, enabling them to cater to unique customer needs more effectively.
Startups boast a flexibility that enables rapid iterations and quick pivots, which is crucial in the fast-paced world of technology. However, their growing pains often include issues such as funding instability and the challenge of scaling quickly. It's crucial for them to find niche markets before the big players arrive, making their ongoing survival a test of their innovation and adaptability.
"In the world of big data, adaptability can make or break companies. It's not just about having data it's about leveraging it intelligently and quickly."
In summary, both major corporations and startups play pivotal roles in the big data analysis landscape. While they each have different advantages and challenges, their collective efforts contribute to a burgeoning industry that continues to shape how organizations understand and utilize their data.
Key Technologies in Big Data Analysis
Understanding the crucial technologies in big data analysis is like having a map in the ever-evolving digital landscape. These technologies form the backbone of how companies analyze massive volumes of data, enabling them to derive actionable insights. Each technology contributes uniquely, offering benefits and addressing specific challenges that come with the territory of handling big data.
Data Mining Techniques
Data mining is the art of extracting valuable information from large datasets. It’s akin to digging for gold in a vast expanse of sand. Companies employ various techniques such as clustering, classification, and regression to uncover patterns and trends that are not readily visible. For instance, clustering can group similar customer behaviors, assisting in targeted marketing strategies. The ability to find hidden correlations not only enhances customer experience but also improves efficiency within organizations.
Yet, while data mining brings its merits, it’s important to tread carefully. Poor application or over-dependence on these techniques can lead to misleading conclusions. This emphasizes the importance of human judgment alongside automated processes.
Machine Learning Algorithms
Machine learning serves as the engine room of big data analysis, optimizing processes through the learning of patterns from vast datasets without direct programming. Whether it's predicting customer churn or enhancing product recommendations, algorithms such as decision trees, neural networks, and support vector machines play a vital role. For instance, Netflix employs sophisticated machine learning algorithms to recommend shows based on user preferences, creating a personalized viewing experience.
Moreover, as the analytics landscape grows, the importance of continuous learning becomes paramount. Algorithms need to adapt to new data streams and changing user behaviors. This ability to learn and improve over time enhances the robustness and accuracy of predictions, providing businesses a competitive edge.
Cloud Computing in Big Data
Cloud computing has revolutionized the way big data is processed and stored. No longer are companies constrained by physical infrastructure. With services from platforms like Amazon Web Services and Microsoft Azure, organizations can scale their data operations seamlessly. Cloud solutions enable companies to access vast computational power and storage capabilities without the heavy upfront costs.
However, it’s crucial to speak about potential pitfalls, such as data security concerns and compliance requirements, which need to be addressed diligently. Businesses must ensure that their data is well-protected against breaches while optimizing their use of cloud resources.
Data Visualization Tools
Purpose
Data visualization tools serve as the storytelling medium for data. Just as a picture is worth a thousand words, a well-designed graph can transform complex datasets into digestible visual insights. The purpose of these tools is to present data in a format that is easy to understand and actionable. By employing visuals, companies can identify trends, patterns, and outliers swiftly, which is vital for timely decision-making.
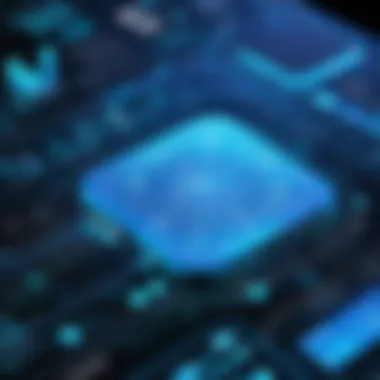
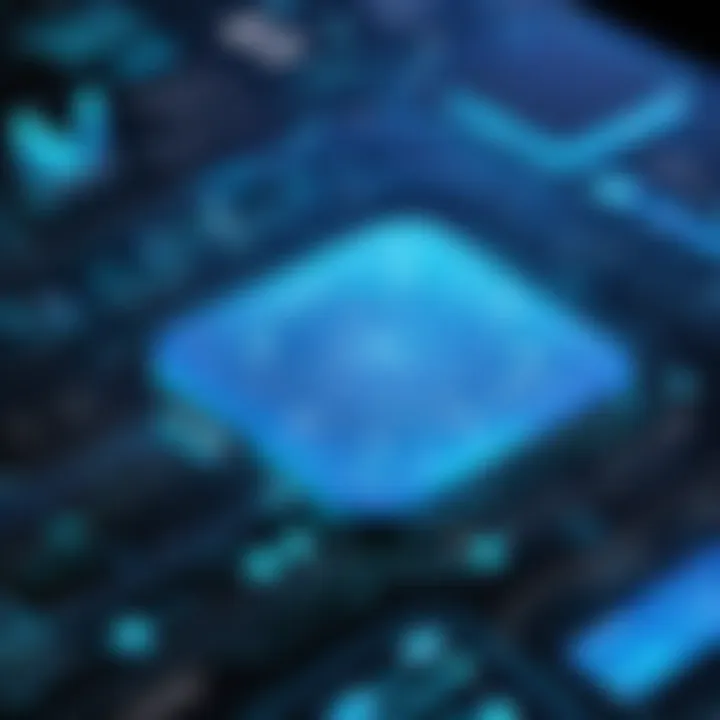
The key characteristic of effective data visualization is clarity. A tool that presents data in a straightforward yet detailed manner is popular among businesses seeking to harness the full potential of their data. Such clarity leads to informed decisions and strategy development, ensuring that stakeholders can grasp essential insights quickly.
Popular Tools
When it comes to popular data visualization tools, Tableau, Power BI, and Looker come to mind. Each of these platforms boasts unique features that cater to different analysis needs. Tableau, for example, is renowned for its ability to create stunning visuals that can narrate data stories compellingly.
The advantages of using these tools include their user-friendly interfaces and powerful analytical capabilities. However, inherent limitations may include steep learning curves for less experienced users or expense associated with licensing. For businesses, the choice of tool often depends on balancing budget constraints with the need for sophisticated data analysis capabilities.
In summary, the technologies driving big data analysis are multifaceted and integral to success in today's data-centric market. Each technology offers unique attributes, yet all share the common goal of translating raw data into insightful, actionable information.
Application of Big Data Analysis
In today's fast-paced digital world, the application of big data analysis extends far beyond mere trend observation or data collection. It’s a critical pillar that supports informed decision-making across a multitude of industries. The beauty of working with big data lies in the insights it can generate, driving businesses to achieve efficiencies and create value. The process goes a step further when companies leverage these insights to predict future outcomes or adjust their strategies. By examining specific applications across different sectors, one can glean how vital big data analysis has become for survival in this competitive landscape.
Industry-Specific Applications
Healthcare
In the realm of healthcare, big data plays a transformative role. Hospitals and clinics utilize vast amounts of data from patient records, clinical trials, and medical imaging to enhance patient care. Consider, for instance, predictive analytics, which aids in forecasting disease outbreaks or predicting patient readmissions. This data-centric approach not only leads to improved outcomes but also contributes to cost savings. The health sector's key characteristic is its reliance on real-time data, essential for quick and impactful decision-making. However, it also grapples with data privacy issues, as sensitive information must be handled with care, balancing innovation with ethical considerations. The unique feature of leveraging big data in healthcare lies in its ability to personalize treatment plans, enhancing the overall patient experience.
Finance
The finance industry stands as a prime example of how big data can reshape traditional practices. With loads of transactional data, financial institutions analyze trends to mitigate risks and enhance customer satisfaction. They harness algorithms to detect fraudulent activities in real-time. The key characteristic of finance data analysis is risk assessment and personalized customer service, which can lead to targeted marketing and investment opportunities. A major benefit is the ability to respond swiftly to market changes, while a notable disadvantage is the ever-present challenge of cybersecurity, where a single breach can undermine trust. The use of big data in finance is indeed a double-edged sword, making it a popular and crucial application.
Retail
In retail, big data fuels business strategies and customer engagement. By analyzing buying patterns, customer preferences, and inventory levels, retailers can offer personalized marketing tactics. Imagine a scenario where a shopper receives tailored discounts on products they frequently purchase. This practice enhances customer loyalty while driving sales. One of the retail sector's standout characteristics is its adaptability; the insights derived from big data support inventory management and supply chain optimization. Nonetheless, the unique feature here is the speed at which retail businesses need to adapt to consumer behavior changes, which can be both an advantage and a pitfall due to external trends.
Manufacturing
Manufacturing has heavily leaned on big data analytics to streamline operations and minimize costs. Through monitoring machinery performance and supply chain metrics, manufacturers can predict maintenance needs, reduce downtime, and enhance productivity. The industry’s key characteristic is the integration of IoT devices to collect data in real time. A beneficial aspect is the ability to improve operational efficiencies and reduce waste, yet the potential downside can be high initial costs for implementing such advanced technologies. The unique feature in the manufacturing sector is the implementation of predictive maintenance, ensuring machinery operates at peak performance, thus safeguarding profits.
Case Studies of Successful Implementations
Through concrete examples, one can observe how various companies have harnessed the power of big data analysis to achieve notable successes. Case studies showcase tailored approaches across industries and reveal both triumphs and challenges. For instance, a healthcare provider that utilized big data analytics experienced a drastic reduction in unnecessary hospital stays driven by informed care plans. Similarly, a finance firm employing advanced data analytics observed a significant decrease in fraudulent transactions over a short period. These implementations not only fortify the arguments for adopting big data analytics but also provide learning points for other companies aiming to enter this evolving landscape.
“Data is the new oil; it’s valuable, but if unrefined, it cannot really be used.”
—Clive Humby
As we delve into the application of big data analysis, it becomes clear that the stakes are high, yet so are the rewards. With a keen focus on industry-specific applications, companies can unlock the benefits of big data while navigating challenges effectively.
Challenges Faced by Big Data Companies
As the world rapidly goes digital, big data analysis companies find themselves navigating a sea of potential while also wrestling with formidable challenges. Understanding these hurdles is crucial, not just for industry insiders, but also for stakeholders who wish to better comprehend the ecosystem's dynamics. The importance of grappling with these challenges lies in the ability to leverage data responsibly and effectively, ensuring that insights derived from analysis genuinely serve their purpose.
Data Privacy Concerns
Data privacy is a monumental issue in today’s landscape. With big data, companies collect vast amounts of information about users, customers, and their behaviors. Failing to secure this data can lead to breaches that not only demolish trust but also invite legal repercussions. Ever noticed how emails flood in about updated privacy policies? This is hardly a mere formality. Companies like Palantir and Splunk grapple with these regulations on an everyday basis, making sure they’re covering all bases, following GDPR and CCPA among others.
Turning heads in this arena requires a delicate balance—gathering necessary data while safeguarding individual privacy. According to a recent survey by IBM, 77% of customers claim they would stop doing business with a company if it misused their personal data. Thus, the stakes elevate, pushing firms to invest in sophisticated data management and protection systems.
Data Quality Issues
When it comes to data, garbage in, garbage out rings true. One of the notable issues is ensuring data accuracy and reliability. With the sheer volume of data in play, organizations like Cloudera or Tableau frequently face obstacles pertaining to data integrity. They must wrestle with inconsistencies and incoherent formats as incoming data is often collected from myriad sources—some reliable, others questionable.
The repercussions of poor-quality data are far-reaching; from misguided strategic decisions to tarnished brand reputations. To combat this, large organizations are employing rigorous data cleaning processes and implementing machine learning methods to improve data quality. Regular audits, checks, and balances in processes become vital in upholding high standards for data management.
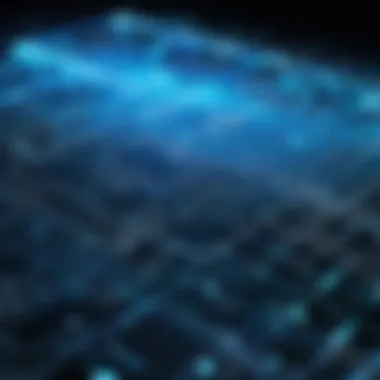
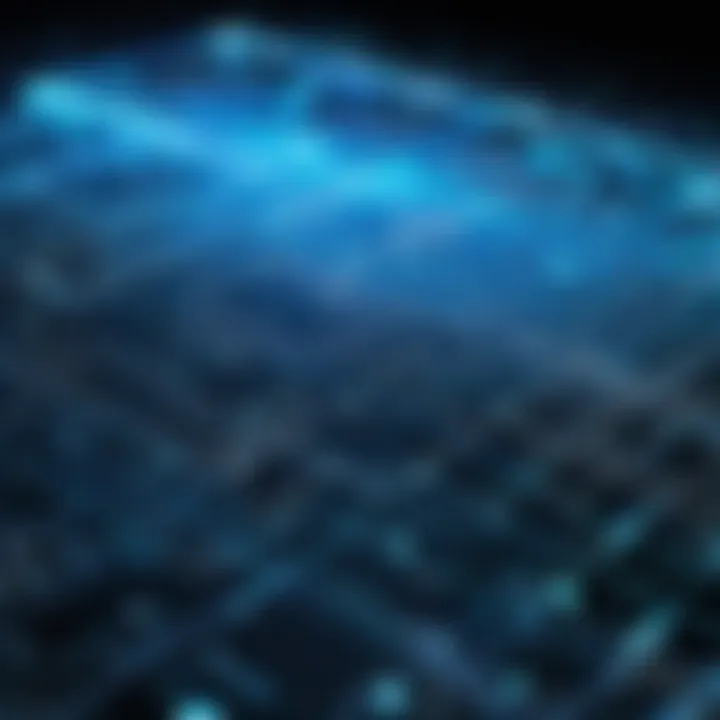
Technical Limitations
Despite the sophistication of many big data tools, technical limitations still loom large. On the one hand, robust algorithms and storage systems abound, yet integrating these components often turns into a puzzle with missing pieces. Companies like Amazon Web Services face the uphill battle of scalability; as data influx grows, the ability to process and analyze this data in real-time needs to keep pace. Problems can arise simply due to system overload or lack of proper infrastructure.
In many cases, businesses are up against the limitations of their own hardware or the prohibitively high costs of advanced technology. Emerging technologies can provide cutting-edge solutions but require skillful handling and specialized knowledge. Moreover, some companies may lack the technical know-how to fully utilize the tools available to them, resulting in a gap that can hinder performance.
"It's not the size of the data; it's how you manage it that counts."
Addressing these challenges is no small feat. Yet, it is vital for big data companies to recognize these limitations and adapt. Only then can they harness the true power of insights, transforming challenges into stepping stones for innovation and growth.
The Future of Big Data Analysis Companies
As we stand at the crossroads of the data revolution, the future of big data analysis companies looms large on the horizon. It's about more than just crunching numbers anymore; it's about transforming those numbers into actionable insights that drive decision-making across industries. Companies in this sector hold the keys to unlocking potential that we have yet to fully explore.
Emerging Trends
AI Integration
Artificial Intelligence, or AI, is increasingly becoming the backbone of big data analysis. Its contribution lies not just in automation but in enhancing analysis quality. The key characteristic of AI integration is its ability to learn from data patterns, enabling more accurate predictions and insights than traditional methods.
What makes AI a beneficial choice for companies is its capacity to handle complex data sets with agility. Unlike older technology that needs a human touch every step of the way, AI can sift through mountains of data on its own, identifying trends that might go unnoticed. A unique feature of AI in this context is its adaptability. It evolves continually, learning from feedback loops and improving over time.
However, there are disadvantages too. For instance, there's the challenge of data bias, which can skew AI outputs. A mismanaged algorithm can lead to misleading results. Still, the overall benefits of speed, scale, and improved accuracy in insights often outweigh these downsides.
Real-time Analytics
Another wave making significant strides is real-time analytics. This area refers to the ability to analyze data as it is created or received, providing up-to-the-minute insights that are invaluable for businesses. The key characteristic of real-time analytics is its immediacy—companies can react on the fly to emerging trends or issues, rather than relying on outdated information.
Real-time analytics stands tall as a beneficial tool because it empowers decision-makers with timely information. When a retailer sees a sudden spike in sales for a particular item, for instance, they can adjust inventory instantly to meet demand. A unique feature of this approach is the ability to monitor customer interactions continuously, allowing personalization in a way that static data can't.
But with its upsides come disadvantages, such as the need for robust infrastructure to handle the data flow. Companies also face challenges in ensuring data quality and reliability in real time. Still, the advantages of agility and improved customer experience often make it a worthy investment for sectors like retail and finance.
Predictions for Market Growth
Anticipating the future, experts predict that the market for big data analysis is set to skyrocket. The increasing digitization across all sectors means that businesses will continue to invest heavily in data tools and technologies. As adoption rates rise, key areas of growth include enhanced data privacy measures, advancements in AI capabilities, and the proliferation of cloud services, which make big data tools more accessible.
Additionally, there’s a forecasted surge in demand for professionals skilled in big data analysis. Organizations will look for experts who can navigate this complex landscape, ensuring that data not only serves its purpose but does so ethically and effectively.
In summary, the future of big data analysis companies is charged with innovation and possibility. By embracing trends like AI integration and real-time analytics, these companies can provide invaluable insights to drive growth and transformation. The key lies in navigating the challenges that come with such rapid change to remain leaders in a data-centric world.
"Data is like oil. It’s worthless until it’s refined."
This quote encapsulates the very essence of what these companies aim to achieve as they innovate and adapt in the evolving digital landscape.
Finale
In a world inundated with data, the role of big data analysis companies has never been more crucial. These companies stand at the crossroads of technology and business, guiding organizations through the complexities of data management to harness insights that drive decision-making and strategy. As we have explored throughout this article, the implications of their work extend to various sectors, fundamentally reshaping how businesses operate and compete.
Summary of Key Points
To encapsulate the main takeaways from this discussion:
- Understanding Big Data: Grasping the foundational elements of big data—its characteristics, importance, and implications—is vital for any organization looking to leverage its capabilities.
- Role of Big Data Analysis Companies: These entities provide expertise, tools, and technologies necessary to process vast amounts of data efficiently.
- Challenges and Solutions: From data privacy issues to technical limitations, big data companies face numerous challenges, yet they continually innovate to overcome these obstacles.
- Future Outlook: With emerging trends like AI integration and real-time analytics, the landscape of big data analysis is poised for rapid evolution.
This summary illustrates a roadmap that organizations must consider when embarking on their data-driven journey, helping them navigate the intricacies of big data effectively.
Final Thoughts on Big Data Analysis
Big data analysis does not merely represent a trend; it's a fundamental shift in how we interact with information. For tech enthusiasts and industry professionals alike, recognizing the importance of big data is essential.
Take a moment to reflect: every data point tells a story—a story that can lead to strategic advantages and growth opportunities. Companies that embrace this narrative will find themselves better equipped to anticipate market shifts and respond to consumer needs.
"Data is the new oil; it's only valuable if refined."
This industry has challenges, but those hurdles are what drive innovation. We are witnessing the dawn of a new era in business, one where data informs everything—from daily operations to long-term strategies. Therefore, as we look ahead, it’s crucial for organizations to invest in big data capabilities, understanding that the potential rewards far exceed the risks when properly managed.