Choosing the Best Tools for Data Analysis
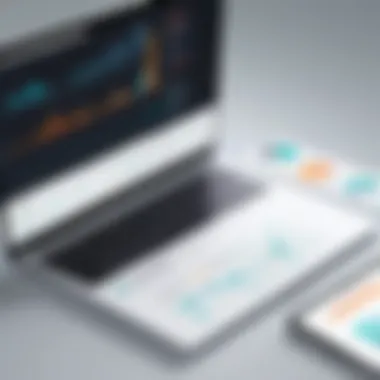
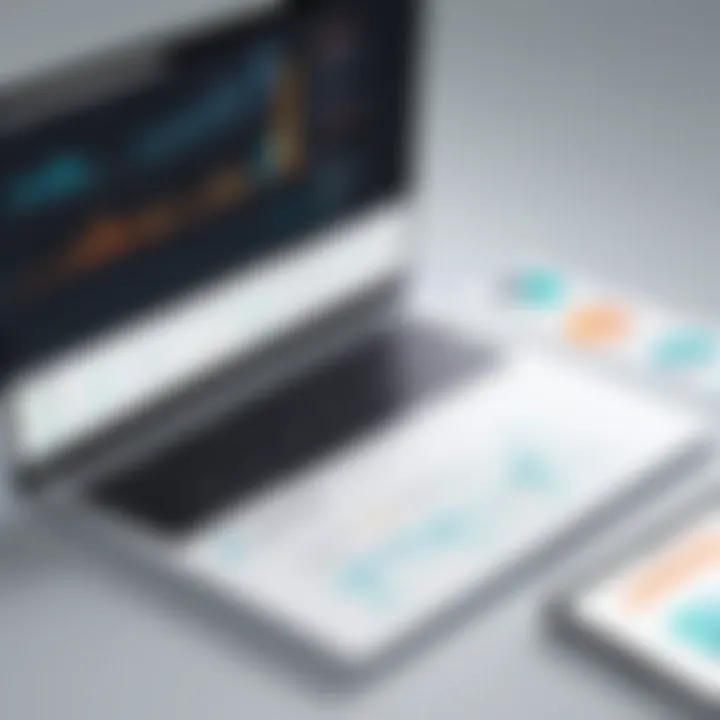
Intro
In today’s data-driven world, selecting the appropriate data analysis tool is crucial for success. Analysts have access to an array of tools, each with unique features and specialized capabilities. Professionals in various fields must decipher which tool best fits their specific analytical demands. Therefore, this discussion will guide readers through essential factors when choosing a data analysis tool to enhance their workflow and decision-making processes.
Understanding the fundamental components of these tools provides clarity. This involves evaluating their functionalities, learning curves, and adaptability to different environments. In the following sections, we will delve into current trends regarding data analysis tools and other key considerations for effectively navigating this landscape.
Tech Trend Analysis
Overview of the current trend
The landscape of data analysis is rapidly evolving. There is an increasing reliance on cloud-based solutions and open-source tools due to their flexibility and scalability. Products like Tableau, Power BI, and R are becoming the standard for organizations seeking agile solutions.
These tools enable analysts to process data more swiftly, making real-time analysis possible. Increased data volumes and the growing need for instantaneous insights are driving these trends. This shift towards modern tools reflects a broader movement toward democratizing data access across industries.
Implications for consumers
For consumers, particularly those in business, these trends imply access to sophisticated tools without the need for extensive training or resources. The rise of user-friendly interfaces means that even those with limited technical expertise can analyze data effectively. The accessibility of educational resources and community support further enhances this experience.
Future predictions and possibilities
Looking ahead, it is likely that artificial intelligence and machine learning will further integrate into data analysis tools. The capability of these technologies to automate complex tasks will reduce manual workload significantly. Moreover, we might see an increase in collaborative features, allowing teams to work more synergistically. Such advancements promise to evolve the way professionals harness data for strategic decisions.
Product Reviews
Overview of the product
One significant angle to consider is the in-depth evaluation of popular data analysis tools. Excel, for example, remains a staple due to its broad adoption and familiarity among users. However, other tools like SAS and SPSS are gaining traction in more specialized environments such as academic and research institutions.
Features and specifications
Each tool has distinct characteristics:
- Excel: Spreadsheet-based tool, offering pivot tables and various functions.
- Tableau: Focuses on visualization with interactive dashboards.
- Power BI: Integrates seamlessly with Microsoft products for robust analytics.
Performance analysis
In terms of performance, tools like Tableau outperform in visualization tasks, while Excel remains unmatched in data manipulation features. Data processing speed is also a vital metric, with cloud-based tools like Google Data Studio making strides in rapid processing.
Pros and cons
- Excel:
- Tableau:
- Pros: Widely used, good for basic analysis.
- Cons: Limited for large datasets and advanced analytics.
- Pros: Excellent visualization capabilities.
- Cons: Higher learning curve, cost associated with licensing.
Recommendation
Choosing the right tool ultimately depends on the specific analytical requirements. For those needing foundational analysis or modeling, Excel may suffice. However, organizations focused on deep insights through visualizations might consider Tableau or Power BI.
How-To Guides
Prologue to the topic
This section focuses on understanding how to effectively select and implement data analysis tools.
Step-by-step instructions
- Identify specific data needs and determine the required analysis type.
- Research tools that align with those requirements.
- Access trial versions or demos to gauge usability.
Tips and tricks
- Always consider scalability based on future needs when selecting a tool.
- Check for community support as it is essential for troubleshooting.
Troubleshooting
In case of issues, utilize online forums or the official documentation provided by the tool's developers. Websites like Reddit often have user discussions that can provide insights into common challenges and solutions.
Industry Updates
Recent developments in the tech industry
The shift toward integrated analytics solutions has taken center stage. Companies are increasingly adopting platforms that allow seamless integration of data from various sources.
Analysis of market trends
Enterprise solutions seem to be focusing on providing tools that foster collaboration while maintaining robust security measures.
Impact on businesses and consumers
As technology advances, businesses that can adapt their data strategies will likely secure a competitive advantage. Users can leverage emerging tools to enhance their analytical capabilities, leading to more informed decision-making.
Preface to Data Analysis Tools
In the ever-evolving landscape of technology, data analysis tools serve as the backbone of informed decision-making. Choosing the right tool is not merely a matter of convenience; it directly impacts the effectiveness of analysis outcomes. This choice can influence the depth of insights, the efficiency of data processing, and the speed at which results are generated.
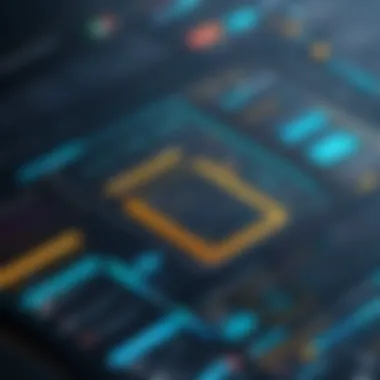
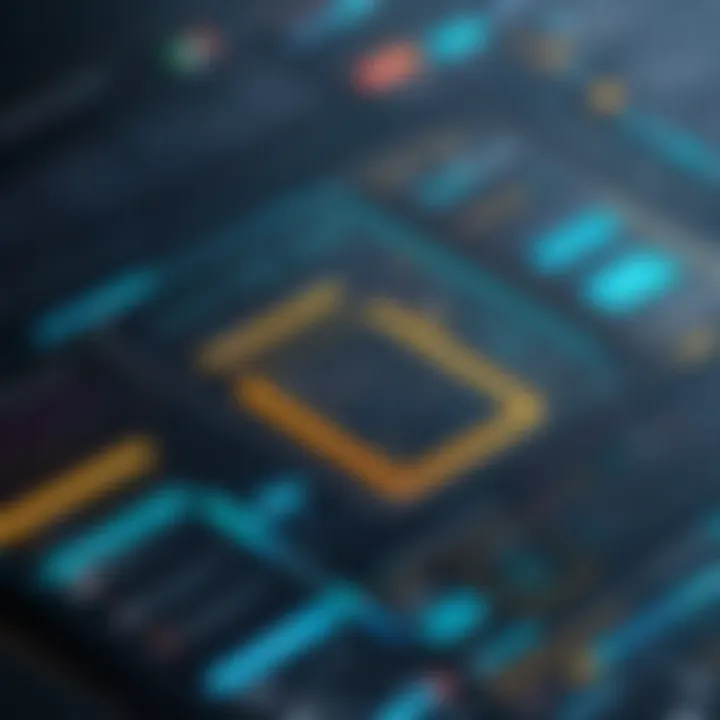
The diversity of tools available today ranges from spreadsheets to complex software designed specifically for data science applications. Understanding the variety and functionalities of these tools is essential for both industry professionals and tech enthusiasts alike. Each tool has its strengths and weaknesses, and the necessity of aligning these with specific analytical needs cannot be overstated.
Selecting the optimal data analysis tool involves careful consideration of factors such as user interface, scalability, integration capabilities, and community support. By exploring these elements, one can better appreciate the landscape of analysis tools. This enables professionals to make informed choices that enhance their productivity and analytical capabilities.
Understanding Data Analysis
Data analysis is a systematic approach to interpreting data in order to extract useful information and make conclusions. It involves various techniques and tools that can be used across different disciplines, including business, healthcare, and scientific research. The fundamental goal of data analysis is to convert raw data into actionable insights through methods such as data cleaning, modeling, and visualization.
Comprehending data analysis techniques can significantly elevate the analytical process. The more familiar a user becomes with data types and analysis methods, the better they can leverage the appropriate tools. The methodologies encompass both qualitative and quantitative approaches, thereby enriching the overall analysis.
The Importance of Choosing the Right Tool
Selecting the correct tool for data analysis cannot be taken lightly. The effectiveness of one's analysis hinges on this choice. A wrong decision in tool selection can lead to inefficiencies, potential errors in interpretation, and ultimately flawed conclusions.
Key considerations include:
- Functionality: Does the tool offer the features required for your analysis?
- User Interface: Is it intuitive and easy to navigate?
- Integration: Can it work seamlessly with other systems you use?
- Community and Support: Is there a robust community for assistance and updates?
"A tool that aligns closely with your analytical needs can enhance productivity and lead to better insights."
By focusing on these criteria, one can narrow down the options available. Ultimately, the right tool enhances decision-making, leads to improved data management, and fosters better outcomes across various applications.
Criteria for Selecting Data Analysis Tools
Selecting the right data analysis tools is essential for effective data-driven decision-making. The choice of tool can influence not just how data is processed, but also the insights that can be gleaned from it. As professionals embark on their analytical tasks, understanding the criteria for tool selection becomes pivotal.
The multifaceted nature of data analysis necessitates that several factors be evaluated before settling on a tool. These factors include functionality, ease of use, integration capabilities with existing systems, scalability for growth, and the availability of community support. These elements form the backbone of efficient data analysis, ensuring that professionals can derive meaningful conclusions from their data without unnecessary hindrances.
Functionality and Features
Functionality refers to the capabilities of a tool and how well it aligns with specific analytical needs. Different tools come packed with various features that cater to distinct requirements. For instance, some tools excel in statistical analysis while others might be superior for data visualization or machine learning.
Key functionalities to look for include:
- Data import/export capabilities. Can the tool handle various data formats?
- Statistical functions. Does it provide the necessary computations for analysis?
- Visualization tools. Are there features that facilitate graphical representation of data?
Choosing a tool with a robust feature set minimizes the chances of outgrowing the tool's capabilities as analytical needs evolve.
Ease of Use
A tool's usability is crucial, particularly for teams that may not possess advanced data analysis skills. An intuitive interface can significantly enhance productivity. Users should feel comfortable navigating the tool, accessing features, and interpreting results.
Consider the following factors when evaluating ease of use:
- User interface design. Is it clean and straightforward?
- Learning curve. How long does it take to become proficient?
- Accessibility of resources. Are there tutorials or help centers available?
Selecting a user-friendly tool allows teams to focus more on analysis rather than grappling with complex software.
Integration with Other Systems
In today’s interconnected work environments, integration capabilities are increasingly important. A data analysis tool should seamlessly integrate with existing systems like databases, software applications, and other analytical platforms. This interoperability aids in streamlining workflows and reduces data silos.
Key considerations include:
- API availability. Can the tool easily connect with other applications?
- Data transfer capabilities. Is it easy to pull data from other sources?
- Collaboration tools. Does it support sharing and collaboration among team members?
The right integrations enable more fluid data processes and contribute to a more holistic analytical view.
Scalability Considerations
A crucial aspect of tool selection is its scalability. As organizations grow and data volumes increase, the tools they use should be able to accommodate this expansion. Tools that are not scalable may lead to performance issues or become obsolete, hindering the analytical process.
To assess scalability, ask the following:
- Performance under load. How does it handle increasing amounts of data?
- Feature expansion. Are there additional features available as needs grow?
- Cost implications. Does scaling up the tool incur significant costs?
Choosing a scalable tool ensures that the analytical framework can evolve alongside the organization.
Community and Support
The level of community engagement and support available for a tool can significantly affect user experience. A strong community can provide assistance, share best practices, and foster collaboration among users.
Look for:
- Active forums or groups. Are there spaces for users to ask questions and share solutions?
- Official support channels. Is there sufficient technical support available from the developers?
- Documentation. Does the tool come with comprehensive user manuals and resources?
A supportive community not only enhances the learning experience but also helps in troubleshooting and enhancing effective use of the tool.
Selecting the right tool requires careful consideration of several criteria. This proactive approach to evaluation ultimately leads to better data insights and informed decision-making.
Overview of Popular Data Analysis Tools
The landscape of data analysis tools is vast and ever-evolving. In this section, we will explore several popular tools that have proven their worth in various fields. Understanding these tools is essential, as they each offer unique features and benefits tailored to different needs. Professionals must be aware of the strengths and limitations of each option to make informed choices.
These tools are selected based on their functionality, community support, and readiness to adapt to emerging data challenges. Knowing the capabilities of various platforms can enhance not only the analysis process but also impact overall data-driven decision-making across organizations.
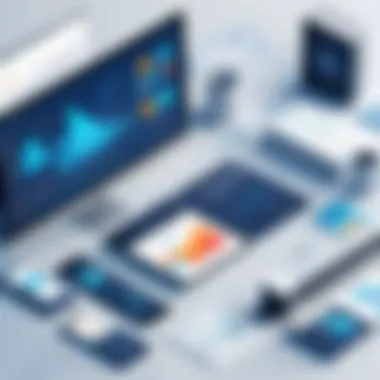
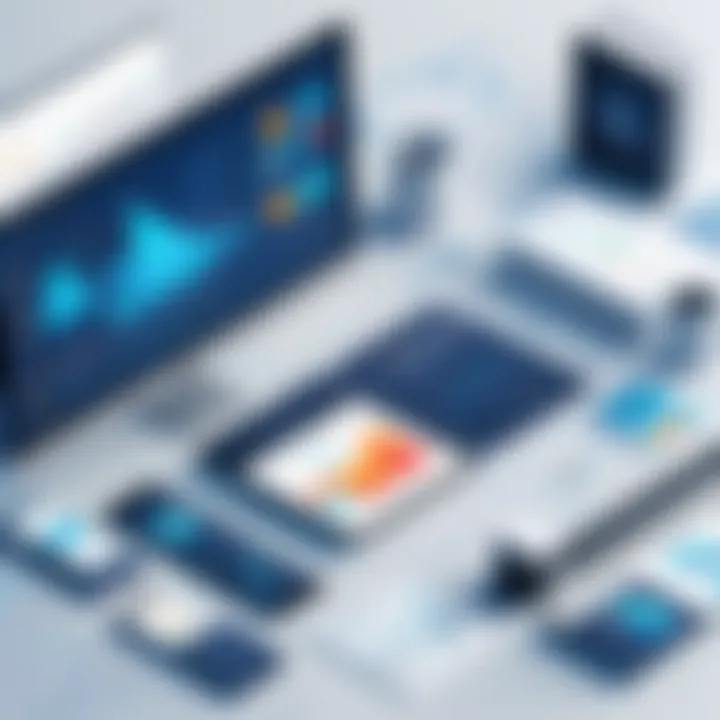
Excel: A Timeless Approach
Microsoft Excel remains one of the most widely-used tools for data analysis. It is often regarded as the go-to solution for many professionals due to its familiarity and accessibility. Excel offers an array of functions, from basic data manipulation to complex statistical analysis.
Key benefits include:
- User-friendly interface: Most users can navigate its features easily.
- Versatile functionality: It accommodates various data formats and offers comprehensive charting capabilities.
- Widely supported: Many organizations already use Excel, making collaboration easier.
However, Excel can struggle with very large datasets and might fall short when advanced analytical capabilities are required.
R: The Go-To for Statistical Analysis
R is a programming language specially designed for statistical computing and graphics. This makes it a preferred tool for statisticians and data miners.
R provides a flexibility that is often unmatched by other tools, allowing for complex analyses and visualizations.
Some elements to note about R include:
- Rich ecosystem of packages: Users can easily find libraries tailored to specific analysis needs.
- Customizable visualizations: Creating detailed plots is straightforward and can be highly interactive.
Despite its depth, R may present a steeper learning curve for non-programmers compared to more visual tools.
Python: Versatile and Powerful
Python is another programming language that has gained traction in the data analysis field. It is known for its simplicity and readability, making it beginner-friendly while offering robust capabilities for experienced users.
Features include:
- Extensive libraries: Libraries such as Pandas and NumPy provide powerful data manipulation capabilities.
- Integration with other technologies: Python easily integrates with web applications and databases.
As data analysis demands continue to grow, Python's versatility makes it a strong contender across sectors.
Tableau: Visual Data Representation
Tableau is software focused on data visualization. Its strength lies in transforming complex datasets into visually appealing and easy-to-understand graphics.
Benefits of using Tableau are:
- Drag-and-drop functionality: Users can quickly create visualizations without extensive technical knowledge.
- Real-time data insights: It allows for live connections to data sources, enabling up-to-date analysis.
Nonetheless, while Tableau excels at visualization, it may not offer the depth of analysis found in tools like R or Python.
Power BI: Business Intelligence Simplified
Microsoft Power BI stands out in the domain of business intelligence. This tool provides a bridge between data analysis and strategic decision-making.
Key features include:
- Integration with Microsoft products: Seamless incorporation with Excel and Azure enhances productivity.
- User-friendly interface: Business users find it approachable for generating reports.
However, some advanced analytics capabilities might not be as robust as those found in other specialized tools.
SAS: Advanced Analytics and Data Management
SAS (Statistical Analysis System) is known for its powerful analytics capabilities. It is often used in industries such as healthcare and finance, where precision is critical.
Noteworthy aspects include:
- Comprehensive suite of tools: SAS provides extensive functionalities for various types of analysis.
- Strong support: A well-established community and customer service enhance user experience.
The main drawback is the high cost associated with obtaining licenses for its software.
Apache Spark: Big Data Processing
Apache Spark excels at handling large-scale data. It is designed for processing vast amounts of distributed data efficiently.
Key advantages are:
- Speed and scalability: Spark is faster than traditional data processing frameworks.
- Support for multiple languages: Users can write applications in Java, Scala, or Python.
However, setting up and managing Spark can be complex, which may deter smaller organizations.
Google Analytics: Web Data Analysis
Google Analytics is dedicated to web data analysis. It offers businesses insight into their online presence and customer behavior.
Notable features include:
- Comprehensive tracking: Track user interactions and website performance easily.
- Free service: Many companies benefit from its free version, which is feature-rich.
However, while suitable for web analytics, it lacks broader data analysis capabilities common in dedicated analytics tools.
Evaluating the Best Tool for Specific Needs
When it comes to data analysis, no one-size-fits-all solution exists. Every organization or individual has unique needs based on the scale of operations, data complexity, and specific objectives. Evaluating the best tool for specific needs is critical because the right choice can enhance productivity, streamline operations, and ultimately lead to more insightful data-driven decisions.
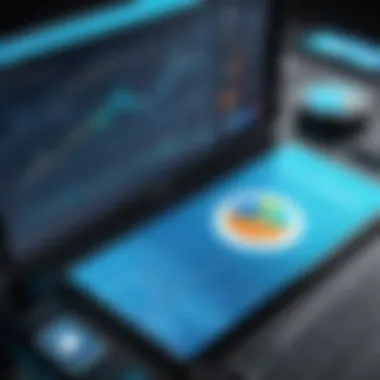
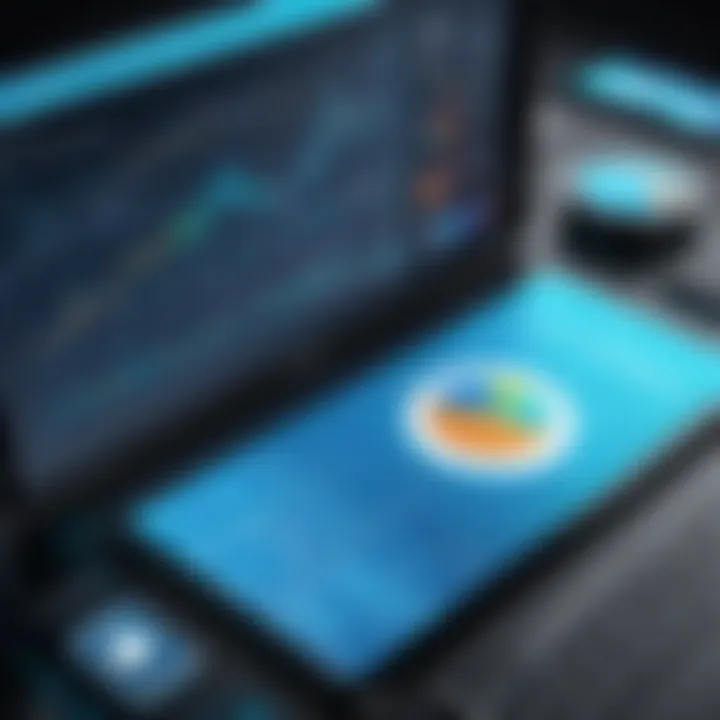
Various factors come into play when determining the optimal tool. These include cost, ease of integration, and the level of expertise required. Each category of user—be it small businesses, large enterprises, or academic institutions—will have different priorities and constraints that shape their decision-making process when selecting tools for data analysis.
Identifying the right tool is not merely about functionality; it encompasses understanding the wider context in which the tool will be used, and how it aligns with specific goals. This section will delve into different user categories to provide insight into how each segment can best approach their selection.
For Small Businesses
Small businesses often operate with limited budgets and resources. Thus, when choosing a data analysis tool, they must prioritize cost-effectiveness alongside functionality. Tools like Microsoft Excel or Google Sheets may serve as affordable entry points for data analysis, offering essential features without overwhelming complexity.
Implementing a robust tool can provide several advantages:
- Accessibility: Many tools have cloud-based options, allowing team members to collaborate in real-time.
- Simplicity: User-friendly interfaces are vital as small business staff may not have technical backgrounds.
- Scalability: As the business grows, the tool should easily adapt to increased data volumes and complexity.
A careful assessment of these factors ensures that small enterprises can efficiently harness data analysis without costly investments or requiring specialized training.
For Large Enterprises
In contrast, large enterprises often have more complex needs due to their size and varied departments. A wide array of data needs and regulatory compliance protocols requires a more advanced tool. Solutions like SAP BI or SAS deliver sophisticated functionalities that allow for in-depth data manipulation and analysis.
Important criteria to consider include:
- Integration: The tool must seamlessly connect with existing systems like CRM platforms or databases.
- Customizability: Enterprises should look for tools that allow for tailored features to meet specific departmental requirements.
- Advanced Analytics: Features such as predictive analytics or machine learning capabilities can support strategic decision-making.
Large organizations should focus on tools that not only facilitate data analysis but also reinforce overall business intelligence initiatives.
For Academic Research
In academic settings, the emphasis often shifts towards statistical rigor and reproducibility of analysis. Tools such as R and Python offer powerful programming environments that support complex statistical tests and data visualization. They are essential for advancing research findings.
When evaluating tools for research, consider:
- Flexibility: The ability to customize analysis according to specific research needs can be crucial.
- Community Support: Many academic tools have active communities that provide resources, tutorials, and forums for discussions.
- Open-Source Alternatives: For budget-conscious academics, open-source tools can offer extensive functionalities without the associated costs of proprietary software.
Selecting the right tool can influence the integrity and outcomes of research projects, shaping the future direction of studies.
For Data Scientists
Data scientists require tools capable of handling large datasets and complex calculations. They often favor programming languages like R or Python that provide extensive libraries for data manipulation and machine learning.
Key considerations include:
- Performance: Tools need to efficiently process large datasets.
- Advanced Capabilities: Features such as model deployment and real-time data analysis can enhance a data scientist’s ability to deliver insights.
- Collaboration Support: Many projects require teamwork, so tools that allow easy sharing and version control are favorable.
The right selection can significantly impact the efficiency and effectiveness of data science projects, ensuring the extraction of valuable insights from data.
For Marketing Professionals
Marketing professionals utilize data analysis to understand consumer behavior, measure campaign effectiveness, and predict future trends. Tools like Google Analytics provide essential insights into web traffic and user engagement. Key factors to consider include:
- Ease of Use: Marketing teams may not always have a technical background; thus, user-friendly interfaces are essential.
- Integration with CRM: Analyzing customer behavior requires data from various sources, necessitating a tool that integrates smoothly with other platforms.
- Real-Time Data: The ability to analyze data in real-time can lead to more agile marketing strategies.
Choosing the optimal tool can empower marketing teams to make informed decisions, aligning their strategies with data-driven insights.
The Future of Data Analysis Tools
The landscape of data analysis is ever-evolving, shaped by technological advancements and changing business needs. Understanding the future of data analysis tools is crucial for professionals aiming to stay ahead in a competitive environment. As the volume of data increases, tools must adapt to enhance performance, usability, and integration capabilities. Recognizing upcoming trends and emerging technologies allows organizations to make informed decisions about their analytical strategies.
Trends Influencing Data Tools
Several key trends are currently influencing the development of data analysis tools. These trends not only shape the tools of today but also lay the groundwork for future innovations:
- Increased Automation: Tools are becoming more automated, meaning less manual intervention and quicker insights. Automated data cleaning, processing, and analysis will save time and minimize human error.
- Augmented Analytics: This trend refers to using machine learning and artificial intelligence to assist in data analysis. By providing analytical insights and recommendations, augmented analytics reduces the burden on data professionals and enhances decision-making processes.
- Real-time Analytics: The shift towards real-time data processing is significant. Businesses require instantaneous insights to respond to market changes promptly. Tools that facilitate real-time analytics will become widespread as organizations seek agility.
- Data Democratization: There is a push to make data accessible to non-experts. Intuitive user interfaces and easy-to-use platforms allow a broader audience to engage with data analytics. This democratization is essential for fostering a culture of data-driven decision-making across different levels of an organization.
These trends indicate a shift towards tools that enhance productivity, accessibility, and effectiveness in data analysis.
Emerging Technologies and Their Impact
Several emerging technologies hold the potential to significantly alter the data analysis landscape:
- Artificial Intelligence and Machine Learning: These technologies are becoming integrated into data tools. They help in automating complex analyses, discovering patterns, and predicting trends. With AI, tools can offer metric insights that were previously unattainable.
- Cloud Computing: The move to the cloud enhances collaborative analytics. Teams can work together in real time, sharing data and insights regardless of location. Cloud tools also provide scalability, allowing companies to manage data needs better as they grow.
- Blockchain Technology: Although primarily known for its association with cryptocurrency, blockchain has applications in securing and validating data integrity. This technology is particularly useful in industries where data provenance is critical, such as finance and healthcare.
- Internet of Things (IoT): As IoT devices proliferate, the data generated from these devices requires sophisticated analytical tools. Analytics tools that can process and interpret data from numerous devices will become essential for comprehensive analysis.
"Emerging technologies will undoubtedly transform the approach individuals and organizations take towards data analysis."
Closure
In the landscape of data analysis, the conclusion drawn from thorough exploration is significant. This section synthesizes insights and considerations discussed throughout the article. The optimal tool for data analysis is not merely a matter of preference; it hinges on various specifications relevant to the user’s requirements. Key aspects include understanding one's objectives clearly and having a firm grasp on the nature of the data being handled.
Choosing an appropriate data analysis tool can enhance productivity, foster insightful interpretation, and streamline workflows.
A reasonable selection enhances a user’s efficiency when conducting analyses. Users must consider various factors, such as the tool’s functionality and features, which dictate how effectively one can manipulate and derive meaning from data. Moreover, factors like ease of use and integration capabilities play crucial roles in ensuring smooth operations within the existing data ecosystem.
Beyond mere functionality, scalability is essential to accommodate future growth and changing demands in data volume. As businesses evolve, their analytical needs will also shift. Therefore, choosing a tool that remains viable in the long term is paramount.
Community support is also a component of successful tool deployment. A rich support network can provide valuable resources, troubleshooting assistance, and a platform for sharing knowledge among users. This enhances the learning experience and increases the capabilities of the tool in question.
Finally, taking insights from the criteria discussed should empower users to navigate the selection process based on their own context. It positions them to make informed decisions that align with their professional and organizational goals.
Key Takeaways
- Understand your specific data analysis needs and objectives.
- Evaluate tools based on functionality, usability, and scalability.
- Consider integration with existing systems to maintain workflow efficiency.
- Leverage community support for ongoing learning and problem-solving.
Final Recommendations
When selecting a data analysis tool, it’s crucial to conduct a comprehensive evaluation tailored to personal or organization objectives. Excel remains a solid choice for beginners and general use, while R and Python offer powerful options for advanced statistical analysis and data science tasks. For visual representation, Tableau and Power BI stand out, while SAS excels in complex data management scenarios. Ultimately, ensure the tool aligns with your long-term goals and evolves with your needs.