Exploring AI Use Cases in the Banking Sector
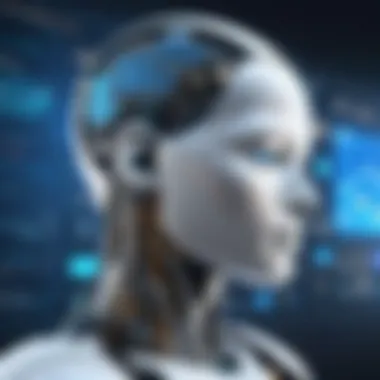
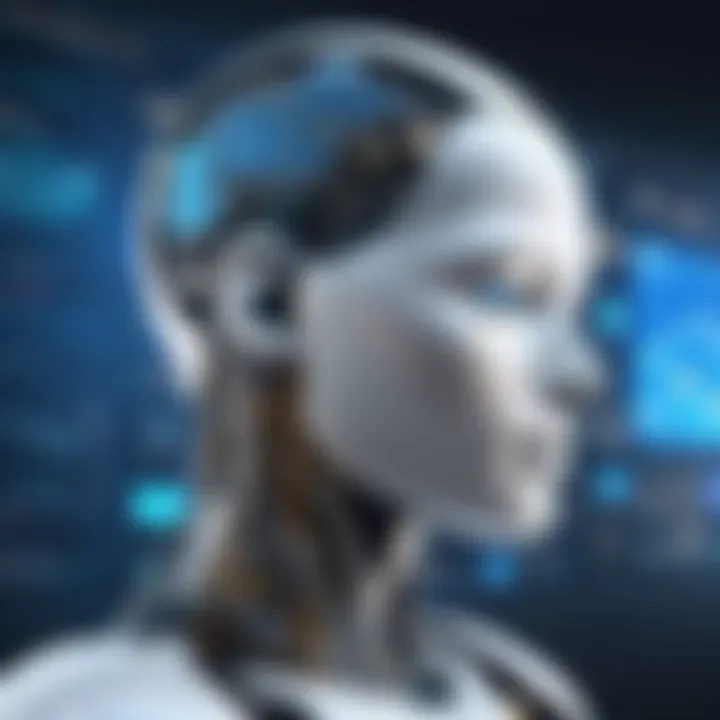
Intro
The intersection of artificial intelligence and banking is not just a trend; it is a significant evolution in financial services. With the capacity to harness vast amounts of data, AI technologies have emerged as essential tools for banks aiming to enhance operational efficiency, improve customer service, and better manage risks. In a sector often defined by regulatory scrutiny and a need for trust, AI introduces a level of sophistication in approach that can fundamentally shift traditional practices.
In this article, we will explore various applications of AI within the banking sector, including risk assessment models and personalized banking services. The examination will also cover the implications of these changes for consumers and financial institutions alike. Let's start with a detailed look at the current state of AI in banking.
Foreword to AI in Banking
Artificial Intelligence (AI) is becoming crucial in the banking sector. The ongoing evolution of technology has transformed how banks operate, innovate, and interact with customers. This segment will discuss the significance of AI in banking and the various advantages it delivers.
AI holds various benefits for the banking industry. First, it enhances operational efficiency by automating routine tasks. This allows employees to focus on more complex responsibilities that require human judgment. Furthermore, AI can analyze vast datasets far quicker than a human could, which leads to more accurate decision-making. It also helps in managing customer relationships via personalized services, creating a better user experience.
However, there are critical considerations to bear in mind regarding the introduction of AI technologies. Implementation costs can be substantial, and there are concerns around data privacy and ethics. Additionally, the perceived threat to jobs due to automation raises questions about the social implications of these technologies.
In summary, the introduction of AI in banking open new doors for both operational growth and service enhancement. Understanding how these technologies function and their integration into existing banking systems is vital. This will help in realizing their full potential while navigating potential challenges.
Overview of AI Technologies
AI technologies can be categorized into several key components that influence the banking sector. Machine learning, natural language processing, and computer vision are central to these advancements.
- Machine Learning: This subset of AI enables systems to learn from data patterns and improve their performance over time without explicit programming. In banking, it is used for tasks such as fraud detection and credit scoring.
- Natural Language Processing (NLP): NLP allows machines to understand and interpret human language. Applications include chatbots and virtual assistants that help customers with inquiries and service requests.
- Computer Vision: This technology enables computers to interpret visual information from the world, which can optimize processes like document verification or streamline administrative tasks.
These tools work collectively to enhance productivity and provide tailored services, showcasing AI's transformative potential in the banking industry.
Historical Context of Banking and Technology
The relationship between banking and technology is not new. Historically, banks have adapted to technological changes to improve services. From the introduction of ATMs to online banking, technology has consistently reshaped how financial institutions operate.
In the late 20th century, internet banking started to emerge. This allowed customers to access their accounts and conduct transactions online. As a result, banks began to focus on improving security and customer experience. The rise of mobile banking in the 21st century marked another milestone. With the advent of smartphones, customers gained unprecedented access to banking services on the go.
Today, we are witnessing another paradigm shift with AI technologies. The integration of AI into banking processes allows for further automation and personalization. As banks aim to stay competitive and meet customer demands, embracing AI is not merely an option but a necessity.
The evolution of technology in banking continues to accelerate. Understanding this historical context can provide insights into the future development of banking services.
Operational Efficiency Enhancement
The significance of operational efficiency in banking cannot be overstated. As the financial services industry evolves, institutions must adapt to stay competitive. In this context, the application of artificial intelligence plays a critical role. AI enhances operational efficiency by streamlining various processes, reducing costs, and improving response times. This section will discuss how automation of routine tasks and AI-powered process optimization contribute to efficiency in banking.
Automation of Routine Tasks
Involving automation in banking processes greatly reduces human error and speeds up standard operations. AI tools are designed to take over repetitive tasks that typically consume valuable employee time. For example, data entry, report generation, and transaction processing can be automated. This allows staff to focus on higher-level tasks, fostering innovation and enhancing customer service.
Some specific advantages of automating routine tasks are:
- Cost Savings: Less manual work means lower labor costs.
- Time Efficiency: Processes that used to take hours can now be completed in minutes.
- Increased Accuracy: Machine learning algorithms reduce the likelihood of errors that might arise from manual data handling.
Many banks have already begun implementing automation solutions. For instance, JPMorgan Chase has utilized AI to automate its legal document review processes, saving significant hours of operations.
AI-Powered Process Optimization
AI empowers banks to optimize their processes, leading to more effective operations overall. Process optimization involves enhancing existing workflows to maximize efficiency and minimize waste. Through data analysis, AI identifies bottlenecks and inefficiencies that may not be visible to human analysts. The continuous feedback loop provided by AI ensures that processes are regularly updated based on real-time data.
Some of the elements accounted for in process optimization include:
- Predictive Maintenance: AI systems can predict issues before they become significant, ensuring smooth operations.
- Dynamic Resource Allocation: Optimizing resources in real time allows banks to better serve customer demand without unnecessary expenditure.
By integrating AI into process optimization strategies, banks not only improve their operational efficiency but also enhance overall service quality. As the industry continues to embrace technology, these enhancements will likely become standard practice.
"The integration of AI in banking represents not just a trend but a fundamental shift in how financial institutions operate. It redefines efficiency and accountability in every transaction and strategy."
Customer Experience Improvement
The concept of customer experience improvement is central within modern banking. As financial institutions face increasing competition and a shift in consumer expectations, enhancing customer experience has become a primary focus. By implementing advanced technologies like artificial intelligence, banks can tailor their services to meet the unique needs of each customer, thus fostering loyalty and satisfaction.
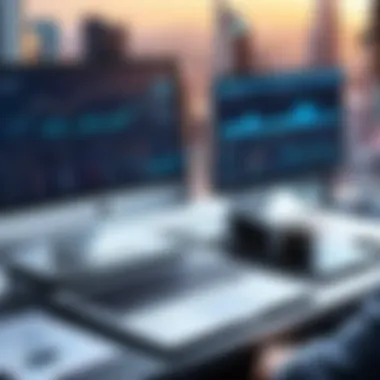
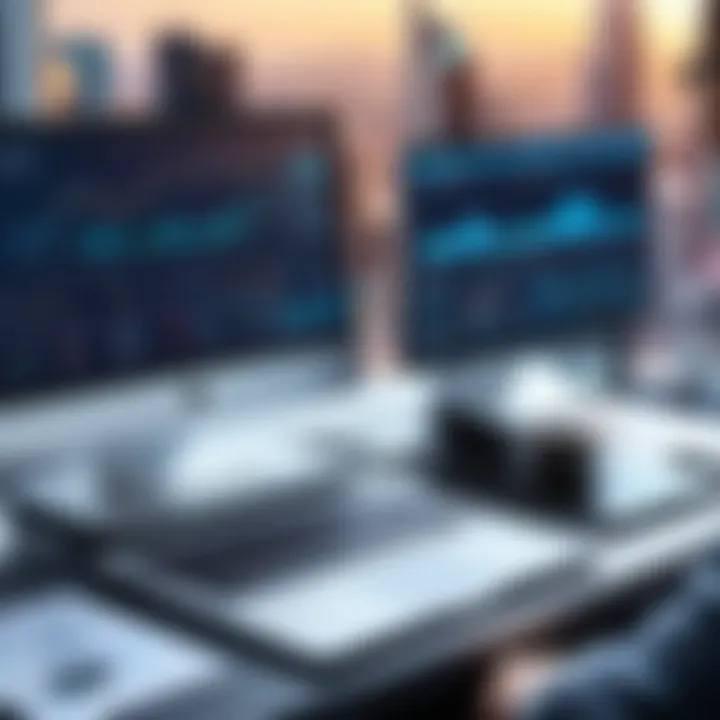
The benefits of improving customer experience are manifold. A more personalized approach can lead to higher customer retention rates, as satisfied customers are less likely to switch to competing banks. Moreover, enhanced customer experience can result in increased revenue through cross-selling and upselling products. Banks can better understand customer behavior and preferences through data analysis, allowing them to create targeted marketing strategies.
It is essential for banks to consider various elements when focusing on customer experience improvement. Key considerations include the accessibility of services, speed of transactions, and the clarity of communication. Moreover, banks must ensure that their technology is user-friendly, particularly for less tech-savvy individuals. Ultimately, a holistic approach that integrates AI can significantly enrich the overall banking experience.
Chatbots and Virtual Assistants
Chatbots and virtual assistants have revolutionized the way banks interact with their customers. These AI-driven tools can provide 24/7 support, answering questions and resolving issues outside of regular business hours. This immediacy plays a crucial role in customer satisfaction, as individuals increasingly expect instant responses to their inquiries.
The implementation of chatbots comes with several advantages. They can handle a large volume of queries simultaneously, drastically reducing wait times compared to traditional customer service channels. Furthermore, chatbots can offer consistent and accurate information while freeing up human agents to focus on more complex issues that require nuanced understanding.
In addition, chatbots are capable of learning from interactions, enhancing their responses over time. This continuous improvement can provide a more tailored experience for users. For instance, a chatbot might recognize a customer’s past interactions and recommend specific services or products based on their preferences. Overall, chatbots and virtual assistants significantly improve the efficiency and effectiveness of customer service in banking.
Personalized Banking Services
Personalized banking services represent a significant advancement in how financial institutions cater to their customers. By leveraging AI technologies, banks can analyze vast amounts of data to identify individual customer needs and deliver customized offerings. This level of personalization enhances customer engagement and fosters long-lasting relationships.
For example, predictive analytics can allow banks to anticipate customer behaviors, such as spending patterns or savings trends. By offering tailored financial advice or specific investment opportunities, banks can effectively address the unique goals of each client. Additionally, personalized services can extend to rewards programs, providing incentives that resonate with individual preferences.
Moreover, personalized banking services can streamline the customer journey. Simplifying processes such as loan applications or account management through tailored interfaces can significantly enhance user satisfaction. When customers feel understood and valued, they are more likely to develop loyalty towards their bank, ultimately benefiting the institution's bottom line.
"The shift to personalized banking services is no longer a trend, but a necessity for banks seeking to maintain relevance in a competitive landscape."
Risk Management Advancements
Risk management is vital for the banking sector. In a world with increasing financial uncertainty, advances in AI technologies offer substantial improvements. These enhancements ensure that banks can adapt quickly to risk factors and reduce potential losses. Using artificial intelligence, financial institutions can identify patterns and anomalies in data far more efficiently than manual processes. This transformation not only protects assets but also supports regulatory compliance.
Fraud Detection Systems
Fraud remains a prominent challenge in banking. Traditional systems often fall short of effectively identifying suspicious activities. AI-based fraud detection systems utilize advanced algorithms to analyze transactions in real-time. These systems learn from historical data, constantly improving their accuracy over time.
- Machine Learning Models: This technology identifies complex behavioral patterns. By analyzing past fraudulent activities, AI can flag unusual transactions.
- Real-Time Monitoring: AI systems evaluate transactions instantly. This capability drastically reduces the time from detection to intervention, protecting customers and banks alike.
- Adaptive Algorithms: With continuous data inputs, these models adapt to new fraud techniques, providing an evolving defense against threats.
"The use of AI in fraud detection redefines what is possible in preventing financial crime."
Credit Scoring Algorithms
Credit scoring is another aspect impacted by AI advancements. Traditional scoring methods often depend on limited financial history and fixed criteria. AI provides a more nuanced approach.
- Dynamic Credit Evaluation: By analyzing various data points, including payment behavior and even social media activity, credit scoring algorithms create a more comprehensive credit profile.
- Predictive Models: These use historical data to forecast future behavior. AI-driven scoring can predict defaults with higher accuracy, helping lenders make informed decisions.
- Risk-Based Pricing: AI allows banks to customize interest rates based on personalized risk assessments, making credit more accessible to a broader audience.
These advancements enhance overall risk management strategies, making banking safer and more efficient.
Regulatory Compliance and Monitoring
In the banking sector, regulatory compliance and monitoring are of paramount importance. Financial institutions operate within a landscape characterized by a myriad of regulations aimed at maintaining the integrity of the financial system. These regulations can range from anti-money laundering (AML) measures to protections surrounding consumer data. Adhering to these regulatory requirements not only mitigates the risk of legal penalties but also preserves the trust of clients.
Artificial Intelligence (AI) plays a crucial role in enhancing compliance functions. By leveraging AI technologies, banks can more efficiently manage the complexities of regulatory frameworks. Automation and predictive analytics enable rapid adaptation to changing regulations. Moreover, continuous monitoring of compliance reduces the chances of oversight, ensuring proactive management of risks associated with non-compliance.
Automation of Compliance Processes
One of the significant advancements in regulatory compliance brought about by AI is the automation of compliance processes. Traditionally, compliance activities were labor-intensive and error-prone, relying heavily on manual processes and extensive documentation. With the integration of AI, banks can efficiently streamline these tasks, saving time and minimizing human errors.
- Risk assessment becomes automated, allowing for real-time analysis of transactions.
- AI-powered systems can flag discrepancies or potential non-compliance issues for immediate review.
- Reporting processes can be expedited through automated generation of compliance reports.
As a result, employees can shift their focus from routine compliance checks to more strategic tasks, enhancing overall productivity within the organization.
AI in Anti-Money Laundering Efforts
AI in anti-money laundering efforts marks another crucial application of technology in regulatory compliance. Money laundering is a significant challenge for the banking sector, as it poses substantial reputational and operational risks. Traditional AML practices often struggle to keep up with evolving tactics employed by criminals. AI offers a robust solution by analyzing vast amounts of transaction data for patterns that may suggest illicit activities.
"AI can significantly increase the efficiency and accuracy of AML measures, allowing banks to better allocate resources and combat financial crime more effectively."
Key capabilities of AI in this domain include:
- Pattern Recognition: AI algorithms can identify suspicious patterns of behavior across diverse customer accounts quickly.
- Real-Time Monitoring: Continuous monitoring of transactions allows for the immediate detection of unusual activities.
- Adaptive Learning: AI systems can learn from previous data, adding to their effectiveness as they identify new emerging threats.
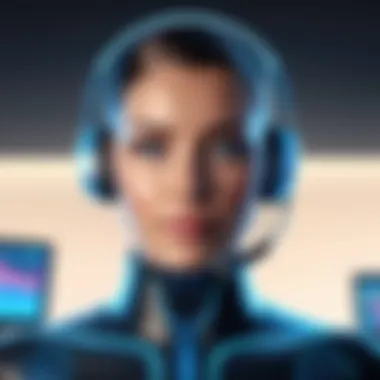
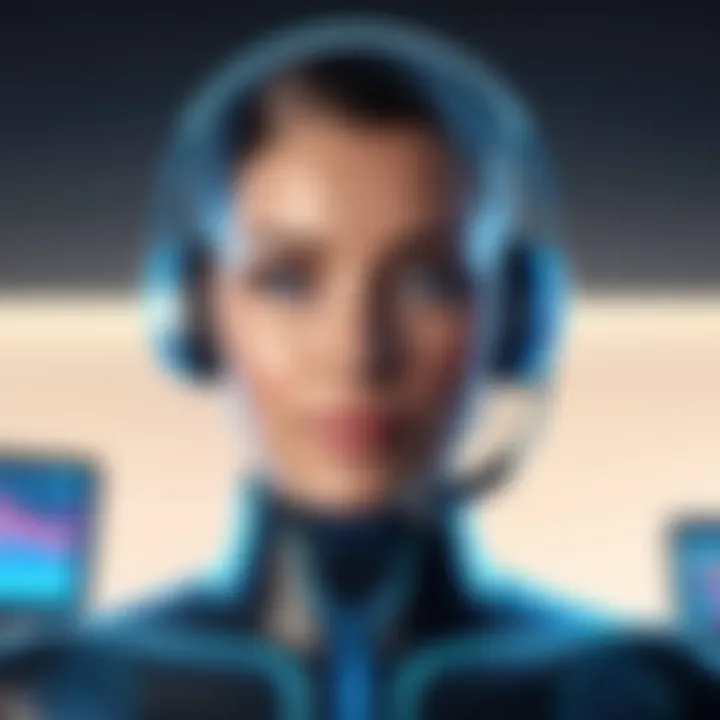
In summary, the integration of AI within regulatory compliance and monitoring processes equips banks with powerful tools to navigate the complex landscape of financial regulations. Through automation and innovative technologies in areas such as AML, banks can improve their compliance strategies while upholding their commitment to ethical practices.
Predictive Analytics in Banking
Predictive analytics has emerged as a cornerstone in modern banking, enabling institutions to leverage vast amounts of data for informed decision-making. This facet of artificial intelligence allows banks to anticipate trends, streamline operations, and enhance customer engagement. The relevance of predictive analytics in banking lies in its ability to provide actionable insights that can drive profitability while minimizing risks. Employing algorithms and statistical models, financial entities can forecast consumer behaviors, market trends, and investment opportunities, which are crucial in a competitive landscape.
Forecasting Consumer Behavior
One of the significant applications of predictive analytics is in the realm of forecasting consumer behavior. By analyzing historical data, banks can predict how customers might react to various banking products and services. This approach encompasses various factors, including spending habits, transaction histories, and demographic information.
Some key advantages of forecasting consumer behavior include:
- Enhanced marketing strategies tailored to specific customer segments.
- Greater retention rates through personalized offerings.
- Anticipation of customer needs leading to proactive service initiatives.
Banks employ several techniques to achieve accurate consumer forecasts, such as logistic regression, decision trees, and clustering algorithms. These methods evaluate large datasets enabling banks to spot patterns that inform their strategies. Moreover, predicting when a customer might require financial assistance or new products aids banks in maintaining relevance and customer loyalty.
Investment and Portfolio Management
Another crucial aspect of predictive analytics in banking is investment and portfolio management. Here, data-driven predictions guide investment decisions, allowing financial advisors and firms to manage portfolios effectively. Predictive analytics tools assist in assessing market risks and opportunities, thus facilitating better-informed investment strategies.
Benefits in this area include:
- Improved risk assessment, leading to more resilient portfolios.
- Dynamic asset allocation based on predictive insights.
- Identification of high-return investment opportunities through data analysis.
Utilizing models such as time series analysis, machine learning, and financial forecasting algorithms enables banks to stay ahead of market trends. By systematically analyzing market data, these institutions can mitigate risks and adapt to fluctuations in real-time, which is vital in the fast-paced financial landscape.
Ultimately, predictive analytics empowers banks to shape strategic decisions rather than just react to market changes.
Challenges and Limitations of AI in Banking
Artificial intelligence is reshaping the banking sector, bringing significant advancements and efficiencies. However, alongside its advantages, there are notable challenges and limitations that need thorough examination. Being aware of these challenges is essential for banks to responsibly implement AI technologies. This section explores two critical areas: data privacy concerns and the integration with legacy systems. Each aspect carries its own risks yet also opportunities that he banks must navigate carefully to ensure ethical and secure use of AI.
Data Privacy Concerns
Data privacy is a primary concern in the implementation of AI within banking. Banks collect vast amounts of personal and financial information from clients. When AI systems are introduced, they often require access to this sensitive data to function effectively. The major worry lies in how this data is processed and safeguarded.
With incidents of data breaches rising globally, customers are increasingly aware of their privacy rights and expect banks to protect their data. A single breach can not only result in financial loss for customers but can also severely damage the bank's reputation and lead to regulatory investigations.
"Data governance should never be an afterthought in AI implementations."
Banks must emphasize robust security measures and transparent data handling practices. This includes
- Continuous monitoring of data practices
- Implementing rigorous encryption methods
- Providing customers with clear privacy policies.
Ultimately, the challenge is balancing the benefits of AI with the necessity of protecting customer data.
Integration with Legacy Systems
Another critical challenge for banks adopting AI is the integration with legacy systems. Many banks were founded decades ago and their operational systems may be outdated. These legacy systems often lack compatibility with modern AI technologies. Consequently, integrating AI solutions into these frameworks is complex and resource-intensive.
Some issues that banks face include:
- High costs associated with upgrading old systems.
- Time requirements for implementation and staff training.
- Increased operational risks during the transition period.
Despite these challenges, successful integration can lead to improved overall efficiency. Banks can modernize their services and gain a competitive edge by adopting AI capabilities. They might consider phased approaches to integration, allowing gradual improvements without disrupting existing services completely. This could also facilitate smoother transitions and reduced risk.
Ethical Considerations in AI Banking Applications
As artificial intelligence (AI) becomes more prevalent in the banking industry, ethical considerations gain prominence. It is essential to address these aspects to foster trust and ensure fair practices. This section highlights two critical elements: bias in machine learning algorithms and transparency and accountability issues.
Bias in Machine Learning Algorithms
Machine learning algorithms are often only as good as the data fed into them. If the training data contains biases, the algorithms may replicate or even amplify these biases. For instance, in lending decisions, biased data might lead to unfair treatment of certain demographic groups. This can result in a discriminatory lending practice, which is not only unethical but can also tarnish the institution's reputation.
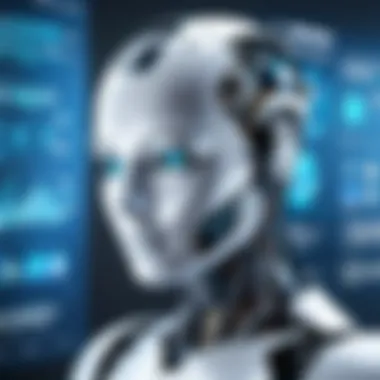
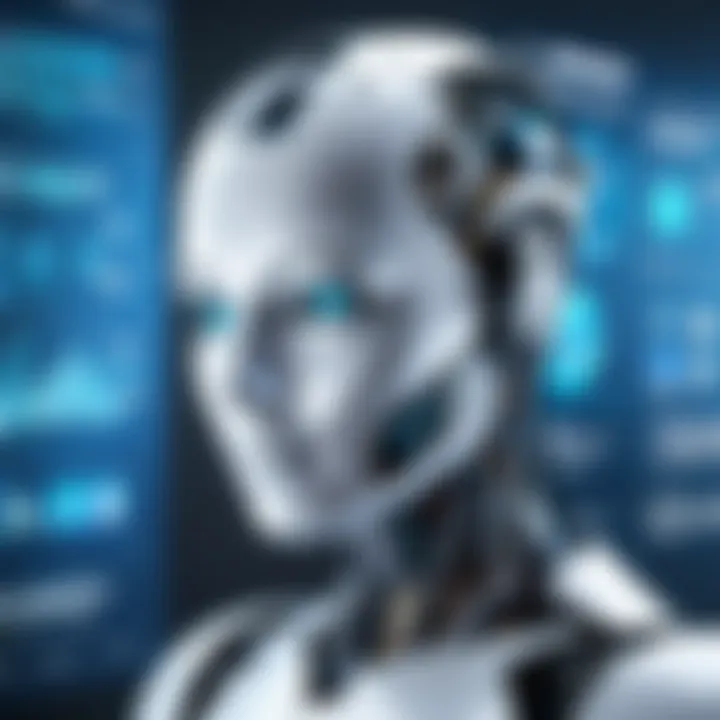
Several banking institutions have recognized the importance of addressing this issue. They are now implementing strategies to mitigate bias. These strategies include:
- Diverse Data Sets: Using diverse data sets to train models can help diminish the impact of bias that arises from non-representative data.
- Regular Audits: Conducting periodic audits of AI systems ensures that the models operate fairly and do not have inherent biases over time.
- Algorithmic Accountability: Having accountability frameworks around AI development can enforce standards to build fair systems.
Organizations need to remain vigilant against biases in AI algorithms to ensure equitable access to financial services. This is not merely a technological issue but a crucial ethical imperative.
Transparency and Accountability Issues
Another vital ethical consideration in AI banking applications is the need for transparency and accountability. As AI systems make decisions that significantly affect customers, stakeholders demand clarity on how these decisions are made. Transparency in AI processes allows customers and regulatory bodies to better understand and trust the systems in place.
Furthermore, accountability mechanisms should be established to address errors and misconduct arising from AI decisions. If a loan is denied or an account is flagged for suspicious activity due to AI-driven conclusions, customers should have pathways to query and challenge those decisions. It underlines the principle of fairness in financial services.
Key aspects to promote transparency and accountability include:
- Explainable AI: Development of models that provide clear explanations and justifications for their decisions can build trust.
- Customer Education: Educating customers about how AI systems work can ease fears associated with automation and loss of human oversight.
- Regulatory Guidelines: Regulatory policies to promote thorough documentation of AI decision-making processes can bolster trust in banking systems.
Maintaining transparency and accountability not only complies with regulatory frameworks but also enhances the customer experience. It promotes a responsible approach to AI deployment in banking.
"Ethical considerations in AI applications are no longer optional; they are essential for sustainable progress and trust."
Future Trends in AI Banking Solutions
The landscape of banking is continually changing due to the advent of artificial intelligence technologies. Understanding the future trends in AI banking solutions is vital for stakeholders who aim to maintain their competitive edge. With the pace of technological development accelerating, banks must adapt to emerging tools that can enhance their operational effectiveness and elevate customer experience. This section will explore the importance of these trends, their implications for the banking industry, and the benefits of integrating innovative AI solutions into existing banking frameworks.
Emerging AI Technologies
The emergence of new AI technologies plays a crucial role in transforming banking practices. Among these technologies, machine learning, natural language processing, and advanced analytics stand out. Each of these contributes to more efficient processes and improved decision-making.
- Machine Learning: This technology allows banks to analyze vast amounts of data, recognizing patterns that can inform risk assessment, fraud detection, and customer behavior analytics. As algorithms evolve, they become more adept at adapting to new data, leading to better predictions and insights.
- Natural Language Processing: NLP is becoming increasingly important in enhancing customer service. It enables chatbots and AI assistants to understand and respond to customer queries in a more human-like manner. This technology improves customer engagement while reducing operational costs associated with traditional customer service methods.
- Advanced Analytics: By leveraging big data and predictive analytics, banks can create tailored products and services. This ability helps financial institutions remain agile, promptly responding to market changes and customer needs.
The incorporation of these technologies does not only provide immediate benefits but also paves the way for long-term strategic advantages. Investing in emerging technologies enables banks to streamline operations and gain deeper insights into the customer base.
Evolution of Customer-Centric Banking
The evolution of customer-centric banking is another significant trend that reflects the increasing focus on tailoring services to individual needs. As banks harness AI technologies, they shift toward creating personalized banking experiences for customers.
Personalization in banking can take various forms:
- Customized financial advice based on personal data analyses.
- Targeted marketing campaigns that align with customer preferences and behaviors.
- Dynamic pricing models for loans and credit products that consider the creditworthiness and history of individual customers.
This evolution is driven by several factors:
- Increased customer expectations in the digital age.
- The desire for banks to differentiate themselves in a crowded market.
As customer-centric approaches proliferate, banks not only enhance customer satisfaction but also cultivate loyalty. A satisfied customer is more likely to continue their banking relationship and recommend the bank to others, leading to increased brand reputation and market share.
Overall, future trends in AI banking solutions are transforming how banks operate, offering both challenges and opportunities. Stakeholders must remain attentive to these trends, investing in technologies that support operational efficiency and foster deeper customer relationships.
"Innovation in banking is no longer optional; it is essential for survival in a rapidly evolving landscape.”
Understanding and implementing these trends is mandatory for financial institutions that aspire to thrive in the age of AI.
Culmination
The conclusion serves as a pivotal section of this article, wrapping up the various aspects of artificial intelligence in banking. Understanding AI's impact on the banking sector is essential to grasp the future of finance.
Summary of Key Findings
Throughout this exploration, the discussion highlighted several crucial points regarding AI implementations in banking.
- Operational Efficiency: Automation and process optimization significantly enhance productivity. Banks can reduce operational costs and streamline workflows using AI technologies.
- Customer Experience: AI-driven chatbots and personalized services improve user engagement and satisfaction, leading to customer loyalty.
- Risk Management: Advanced algorithms for fraud detection and credit scoring provide banks with robust tools to manage risks effectively.
- Regulatory Compliance: Automating compliance processes ensures adherence to regulations while minimizing human error.
- Predictive Analytics: Forecasting consumer behavior and managing investments through AI delivers insights that drive strategic decision-making.
- Challenges and Bias: Addressing data privacy, system integration, and ethical issues is vital for successful AI adoption.
Overall, these findings illustrate how AI not only streamlines operations but also transforms banking into a more customer-centric, efficient, and secure industry.
Final Thoughts on AI in Banking
As we look ahead, the future of banking will likely continue to evolve alongside advancements in AI technology. Banks must remain agile, adapting to the rapid developments within AI. Successful implementation fosters a competitive edge. Moreover, addressing challenges such as data privacy and algorithmic bias remains crucial.
"The integration of AI into banking practices is not merely a trend; it is a significant shift defining the future of finance."
As AI continues to grow, staying informed and engaged with these technological changes will be key for industry professionals and stakeholders in the banking sector.